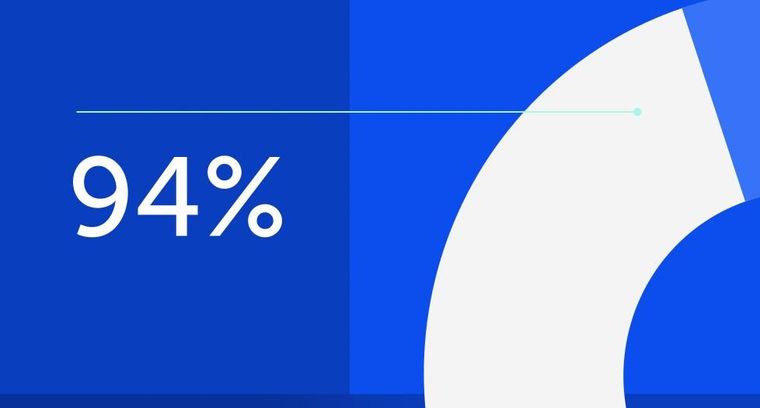
94% of researchers rate our articles as excellent or good
Learn more about the work of our research integrity team to safeguard the quality of each article we publish.
Find out more
ORIGINAL RESEARCH article
Front. Cognit., 01 March 2024
Sec. Learning and Cognitive Development
Volume 2 - 2023 | https://doi.org/10.3389/fcogn.2023.1326191
This article is part of the Research TopicLearning and Memory 2023 - Volume IIView all 30 articles
Introduction: Generalization is fundamental to cognition. In acquired equivalence, two stimuli that share a common association become treated as equivalent, with information acquired about one stimulus generalizing to the other. Acquired equivalence has been thought to rely on integrating related memories as they are encoded, resulting in fast spontaneous generalization, but other studies suggested effortful on-demand recombination of initially separate memories at retrieval. Here, we tested whether the tendency to separate vs. integrate related information may depend on a methodological detail of a traditional acquired equivalence paradigm.
Methods: Human participants underwent feedback-based learning of overlapping face-scene associations, choosing a correct scene for a face from two options on each trial. Foil (incorrect) scenes were controlled for half of the participants to ensure that they can only learn from corrective feedback. The other half had foils selected randomly on each trial, allowing statistical learning of face-scene co-occurrence to supplement feedback-based learning. We hypothesized that the opportunity for statistical learning would boost learning and generalization and facilitate memory integration.
Results: The opportunity for statistical learning increased associative learning and generalization. However, rather than integrated memories, generalization was increased through learning during test.
Discussion: The results indicate that the tendency for generalization in the acquired equivalence is rather small, with no evidence for integrative encoding irrespective of group. The results inform current debates regarding encoding-based vs. retrieval-based mechanisms of generalization. They also highlight how methodological details may alter performance and the involvement of cognitive processes that underlie it.
The groundbreaking work of Pavlov (2010) demonstrated how a response conditioned to a stimulus can be elicited by novel stimuli that are physically similar to the conditioned stimulus. This sparked a wealth of cognitive research focusing on how perceptually similar stimuli excite common elements in the mind and brain, allowing one to generalize a learned behavioral response to new stimuli (Shepard, 1957, 1987; Estes et al., 1964; Hill and Mackintosh, 1976; Rescorla, 1976). While this research program has contributed immensely to our understanding of how conditioned behaviors transfer to novel situations, stimulus generalization does not require physical similarity. Quite early on, Miller and Dollard (1941) argued that the transfer of a response to novel stimuli or contexts does not have to depend on the intrinsic properties of the stimuli but rather can also be achieved via their associative history. The important idea behind associative-based transfer is that stimuli that are not perceptually alike but that share a common associate will be represented as more similar than two stimuli that do not share an associate (Honey and Hall, 1989; Coutureau et al., 2002). In other words, stimuli with a shared associate can become treated as equivalent, or acquire their equivalence. Acquired equivalence has been demonstrated across a variety of stimuli, including faces, abstract symbols, odors, or tones (Honey and Hall, 1989; Stevenson, 2001; Hall et al., 2003; Stevenson et al., 2003). Since its conceptualization, the cognitive mechanisms underlying acquired equivalence have been of broad interest, given that the tendency to generalize across associatively related stimuli can help us understand healthy cognition (Meeter et al., 2009; Doll et al., 2015) as well as cognitive changes in clinical populations (Bódi et al., 2009; Kostek et al., 2014; Foerde and Steinglass, 2017). For example, dementia has been shown to impair (Bódi et al., 2009) while posttraumatic stress disorder enhances (Kostek et al., 2014) acquired equivalence.
The acquired equivalence paradigm, that can be used in both animals and humans, tests spontaneous generalization of associations learned through feedback-based training (Edwards et al., 1982; Honey and Hall, 1991; Meeter et al., 2009; Doll et al., 2015). In a typical acquired equivalence task (Figure 1), participants learn that two cue stimuli (such as two faces, F1 and F2) share a common association (for example, both preferring snowy mountains over a canyon). This establishes the equivalency between the two stimuli. Participants also learn a second preference for one of the cue stimuli (such as F1 preferring the city over the beach). In a critical untrained test trial, reliably choosing the city over the beach for the second face would be indicative of generalization of preferences through acquired equivalence.
Figure 1. The acquired equivalence paradigm. Stimuli are organized into quadruplets, where two cue stimuli (here, Face 1 and Face 2) are associated with two response stimuli (here, Scene 1 and Scene 2). Training includes three of the four possible cue-response associations (F1-S1, F1-S2, F2-S1), learned via feedback-based training. Here, the cue face is presented at the top and the participant needs to decide which of the bottom scenes is preferred by the cue face. Correct preference for each face is highlighted in this figure by a circle, the other scene is a foil from a different quadruplet. Participants receive feedback after each guess. Test includes trained associations and the untrained Face2-Scene2 associations (dashed line, dashed border), with no feedback provided. Response bias (here, the tendency to select city over palms as a preference for Face 2) would indicate spontaneous generalization of preferences across Face 1 and Face 2. Participants are not informed about the structure of the stimuli and they are not told that the test includes preferences they had not studied.
Two classes of mechanisms have been put forth as explanations for acquired equivalence (Figure 2). Encoding-based models suggest that the brain integrates related experiences into a network of associations that link all the elements within a quadruplet together (e.g., F1-F2-S1-S2; Figure 2 Top) at the time of encoding (Shohamy and Wagner, 2008). Presumably, prior associations are reactivated during encoding of related associations, leading to the integration of overlapping associations into a combined memory representation (see also Zeithamova et al., 2012). As a consequence, one should be able to directly retrieve the untrained association (F2-S2) from memory, with the same ease as trained associations. In contrast, retrieval-based models argue that learned associations are encoded separately (F1-S1, F1-S2, F2-S1). Generalization happens on-demand at test, whereby memory traces are sequentially retrieved and recombined in the moment to infer the untrained association (Figure 2 Bottom). Evidence implicating both models has surfaced in the literature.
Figure 2. Memory representations proposed to underly generalization in acquired equivalence. (Top) Related experiences are integrated into a combined memory representation that links all elements together. The red line indicates that even associations that had not been directly encountered are already represented as a part of the integrated representation. (Bottom) Retrieval-based models assume that individual associations are encoded as separate memory representations (blue lines). Generalization judgments for untrained associations can be inferred on-demand by recursive retrieval of individual trained associations, as indicated by the retrieval path marked by black arrows.
Encoding-based theories assume that participants during training form an integrated memory representation that already links all related elements within a quadruplet together (Figure 2). As a consequence, processes needed during the test of the untrained association should be no different from retrieving a trained association. Indeed, a landmark study using the acquired equivalence paradigm (Shohamy and Wagner, 2008) showed that among those with a strong tendency to generalize, reaction times were nearly identical for trained and untrained associations. Furthermore, hippocampal responses during training, but not during the test, were related to generalization performance. As a flip side, integration during encoding may lead to false memories for inferred information because the inferred association becomes and integral part of the stored memory representation (Shohamy and Wagner, 2008; Carpenter and Schacter, 2017, 2018).
While the evidence for integration during encoding in acquired equivalence aligns with evidence for integration in other types of generalization paradigms (Zeithamova et al., 2012; Schlichting et al., 2014; Schlichting and Preston, 2015; Richter et al., 2016; Bowman and Zeithamova, 2018), it has been difficult to replicate. In the most direct attempt, de Araujo Sanchez and Zeithamova (2023) closely followed the procedures from Shohamy and Wagner (2008), with an explicit source memory measure added at the end to test the prediction that generalization leads to false memory. Contrary their prediction, they found that participants were largely aware they were making novel judgments on untrained trials, with no relationship between generalization tendency and false memory (de Araujo Sanchez and Zeithamova, 2023). This particular finding did not directly conflict with the prior study—the relationship between false memory (false belief that one actually studied the untrained association) and generalization in Shohamy and Wagner was only anecdotal, based on an informal debriefing. But other behavioral evidence for integration was also not replicated. de Araujo Sanchez and Zeithamova (2023) found large reaction time cost for untrained trials compared to trained trials that was present even among the top generalizers. The tendency to generalize was on average rather small (around 55%, with 50% being chance), compared to 81% found in Shohamy and Wagner, 2008. As in Shohamy and Wagner (2008), each test trial was repeated 6 times for a better estimate of generalization tendency. When analyzing each repetition separately, the generalization scores were not reliably different from 50% on the first repetition and increased across repeated testing. In other words, the pattern of results was more consistent with retrieval-based accounts, assuming separate representations formed at encoding being linked on-demand during test. Thus, both evidence for integrative encoding and retrieval-based on-demand generalization processes have been found in prior work, without a clear way to reconcile them. Finally, even though most studies using acquired equivalence have not reported analyses that could resolve between encoding-based and retrieval-based accounts, the magnitude of the generalization tendency observed in Shohamy and Wagner (2008) was much larger than any subsequent study (Duncan et al., 2012; Doll et al., 2015; Foerde and Steinglass, 2017; de Araujo Sanchez and Zeithamova, 2023). Thus, conflicting findings regard not only the mechanisms of generalization, but also its prevalence.
In the current paper, we asked what might be the reason for these mixed and seemingly conflicting results. We hypothesized that a nuanced aspect of the procedure may push participants toward separation vs. integration of related experiences, with consequences for learning as well as generalization. To ensure that participants only learn via the corrective feedback, most acquired equivalence studies control for cue-response co-occurrence by “yoking” pairs of quadruplets together (Myers et al., 2003; Meeter et al., 2009; Doll et al., 2015; Foerde and Steinglass, 2017), as illustrated in Figure 3. For example, quadruplet one (F1, F2, S1, S2) is yoked with quadruplet two (F3, F4, S3, S4), such that the correct choices of one quadruplet serve as foils for the other quadruplet and vice versa. During training and test, F1-S1 trials always include not only the same F1 and S1 stimulus, but also the same foil scene S3. For example, the choice may always be between the mountains and the canyon (F1: S1 vs. S3), with the mountains being the correct choice. The same options would also be used for F3 (F3: S1 vs. S3), but now the canyon would be the correct choice for F3. This way, the frequency of co-occurrence is equated between the two choice scenes (F1 was seen equally often with both S1 and S3) and participants can only learn the correct choice based on the corrective feedback. This design also aligns with animal and early human studies that only used two quadruplets (Bódi et al., 2009; Meeter et al., 2009), whose stimuli served as each other's foils, akin the yoked example in Figure 3.
Figure 3. The difference between yoked and non-yoked design. Participants are learning the same quadruplets from overlapping face-scene associations in both conditions, but the conditions differ in the selection of foils for feedback-based training. In the yoked task design (top half), two quadruplets (here, Face1-Face2-Scene1-Scene2 and Face3-Face4-Scene3-Scene4) are “yoked” together to serve as each other's foil stimuli. For example, Face 1-Scene 1 association is trained and tested with Scene 3 always serving as a foil (Face1: Scene1 vs. Scene3, with Scene1 being the correct choice), and Face 3-Scene 3 association is trained and tested with Scene 1 always serving as a foil (Face3: Scene1 vs. Scene3, with Scene3 being the correct choice). As the same foil is repeated across all Face1-Scene1 trials, participants cannot use the frequency of co-occurrence as another learning cue about which face goes with which scene; they can only learn via the corrective feedback. In the non-yoked task design, foil scenes are chosen randomly or pseudo-randomly on each trial. As a consequence, each face is presented most often with its associated scene, and participants can use statistical learning off the frequency of co-occurrence (familiarity of the face-scene pairing) as another cue about which scene is correct.
Specifically, we hypothesized that an opportunity to supplement feedback-based associative learning with statistical learning may lead to faster learning of trained associations and a stronger tendency to integrate related experiences. Notably, participants are assumed to learn the trained associations through feedback. Nevertheless, unless the selection of foil scenes on each 2-alternative forced choice trial is strictly controlled, participants may have another clue available: the frequency of stimulus co-occurrence. For example, if the foil scene is selected randomly on each trial, participants may not only learn the correct scene for each face from the feedback, but may be also learning the associations from frequency statistics, as associated stimuli co-occur more often (Figure 3, Non-yoked design). For example, first F1-S1 training trial may require a choice between mountains and the canyon, second F1-S1 trial mountains and a lagoon, third F1-S1 trial mountains and a field. This offers another route to learning F1-S1 association through statistical learning, as F1 is more often co-occurring with S1 compared to other scenes. As both statistical learning and integrative encoding are thought to rely on the same neural mechanisms (Schapiro et al., 2017), this may not only boost learning of the trained associations but also bias participants toward memory integration, boosting generalization. As Shohamy and Wagner (2008) did not report all the details of the foil selection in their study, it is possible that the strong learning and generalization they observed could be in part because of the contribution of statistical learning, if the foils were indeed varying across repetitions.
We hypothesized that these nuanced details of task design may affect the degree of generalization as well as the degree to which generalization is based on integrative encoding vs. retrieval-based strategies. In contrast to the random foil design where statistical learning can contribute to learning and generalization, we hypothesized that the more controlled yoked design may bias participants toward separate encoding of individual associations to prevent interference (O'Reilly and Rudy, 2001; Kirwan and Stark, 2007; Favila et al., 2016). Specifically, the yoked design necessitates that participants differentiate very similar trials to make the correct choice: S1 should be picked over S3 in context of F1, but S3 should be picked over S1 in context of F3 (Figure 3, Yoked design). Notably, it is important that participants do not integrate across faces F1 and F3 that have distinct preferences, even though those faces are repeatedly presented with the same scene options. Thus, we hypothesized that when foil scenes are strictly controlled to prevent statistical learning, participants may be biased to pattern separate each association, with integrative encoding being less likely.
To test whether it is possible to bias participants toward or away from integration by enabling or preventing statistical learning, we manipulated the structure of foils in the feedback-based learning trials (Figure 3). In the yoked group, foils and correct choices were yoked and participants could only learn face-scene associations through corrective feedback. In the non-yoked group, participants underwent the same training and test structure, but with foil stimuli varying across trial repetitions, giving them the opportunity to supplement the feedback-based training with statistical learning, as the frequency of co-occurrence between each face and its correct scene offered another associative cue. We hypothesized that the opportunity for statistical learning in the non-yoked group will result in faster learning. Furthermore, because statistical learning and memory integration are thought to depend on the same neural mechanisms (Schapiro et al., 2017), we also predicted greater tendency to generalize, as well as greater evidence for integrative encoding in the non-yoked than yoked condition. To foreshadow the results, we found evidence for enhanced learning of trained associations in the non-yoked group, but limited generalization in both groups.
A total of 107 people participated in the study. All were undergraduate students at the University of Oregon who had signed up for the study through SONA for course credit and provided written consent before taking part in the study. The age of participants ranged from 19–24, with a mean of 19 years. When asked about gender, 76 identified as female, 29 as male, and 2 as non-binary. All participants were included in the analyses. Experimental procedures were approved by Research Compliance Services at the University of Oregon.
Stimuli included 16 grayscale images of neutral faces (8 male, 8 female, varying races) and 16 colored scene images, randomly assigned into 8 quadruplets, varying across participants. Within each quadruplet, there were 2 faces (e.g., F1 and F2) associated with 2 scenes (e.g., S1 and S2), resulting in four possible face-scene associations within each quadruplet: F1-S1, F2-S1, F1-S2, and F2-S2. Participants were trained through corrective feedback on three of the face-scene associations (F1-S1, F2-S1, F1-S2), and then tested without feedback on all four (Figure 1).
We employed a between-subjects procedure in which participants completed one of two versions of the acquired equivalence task, differing only in the way the foil stimuli were controlled. For both conditions, the experiment consisted of (1) pre-exposure to all facial stimuli, (2) feedback-based training of the three premise associations, and (3) a test for generalization via acquired equivalence that included both trained and untrained associations. In both training and testing, participants were shown a face along with two scenes for each trial and asked to choose the scene that correctly corresponded to the presented face. In the yoked condition, the foil scene was consistent across trials and thus co-occurred with the cue at the same frequency as the correct scene. In the non-yoked condition, the incorrect scene changed pseudo-randomly with each iteration. The stimuli, number of trials, presence of feedback, and number of trial iterations were equal across conditions. Complete details of the two conditions are provided below.
Participants first underwent pre-exposure, in which they successively viewed the 16 faces that would be shown to them during the task. Participants were not asked to do anything but familiarize themselves with the stimuli. Each face was shown three times in a random order, presented one-by-one for 2 s and followed by a 1 s fixation cross that appeared between them. This phase of the experiment was included for participants to familiarize themselves with the stimuli (Shohamy and Wagner, 2008) and no data was collected.
Participants were then trained on three of the four face-scene associations (F1-S1, F1-S2, and F2-S1) from each quadruplet, using corrective feedback given after each trial. For each trial, participants were presented with a face at the top of the screen and two choice scenes (a correct scene and a foil scene) below it. Stimuli stayed on the screen for 3 s, during which participants had to select the scene that correctly corresponded to the presented face. Participants were then shown feedback based on their response (“Correct!,” “Incorrect!,” or “Too late!”) for 1 s, which was then followed by a 1 s fixation cross. Each association was presented 7 times in random order, resulting in a total of 168 training trials (8 quadruplets × 3 trained associations in each × 7 repetitions). To ensure that participants were learning the associations and not relying on spatial cues to infer the answer, correct scenes were shown about an equal number of times on the left and right side of the screen.
To see how the absence of yoking and the opportunity to use statistical learning may affect acquired equivalence, the stimuli and procedure were the same for both conditions except for the way in which the foil scene was controlled (Figure 3).
In the yoked task version, each quadruplet was yoked to another quadruplet to serve as each other's foils (Bowman et al., 2021; de Araujo Sanchez and Zeithamova, 2023). This meant that the foil for each face was the same across all repetitions of the same trial type. For example, all F1-S1 trials consisted of F1: S1 vs. S3 choice, with only the left-right presentation of scenes randomized across trials. It also meant that the same two scenes switched roles across trials. For example, all F3-S3 trials consisted of F3: S1 vs. S3 choice, but the correct option would now be S3 rather than S1. This way, participants had to learn via the corrective feedback as the frequency of face-scene co-occurrence was equated between the correct choice and the foil. Furthermore, participants could not just remember which scene among two they should pick without considering the associated face, which could be the case if foil assignment was not reciprocal.
In the non-yoked version, the foil was not consistent across trials but rather was a pseudo-randomly selected scene from a different quadruplet that changed with each iteration. The foils were constrained to be scenes with an analogous position in another quadruplet. For example, for S1, only the shared scenes from other quadruplets (S3, S5, S7, etc.) would be used as foils. To make the non-yoked condition maximally distinct from the yoke condition and maximize the statistical learning opportunity, the foils never repeated across trials in the current study, rather than being completely random. This was also the reason why training in the current study included 7 repetitions of each trained associations, rather than 8 used in the studies that directly inspired us (Shohamy and Wagner, 2008; de Araujo Sanchez and Zeithamova, 2023).
After training, participants were tested on the three premise associations learned during training, as well as the fourth, untrained association F2-S2 (Figures 1, 3). The tendency to choose S2 for the F2 face is the key measure of generalization in this task. Although we will sometimes refer to the proportion of S2 responses to F2 cue face as “proportion correct,” the participants are never told what is expected of them on the untrained trials (or even that there are untrained trials included), so there is not an objectively correct response on those trials. Just like in training, stimuli were presented for 3 s and followed by a 1 s fixation cross. To be consistent with prior acquired equivalence studies and to be able to look for learning-during-test (Shohamy and Wagner, 2008; de Araujo Sanchez and Zeithamova, 2023), each test trial was repeated 6 times, resulting in a total of 192 test trials (8 quadruplets × 4 associations in each × 6 repetitions). As during study, the control of the foils during test differed between groups, with the yoked group continuing the yoked procedure and the non-yoked group being tested using foils pseudo-randomly varying across repetitions. The order of the trials within each repetition block was randomized and the correct scenes were presented on either side of the screen an equal number of times. Corrective feedback was not given during test.
For all statistical analyses, we report results from all participants. There were, however, eight participants in the non-yoked group and fifteen participants in the yoked group that demonstrated no learning during the training phase (<57% correct, cutoff determined using a one-tailed binomial test against chance). To ensure that the results are robust with respect to the inclusion criterion, we verified that the results of each analysis would be comparable had we excluded non-learners. Any differences are noted at the end of each analysis.
To evaluate training performance, we first calculated the average overall accuracy for each participant in both groups and compared the group averages against chance levels. To evaluate training performance over time in both groups, we split training into mean accuracy for each block and entered these scores into a repeated measures ANOVA between groups.
To evaluate testing performance, we first calculated the average overall accuracy for trained trials and generalization scores on untrained trials for each participant in both groups and compared the group averages against chance levels. We tested for group differences in performance on trained and untrained trials using a mixed ANOVA with trial type (trained, untrained) as a within-subject factor and group (yoked, non-yoked) as a between-subject factor.
We have previously observed learning during test, consistent with the notion that generalization can rely on on-demand recursive retrieval of individual trained associations to inform untrained trials. Moreover, the non-yoked group would have an opportunity to continue utilizing statistical learning based on co-occurrence frequency, including on the untrained trial. Thus, here we tested for a learning effect during the testing phase by running a 2 × 2 × 6 mixed ANOVA (2 groups × 2 trial types × 6 testing blocks).
As response times can provide important insight into the cognitive processes underlying decision-making in the acquired equivalence paradigm (Shohamy and Wagner, 2008; de Araujo Sanchez and Zeithamova, 2023), we compared raw response times in both groups for trained and untrained trials (via a 2 × 2 repeated measures ANOVA) during the testing phase. An interaction between group and trial type showing smaller response time cost on untrained trials for the non-yoked group would be consistent with a greater reliance on integration during encoding in that group.
Strong behavioral evidence for encoding-based models of acquired equivalence came from Shohamy and Wagner (2008), who showed that untrained test trials incurred minimal response time costs when generalization was high. To obtain response time costs for each participant, we took the log transformed mean response times on correct trained test trials and subtracted it from the log transformed mean response times on correct untrained test trials. Small response time cost would indicate that untrained trials were not substantially more demanding than trained trials, as would be expected if all the stimuli within a quadruplet were already integrated into a combined memory representation during encoding (Shohamy and Wagner, 2008). We then ran a Pearson correlation test between response time costs and generalization scores to replicate the analysis of Shohamy & Wagner reporting a strong negative correlation, with minimal response time cost observed for those with strong generalization tendency. We performed this analysis for both groups and corrected for multiple comparisons by setting alpha equal to 0.025.
The proportion of correct responses for each participant during each block of training is presented in Figure 4. We found that the average accuracy for both the yoked (μ = 62%, SD = 9%) and non-yoked (μ = 69%, SD = 10%) group was well above chance (yoked: t(51) = 9.33, 95% CI = [0.59, 0.65], d = 1.29, p < 0.001; non-yoked: t(54) = 13.97, 95% CI = [0.66, 0.71], d = 1.88, p < 0.001). To test for an effect of training block, we entered mean accuracy per participant for each block into a 2 (group: yoked, non-yoked) × 7 (repetitions) mixed effects ANOVA. As seen in Figure 4, participants across conditions demonstrated increased accuracy across iterations of the premise associations during training [main effect of repetition, F(6, 630) =132.88, p < 0.001, = 0.56]. These results show that participants were paying attention and were trying to learn the task. The main effect of group (yoked vs. non-yoked) was also significant [F(6, 630) = 13.5, = 0.11, p < 0.001], indicating that accuracy for premise associations during training was greater when the structure of foils allowed for statistical learning to supplement feedback-based learning. The degree of improvement across iterations during training was similar across conditions [interaction F(6, 630) = 1.14, = 0.01, p = 0.339]. To summarize, participants were learning the task, improving with time, though the opportunity for statistical learning in non-yoked condition led to better training accuracy than observed when foils were strictly controlled in the yoke condition. These results from training do not change qualitatively after excluding non-learners.
Figure 4. Training performance. Proportion of all trials that were correct across training blocks. The y-axis denotes accuracy while the x-axis denotes block. Color dissociates the yoked (teal) and non-yoked (orange) groups. Thick lines represent group averages and thin lines with dots represent individual performance. Error bars represent ± SEM (standard error of the mean).
The proportion of responses that were correct for each group and each trial type are displayed in Figure 5. Please note that untrained trials do not technically have a correct response, though for simplicity, we refer to responses that demonstrate acquired equivalence as “correct.” We were interested in uncovering differences in testing accuracy between groups for each association type (trained vs. untrained). In addition to the main effect of group and trial type, we hypothesized that there would be a disproportionate group difference in untrained trial performance, meaning we expected to see an interaction between group and association type. We observed a main effect of association type [F(1, 105) = 222.81, = 0.68, p < 0.001], indicating that both groups were more accurate for trained than untrained associations. There was also a main effect of group [F(1, 105) = 41.9, = 0.29, p < 0.001], meaning the non-yoked group performed overall better than the yoked group during testing. Finally, we observed a marginal group-by-association type interaction [F(1, 105) = 3.29, = 0.03, p = 0.072]. To unpack this interaction, we ran independent sample t-tests, which showed that the non-yoked group scored significantly higher than the yoked group on both trained [t(105) = 3.77, 95% CI = [0.05, 0.15], d = 0.73, p < 0.001] and untrained [t(105) = 6.68, 95% CI = [0.11, 0.20], d = 1.29, p < 0.001], but the effect size was numerically greater for untrained trial. This result suggests that participants in the non-yoked group exhibited acquired equivalence to a greater degree than participants in the yoked group, above and beyond of the difference in learning the training associations. After excluding non-learners, main effects of association type and group remain the same, and the association type-by-group interaction becomes significant, strengthening the claim that the non-yoked group exhibited acquired equivalence to a degree that is not explained by differences in learning the training associations.
Figure 5. Testing performance. Mean proportion correct for trained associations and mean proportion of responses consistent with acquired equivalence for untrained associations. Dots are individual participants. Upper and lower boxplot hinges denote the 75th and 25th percentiles and the middle hinge is the median accuracy. Whiskers extend to the minimum and maximum scores. Half violin plots are accuracy distributions.
The marginal interaction between trial type and group suggests that the generalization differences between groups are greater than the differences in accuracy for trained trials. In other words, the opportunity for statistical learning may have boosted generalization above and beyond what would be expected from just having better memory for trained trials. Furthermore, while generalization in the non-yoked condition did not reach the levels observed in Shohamy and Wagner (2008), the memory for trained associations was also lower. Thus, to further compare generalization tendency between the yoked and non-yoked group, and between the non-yoked group and the prior report of strong generalization (Shohamy and Wagner, 2008) while taking account the differences observed for trained trials, we performed two control analyses (Figure 6). Both analyses focused on controlling for direct performance at test. The first approach was to limit the analyses to untrained trials for which all the corresponding trained trials were remembered well. Given that there were 6 testing blocks, we set our inclusion criterion to at least 5 out of the 6 responses correct for each trained association from a given quadruplet. When only considering untrained trials for which trained associations were remembered (Figure 6A), the yoked group's mean generalization on untrained trials rose to 55% (SD = 27%), while in non-yoked group, generalization rose to 67% (SD = 15%). As the difference remained significant [t(86) = 2.76, 95% CIdifference = [0.04, 0.22], d = 0.59, p = 0.007], this analysis again suggests that group differences in generalization are not entirely explainable by differences in memory for trained associations. Yet, the non-yoked group still did not generalize to the same degree as observed in Shohamy and Wagner (2008).
Figure 6. Performance on untrained trials while controlling for trained accuracy. (A) Generalization scores limited to untrained trials for which the participants remembered the corresponding premise associations at test. (B) Test performance on trained and untrained trials after excluding participants that scored below 80% on trained test trials. In both plots, the y-axis is the accuracy for trained trials or the proportion of generalization-consistent responses on untrained trials; x-axis denotes trial type, and color denotes group assignment.
The second control analysis involved excluding all participants whose test performance was below 80% on trained trials. The goal was to only include participants whose memory for trained associations was comparable to the participants reported in Shohamy and Wagner (2008). This led to the exclusion of 49 participants, resulting in a subsample size of n = 58 (19 in the yoked group, 39 in the non-yoked group; Figure 6B). By only taking participants that displayed robust premise knowledge, we should be again able to evaluate if the structure of foil selection affects generalization across related experience above and beyond its effect on learning per se. As can be seen in Figure 6B, there were large individual differences in the tendency to generalize even among those who remembered the trained associations well. To compare group averages, a 2 × 2 ANOVA with group as a between subject factor and trial type as a within subject factor showed a main effect of association type [F(1, 56) = 336.7, = 0.86, p < 0.001] and group [F(1, 56) = 20.5 = 0.27, p < 0.001], as well as a significant interaction [F(1, 56) = 21.4, = 0.28, p < 0.001]. Follow up pair-wise comparisons between groups showed comparable memory for trained associations [t(56) = 1.04, 95% CIdifference = [−0.01, 0.04], d = 0.29, p = 0.303], but significantly greater generalization in the non-yoked group compared to the yoked group [t(56) = 4.90, 95% CI = [0.10, 0.24], d = 1.37, p < 0.001]. Thus, the difference in generalization between groups cannot be fully explained by differential knowledge of premise associations. Notably, even among the strong learners in the yoked group, the average tendency to generalize on untrained trials did not exceed chance, with some participants reliably choosing scenes not associated with the pairmate from the same quadruplet (Figure 6B).
In our prior study using yoked design, we found little evidence for generalization on the first test trial for each untrained association that then slightly but reliably increased across repetitions (de Araujo Sanchez and Zeithamova, 2023). This is possible for untrained trials as participants can be linking the initially separate trained memories on-demand at test. Here, we hypothesized that learning during test may be even more pronounced in the non-yoked group that could additionally utilize statistical learning. Specifically, because the foil scene varied across test repetitions in the non-yoked group, these participants could use statistical learning also during the test phase which could further boost their generalization scores. To test for this possibility, we ran a 2 groups × 2 trial types × 6 repetitions mixed design ANOVA on the generalization scores for untrained trials (Figure 7). The full ANOVA results are reported in Table 1. There was a main effect of group, a main effect of trial type, and a marginal group-by-trial type interaction, all of which reiterates the analysis reported in the “Testing” section above. Of main interest was the effect of block and any interactions with block. The main effect of block did not reach significance. However, there was a block X group, block X trial type, as well as a three-way block X group X trial type interaction.
Figure 7. Learning during test. Testing performance for trained (A) and untrained (B) trial types for each testing block. Color denotes the yoked (teal) and non-yoked (orange) between-subjects conditions. Thick lines represent group averages and thin lines with dots represent individual performance. Error bars denote ± SEM.
As illustrated in Figure 7, performance was relatively stable across test repetitions for trained trials (β = −0.003, p = 0.351), but increased across repetitions for untrained trials, as confirmed by a significant linear trend across testing blocks for untrained trials (β = 0.01, p = 0.011). However, the increase across untrained trials was driven specifically by the non-yoked group, which started the first block of testing with generalization scores only marginally above chance [t(54) = 1.96, 95% CI = [0.49, 0.59], d = 0.26, p = 0.055] and then increased accuracy across repeated testing. In contrast, the generalization tendency for the yoked group remained approximately at chance throughout the testing phase (linear trend β = −0.005, p = 0.310). All main effects and interactions remain qualitatively unchanged after excluding non-learners.
The mean response time for each group and trial is shown in Figure 8. A 2 × 2 mixed ANOVA showed a main effect of trial type on response time [F(1, 105) = 170.6, = 0.62, p < 0.001], with response times faster for trained than untrained trials. There was no effect of group [F(1, 105) = 0.378, <0.01, p = 0.540], but there was a group X trial type interaction [F(1, 105) = 15.1, = 0.125, p < 0.001]. Post-hoc group comparisons revealed that the interaction was driven by significantly faster response times in the non-yoked group than yoked group on trained trials [t(105) = 2.09, 95% CI = [0.01, 0.18], d = 0.40, p = 0.039], but comparable response times across groups for untrained trials [t(105) = −0.13, 95% CI = [−0.12, 0.10], d = 0.03, p = 0.893]. Thus, contrary to our hypothesis, there was no evidence that untrained trials would be less effortful for non-yoked compared to the yoked group. All results were replicated after excluding non-learners.
Figure 8. Response time differences. Raw response times for each group and trial type. Y-axis represents response times in seconds and the x-axis denotes trial type. Dots are individual participants and color denotes group. Error bars are ± SEM.
To try and tease apart generalization strategies, we computed response time costs (for correct untrained test trials minus correct trained test trials) for every participant and then correlated response time costs with acquired equivalence (generalization) scores. A striking negative correlation (r = −0.69) was observed in Shohamy and Wagner (2008), where those with the highest generalization scores showed minimal reaction time cost, as would be predicted if generalization relied on integrated memories formed during encoding. Although the current study was not a priori powered for analysis of individual differences, we wanted to see whether this finding can be numerically replicated, at least in the non-yoked condition. Post-hoc power analysis indicated that the current sample size (N > 50 in each group) would be sufficient to detect correlations with absolute r > 0.33. The results are presented in Figure 9. In the yoked condition, we found no relationship between response time cost and generalization (r = −0.06, p = 0.661), replicating the near-zero correlation found with a much larger sample in de Araujo Sanchez and Zeithamova (2023). In the non-yoked condition, where we expected greater evidence for integrative encoding, we instead found a non-significant, numerically positive correction (r = 0.19, p = 0.155), indicating that greater generalization success came at the cost of increased effort. Thus, the reaction time analyses did not find any evidence that integration would be greater in the non-yoked condition than yoked condition. These results were replicated after we excluded non-learners.
Figure 9. Relationship of response time costs and acquired equivalence. Correlations between response time cost and acquired equivalence scores for yoked (teal) and non-yoked (orange) group. Dots represent individual participants. Ribbons denote 95% confidence intervals around the best fitting line.
The transfer of previously acquired knowledge to novel stimuli can be facilitated by intrinsic properties of stimuli, such as their physical characteristics (Shepard, 1957, 1958, 1987; Rescorla, 1976; Pavlov, 2010). But transfer, or generalization, of a learned response to a new stimulus does not require physical similarity and instead can be based on a shared response history or associative relation. The acquired equivalence paradigm has been an important paradigm used to measure the spontaneous tendency to generalize across associatively related stimuli as it can be used in both animal and human studies. Moreover, the patterns of responses and reaction times in this paradigm have been used to elucidate the cognitive processes and memory representations underlying relational generalization—integrative encoding or on-demand inference from separate representations. Even though findings in individual studies have provided compelling evidence for one or the other mechanism, they have been conflicting across studies. We hypothesized that this discrepancy in the literature could arise from subtle methodological differences of the feedback-based paradigm, such as the selection of foils on each trial. Specifically, we predicted that memory integration and generalization via acquired equivalence would be boosted when foils are selected randomly, providing an opportunity to supplement feedback-based learning with statistical learning of stimulus co-occurrence. The results were mixed with respect to our hypothesis. We found that the opportunity for statistical learning boosted training and test performance, but the boost to generalization was driven by learning during test rather than memory integration. The findings provide additional support to retrieval-based theories of generalization and highlight how subtle differences in methodological procedures can modulate the cognitive processes involved in a seemingly identical task.
We found ample evidence for overall better learning when people have the opportunity for statistical learning, for participants in the non-yoked group indeed performed better during both training and testing. Furthermore, this boost was disproportionately large for untrained test trials, even when controlling for the differences in learning of trained associations. Having found that statistical learning increased generalization, we returned to the original question that Shohamy and Wagner (2008) attempted to answer: whether generalization through acquired equivalence was enacted through integrative encoding or retrieval-based mechanisms. Encoding-based acquired equivalence assumes that the premise associations become integrated within a single network of associations. As such, integrated memories should lead to a high degree of generalization and minimal response time cost for untrained trials, as they would involve a simple retrieval of associations already formed during encoding. Contrary to this hypothesis, we found large reaction time cost for untrained trials in both groups, and no evidence that higher generalization would be associated with smaller reaction time cost. Moreover, we found no evidence for generalization through acquired equivalence in the first block of testing phase for either group, yet the encoding hypothesis assumes that knowledge of the untrained association should be present prior to testing.
Retrieval-based models assume that individual overlapping experiences are encoded into separate representations, but those separate memories can be recursively recalled and recombined to infer new information (Kumaran and McClelland, 2012; Banino et al., 2016). When the recursive recall and inference takes place on-demand at test, it would be expected to be more effortful than direct retrieval and incur longer response times, as observed here. While some differences in response latencies between trained and untrained trials could be expected even for integrated memories because of differential memory strength, the fact that the response time cost was not decreased for strong generalizers contradicts the prediction of integrative encoding. Interestingly, the current data do not show much evidence for retrieval-based inference either. Because the output of the retrieval-based inference can get re-encoded back to the hippocampus (Koster et al., 2018), participants may continue linking trained associations to infer untrained associations during test, thereby increasing the likelihood of generalization responses with every subsequent test. This was observed as learning during test for untrained associations by de Araujo Sanchez and Zeithamova (2023), even though all their experiments included yoked design preventing statistical learning. In contrast, here both groups performed at chance level for untrained associations during the first block of testing, and the yoked group remained at chance throughout test phase. These results may indicate a lack of tendency for acquired equivalence in both groups. Although the non-yoked group did show reliable learning during test and above chance generalization when averaged across the testing phase, this could result from statistical learning alone, as the untrained face-scene pairings became more and more familiar across repeated testing.
In conclusion, we found that the opportunity for statistical learning offered by less controlled foil selection in the non-yoked condition led to better training performance of premise associations as well as greater acquired equivalence scores. However, contrary to our prediction, we found no evidence for integrative encoding in either task version. While the conflicting findings in the literature remain unresolved, our study highlights the challenges with the assumption that a specific task (such as the acquired equivalence paradigm) measures a specific process of interest (such as generalization through acquired equivalence). In addition to the potential role of paradigm details in enabling or preventing a contribution of other cognitive processes, these challenges further highlight the benefits of including additional analyses or measures that can corroborate accuracy measures and resolve between competing interpretations (Shohamy and Wagner, 2008; Schlichting et al., 2014; Carpenter and Schacter, 2017, 2018; de Araujo Sanchez and Zeithamova, 2023).
The datasets presented in this study can be found in online repositories. The names of the repository/repositories and accession number(s) can be found below: https://osf.io/7kje8/.
The studies involving humans were approved by the University of Oregon Institutional Review Board. The studies were conducted in accordance with the local legislation and institutional requirements. The participants provided their written informed consent to participate in this study.
TH: Data curation, Formal analysis, Investigation, Visualization, Writing – original draft. LK: Data curation, Formal analysis, Investigation, Visualization, Writing – original draft. DZ: Conceptualization, Formal analysis, Funding acquisition, Investigation, Methodology, Project administration, Resources, Supervision, Validation, Visualization, Writing – review & editing.
The author(s) declare financial support was received for the research, authorship, and/or publication of this article. This work was supported by the National Institute for Neurological Disease and Stroke Grant R01-NS-112366 (DZ).
The authors declare that the research was conducted in the absence of any commercial or financial relationships that could be construed as a potential conflict of interest.
All claims expressed in this article are solely those of the authors and do not necessarily represent those of their affiliated organizations, or those of the publisher, the editors and the reviewers. Any product that may be evaluated in this article, or claim that may be made by its manufacturer, is not guaranteed or endorsed by the publisher.
Banino, A., Koster, R., Hassabis, D., and Kumaran, D. (2016). Retrieval-based model accounts for striking profile of episodic memory and generalization. Sci. Rep. 6, 31330. doi: 10.1038/srep31330
Bódi, N., Csibri, É., Myers, C. E., Gluck, M. A., and Kéri, S. (2009). Associative learning, acquired equivalence, and flexible generalization of knowledge in mild Alzheimer disease. Cogn. Behav. Neurol. 22, e318192ccf0. doi: 10.1097/WNN.0b013e318192ccf0
Bowman, C. R., de Araujo Sanchez, M. A., Hou, W., Rubin, S., and Zeithamova, D. (2021). Generalization and false memory in an acquired equivalence paradigm: the influence of physical resemblance across related episodes. Front. Psychol. 12, 669481. doi: 10.3389/fpsyg.2021.669481
Bowman, C. R., and Zeithamova, D. (2018). Abstract memory representations in the ventromedial prefrontal cortex and hippocampus support concept generalization. J. Neurosci. 38, 2605–2614. doi: 10.1523/JNEUROSCI.2811-17.2018
Carpenter, A. C., and Schacter, D. L. (2017). Flexible retrieval: when true inferences produce false memories. J. Exper. Psychol. 43, 340. doi: 10.1037/xlm0000340
Carpenter, A. C., and Schacter, D. L. (2018). False memories, false preferences: Flexible retrieval mechanisms supporting successful inference bias novel decisions. J. Exper. Psychol. 147, 391. doi: 10.1037/xge0000391
Coutureau, E., Killcross, A. S., Good, M., Marshall, V. J., Ward-Robinson, J., and Honey, R. C. (2002). Acquired equivalence and distinctiveness of cues: II. Neural manipulations and their implications. J. Exper. Psychol. 28, 388–396. doi: 10.1037/0097-7403.28.4.388
de Araujo Sanchez, M. A., and Zeithamova, D. (2023). Generalization and false memory in acquired equivalence. Cognition 234, 105385. doi: 10.1016/j.cognition.2023.105385
Doll, B. B., Shohamy, D., and Daw, N. D. (2015). Multiple memory systems as substrates for multiple decision systems. Neurobiol. Lear. Memory 117, 4–13. doi: 10.1016/j.nlm.2014.04.014
Duncan, K., Sadanand, A., and Davachi, L. (2012). Memory's Penumbra: episodic memory decisions induce lingering mnemonic biases. Science 337, 1221936. doi: 10.1126/science.1221936
Edwards, C. A., Jagielo, J. A., Zentall, T. R., and Hogan, D. E. (1982). Acquired equivalence and distinctiveness in matching to sample by pigeons: mediation by reinforcer-specific expectancies. J. Exper. Psychol. 8, 244–259. doi: 10.1037/0097-7403.8.3.244
Estes, W. K., Luce, R. D., Bush, R. R., and Galanter, E. (1964). Handbook of Mathematical Psychology, Volume I. J. Am. Statist. Assoc. 59, e2283012. doi: 10.2307/2283012
Favila, S. E., Chanales, A. J. H., and Kuhl, B. A. (2016). Experience-dependent hippocampal pattern differentiation prevents interference during subsequent learning. Nat. Commun. 7, 11066. doi: 10.1038/ncomms11066
Foerde, K., and Steinglass, J. E. (2017). Decreased feedback learning in anorexia nervosa persists after weight restoration. Int. J. Eating Disor. 50, 22709. doi: 10.1002/eat.22709
Hall, G., Mitchell, C., Graham, S., and Lavis, Y. (2003). Acquired equivalence and distinctiveness in human discrimination learning: evidence for associative mediation. J. Exp. Psychol. 132, 266–276. doi: 10.1037/0096-3445.132.2.266
Hill, W. F., and Mackintosh, N. J. (1976). The psychology of animal learning. Am. J. Psychol. 89, 1421765. doi: 10.2307/1421765
Honey, R. C., and Hall, G. (1989). Acquired equivalence and distinctiveness of cues. J. Exper. Psychol. 15, 338–348. doi: 10.1037/0097-7403.15.4.338
Honey, R. C., and Hall, G. (1991). Acquired equivalence and distinctiveness of cues using a sensory-preconditioning procedure. Quart. J. Exper. Psychol. B 43, 121–135.
Kirwan, C. B., and Stark, C. E. L. (2007). Overcoming interference: an fMRI investigation of pattern separation in the medial temporal lobe. Learn. Memory 14, 625–633. doi: 10.1101/lm.663507
Kostek, J. A., Beck, K. D., Gilbertson, M. W., Orr, S. P., Pang, K. C. H., Servatius, R. J., et al. (2014). Acquired equivalence in U.S. veterans with symptoms of posttraumatic stress: reexperiencing symptoms are associated with greater generalization. J. Traum. Stress 27, 717–720. doi: 10.1002/jts.21974
Koster, R., Chadwick, M. J., Chen, Y., Berron, D., Banino, A., Düzel, E., et al. (2018). Big-loop recurrence within the hippocampal system supports integration of information across episodes. Neuron 99, 9. doi: 10.1016/j.neuron.2018.08.009
Kumaran, D., and McClelland, J. L. (2012). Generalization through the recurrent interaction of episodic memories: a model of the hippocampal system. Psychol. Rev. 119, 573–616. doi: 10.1037/a0028681
Meeter, M., Shohamy, D., and Myers, C. E. (2009). acquired equivalence changes stimulus representations. J. Exper. Anal. Behav. 91, 127–141. doi: 10.1901/jeab.2009.91-127
Myers, C. E., Shohamy, D., Gluck, M. A., Grossman, S., Kluger, A., Ferris, S., et al. (2003). Dissociating hippocampal versus basal ganglia contributions to learning and transfer. J. Cogn. Neurosci. 15, 185–193. doi: 10.1162/089892903321208123
O'Reilly, R. C., and Rudy, J. W. (2001). Conjunctive representations in learning and memory: principles of cortical and hippocampal function. Psychol. Rev. 108, 311–345. doi: 10.1037/0033-295X.108.2.311
Pavlov, I. P. (2010). Conditioned reflexes: an investigation of the physiological activity of the cerebral cortex. Ann. Neurosci. 17, 309. doi: 10.5214/ans.0972-7531.1017309
Rescorla, R. A. (1976). Stimulus generalization: some predictions from a model of Pavlovian conditioning. J. Exper. Psychol. 2, 88–96. doi: 10.1037/0097-7403.2.1.88
Richter, F. R., Chanales, A. J. H., and Kuhl, B. A. (2016). Predicting the integration of overlapping memories by decoding mnemonic processing states during learning. NeuroImage 124, 51. doi: 10.1016/j.neuroimage.2015.08.051
Schapiro, A. C., Turk-Browne, N. B., Botvinick, M. M., and Norman, K. A. (2017). Complementary learning systems within the hippocampus: a neural network modelling approach to reconciling episodic memory with statistical learning. Philos. Trans. R. Soc. B. 372, 1711. doi: 10.1098/rstb.2016.0049
Schlichting, M. L., and Preston, A. R. (2015). Memory integration: neural mechanisms and implications for behavior. Curr. Opin. Behav. Sci. 1, 1–18. doi: 10.1016/j.cobeha.2014.07.005
Schlichting, M. L., Zeithamova, D., and Preston, A. R. (2014). CA1 subfield contributions to memory integration and inference. Hippocampus 24, 22310. doi: 10.1002/hipo.22310
Shepard, R. N. (1957). Stimulus and response generalization: a stochastic model relating generalization to distance in psychological space. Psychometrika 22, 88967. doi: 10.1007/BF02288967
Shepard, R. N. (1958). Stimulus and response generalization: tests of a model relating generalization to distance in psychological space. J. Exper. Psychol. 55, 42354. doi: 10.1037/h0042354
Shepard, R. N. (1987). Toward a universal law of generalization for psychological science. Science 237, 1317–1323. doi: 10.1126/science.3629243
Shohamy, D., and Wagner, A. D. (2008). Integrating memories in the human brain: hippocampal-midbrain encoding of overlapping events. Neuron 60, 23. doi: 10.1016/j.neuron.2008.09.023
Stevenson, R. J. (2001). The acquisition of odour qualities. Q. J. Exp. Psychol. Sec. A 54, 561–77. doi: 10.1080/713755972
Stevenson, R. J., Case, T. I., and Boakes, R. A. (2003). Smelling what was there: acquired olfactory percepts are resistant to further modification. Learn. Motiv. 34, 185–202. doi: 10.1016/S0023-9690(03)00002-X
Keywords: acquired equivalence, integrative encoding, retrieval-based inference, statistical learning, memory
Citation: Houser TM, Krantz L and Zeithamova D (2024) Retrieval-based inference in the acquired equivalence paradigm. Front. Cognit. 2:1326191. doi: 10.3389/fcogn.2023.1326191
Received: 24 October 2023; Accepted: 18 December 2023;
Published: 01 March 2024.
Edited by:
Brock Kirwan, Brigham Young University, United StatesReviewed by:
Catherine E. Myers, United States Department of Veterans Affairs, United StatesCopyright © 2024 Houser, Krantz and Zeithamova. This is an open-access article distributed under the terms of the Creative Commons Attribution License (CC BY). The use, distribution or reproduction in other forums is permitted, provided the original author(s) and the copyright owner(s) are credited and that the original publication in this journal is cited, in accordance with accepted academic practice. No use, distribution or reproduction is permitted which does not comply with these terms.
*Correspondence: Dagmar Zeithamova, ZGFzYUB1b3JlZ29uLmVkdQ==
†These authors have contributed equally to this work
Disclaimer: All claims expressed in this article are solely those of the authors and do not necessarily represent those of their affiliated organizations, or those of the publisher, the editors and the reviewers. Any product that may be evaluated in this article or claim that may be made by its manufacturer is not guaranteed or endorsed by the publisher.
Research integrity at Frontiers
Learn more about the work of our research integrity team to safeguard the quality of each article we publish.