- Department of Psychology, University of Oregon, Eugene, OR, United States
It is known that animals foraging in the wild must balance their levels of exploitation and exploration so as to maximize resource consumption. This usually manifests as an area-restricted search strategy, such that animals tend to exploit environmental patches and make long excursions between patches. This optimal foraging strategy, however, relies on an underlying assumption: nearby locations yield similar resources. Here, we offer an explanation as to how animals utilize this assumption, which implicitly involves generalization. We also describe the computational mechanisms hypothesized to incorporate factors of exploitation, exploration, and generalization, thus, providing a more holistic picture of animal search strategies. Moreover, we connect this foraging behavior to cognition in general. As such, we suggest that cognitive processes, particularly those involved in sequential decision-making, reuse the computational principles grafted into neural activity by the evolution of optimal foraging. We speculate as to what neurobiological substrates may be using area-restricted search, as well as how a model of exploitation, exploration, and generalization can inform psychopathology.
Introduction
Generalization is the use of previously acquired knowledge in novel situations (Taylor et al., 2021). The notion that the response to novel stimuli is a decaying exponential function of physical similarity to learned stimuli has been posited as a first principle of psychology, or the universal law of generalization (Shepard, 1987), and indeed this notion has withstood the test of time and rigorous experimentation across species and cognitive domains (Sims, 2018). The significance of generalization is apparent in everyday life: By generalizing, we know where to look for the milk in a new grocery store, we know what restaurants might be good in a new city, we know how long to wait for the bus at a new bus stop, etc. In fact, generalization may very well be ubiquitous in human cognitive processes given that we must always generalize from the past to the future (Stephen and Bergson, 2018). While its utility and characteristics are well-described, the origins and evolutionary functions of generalization are less understood. It is important to understand the evolutionary basis for generalization as it might shed light on the neurobiological mechanisms supporting generalization, as well as inform the treatment of a number of psychiatric disorders that show signs of maladaptive generalization processes. In this paper, we outline a conceptual framework that is meant to illustrate how generalization plays an integral role in the tradeoff between exploration and exploitation which is itself a result of optimal foraging.
How animals forage optimally and resolve the exploration-exploitation dilemma in doing so has become a topic of recently revivified interest (Hunt et al., 2021). One reason for this recent surge is the realization that the exploration-exploitation dilemma is ubiquitous in decision-making. In sequential decision-making tasks, where options are considered serially, subjects must choose whether to engage with the current option or search for a better option (Hayden et al., 2011; Kolling et al., 2012, 2016). Sequential decision-making maps on nicely to the exploration-exploitation dilemma, where choosing the current option corresponds to exploiting what is already known and searching for a better option corresponds to exploration. Moreover, sequential decision-making tasks themselves have recently become popular in neuroscience, psychology, and economics because they resemble real-world settings, such as employment (accept the currently offered job or keep going to interviews), dating (stay with the current partner or try a new one), Internet search, and foraging. Foraging in particular is a key analogous behavior because it provides an evolutionary explanation for the other sequential decision-making behaviors. That is, assuming that animals that had to forage for food were constantly faced with choosing to explore or exploit, there is a strong evolutionary basis for this dilemma to crop up in everyday decision-making. If we view evolution as an optimization process (Smith, 1978), then it stands to reason that significant deviations from optimal foraging may result in maladaptive behaviors, which is already apparent in the vast literature demonstrating physical and mental health detriments from abnormal responses to uncertainty in the environment (Gao and Gudykunst, 1990; Grupe and Nitschke, 2013) and reward processing (Vrieze et al., 2013; Der-Avakian et al., 2016; Safra et al., 2019). Moreover, there is a considerable number of psychiatric disorders associated with maladaptive generalization processes, including posttraumatic stress disorder (Aupperle et al., 2012), semantic dementia (Knibb and Hodges, 2005), and depression (Silberman et al., 1983). Together, assuming that generalization plays a role in how animals balance exploration and exploitation, animal foraging is a behavior crucial to understanding higher-order cognition and mental health, which is what we attempt to show in the current paper. In what follows, we first detail a computational model that sets the stage for uniting animal foraging and “mental navigation” under a common conceptual framework. This machine learning model has interesting psychological interpretations and affords quantification of a complex behavioral phenomenon, as well as individual differences in a number of finer-grained cognitive processes. We next connect the computational principles afforded by the machine learning approach to an evolutionarily ancient foraging strategy that we posit led to its reuse in (abstract) cognitive domains. Finally, we speculate on the neurochemical and neurobiological underpinnings of certain relevant phenomena outlined throughout and how these biological processes relate to psychiatric conditions, which can in turn inform treatment options and future research.
Computational mechanisms of generalization-based explore-exploit behavior
Modeling exploitation
Biological systems are resource dependent. This means that living things require other things from their environment in order to continue living. As such, foraging for food is necessary and learning how to find food efficiently is an adaptive skill that assumes that there is a systematic distribution of food that even can be learned. Fortunately, natural distributions of resources do tend to be patchy (e.g., forests, herds, bodies of water), and animals have evolved search strategies that approximate optimal computations for foraging in patchy environments (Krebs et al., 1974, 1978).
In the ethology literature, optimal foraging is studied by presenting animals with a series of patches with depleting resources and the animals must decide whether to spend time exploiting the current patch or exploring alternative patches. Hence the exploration-exploitation dilemma. Optimal foraging theory suggests that it is beneficial to balance both of these factors. The marginal value theorem (MVT) is an optimal principle that describes the most economic strategy to balance resource consumption with energy expenditure in patch foraging (Charnov, 1976) and has been used extensively in the ethology literature. Specifically, MVT says that optimal decision-making simply requires comparing immediate reward feedback from engaging with a current patch to a threshold that is the cost incurred from the time required to engage with the current patch. The incurred (opportunity) cost is a measure of overall environmental richness, as one needs to compute the long-run average of expected rewards while foraging in the environment for time equal to the time required to engage with the current patch. In other words, if the expected immediate reward from the current patch is greater than the expected average reward from foraging in the environment instead, then one should exploit. If the expected long-run average reward obtained while foraging instead of exploiting is greater, then one should explore. MVT thus makes the quite simple prediction that opportunity cost can be known by tracking average reward in the environment (Niv et al., 2006, 2007; Constantino and Daw, 2015). MVT however is a myopic decision strategy, for it compares one-step reward averages (Constantino and Daw, 2015), meaning animals using MVT are more prone to learn via trial-and-error.
An alternative reward-learning computational architecture is the temporal difference (TD) algorithm from reinforcement learning theory that captures learning the non-immediate value of sequentially encountered options via an incremental update (Rescorla-Wagner) rule that chains rewards to earlier predictors to estimate future reward (Sutton and Barto, 1998). There is a remarkable wealth of neural and behavioral support for TD learning across species, most notably in midbrain phasic dopamine responses (Montague et al., 1996; Schultz et al., 1997). TD learning differs from classical operant conditioning models because it defines value as the cumulative future reward that follows a decision. In this way, TD learning is suitable for modeling decision-making during foraging and in real-world settings. It is calculated as:
where Q represents the subjective value estimate for stimulus i at time t, r is the observed reward, α is the learning rate, and γ∈[0, 1] is a temporal discount factor. α simply scales the prediction error δ = γrt−Qit such that the value of α is how much of the prediction error is retained in memory. The prediction error itself represents the discrepancy between predicted values for stimuli and observed values for the same stimuli. Thus, for every timepoint, subjective value is updated in proportion to the magnitude of the prediction error.
Temporal difference learning has proved to be one of the most successful pairings of computational modeling and neurobiology, for midbrain neurons release dopamine in proportion to the predicted value of upcoming stimuli (Schultz et al., 1997). Implicit in this algorithm is the importance of uncertainty, or prediction error. Larger prediction errors lead to larger learning rates, for it requires the model to update previous values by a larger amount (Behrens et al., 2007). Temporal difference learning always converges to the true stimulus values; however, people often deviate from this linear learning trajectory in important ways. For example, there are asymmetric learning rates for rewarding vs. punishing stimuli (Muller et al., 2021), the value function to be learned can be non-linear and learning itself is often distributed (François-Lavet et al., 2018). Further, when the number of states (e.g., a spatial location, a physiological state of being, or a mental state of mind) is large the time it takes to learn the value function is infeasible for animals. Thus, traditional temporal difference learning is computationally intractable in high-dimensional spaces. This translates to the fact that predicting the future is computationally intractable, and thus, every decision that animals make is equipped with some uncertainty. To capture the influence of uncertainty on decision-making, we can cast temporal difference learning in its Bayesian form, which makes independent and normally distributed predictions of state values as opposed to point estimates. When option j is selected at time t the posterior mean m and variance v are updated according to:
where δjt = 1, yt is the observed reward (equivalent to rt from above) and Gjt is the Kalman Gain which is defined as:
where θ2 is the error variance. Error variance is an inverse sensitivity parameter, where smaller values result in more substantial updates to the posterior mean. This model is known as a Bayesian Mean Tracker (BMT) and it captures learning in dynamic environments. The Kalman Gain is what separates BMT from temporal difference learning, as it represents the relative importance of the prediction error given the prior subjective value estimate, which enables it to modulate the form of the convergence over time. By estimating posterior mean and variance, BMT captures the distinct influences of exploitation and exploration, respectively. How are factors of exploration and exploitation combined to make a decision?
Modeling exploration
The way we treat uncertainty remains a topic of debate due to its varied effects on behavior. For example, animals often display tendencies to explore novel environments, attend to novel stimuli, and even trade reward for information (Tolman and Honzik, 1930; Nunnally and Lemond, 1974; Blanchard et al., 2015). Real world consumers will choose newly packaged goods over the same goods in old packaging (Steenkamp and Gielens, 2003) and rodents will withstand electroshocks to experience novelty (Nissen, 1930). Interestingly, there is also substantial evidence suggesting that animals tend to display novelty avoidant behavior. The mere exposure effect illustrates this, as it characterizes the preference people show for repeated over novel objects (Zajonc, 2001). Moreover, self-directed learning paradigms have demonstrated that people choose options with more robustly known outcomes (Markant et al., 2016).
Perhaps confusingly, both behaviors that favor and disfavor novelty provide benefits. Treating novelty as rewarding in itself leads to exploration and more adaptive choices in the long run. One heuristic strategy that favors exploration and treats uncertainty as reward is to assign an exploration bonus to options (Daw et al., 2006; Friston et al., 2014). This is captured mathematically with an upper confidence bound (UCB; Auer, 2003). The frequentist, or count-based, expression of an UCB is:
where Nit is the number of times that option i has been visited up until trial t, and β is a free parameter that scales the UCB. β is the exploration bonus and by making it a free parameter, computational models can capture individual differences in how much people value the uncertainty of an option. Treating uncertainty as equivalent to reward is implicit in a number of motivational learning theories, such as intrinsic motivation (Leotti and Delgado, 2011, 2014), exploratory motivation (Murty and Adcock, 2017), information-seeking (Gottlieb et al., 2013), and curiosity drive (Loewenstein, 1994), all of which have learning and memory gains. A Bayesian UCB would simply be the square root of the posterior variance v. An option's overall value estimate is then simply the posterior mean plus the UCB:
Because Qucb leads one to choose more uncertain options, it is taken as a measure of directed exploration, which is contrasted with random exploration (Wilson et al., 2014), which is the temperature of a softmax choice rule (Luce, 1963):
Where, τ is called the temperature parameter and injects stochasticity into the decision-making process. Intuitively, soft maximization differs from UCB because UCB values will differ depending on the number of times that a given stimulus was seen or visited whereas soft maximization, all else (e.g., reward) being equal, produces the same probabilities regardless of how many times in the past one saw the stimulus. This use of the temperature parameter contrasts with its more traditional usage as controlling the tradeoff between exploration and exploitation. Using the temperature parameter to control the tradeoff means that when τ is low, one exploits and when it is high, one explores. Here, τ can be low (indicating little random exploration), while directed exploration is still high. Recent work has shown exploitation and both forms of exploration to be dissociable with distinct effects on decision-making in ecologically valid tasks (Wilson et al., 2014; Gershman, 2018a,b, 2019; Tomov et al., 2020; Bhui et al., 2021), are underpinned by dissociable genetic components (Gershman and Tzovaras, 2018) and neural activity (Warren et al., 2017; Zajkowski et al., 2017; Dubois et al., 2021), and are associated with distinct psychopathologies (Smith et al., 2022). For example, lower levels of directed exploration have associated with problem gambling (Wiehler et al., 2021), trait somatic anxiety (Fan et al., 2021), and depression (Smith et al., 2022) and the combination of both directed and random exploration has been shown to be optimal for reward learning (Wilson et al., 2014; Gershman, 2018a, 2019; Tomov et al., 2020). On the other hand, there are nontrivial advantages to being novelty averse. For instance, it is sometimes better to avoid high-risk situations regardless of the novelty that they offer (Schulz et al., 2018a; Stojic et al., 2020). Moreover, random exploration may reflect increased confusion or worse overall learning (Wu et al., 2020) and has been linked to impulsivity (Dubois and Hauser, 2022). Directed exploration can be detrimental in contexts with short time horizons, though this is speculation based on the findings that directed exploration in healthy adults increases with increased time horizons (Wilson et al., 2014; Wu et al., 2022) and that directed exploration correlates with temporal discounting (Sadeghiyeh et al., 2020). Finally, in self-directed learning studies, people show enhanced learning and memory for objects associated with lower levels of uncertainty (Voss et al., 2011a,b; Houser et al., 2022). How can we reconcile these seemingly disparate threads of research on animal responses to novelty?
Modeling generalization
To explain the co-occurrence of novelty preference and novelty avoidance, Gershman and Niv (2015) proposed that contextual influences shape the way uncertainty is processed. For example, one may be novelty seeking in a candy store, where the rewards are plentiful, but novelty averse in a dark forest with snakes and spiders. Using the context, or the structural form, from a previously learned environment to inform decision-making in a novel environment is a way to speed up learning and maximize reward (Wu et al., 2018, 2020, 2021; Bhui et al., 2021). This ability to leverage learned information in a new situation is generalization (Taylor et al., 2021). Thus, generalization can be viewed as an arbitrator between exploration and exploitation given the current context. A number of recent studies have demonstrated the adaptive advantages that generalization has on goal-directed (Schulz et al., 2018a,b,c, 2020; Wu et al., 2018, 2020, 2021; Stojic et al., 2020), concept (Shi et al., 2008, 2010; Lucas et al., 2015), and social (Naito et al., 2022) learning, and across development (Schulz et al., 2019; Meder et al., 2021; Giron et al., 2022).
Generalization offers learning of correlated features, states, or values, such that knowledge of one informs knowledge of those that are similar. A recently proposed non-parametric Bayesian model (Gaussian Process) of function learning offers an end-to-end computational architecture that characterizes exploration, exploitation, and generalization (Lucas et al., 2015). When combined with a sampling strategy and a decision rule, this working model of reinforcement learning and decision-making, i.e., goal-directed cognition, unveils how generalization interrelates with the balance of exploration and exploitation. This model offers a complementary approach to the value function approximation approach in reinforcement learning (Schaul et al., 2015). In fact, Gaussian Processes can be interpreted as universal function approximators, and have a number of psychologically interpretable components.
A Gaussian Process (GP) defines a multivariate normal distribution over functions f(s) that map input s to output y = f(s). The function corresponds to a random draw from the GP:
where:
and:
Here, m is the mean function, or simply a vector of averages for each variable that is being measured (e.g., options, states) and k is the covariance, or kernel, function that determines the smoothness of relatedness between stimuli, thus expressing the similarity between s and s′. The kernel is what enables the model to learn correlated option values, i.e., generalize, and corresponds exactly to Shepard's universal law of generalization. That is, the kernel function learns psychological distances between stimuli.
There are many options for kernel functions, but a common choice is the radial basis function kernel (RBFK), which can approximate any function:
Where, λ is called the length-scale parameter and captures how smoothly correlations between s and s′ decay (Figure 1) as a function of squared Euclidean distance when r = 2 or city block distance when r = 1. f(s) is thus a random sample from a distribution of latent functions that has incorporated the pairwise covariances between variables, such that learning does not happen independently for each variable. A brief tutorial on Gaussian Process basics using R can be found at https://github.com/troyhouser/gaussian-processes.
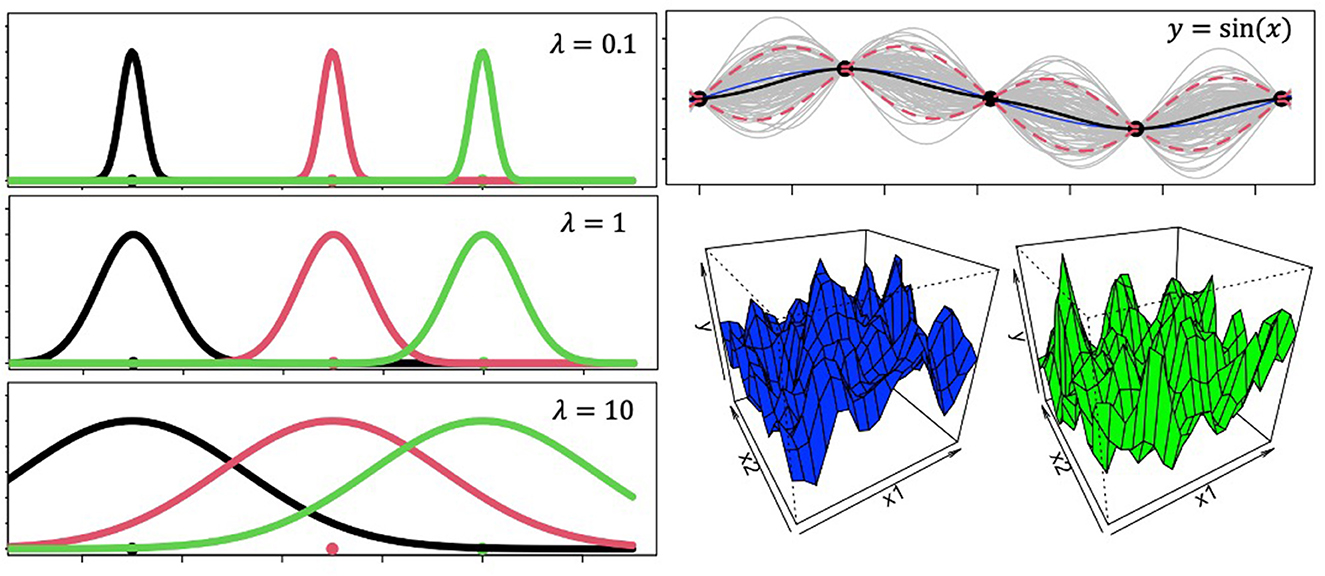
Figure 1. Gaussian process ingredients. The panels on the left show Gaussian radial basis function tuning curves with different values for the lengthscale parameter. These plots demonstrate how larger lengthscales lead to wider generalization gradients. The panel on the top right reveals Gaussian Process predictions for out-of-sample datapoints. The model was trained on sequential inputs x = [0.1, 2π] with output labels y = sin(x). Then the model was shown novel inputs x′ = [−0.1, 0.1], for which it generated the plot at the top right panel. The blue line is the actual outputs for the novel inputs according to the function y = sin(x), the black line is the model's mean function, and the dotted red lines are 5th and 95th percent quantiles. Gray lines represent random samples from the distribution. 3D grids in the bottom right represent two randomly sampled functions from a 2-dimensional Gaussian Process.
Putting the pieces together
Importantly, a GP model can simulate the learning process from end-to-end. First, we calculate the pairwise similarities between a set of training data:
Where, e is noise and s are stimuli whose values have been learned. We then obtain a precision matrix P by inverting Strain, . Next, we project the matrix of pairwise similarities between novel stimuli s′ and the training set onto P, which will map the novel stimulus values into the similarity space of training data, describing the influence that training labels y have on the novel stimuli. This yields the posterior mean function:
Where, I is the identity matrix. The posterior mean is the expected value estimates for novel stimuli s′ (Figure 1). To obtain the posterior variance, we subtract the similarities between novel and training data from the pairwise similarities between novel data alone :
which captures the uncertainty associated with the expected value estimates. Now that we have calculated the posterior mean and variance, we can obtain UCB estimates and transform these value estimates into probabilities with the softmax equation. Decisions are made by sampling options with probabilities equal to the outputs of the softmax. There are three free parameters in this model: λ, β, and τ, i.e., lengthscale, exploration bonus, and temperature. However, for the purposes of this paper, we can think of them as generalization, directed exploration, and random exploration, respectively. By estimating λ, the model learns correlations in the environment, while estimating β accounts for safe optimization techniques (Schulz et al., 2018a), e.g., only exploring if certain conditions are met, and τ factors in certain levels of stochasticity that have been found to be adaptive in goal-directed tasks (Wilson et al., 2014; Gershman, 2018a, 2019; Luthra et al., 2020; Tomov et al., 2020).
Recent studies employing the GP model are lab experiments with humans, so it is possible that this leveraging of generalization to maximize reward is a relatively recent evolution that emerges with advanced cognition. We think it far more likely, however, that generalization is an evolutionarily ancient strategy for boosting adaptive decision-making. Specifically, we propose that the area-restricted search (ARS) foraging strategy (Tinbergen et al., 1967) resolves the exploration-exploitation dilemma by using generalization processes.
Bridging physical and mental navigation via boundedly rational computational mechanisms
Imagine being hungry and alone in a forest. The only knowledge you have is that resources tend to be distributed in patches. What is the most effective search strategy? It would be to make long quasi-linear excursions until stumbling upon some food, at which point you should concentrate your movements to nearby locations. However, resources also naturally deplete, which is why this strategy must be constantly recycled. That is, after a patch has been exhausted of its resources, one must make another long excursion. Excursions following patch depletion should be long because of the natural patchiness of resources. This is called area-restricted search (ARS) and it is a hallmark of foraging in evolutionarily distinct taxa such as protists, nematodes, insects, birds, and mammals, including humans (Hills et al., 2004; Hills, 2006; Dorfman et al., 2022). In short, ARS is the cycling between directed exploration (long, quasi-linear excursions) and focused exploitation (exhausting a patch of its resources). It was first reported by Laing (1937) who noted that parasitoid wasps reduce their movement speed and increase their turn rate upon contacting its host eggs. In other words, this parasitic creature focuses its exploration to the area it previously found a viable host. Since then, ARS has been noted in a broad range of species (Chandler, 1969; Glen, 1975; Bond, 1980; Eveleigh and Chant, 1982; Strand and Vinson, 1982; Hoffmann, 1983a,b; Schal et al., 1983; Ferran et al., 1994; Einoder et al., 2011). Many computational models have also suggested that ARS emerges when animals are in a patchily-distributed environment and that it approximates patterns predicted by MVT (Adler and Kotar, 1999; Scharf et al., 2009). Humans foraging in a virtual reality environment also demonstrate ARS (T. T. Hills et al., 2013). Particularly telling, Ross and Winterhalder (2018) found that blowgun hunters slow down and increase their turning angle as a function of prey encounters in the wild.
ARS is not the only foraging strategy that animals exhibit. Certain conditions such as the absence of accurate sensory cues must be met for animals to use ARS (Dorfman et al., 2022); however, the widespread use and evolutionary preservation of ARS makes it possible that it was a primary driver of higher-order cognitive processes (Hills, 2006). For example, memory is required to exploit a previously exhausted patch, inhibition is needed to avoid searching a patch for too long, and temporal interval estimation is necessary to maintain the delicate balance of exploration and exploitation. An overlooked cognitive process that we suggest is fundamental to ARS is generalization.
An implicit assumption that ARS makes is that nearby locations yield similar resources. In order to even make this assumption, it is logically necessary to be able to generalize information associated with one location to nearby locations. In terms of patch foraging, upon encountering a patch with food, an animal generalizes the food that a particular location affords to the entire patch, enabling animals to subsequently exploit the patch. That is, the naturally spatially-correlated distribution of resources led to major advantages for those animals that evolved generalization capacities, for generalization enables equivocating outcomes of two actions or states, one of which was learned previously and one that is completely novel.
The important intuition that ARS behavioral patterns contributes to both goal-directed behaviors and generalization processes is that learning of environmental states does not occur independently. The spatial correlations of natural resources likely led to the evolution of cognitive processes that generalize in accordance with spatial distributions, which is exactly what the universal law of generalization embodies. In Figure 2, we show the three steps of the GP model, including how agents may represent the environment in terms of exploitation, exploration, and their combination. The actual resource distribution was an example reward function from (Wu et al., 2020), which varied the strength of correlation between mean reward values as a function of the distance between locations.
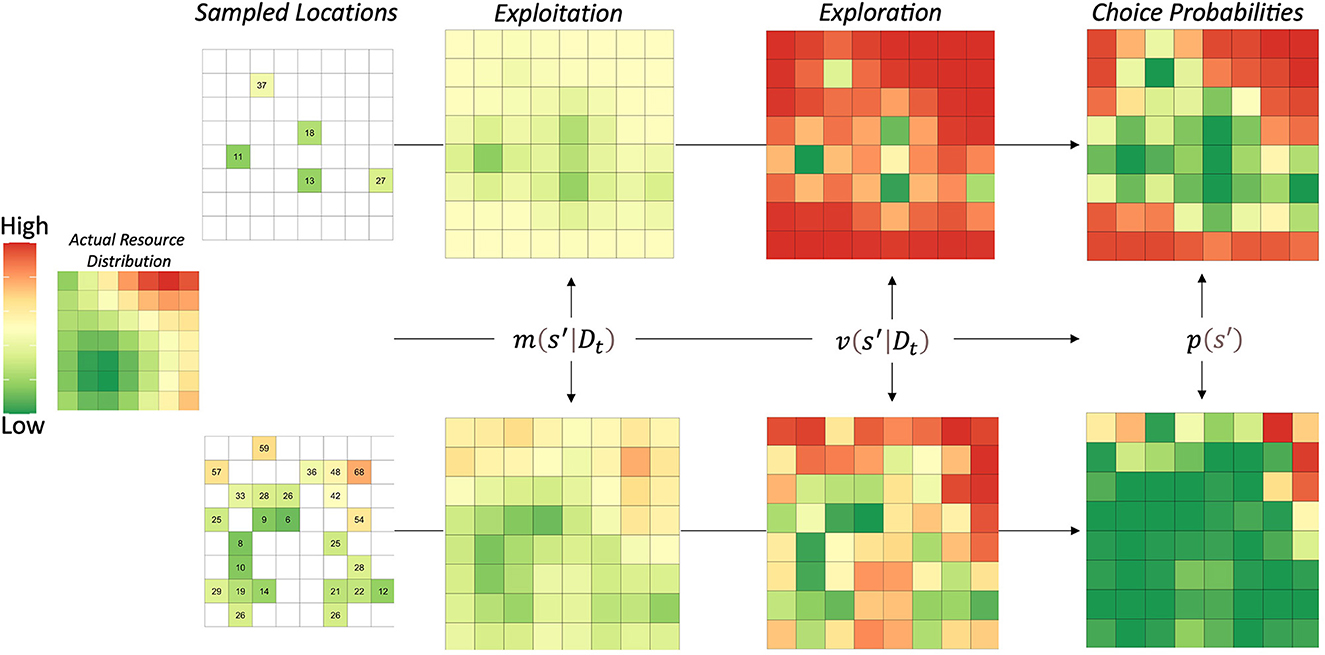
Figure 2. Visualization of Gaussian process model steps. The left side shows the actual resource'distribution. Numbers represent the rewards/resources obtained by sampling that location. The model proceeds in three steps: (1) obtain posterior estimates of both mean and variance, (2) compute the upper confidence bounds for each location, and (3) apply a softmax choice rule to obtain choice probabilities for each location. The model generalizes information from directly experienced to novel locations, enabling it to learn the entire reward function faster. The upper confidence bound also biases decision-making to novel locations, which is applied adaptively in this GP-ARS model. In other words, the GP-ARS agent chooses from novel options that are nearby locations previously learned to predict high reward.
Neurobiological substrates
While a central nervous system is not necessary for ARS patterns, a common neural network seems to underly ARS in animals (Dorfman et al., 2022). One study showed that C. elegans performs ARS in response to food deprivation (Hills et al., 2004), which is inhibited by the dopamine antagonist raclopride or the genetic mutation-induced ablation of dopaminergic neurons. Specifically, the prevention of dopamine synthesis modulates turning frequency in response to food deprivation, implicating dopamine in the direct control of responses to food deprivation. This was further supported by the finding that exogenously supplied dopamine restored ARS in worms with ablated dopaminergic neurons (Hills et al., 2004). Dopamine controls these behavioral responses by acting on glutamatergic signaling pathways (Zheng et al., 1999). Specifically, the dopamine-activated second messenger cAMP leads to phosphorylation of AMPA-type ionotropic glutamate receptors that ultimately results in a net activation of DARPP-32 (Yan et al., 1999). DARPP-32 is a phosphoprotein that mediates responses to naturally positive stimuli (Scheggi et al., 2018) via modulation of excitability and plasticity in striatal neurons (Fienberg et al., 1998; Schiffmann, 1998). Essentially, DARPP-32 increases the gain of neurons expressing the D1 receptor, leading to higher levels of exploitation.
Exploratory behaviors have been localized to prefrontal brain regions (Daw et al., 2006; Averbeck, 2015; Ebitz et al., 2018), specifically, the frontopolar cortex (Averbeck, 2015; Hogeveen et al., 2022; FPC). One study used TMS to lesion the right FPC and found that it selectively inhibited directed but not random exploration (Zajkowski et al., 2017). Moreover, random exploration is likely driven by diffuse noradrenergic projections from the locus coerulus (Cohen et al., 2007). Substantiating this claim, van Dooren et al. (2021) showed that arousal increases exploratory behavior. Interestingly, increasing noradrenaline with atomoxerine (Warren et al., 2017) or propranolol (Dubois et al., 2021) reduces random exploration, though this may be due to the nonlinear relationship between tonic norepinephrine release and cognition (Valentino and Foote, 1988; Berridge and Waterhouse, 2003; Aston-Jones and Cohen, 2005a,b; Cohen et al., 2007; Warren et al., 2017). Random exploration in particular requires more work to elucidate the neurobiological mechanisms and fine-grained processes that underpin its realization. For example, how is exploration by chance under cognitive control? Do all brain regions downstream of locus coerulus perform random exploration in some form? An intriguing simulation study of the mutual evolution of cognition and environmental patchiness suggests that it is adaptive to explore randomly within patches (Luthra et al., 2020), in which case random exploration is likely better explained as random exploitation. That is, because animals generalize reward throughout a patch, randomly exploring the patch is more of an exploitative behavior. Assuming that this interpretation of random exploration is correct, at least in a nontrivial amount of cases, this would warrant further studies on the hierarchical nature of patch foraging in a reinforcement learning context (i.e., random exploitation assumes that the option being exploited is the entire patch, not individual states within a patch).
While there has been a considerable amount of work focused on uncovering the neural correlates of both exploration and exploitation, there has been much less attention dedicated to understanding how generalization facilitates the tradeoff between exploration and exploitation adaptively. Though future empirical work will be needed to confirm our prediction, we hypothesize that ARS is dependent upon functional connectivity between the hippocampus and midbrain dopaminergic hubs (e.g., ventral tegmental area, substantia nigra, and striatum). This makes sense because the GP-ARS model is describing reinforcement learning in environments with correlated states or features, thus necessitating goal-directed cognition from the midbrain and generalization processes from the hippocampus. There is no work examining hippocampal-midbrain coupling through a GP-ARS lens, however, there are some existing studies that support this interpretation. Multiple fMRI studies have found that generalization gradients are underpinned by hippocampal-midbrain functional connectivity (Kahnt et al., 2012, 2015), and one study found that blocking dopamine receptors narrows generalization gradients (Kahnt et al., 2015). Shohamy and Wagner (2008) also found hippocampal-midbrain coupling using an acquired equivalence paradigm, known to require generalization to arbitrary stimuli.
Together, the existing literature points to a network of brain regions, including the hippocampus, dopaminergic midbrain, and prefrontal cortex, that supports ARS in physical and psychological spaces, perhaps via the GP model introduced above.
Discussion
Evolution is a story of biological optimization, whereby living systems adapt to a co-evolving environment, and in doing so, are continually faced with the challenge of obtaining resources to go on living. While factors of exploitation and exploration have been known to shape decision-making under naturalistic demands, it was relatively recently discovered that generalization plays an equally fundamental role. Imagine learning that the Starbucks on 7th Street is now selling pumpkin spice lattes. We will likely generalize the knowledge that the Starbucks on 7th Street is selling pumpkin spice lattes to all Starbucks stores, enabling us to infer what stores that we have never had experience with are selling. Moreover, the reason that generalization is used from an evolutionary standpoint has rarely been considered. The evolutionary impetus for generalization is crucial for understanding a host of psychiatric conditions that present with maladaptive generalization strategies. In the present paper, we attempted to unite decision-making, a computational model, and generalization's evolutionary origins in a common framework. Additionally, we outlined possible neurobiological substrates responsible for the complex computations underlying value-based learning with correlated states. The central prediction made here was that these computations evolved because of area-restricted search strategies and are reused for cognitive search strategies in abstract, psychological spaces induced by neural activity. By decomposing choices into exploitation, random and directed exploration, and generalization, we think the GP model can cover a wide range of decision types in both laboratory and real-world settings, describe individual differences in cognition, and provide a more thorough understanding of the origins of, and treatment options for, psychiatric disorders. For example, it is an open question of why people with panic, anxiety, and posttraumatic stress disorders overgeneralize (Dunsmoor et al., 2009, 2011; Dunsmoor and Paz, 2015; Dymond et al., 2015; Struyf et al., 2017). This could result from novelty avoidance or perceptual distortions, in which case beneficial cognitive-behavioral treatments might include exposure therapy or discrimination training, respectively. By characterizing people's decision-making with a GP model, researchers can obtain estimates of the influences of both novelty (β) and perceptual distortion (λ), thereby informing a more nuanced treatment plan.
Limitations
The hypotheses and theory as presented here suffers from limitations. First, the integration of large-scale cognitive processes such as exploration, exploitation, and generalization will likely require a thorough explanation of the biophysical mechanisms underlying how such signals combine to shape decision-making, which we have not provided here. Such an explanation will likely require models characterizing differences in dopaminergic signaling when it is influenced and not influenced by hippocampal generalization processes. Similarly, the current paper lacks an explanation for how hippocampal-midbrain coupling integrates with exploratory signals in the prefrontal cortex.
Data availability statement
The original contributions presented in the study are included in the article/supplementary material, further inquiries can be directed to the corresponding author/s.
Author contributions
The author confirms being the sole contributor of this work and has approved it for publication.
Funding
Open Access-Article Processing Charge (OA-APC) award fund.
Conflict of interest
The author declares that the research was conducted in the absence of any commercial or financial relationships that could be construed as a potential conflict of interest.
Publisher's note
All claims expressed in this article are solely those of the authors and do not necessarily represent those of their affiliated organizations, or those of the publisher, the editors and the reviewers. Any product that may be evaluated in this article, or claim that may be made by its manufacturer, is not guaranteed or endorsed by the publisher.
References
Adler, F. R., and Kotar, M. (1999). Departure time versus departure rate: How to forage optimally when you are stupid. Evolut. Ecol. Res. 1, 4.
Aston-Jones, G., and Cohen, J. D. (2005a). Adaptive gain and the role of the locus coeruleus-norepinephrine system in optimal performance. J. Comp. Neurol. 493, 723. doi: 10.1002./cne.20723
Aston-Jones, G., and Cohen, J. D. (2005b). “An integrative theory of locus coeruleus-norepinephrine function: adaptive gain and optimal performance,” in Annual Review of Neuroscience (Vol. 28). doi: 10.1146./annurev.neuro.28.061604.135709
Auer, P. (2003). Using confidence bounds for exploitation-exploration trade-offs. J. Mac. Learn. Res. 3, 663. doi: 10.1162./153244303321897663
Aupperle, R. L., Melrose, A. J., Stein, M. B., and Paulus, M. P. (2012). “Executive function and PTSD: Disengaging from trauma,” in Neuropharmacology (Vol. 62, Issue 2).
Averbeck, B. B. (2015). Theory of Choice in Bandit, Information Sampling and Foraging Tasks. PLoS Comput. Biol. 11, 3. doi: 10.1371./journal.pcbi.1004164
Behrens, T. E. J., Woolrich, M. W., Walton, M. E., and Rushworth, M. F. S. (2007). Learning the value of information in an uncertain world. Nature Neurosci. 10, 1954. doi: 10.1038./nn1954
Berridge, C. W., and Waterhouse, B. D. (2003). “The locus coeruleus-noradrenergic system: Modulation of behavioral state and state-dependent cognitive processes,” in Brain Research Reviews (Vol. 42, Issue 1). doi: 10.1016./S0165-0173(03)00143-7
Bhui, R., Lai, L., and Gershman, S. J. (2021). “Resource-rational decision making.” in Current Opinion in Behavioral Sciences (Vol. 41). doi: 10.1016/j.cobeha.02015
Blanchard, T. C., Hayden, B. Y., and Bromberg-Martin, E. S. (2015). Orbitofrontal cortex uses distinct codes for different choice attributes in decisions motivated by curiosity. Neuron 85, 50. doi: 10.1016/j.neuron.12050
Bond, A. B. (1980). Optimal foraging in a uniform habitat: the search mechanism of the green lacewing. Animal Behav. 28, 3. doi: 10.1016./S0003-3472(80)80003-0
Chandler, A. E. F. (1969). Locomotory behaviour of first instar larvae of aphidophagous syrphidae (Diptera) after contact with aphids. Animal Behav. 17, 4. doi: 10.1016./S0003-3472(69)80011-4
Charnov, E. L. (1976). Optimal foraging: attack strategy of a mantid. Am. Natural. 110, 971. doi: 10.1086./283054
Cohen, J. D., McClure, S. M., and Yu, A. J. (2007). Should I stay or should I go? How the human brain manages the trade-off between exploitation and exploration. Philosoph. Transact. Royal Soc. B Biol. Sci. 362, 1481. doi: 10.1098./rstb.2007.2098
Constantino, S. M., and Daw, N. D. (2015). Learning the opportunity cost of time in a patch-foraging task. Cogn. Affect. Behav. Neurosci. 15, 350. doi: 10.3758./s13415-015-0350-y
Daw, N. D., O'Doherty, J. P., Dayan, P., Seymour, B., and Dolan, R. J. (2006). Cortical substrates for exploratory decisions in humans. Nature 441, 7095. doi: 10.1038./nature04766
Der-Avakian, A., Barnes, S. A., Markou, A., and Pizzagalli, D. A. (2016). “Translational assessment of reward and motivational deficits in psychiatric disorders,” in Current Topics in Behavioral Neurosciences (Vol. 28). doi: 10.1007./7854_2015_5004
Dorfman, A., Hills, T. T., and Scharf, I. (2022). A guide to area-restricted search: a foundational foraging behaviour. Biol. Rev. 97, 2076–2089. doi: 10.1111/brv.12883
Dubois, M., Habicht, J., Michely, J., Moran, R., Dolan, R. J., Hauser, T. U., et al. (2021). Human complex exploration strategies are enriched by noradrenaline-modulated heuristics. ELife 10, 9907. doi: 10.7554./eLife.59907
Dubois, M., and Hauser, T. U. (2022). Value-free random exploration is linked to impulsivity. Nature Commun. 13, 4542. doi: 10.1038/s41467-022-31918-9
Dunsmoor, J. E., Mitroff, S. R., and LaBar, K. S. (2009). Generalization of conditioned fear along a dimension of increasing fear intensity. Learn. Memory 16, 1609. doi: 10.1101./lm.1431609
Dunsmoor, J. E., and Paz, R. (2015). “Fear Generalization and Anxiety: Behavioral and Neural Mechanisms,” in Biological Psychiatry (Vol. 78, Issue 5). doi: 10.1016/j.biopsych.04010
Dunsmoor, J. E., Prince, S. E., Murty, V. P., Kragel, P. A., and LaBar, K. S. (2011). Neurobehavioral mechanisms of human fear generalization. NeuroImage 55, 41. doi: 10.1016/j.neuroimage.01041
Dymond, S., Dunsmoor, J. E., Vervliet, B., Roche, B., and Hermans, D. (2015). Fear generalization in humans: systematic review and implications for anxiety disorder research. Behav. Therapy 46, 1. doi: 10.1016/j.beth.10001
Ebitz, R. B., Albarran, E., and Moore, T. (2018). Exploration disrupts choice-predictive signals and alters dynamics in prefrontal cortex. Neuron 97(2). doi: 10.1016/j.neuron.12007
Einoder, L. D., Page, B., Goldsworthy, S. D., Little, d. e., and Bradshaw, S. C. (2011). Exploitation of distant Antarctic waters and close neritic waters by short-tailed shearwaters breeding in South Australia. Austral Ecol. 36, 2176. doi: 10.1111./j.1442-9993.2010.02176.x
Eveleigh, E. S., and Chant, D. A. (1982). The searching behaviour of two species of phytoseiid mites, Phytoseiulus persimilis Athias-Henriot and Amblyseius degenerans (Berlese), in relation to the density and distribution of prey in an homogeneous area (Acarina: Phytoseiidae). Can. J. Zool. 60, 94. doi: 10.1139./z82-094
Fan, H., Gershman, S. J., and Phelps, E. A. (2021). Trait Somatic Anxiety is Associated With Reduced Directed Exploration and Underestimation of Uncertainty. PsyArXiv Preprints.
Ferran, A., Ettifouri, M., Clement, P., and Bell, W. J. (1994). Sources of variability in the transition from extensive to intensive search in coccinellid predators (Homoptera: Coccinellidae). J. Insect Behav. 7, 436. doi: 10.1007./BF01997436
Fienberg, A. A., Hiroi, N., Mermelstein, P. G., Song, W. J., Snyder, G. L., Nishi, A., et al. (1998). DARPP-32: regulator of the efficacy of dopaminergic neurotransmission. Science 281, 5378. doi: 10.1126./science.281.5378.838
François-Lavet, V., Henderson, P., Islam, R., Bellemare, M. G., and Pineau, J. (2018). An introduction to deep reinforcement learning. Found. Trends Machine Learn. 11, 71. doi: 10.1561./2200000071
Friston, K., Schwartenbeck, P., FitzGerald, T., Moutoussis, M., Behrens, T., and Dolan, R. J. (2014). The anatomy of choice: dopamine and decision-making. Philosophical Transact. Royal Soc. B Biol. Sci. 369, 481. doi: 10.1098./rstb.2013.0481
Gao, G., and Gudykunst, W. B. (1990). Uncertainty, anxiety, and adaptation. Int. J. Intercult. Relat. 14, 17. doi: 10.1016./0147-1767(90)90017-Q
Gershman, S. J. (2018a). Deconstructing the human algorithms for exploration. Cognition, 17314. doi: 10.1016/j.cognition.12014
Gershman, S. J. (2018b). The successor representation: its computational logic and neural substrates. J. Neurosci. 38, 7193–7200. doi: 10.1523/JNEUROSCI.0151-18.2018
Gershman, S. J., and Niv, Y. (2015). Novelty and inductive generalization in human reinforcement learning. Topics Cogn. Sci. 7, 213. doi: 10.1111./tops.12138
Gershman, S. J., and Tzovaras, B. G. (2018). Dopaminergic genes are associated with both directed and random exploration. Neuropsychologia 120, 9. doi: 10.1016/j.neuropsychologia.10009
Giron, A. P., Ciranka, S., Schulz, E., van den Bos, W., Ruggeri, A., Meder, B., et al. (2022). Developmental Changes Resemble Stochastic Optimization. PsyArXiv [Preprint]. doi: 10.31234/osf.io/9f4k3
Glen, D. M. (1975). Searching Behaviour and Prey-Density Requirements of Blepharidopterus angulatus (Fall.) (Heteroptera: Miridae) as a Predator of the Lime Aphid, Eucallipterus tiliae (L.), and Leafhopper, Alnetoidea alneti (Dahlbom). J. Animal Ecol. 44, 3854. doi: 10.2307./3854
Gottlieb, J., Oudeyer, P. Y., Lopes, M., and Baranes, A. (2013). “Information-seeking, curiosity, and attention: Computational and neural mechanisms,” in Trends in Cognitive Sciences (Vol. 17, Issue 11). doi: 10.1016/j.tics.09001
Grupe, D. W., and Nitschke, J. B. (2013). “Uncertainty and anticipation in anxiety: an integrated neurobiological and psychological perspective,” in Nature Reviews Neuroscience (Vol. 14, Issue 7). doi: 10.1038./nrn3524
Hayden, B. Y., Pearson, J. M., and Platt, M. L. (2011). Neuronal basis of sequential foraging decisions in a patchy environment. Nature Neurosci. 14, 2856. doi: 10.1038./nn.2856
Hills, T., Brockie, P. J., and Maricq, A. V. (2004). Dopamine and glutamate control area-restricted search behavior in caenorhabditis elegans. J. Neurosci. 24, 4. doi: 10.1523./JNEUROSCI.1569-03.2004
Hills, T. T. (2006). Animal foraging and the evolution of goal-directed cognition. Cogn. Sci. 30, 50. doi: 10.1207./s15516709cog0000_50
Hills, T. T., Kalff, C., and Wiener, J. M. (2013). Adaptive Lévy processes and area-restricted search in human foraging. PLoS ONE 8, 6488. doi: 10.1371./journal.pone.0060488
Hoffmann, G. (1983a). The random elements in the systematic search behavior of the desert isopod Hemilepistus reaumuri. Behav. Ecol. Sociobiol. 13, 3798. doi: 10.1007./BF00293798
Hoffmann, G. (1983b). The search behavior of the desert isopod Hemilepistus reaumuri as compared with a systematic search. Behav. Ecol. Sociobiol. 13, 3799. doi: 10.1007./BF00293799
Hogeveen, J., Mullins, T. S., Romero, J. D., Eversole, E., Rogge-Obando, K., Mayer, A. R., et al. (2022). The neurocomputational bases of explore-exploit decision-making. Neuron 110, 1869–1879. doi: 10.1016/j.neuron.03014
Houser, T. M., Tompary, A., and Murty, V. (2022). Agency Enhances Temporal Order Memory in an Interactive Exploration Game. Psychon. Bull. Rev. 29, 2219–2228. doi: 10.3758/s13423-022-02152-7
Hunt, L. T., Daw, N. D., Kaanders, P., MacIver, M. A., Mugan, U., Procyk, E., et al. (2021). “Formalizing planning and information search in naturalistic decision-making,” in Nature Neuroscience (Vol. 24, Issue 8). doi: 10.1038./s41593-021-00866-w
Kahnt, T., Park, S. Q., Burke, C. J., and Tobler, P. N. (2012). How glitter relates to gold: similarity-dependent reward prediction errors in the human striatum. J. Neurosci. 32, 46. doi: 10.1523./JNEUROSCI.2383-12.2012
Kahnt, T., Weber, S. C., Haker, H., Robbins, T. W., and Tobler, P. N. (2015). Dopamine D2-receptor blockade enhances decoding of prefrontal signals in humans. J. Neurosci. 35, 14. doi: 10.1523./JNEUROSCI.4182-14.2015
Knibb, J. A., and Hodges, J. R. (2005). Semantic dementia and primary progressive aphasia: a problem of categorization? Alzheimer Dis. Assoc. Disord. 19(SUPPL. 1), 13. doi: 10.1097./01.wad.0000183085.22562.13
Kolling, N., Behrens, T. E. J., Mars, R. B., and Rushworth, M. F. S. (2012). Neural mechanisms of foraging. Science 335, 6930. doi: 10.1126./science.1216930
Kolling, N., Wittmann, M. K., Behrens, T. E. J., Boorman, E. D., Mars, R. B., Rushworth, M. F. S., et al. (2016). “Value, search, persistence and model updating in anterior cingulate cortex,” in Nature Neuroscience (Vol. 19, Issue 10). doi: 10.1038./nn.4382
Krebs, J. R., Kacelnik, A., and Taylor, P. (1978). Test of optimal sampling by foraging great tits. Nature 275, 5675. doi: 10.1038./275027a0
Krebs, J. R., Ryan, J. C., and Charnov, E. L. (1974). Hunting by expectation or optimal foraging? A study of patch use by chickadees. Ani. Behav. 22(PART 4), 9. doi: 10.1016./0003-3472(74)90018-9
Laing, J. (1937). Host-finding by insect parasites. 1. observations on the finding of hosts by alysia manducator, mormoniella vitripennis and trichogramma evanescens. J. Ani. Ecol. 6, 1186. doi: 10.2307./1186
Leotti, L. A., and Delgado, M. R. (2011). The inherent reward of choice. Psychol. Sci. 22, 7005. doi: 10.1177./0956797611417005
Leotti, L. A., and Delgado, M. R. (2014). The value of exercising control over monetary gains and losses. Psychol. Sci. 25, 589. doi: 10.1177./0956797613514589
Loewenstein, G. (1994). The psychology of curiosity: a review and reinterpretation. Psychol. Bullet. 116, 75. doi: 10.1037//0033-116, 1.75
Lucas, C. G., Griffiths, T. L., Williams, J. J., and Kalish, M. L. (2015). “A rational model of function learning,” in Psychonomic Bulletin and Review (Vol. 22, Issue 5). doi: 10.3758./s13423-015-0808-5
Luce, R. D. (1963). A threshold theory for simple detection experiments. Psychol. Rev. 70, 9723. doi: 10.1037./h0039723
Luthra, M., Izquierdo, E. J., and Todd, P. M. (2020). “Cognition evolves with the emergence of environmental patchiness,” in Proceedings of the ALIFE 2020: The 2020 Conference on Artificial Life. ALIFE 2020: The 2020 Conference on Artificial Life. p. 450–458. doi: 10.1162./isal_a_00330
Markant, D. B., Settles, B., and Gureckis, T. M. (2016). Self-directed learning favors local, rather than global, uncertainty. Cogn. Sci. 40, 220. doi: 10.1111./cogs.12220
Meder, B., Wu, C. M., Schulz, E., and Ruggeri, A. (2021). Development of directed and random exploration in children. Develop. Sci. 24, 13095. doi: 10.1111./desc.13095
Montague, P. R., Dayan, P., and Sejnowski, T. J. (1996). A framework for mesencephalic dopamine systems based on predictive Hebbian learning. J. Neurosci. 16, 56. doi: 10.1523./jneurosci.16-05-01936.1996
Muller, T. H., Butler, J. L., Veselic, S., Miranda, B., Behrens, T. E. J., Kurth-Nelson, Z., et al. (2021). Distributional Reinforcement Learning in Prefrontal Cortex. BioRxiv [Preprint]. doi: 10.1101/2021.06.14.448422
Murty, V. P., and Adcock, R. A. (2017). “Distinct medial temporal lobe network states as neural contexts for motivated memory formation,” in The Hippocampus from Cells to Systems: Structure, Connectivity, and Functional Contributions to Memory and Flexible Cognition. doi: 10.1007./978-3-319-50406-3_15
Naito, A., Katahira, K., and Kameda, T. (2022). Insights about the common generative rule underlying an information foraging task can be facilitated via collective search. Sci. Rep. 12, 8047. doi: 10.1038/s41598-022-12126-3
Nissen, H. W. (1930). A study of exploratory behavior in the white rat by means of the obstruction method. Pedag. Semin. J. Gen. Psychol. 37, 162. doi: 10.1080./08856559.1930.9944162
Niv, Y., Daw, N. D., Joel, D., and Dayan, P. (2007). Tonic dopamine: opportunity costs and the control of response vigor. Psychopharmacology 191, 4. doi: 10.1007./s00213-006-0502-4
Niv, Y., Joel, D., and Dayan, P. (2006). A normative perspective on motivation. Trends Cogn. Sci. 10, 6010. doi: 10.1016/j.tics.06010
Nunnally, J. C., and Lemond, L. C. (1974). Exploratory behavior and human development. Adv. Child Develop. Behav. 8, 493. doi: 10.1016./S0065-2407(08)60493-0
Ross, C. T., and Winterhalder, B. (2018). Evidence for encounter-conditional, arearestricted search in a preliminary study of Colombian blowgun hunters. PLoS ONE 13, 633. doi: 10.1371./journal.pone.0207633
Sadeghiyeh, H., Wang, S., Alberhasky, M. R., Kyllo, H. M., Shenhav, A., Wilson, R. C., et al. (2020). Temporal discounting correlates with directed exploration but not with random exploration. Sci. Rep. 10, 4. doi: 10.1038./s41598-020-60576-4
Safra, L., Chevallier, C., and Palminteri, S. (2019). Depressive symptoms are associated with blunted reward learning in social contexts. PLoS Comput. Biol. 15, 224. doi: 10.1371./journal.pcbi.1007224
Schal, C., Tobin, T. R., Surber, J. L., Vogel, G., Tourtellot, M. K., Leban, R. A., et al. (1983). Search strategy of sex pheromone-stimulated male German cockroaches. J. Insect Physiol. 29, 9. doi: 10.1016./0022-1910(83)90023-9
Scharf, I., Kotler, B., and Ovadia, O. (2009). Consequences of food distribution for optimal searching behavior: an evolutionary model. Evolut. Ecol. 23, 6. doi: 10.1007./s10682-007-9220-6
Schaul, T., Horgan, D., Gregor, K., and Silver, D. (2015). Proceedings of the 32nd international conference on machine learning. PMLR 37:1312–1320.
Scheggi, S., Montis, d. e., and Gambarana, M. G. (2018). “DARPP-32 in the orchestration of responses to positive natural stimuli,” in Journal of Neurochemistry (Vol. 147, Issue 4). doi: 10.1111./jnc.14558
Schiffmann, S. N. (1998). Modulation of the voltage-gated sodium current in rat striatal neurons by DARPP-32, an inhibitor of protein phosphatase. Eur. J. Neurosci. 10, 142. doi: 10.1046./j.1460-9568.1998.00142.x
Schultz, W., Dayan, P., and Montague, P. R. (1997). A neural substrate of prediction and reward. Science 275, 1593 doi: 10.1126./science.275.5306.1593
Schulz, E., Franklin, N. T., and Gershman, S. J. (2020). Finding structure in multi-armed bandits. Cogn. Psychol. 119, 1261. doi: 10.1016./j.cogpsych.2019.101261
Schulz, E., Konstantinidis, E., and Speekenbrink, M. (2018a). Putting bandits into context: how function learning supports decision making. J. Exp. Psychology: Learn. Mem. Cogn. 44, 463. doi: 10.1037./xlm0000463
Schulz, E., Speekenbrink, M., and Krause, A. (2018b). A tutorial on Gaussian process regression: modelling, exploring, and exploiting functions. J. Math. Psychol. 85, 1. doi: 10.1016/j.jmp.03001
Schulz, E., Wu, C. M., Huys, Q. J. M., Krause, A., and Speekenbrink, M. (2018c). Generalization and search in risky environments. Cogn. Sci. 42, 695. doi: 10.1111./cogs.12695
Schulz, E., Wu, C. M., Ruggeri, A., and Meder, B. (2019). Searching for rewards like a child means less generalization and more directed exploration. Psychol. Sci. 30, 11. doi: 10.1177./0956797619863663
Shepard, R. N. (1987). Toward a universal law of generalization for psychological science. Science 237, 4820. doi: 10.1126./science.3629243
Shi, L., Feldman, N., and Griffiths, T. (2008). Performing Bayesian inference with exemplar models. Proceedings of the 30th Annual Conference of the Cognitive Science Society. Available online at: https://escholarship.org/uc/item/4kt2j29t
Shi, L., Griffiths, T. L., Feldman, N. H., and Sanborn, A. N. (2010). Exemplar models as a mechanism for performing Bayesian inference. Psychon. Bullet. Rev. 17, 443. doi: 10.3758./PBR.17.4.443
Shohamy, D., and Wagner, A. D. (2008). Integrating memories in the human brain: hippocampal-midbrain encoding of overlapping events. Neuron 60, 23. doi: 10.1016/j.neuron.09023
Silberman, E. K., Weingartner, H., and Post, R. M. (1983). Thinking disorder in depression: logic and strategy in an abstract reasoning task. Arch. Gen. Psychiatry 40, 3009. doi: 10.1001./archpsyc.1983.01790060073009
Sims, C. R. (2018). Efficient coding explains the universal law of generalization in human perception. Science 360, 1118. doi: 10.1126./science.aaq1118
Smith, J. M. (1978). Optimization theory in evolution. Ann. Rev. Ecol. Syst. 9, 335. doi: 10.1146./annurev.es.09.110178.000335
Smith, R., Taylor, S., Wilson, R. C., Chuning, A. E., Persich, M. R., Wang, S., et al. (2022). Lower levels of directed exploration and reflective thinking are associated with greater anxiety and depression. Front. Psychiatry 12, 136. doi: 10.3389./fpsyt.2021.782136
Steenkamp, J. B. E. M., and Gielens, K. (2003). Consumer and market drivers of the trial probability of new consumer packaged goods. J. Cons. Res. 30, 8615. doi: 10.1086./378615
Stephen, K., and Bergson, H. (2018). “Matter and memory,” in Misuse of Mind (pp. 75–107). doi: 10.4324./9781315822990-3
Stojic, H., Schulz, E., Analytis, P. P., and Speekenbrink, M. (2020). It's new, but is it good? how generalization and uncertainty guide the exploration of novel options. J. Exp. Psychol. Gen. 4, 749. doi: 10.1037./xge0000749
Strand, M. R., and Vinson, S. B. (1982). Behavioral response of the parasitoid cardiochiles nigriceps to a kairomone. Entomol. Exp. et Applicata 31, 3150. doi: 10.1111./j.1570-7458.1982.tb03150.x
Struyf, D., Zaman, J., Hermans, D., and Vervliet, B. (2017). Gradients of fear: How perception influences fear generalization. Behav. Res. Therapy 93, 1. doi: 10.1016/j.brat.04, 001.
Sutton, R. S., and Barto, A. G. (1998). Reinforcement learning: an introduction. IEEE Transact. Neural Networks 9, 2192. doi: 10.1109./tnn.1998.712192
Taylor, J. E., Cortese, A., Barron, H. C., Pan, X., Sakagami, M., Zeithamova, D., et al. (2021). How do we generalize? Neurons Behav. Data Anal. Theory. 4, 7867. doi: 10.51628./001c.27687
Tinbergen, N., Impekoven, M., and Franck, D. (1967). An experiment on spacing-out as a defence against predation. Behaviour 28, 64. doi: 10.1163./156853967X00064
Tolman, E., and Honzik, C. (1930). “Introduction and removal of reward, and maze performance in rats,” in University of California Publications in Psychology (Vol. 4).
Tomov, M. S., Truong, V. Q., Hundia, R. A., and Gershman, S. J. (2020). Dissociable neural correlates of uncertainty underlie different exploration strategies. Nature Commun. 11, 766. doi: 10.1038./s41467-020-15766-z
Valentino, R. J., and Foote, S. L. (1988). Corticotropin-releasing hormone increases tonic but not sensory-evoked activity of noradrenergic locus coeruleus neurons in unanesthetized rats. J. Neurosci. 8, 198. doi: 10.1523./jneurosci.08-03-01016.1988
van Dooren, R., Kleijn, d. e., Hommel, R. B., and Sjoerds, Z. (2021). The exploration-exploitation trade-off in a foraging task is affected by mood-related arousal and valence. Cogn. Affect. Behav. Neurosci. 21, 3. doi: 10.3758./s13415-021-00917-6
Voss, J. L., Gonsalves, B. D., Federmeier, K. D., Tranel, D., and Cohen, N. J. (2011a). Hippocampal brain-network coordination during volitional exploratory behavior enhances learning. Nature Neurosci. 14, 1. doi: 10.1038./nn.2693
Voss, J. L., Warren, D. E., Gonsalvesa, B. D., Federmeier, K. D., Tranel, D., Cohen, N. J., et al. (2011b). Spontaneous revisitation during visual exploration as a link among strategic behavior, learning, and the hippocampus. Proceed. Nat. Acad. Sci. USA, 108, 31. doi: 10.1073./pnas.1100225108
Vrieze, E., Pizzagalli, D. A., Demyttenaere, K., Hompes, T., Sienaert, P., de Boer, M., and Claes, S. (2013). Reduced reward learning predicts outcome in major depressive disorder. Biol. Psychiatry 73, 14. doi: 10.1016/j.biopsych.10014
Warren, C. M., Wilson, R. C., van der Wee, N. J., Giltay, E. J., van Noorden, M. S., Cohen, J. D., et al. (2017). The effect of atomoxetine on random and directed exploration in humans. PLoS ONE, 12, 6034. doi: 10.1371./journal.pone.0176034
Wiehler, A., Chakroun, K., and Peters, J. (2021). Attenuated directed exploration during reinforcement learning in gambling disorder. J. Neurosci. 41, 11. doi: 10.1523./JNEUROSCI.1607-20.2021
Wilson, R. C., Geana, A., White, J. M., Ludvig, E. A., and Cohen, J. D. (2014). Humans use directed and random exploration to solve the explore-exploit dilemma. J. Exp. Psychol. General 143, 8199. doi: 10.1037./a0038199
Wu, C. M., Schulz, E., Garvert, M. M., Meder, B., and Schuck, N. W. (2020). Similarities and differences in spatial and non-spatial cognitive maps. PLoS Comp. Biol. 16, 8149. doi: 10.1371./JOURNAL.PCBI.1008149
Wu, C. M., Schulz, E., and Gershman, S. J. (2021). Inference and search on graph-structured spaces. Comp. Brain Behav. 4, 91. doi: 10.1007./s42113-020-00091-x
Wu, C. M., Schulz, E., Pleskac, T. J., and Speekenbrink, M. (2022). Time pressure changes how people explore and respond to uncertainty. Sci. Reports 12, 1. doi: 10.1038./s41598-022-07901-1
Wu, C. M., Schulz, E., Speekenbrink, M., Nelson, J. D., and Meder, B. (2018). “Generalization guides human exploration in vast decision spaces,” in Nature Human Behaviour (Vol. 2, Issue 12). doi: 10.1038./s41562-018-0467-4
Yan, Z., Hsieh-Wilson, L., Feng, J., Tomizawa, K., Allen, P. B., Fienberg, A. A., et al. (1999). Protein phosphatase 1 modulation of neostriatal AMPA channels: regulation by DARPP-32 and spinophilin. Nature Neurosci. 2, 4516. doi: 10.1038./4516
Zajkowski, W. K., Kossut, M., and Wilson, R. C. (2017). A causal role for right frontopolar cortex in directed, but not random, exploration. ELife 6, 27430. doi: 10.7554./eLife.27430
Zajonc, R. B. (2001). Mere exposure: a gateway to the subliminal. Curr. Direct. Psychol. Sci. 10, 6. doi: 10.1111./1467-8721.00154
Keywords: explore-exploit dilemma, generalization (psychology), Gaussian process (GP), reinforcement learning, uncertainty, optimal foraging
Citation: Houser TM (2023) How generalization relates to the exploration-exploitation tradeoff. Front. Cognit. 2:1132766. doi: 10.3389/fcogn.2023.1132766
Received: 27 December 2022; Accepted: 29 June 2023;
Published: 19 July 2023.
Edited by:
Aaron Gruber, University of Lethbridge, CanadaReviewed by:
Christian Lebiere, Carnegie Mellon University, United StatesLihui Wang, Shanghai Jiao Tong University, China
Eric Chalmers, Mount Royal University, Canada
Copyright © 2023 Houser. This is an open-access article distributed under the terms of the Creative Commons Attribution License (CC BY). The use, distribution or reproduction in other forums is permitted, provided the original author(s) and the copyright owner(s) are credited and that the original publication in this journal is cited, in accordance with accepted academic practice. No use, distribution or reproduction is permitted which does not comply with these terms.
*Correspondence: Troy M. Houser, dGhvdXNlciYjeDAwMDQwO3VvcmVnb24uZWR1