- 1Department of Psychology, Vanderbilt University, Nashville, TN, United States
- 2Department of Psychology, Sungshin Women's University, Seoul, South Korea
Over the past three decades, object recognition moved away from domain-general questions, favoring investigation of putative category-specific mechanisms. But growing interest in individual differences in object recognition is putting the spotlight back on domain-general mechanisms, supporting their existence rather than simply assuming them. We reflect on the relative progress in three areas of visual cognition—visual object recognition, ensemble perception, and holistic processing. The study of visual object recognition abilities, based on latent variable modeling, provides strong evidence for domain-general mechanisms with neural correlates in visual areas and contributions to many real-world visual problems. The study of ensemble perception includes evidence for interesting individual differences but the structure of abilities is unclear, with a need for multivariate investigation. The study of individual differences in holistic processing has been unable to establish the construct validity of its measures. Each of these areas is at a different stage of understanding relevant underlying abilities, each one helping to illustrate different challenges that may be unfamiliar to experimentalists.
A pendulum swing on domain-generality
How did early debates in human visual cognition influence research on individual differences in high-level vision? Two topics were particularly important: how to deal with the problem of invariance (Lowe, 1987) and what to make of category-specific effects (Sergent et al., 1992). The invariance debate contrasted one view in which object representations abstract away from viewpoint-induced variability (Biederman, 1987) to another in which object representations preserve information from experienced views (Bülthoff et al., 1995). Non-human physiology had paved the way for these debates (e.g., Gross et al., 1969; Riesenhuber and Poggio, 1999) and as neural evidence supported both models (Tanaka, 1996), increased access to brain imaging spurred a new focus about the meaning of category-specific neural responses (Kanwisher et al., 1997) and by extension, of category-specific behavioral effects (Tanaka and Farah, 1993; Farah et al., 1995).
Slowly, emphasis shifted from domain-general to domain-specific mechanisms. Marr's foundational work (Marr and Nishihara, 1978), concerned the common act of recognizing objects at their basic-level, as cats or as chairs (Rosch et al., 1976; Biederman, 1987). Even when novel objects were used to study subordinate-level recognition (Tarr et al., 1998), the point was never to understand how people recognize strange-looking objects. Novel objects can stand for any object that could become familiar and can inform general object recognition functions. As the study of category-specificity encouraged specialization into sub-fields along category lines (e.g., faces, words, scenes), face recognition became a darling of cognitive neuroscience, in part due to domain-specific claims of its mechanisms (Kanwisher, 2000). Along the way, behavioral research in object recognition all but appeared to abandon questions about domain-general mechanisms.
A growing focus on individual differences in vision (e.g., Duchaine and Nakayama, 2006; Wilmer, 2008; McGugin et al., 2012; Richler et al., 2019; Meyer et al., 2021) is swinging the pendulum back to domain-general questions. A Web of Science search for articles with keywords of “individual differences/ability” and “face/object”, in psychology or neuroscience, shows <50 publications/year between 1990 and 1994, and more than 500 publications/year in the years 2017 to 2021. We review recent work that uses psychometrically-sound measurements of object recognition skills and confirmatory factor analysis to characterize domain-general abilities (that generalize over tasks and categories). We then turn to the area of ensemble perception, where good measurement is achieved but the lack of multivariate studies limits knowledge about domain-general abilities. Finally, we discuss why holistic processing has not benefited from multivariate approaches, as it deals with serious challenges with measurement and construct validation.
O: Pairwise correlations can underestimate domain-general effects
Initially, perceptual expertise seemed to pertain to rare individuals (Diamond and Carey, 1986), but later studies revealed high variability in the general population. Capturing a continuum of performance seemed more appropriate than dichotomizing expertise (Barton et al., 2009; Van Gulick et al., 2016). Behavioral hallmarks of expertise arise rapidly in training studies (Gauthier and Tarr, 1997; Wong et al., 2009; Chua et al., 2014; Chua and Gauthier, 2020b), and perceptual expertise may represent the high-end of the normal curve for the general population. But to ask whether a general ability transcends categories despite large effects of experience, it is critical to sample a broad range of categories.
Good psychometric measures for the recognition of many object categories moved the field ahead. Back when reliable tests existed only for faces and cars, it was challenging to interpret a correlation of r = 0.37 between face and car memory tests (Dennett et al., 2012). It was unclear if cars could stand for all objects (they cannot, see Cepulić et al., 2018; Sunday et al., 2019), and what kind of correlation to expect between different object categories. To address this, new tests were created (e.g., for cars, planes, birds, leaves, shoes… McGugin et al., 2012; Van Gulick et al., 2016). Pairwise correlation between any two non-face categories (r = 0.34, or 12% shared variance) was no larger than the observed correlation between faces and cars. In addition, because all these tests had the same format (learn, then recognize 6 target items in a series of trials), some of the shared variance was possibly attributable to task requirements.
Interpretation is challenging when dissociations are between pairs of tests rather than between latent variables that use several tasks to target constructs (Russell et al., 1998). Thus, we turned to a latent-variable approach (Bollen and Hoyle, 2012) which had already been used to study the structure of face perception (Wilhelm et al., 2010; Kaltwasser et al., 2014; Rostami et al., 2017) and tested 246 subjects with 3 different tests that differed in various requirements (e.g., memory vs. perceptual task, attention to wholes vs. parts, Richler et al., 2019). Five novel object categories were used to avoid confounds from experience. They varied on perceptual dimensions known to recruit different parts of the visual system (e.g., animacy or symmetry). We used confirmatory factor analysis (or CFA, a special case of structural equation modeling, or SEM) to create a latent variable for each category. For instance, the ability to recognize curvy animate symmetrical “greeble” objects was measured through three different tasks that used greebles. We found support for an overarching ability accounting for 89% of the variance in these five lower-order category factors (Figure 1). This high-order factor represents a domain-general object recognition ability, which we called “o”. We found that o dissociates from general intelligence (Richler et al., 2017, 2019).
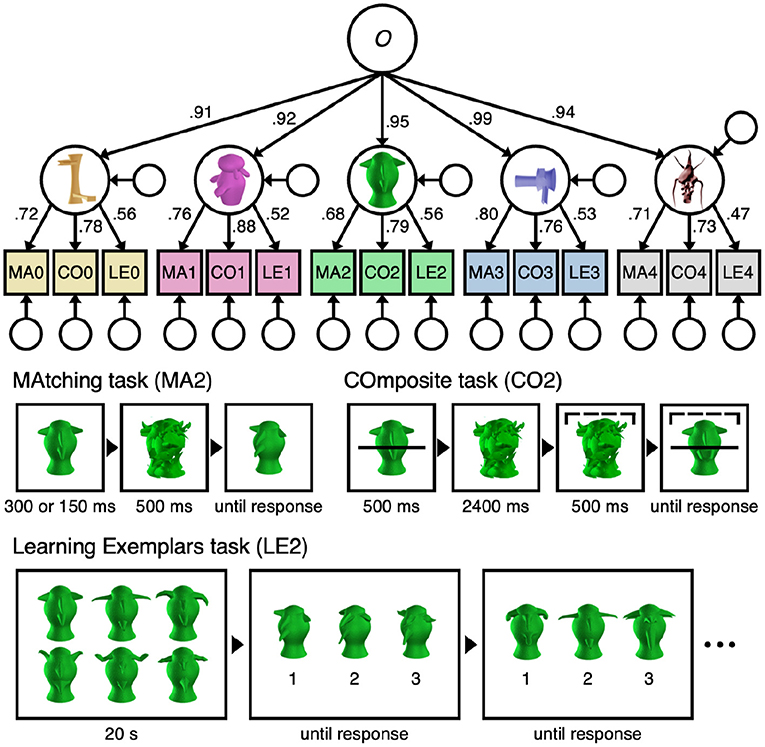
Figure 1. Hierarchical model for OR and experimental procedures for the indicators. In the model shown in the top, a circle with “o” represents a latent variable for the domain-general OR ability, circles with novel object images represent OR ability for specific categories, and empty circles represent measurement errors. Squares represent indicators (behavioral tests), which are exemplified in the middle and the bottom. This hierarchical model suggests that, when measurement errors are accounted for and latent variables for OR abilities for specific object categories are inferred, large portion of the variance in OR abilities is explained with “o.” In the middle left is an example of a single trial of the matching task. Participants judged whether the two images showed the same exemplar, regardless of the viewpoints. In the middle right is an example of a single trial of the composite task. Participants judged whether the cued parts (top or bottom, top part cued with a dashed line in this example) were the same between the two images, regardless of the non-cued part (bottom in this example). In the bottom is an example of the study phase and two trials of the test phase of the learning exemplars task. Participants first studied 6 exemplars, and then judged which one of the 3 images was the studied exemplar, regardless of the viewpoints.
This 89% domain-general variance may seem incommensurable with the approximately 12% shared variance across categories based on pairwise correlations. The high amount of variance accounted by o, or for that matter a nearly perfect correlation between abilities for novel and familiar objects, only make sense once we remember that the interpretation lies at the level of latent variables. In the same study where o accounted for 89% of the variance in performance within a category, the bivariate correlations between specific tests, especially pairs with different tasks and different categories, are themselves in the range of r = 0.3 to.4. CFA allows us to quantify relations after excluding aspects of performance that are not due to the substantive constructs of interest (e.g., task differences or random error). The magnitude of correlations between constructs can be underestimated by other approaches (Little et al., 1999). Even when correlations in CFA are modest, we can have more confidence in interpreting the meaning of the unshared variance in terms of domain-specific effects at the level of constructs, rather than measures.
We have used o to predict learning on a lung nodule detection task, over and above experience in that field and g (Sunday et al., 2018; see also Trueblood et al., 2018; Carrigan et al., 2020). For reading musical notation, o and g contributed differently to performance in experts vs. novices (Chang and Gauthier, 2021b), suggesting there can be a shift between domain-general and domain-specific abilities during learning. Recently, o predicted performance in food recognition tasks (Gauthier and Fiestan, 2022), and being able to control for o was critical in revealing a food-specific recognition ability. The work of exploring o's boundaries and underlying mechanisms has just begun. Intriguingly, o correlates with performance on a haptic object recognition task, suggesting common mechanisms across modalities (Chow et al., 2021).
Like g, o is a statistical concept that begs the question of underlying mechanisms (Chabris, 2007). A recent fMRI study measured associations between a psychometrically-sensitive measure of o and a neurometrically-sensitive measure of visual sensitivity to shape (McGugin et al., 2022). Neural selectivity to shape was associated with o in several regions of the ventral pathway, and in parietal and premotor cortex. Multivariate analyses suggested a common mechanism behind the distributed effects in ventral cortex. Interestingly, the pattern of visual areas predicted by o resembles that recruited by “minimal recognizable configurations” (Holzinger et al., 2019), or critical features proposed to be atoms of object recognition (Ullman et al., 2002, 2016). Computational models of vision that can account for both the learning of such features and individual differences in object recognition may result in better explanations of human vision (Shen and Palmeri, 2016; Ben-Yosef et al., 2018; Annis et al., 2021).
EP: Multivariate work is necessary to propose a domain-general construct
Ensemble Perception (EP) is the ability that supports performance on judgments regarding the statistical summaries of a feature for a group of objects. You use EP when trying to choose the box of assorted chocolates that best suits your preferences or decide whether your audience is more offended or amused by a joke. EP is studied with a wide range of tasks and stimuli, including judgments for different statistics (e.g., mean, variance, range, numerosity) for simple visual features (e.g., size, orientation, color/hue, luminance/contrast, motion direction) (Brady and Alvarez, 2011; Haberman et al., 2015) or more complex features like face identity or expression (Haberman and Whitney, 2009). Some tasks show objects simultaneously, either briefly (Chong and Treisman, 2003) or not (Whiting and Oriet, 2011), while others present them sequentially (Haberman et al., 2009).
Despite all this work, it is unknown how many distinct abilities support EP judgments. Most individual differences studies of EP correlate only pairs of tasks, limiting interpretation. A second limitation is that such pairs of tasks usually only differ on a single dimension (e.g., mean length vs. mean orientation, mean orientation vs. orientation variance). This limits knowledge about a possible overarching domain-general EP ability.
One seminal paper (Haberman et al., 2015) found correlations around 0.4–0.6 between pairs of EP tasks for low-level features (average color and orientation) and for higher-level features (average face expression and identity), but lower correlations (0.1–0.3) across levels, and proposed two distinct EP abilities. These studies were strong due to their large sample size, but results based on bivariate correlations can be difficult to interpret. The authors required correlations between two EP tasks to exceed a small “floor” correlation (r = 0.21) between one EP task and a letter span task not expected to share any variance with EP. In one case, the correlation between color and face identity averaging tasks was 0.29, p = 0.003, significant but not different from the floor correlation. This logic assumes that the two EP tasks share variance due to the same influence that explains the overlap of one EP task and the letter span task. Unfortunately, this may not be a safe assumption. A stronger approach is to partial out unwanted sources of variance using regression (Chang and Gauthier, 2020; Gauthier, 2020).
Although much has been made of evidence that EP judgments can be made under conditions where single-item recognition fails (Haberman and Whitney, 2011), the two kinds of judgments are often correlated. Within the domains of high-level or low-level tasks, respectively, performance on EP judgments correlate with individual item judgments within the same category (Haberman et al., 2015). However, the only high-level category was faces. To address this shortcoming, we used regression to control for individual recognition of objects from 2 categories (i.e., cars and birds) as we estimated the partial correlation between average judgments of identity for arrays in each category (Chang and Gauthier, 2021a). We found a correlation between the Bird and Car EP tasks, controlling for the recognition of single objects. A regression approach was also successful in revealing a correlation between judgments of average size and of variability in size for arrays of circle (Cha et al., 2021), even when controlling for performance judging the size of single circles. These studies are consistent with at least one domain-general ability for EP judgments, relevant to most visual features and statistical summaries.
This progress suggests the feasibility of larger multivariate studies of EP abilities. In the only study so far to use CFA with EP tasks, we created a latent variable based on 6 indicators using average identity judgments for novel and familiar objects, and related it to o for novel and familiar objects (Sunday et al., 2021). Each of 6 different EP tests loaded strongly on a latent variable for EP, and that EP factor shared about 42% of the variance with o (Figure 2). By extension, nearly 60% of the shared variance between EP tests did not relate to o, suggesting possible EP-specific mechanisms (Chong et al., 2008). But while the construct coverage was strong for categories, it may have been biased because all EP measures used the same task (6-alternative forced choice for average identity of 4 objects).
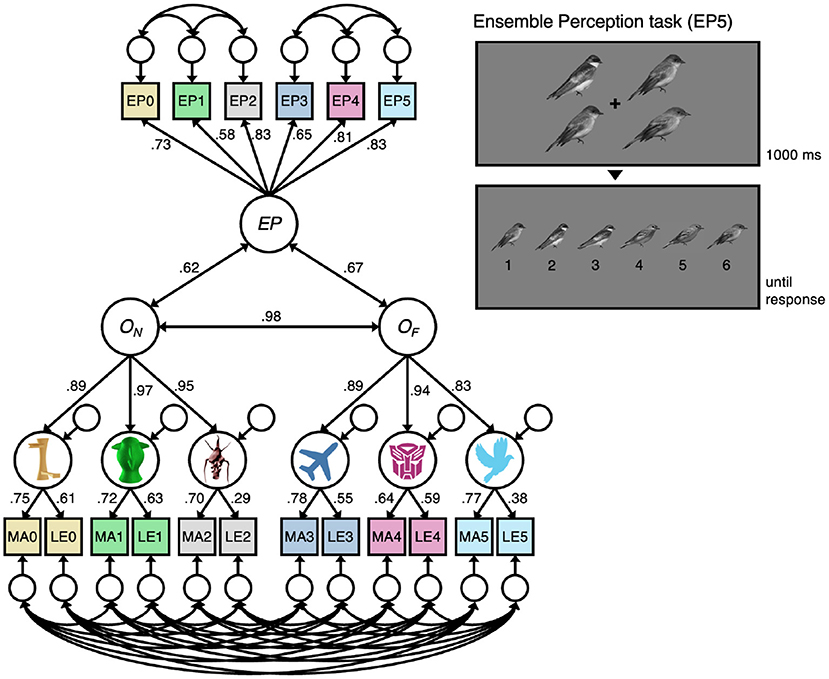
Figure 2. Hierarchical model for O and EP abilities, and experimental procedure for the EP indicator. In the model shown in the left, circles with “OF,” “ON,” and “EP” represent latent variables for OR ability for novel object, OR ability for familiar object, and EP ability, respectively. Circles with novel object images or familiar object icons represent OR ability for specific categories, and empty circles represent measurement errors. Squares represent indicators (behavioral tests). The model suggests that OR abilities are basically the same for novel and familiar objects and that EP ability has a unique variance that cannot be explained with OR abilities. In the top right is an example of a single trial of the EP task. Participants saw 4 images and chose one of the 6 images that was closest to the mean of the 4 images. See Figure 1 for examples of trials for the other indicators.
The study of EP is vibrant, with tasks that vary on many dimensions but are postulated to have something in common (Whitney and Yamanashi Leib, 2018). There is growing support for domain-general EP abilities that are separate from domain-general object recognition, and for a distinction between EP judgments for high- and low-level dimensions. We should be careful with theoretical implications of bivariate analyses, and seek construct validation in multivariate studies.
HP: When construct validity is not achieved
Holistic processing (HP) refers to a variety of effects whereby a whole object is processed better than parts of the object (Richler et al., 2012). HP is a theoretical hub in face recognition research. Across tasks targeting HP, faces consistently show large effects (Maurer et al., 2002; Richler and Gauthier, 2014) while non-face objects in novices do not (Wong et al., 2009; Chua and Gauthier, 2020a). There are exceptions whereby gestalt principles with shapes can produce HP (Zhao et al., 2016), but their relation to face HP is debated (Curby and Moerel, 2019; Curby et al., 2019). Judgments with non-face objects can result in HP as a function of experience, whether experience is measured (Gauthier et al., 2003; Bukach et al., 2010) or manipulated (Gauthier and Tarr, 1997; Chua and Gauthier, 2020b). But whether there is a domain-general influence on HP is a complicated question.
It has been difficult to establish a coherent latent HP construct to test this prediction. The typical finding is of small to non-existent correlations between HP tasks (Rezlescu et al., 2017; Gauthier, 2020; Boutet et al., 2021). The construct validity of any measure of HP is difficult to establish without these relationships. Even different versions of the same task can fail to correlate (Wang et al., 2016; Ventura et al., 2022). It is not always clear whether the reasons are substantive (Redick and Lindsey, 2013) and pointing to problems with the construct legitimacy (Stone, 2019) of HP, or are methodological (Draheim et al., 2019) and can eventually be resolved (Feest, 2020).
Unique challenges arise in the differential study of HP. One is the multiplicity of incommensurate meanings of HP (Richler et al., 2012). Another lies in measures that have insufficient reliability, in terms of consistently ranking individuals. Most measures of HP were not designed for individual differences and result in very low reliability (Ross et al., 2015), limited by the use of difference scores or response times (Peter et al., 1993; Draheim et al., 2019), both known to limit reliability.
A third challenge is that even reliable measures may still lack validity. For instance, the partial design of the composite task, a popular measure of HP, confounds HP effects and a response bias (Richler et al., 2009), rendering it susceptible to manipulations that should not affect HP. Some authors consider inversion effects (the difference between performance on upright vs. inverted images) to be a measure of HP, but other work reveals inverted faces can be processed holistically (Sekuler et al., 2004; Richler et al., 2011). When claims depend on single tasks because they fail to converge on latent variables, the choice of measure becomes their Achillle's heel. Progress may require even more work focused on measurement, either by shifting away from tasks designs for experimental purposes (Draheim et al., 2021) or by using sample sizes large enough for models to converge despite weak indicators (Unsworth et al., 2020).
But what if all measurement problems were solved and we found that latent variables for face HP and non-face object HP still do not correlate? A lack of a correlation between two latent variables suggest distinct abilities, but not necessarily different mechanisms. By one account, the domain-specificity of HP effect likely stems from the domain-specificity of experience (Gauthier and Tarr, 1997). This is not a new idea in psychology. For example, instance theory postulates that with practice and experience, performance shifts from being limited by properties of a general algorithm to being limited by the accumulation of knowledge (Logan, 1988). This can produce apparent qualitative changes with practice, even though the underlying mechanisms have not changed. There are clear demonstrations that HP for a category grows with experience (Wong et al., 2009; Chua et al., 2014, 2015). In one study, we parametrically manipulated experience to make predictions about the resulting pattern of correlations in HP for different object categories (Chua and Gauthier, 2020b), showing how differential and experimental methods can be integrated in theory building. It appears that recognition ability for objects is caused both by a domain-general trait like o and by experience, but that what drives holistic processing is entirely determined by experience [at least for non-face objects—explaining variability in face HP is more complicated (Gauthier, 2020)].
Concluding remarks
In martial arts, we say that the black belt is where the journey begins. With much yet to accomplish, the study of o may have just reached this stage. Latent factors can be instantiated and show strong convergent and divergent validity. It accounts for learning aptitude in real-world domains. The differential study of EP is moving rapidly toward this stage. Reliable measures for a variety of tasks are easily developed, as they do not require difference scores or the use of response times. However, the structure of abilities that support EP, and their relation to o, remains unclear because the empirical evidence is so far limited to pairwise correlations. As it stands, the study of individual differences in HP is still at white belt level. Individual differences in HP may not reflect coherent abilities, but interactions between domain-general mechanisms like attention (Chua et al., 2015) and experience, which can be a powerful category-specific influence.
Author contributions
All authors worked on the first version. IG edited the last version.
Funding
This work was supported by a grant from the National Science Foundation (BCS-1840896) and the David J. Wilson Chair Research Fund at Vanderbilt University.
Acknowledgments
We thank the members of the OPLab and CATLAB at Vanderbilt and Michael J. Tarr for their comments on an earlier version.
Conflict of interest
The authors declare that the research was conducted in the absence of any commercial or financial relationships that could be construed as a potential conflict of interest.
Publisher's note
All claims expressed in this article are solely those of the authors and do not necessarily represent those of their affiliated organizations, or those of the publisher, the editors and the reviewers. Any product that may be evaluated in this article, or claim that may be made by its manufacturer, is not guaranteed or endorsed by the publisher.
References
Annis, J., Gauthier, I., and Palmeri, T. J. (2021). Combining convolutional neural networks and cognitive models to predict novel object recognition in humans. J. Exp. Psychol. Learn. Mem. Cogn. 47, 785–807. doi: 10.1037/xlm0000968
Barton, J. J. S., Hanif, H., and Ashraf, S. (2009). Relating visual to verbal semantic knowledge: The evaluation of object recognition in prosopagnosia. Brain 132, 3456–3466. doi: 10.1093/brain/awp252
Ben-Yosef, G., Assif, L., and Ullman, S. (2018). Full interpretation of minimal images. Cognition. 171, 65–84. doi: 10.1016/j.cognition.2017.10.006
Biederman, I. (1987). Recognition-by-components: A theory of human image understanding. Psychol. Rev. 94, 115–147. doi: 10.1037/0033-295X.94.2.115
Boutet, I., Nelson, E. A., Watier, N., Cousineau, D., Béland, S., Collin, C. A., et al. (2021). Different measures of holistic face processing tap into distinct but partially overlapping mechanisms . Atten. Percept. Psychophys. 83, 2905–2923. doi: 10.3758/s13414-021-02337-7
Brady, T. F., and Alvarez, G. A. (2011). Hierarchical encoding in visual working memory: ensemble statistics bias memory for individual items. Psychol. Sci., 22, 384–392. doi: 10.1177/0956797610397956
Bukach, C. M., Phillips, W. S., and Gauthier, I. (2010). Limits of generalization between categories and implications for theories of category specificity. Atten. Percept. Psychophys. 72, 1865–1874. doi: 10.3758/APP.72.7.1865
Bülthoff, H. H., Edelman, S. Y., and Tarr, M. J. (1995). How are three-dimensional objects represented in the brain? Cereb. Cortex 5, 247–260. doi: 10.1093/cercor/5.3.247
Carrigan, A. J., Stoodley, P., Fernandez, F., Sunday, M. A., and Wiggins, M. W. (2020). Individual differences in echocardiography: Visual object recognition ability predicts cue utilization. Appl. Cogn. Psychol. 34, 1369–1378. doi: 10.1002/acp.3711
Cepulić, D-, B., Wilhelm, O., Sommer, W., and Hildebrandt, A. (2018). All categories are equal, but some categories are more equal than others: The psychometric structure of object and face cognition. J. Exp. Psychol. Learn. Mem. Cogn. 44, 1254–1268. doi: 10.1037/xlm0000511
Cha, O., Blake, R., and Gauthier, I. (2021). Contribution of a common ability in average and variability judgments. Psychon. Bull. Rev. 29, 1–8. doi: 10.3758/s13423-021-01982-1
Chabris, C. F. (2007). “Cognitive and neurobiological mechanisms of the Law of General Intelligence,” in Integrating the Mind: Domain General vs Domain Specific Processes in Higher Cognition, eds M. J. Roberts (London, England: Psychology Press), 449–491.
Chang, T., Y., and Gauthier, I. (2021a). Domain-general ability underlies complex object ensemble processing. J. Exp. Psychol. Gen. 151, 966–972. doi: 10.1037/xge0001110
Chang, T., Y., and Gauthier, I. (2021b). Domain-specific and domain-general contributions to reading musical notation. Atten. Percept. Psychophys. 83, 2983–2994. doi: 10.3758/s13414-021-02349-3
Chang, T.-., Y., and Gauthier, I. (2020). Distractor familiarity reveals the importance of configural information in musical notation. Atten. Percept. Psychophys. 82, 1304–1317. doi: 10.3758/s13414-019-01826-0
Chong, S. C., Joo, S. J., Emmmanouil, T.-., A., and Treisman, A. (2008). Statistical processing: Not so implausible after all. Percept. Psychophys. 70, 1327–1334. doi: 10.3758/PP.70.7.1327
Chong, S. C., and Treisman, A. (2003). Representations of statistical properties. Vis. Res. 43, 393–404. doi: 10.1016/S0042-6989(02)00596-5
Chow, J. K., Palmeri, T. J., and Gauthier, I. (2021). Haptic object reCogn. based on shape relates to visual object recognition ability. Psychol. Res., 1–12. doi: 10.1167/jov.22.7.1
Chua, K.-., W., and Gauthier, I. (2020a). The vanderbilt holistic processing tests for novel objects: validation in novice participants. J. Expert., 3, 20–31.
Chua, K.-., W., and Gauthier, I. (2020b). Domain-specific experience determines individual differences in holistic processing. J. Exp. Psychol. Gen., 149, 31–41. doi: 10.1037/xge0000628
Chua, K.-., W., Richler, J. J., and Gauthier, I. (2014). Becoming a Lunari or Taiyo expert: learned attention to parts drives holistic processing of faces. J. Exp. Psychol. Hum. Percept. Perform., 40, 1174–1182. doi: 10.1037/a0035895
Chua, K.-., W., Richler, J. J., and Gauthier, I. (2015). Holistic processing from learned attention to parts. J. Exp. Psychol. Gen. 144, 723–729. doi: 10.1037/xge0000063
Curby, K. M., Huang, M., and Moerel, D. (2019). Multiple paths to holistic processing: Holistic processing of Gestalt stimuli do not overlap with holistic face processing in the same manner as do objects of expertise. Atten. Percept. Psychophys. 81, 716–726. doi: 10.3758/s13414-018-01643-x
Curby, K. M., and Moerel, D. (2019). Behind the face of holistic perception: holistic processing of Gestalt stimuli and faces recruit overlapping perceptual mechanisms. Atten. Percept. Psychophys. 81, 2873–2880. doi: 10.3758/s13414-019-01749-w
Dennett, H. W., McKone, E., Tavashmi, R., Hall, A., Pidcock, M., Edwards, M., et al. (2012). The Cambridge Car Memory Test: A task matched in format to the Cambridge Face Memory Test, with norms, reliability, sex differences, dissociations from face memory, and expertise effects. Behav. Res. Methods 44, 587–605. doi: 10.3758/s13428-011-0160-2
Diamond, R., and Carey, S. (1986). Why faces are and are not special: An effect of expertise. J. Exp. Psychol. Gen. 115, 107–117. doi: 10.1037/0096-3445.115.2.107
Draheim, C., Mashburn, C. A., Martin, J. D., and Engle, R. W. (2019). Reaction time in differential and developmental research: A review and commentary on the problems and alternatives. Psychol. Bull. 145, 508–535. doi: 10.1037/bul0000192
Draheim, C., Tsukahara, J. S., Martin, J. D., Mashburn, C. A., and Engle, R. W. (2021). A toolbox approach to improving the measurement of attention control. J. Exp. Psychol.: General 150, 242–275. doi: 10.1037/xge0000783
Duchaine, B., and Nakayama, K. (2006). The Cambridge Face Memory Test: Results for neurologically intact individuals and an investigation of its validity using inverted face stimuli and prosopagnosic participants. Neuropsychologia 44, 576–585. doi: 10.1016/j.neuropsychologia.2005.07.001
Farah, M. J., Tanaka, J. W., and Drain, H. M. (1995). What causes the face inversion effect? J. Exp. Psychol. Hum. Percept. Perform. 21, 628–634. doi: 10.1037/0096-1523.21.3.628
Feest, U. (2020). Construct validity in psychological tests–the case of implicit social cognition. Eur. J. Philos. Sci. 10, 1–24. doi: 10.1007/s13194-019-0270-8
Gauthier, I. (2020). What we could learn about holistic face processing only from nonface objects. Curr. Dir. Psychol. Sci., 29, 419–425. doi: 10.1177/0963721420920620
Gauthier, I., Curran, T., Curby, K. M., and Collins, D. (2003). Perceptual interference supports a non-modular account of face processing. Nat Neurosci. 6, 428–432. doi: 10.1038/nn1029
Gauthier, I., and Fiestan, G. (2022). Food neophobia predicts visual ability in the recognition of prepared food. Food Qual. Pref. 103, 104702. doi: 10.1016/j.foodqual.2022.104702
Gauthier, I., and Tarr, M. J. (1997). Becoming a “greeble” expert: exploring mechanisms for face recognition. Vis. Res. 37, 1673–1682. doi: 10.1016/S0042-6989(96)00286-6
Gross, C. G., Bender, D. B., and Rocha-Miranda, C. D. (1969). Visual receptive fields of neurons in inferotemporal cortex of the monkey. Science 166, 1303–1306. doi: 10.1126/science.166.3910.1303
Haberman, J., Brady, T. F., and Alvarez, G. A. (2015). Individual differences in ensemble perception reveal multiple, independent levels of ensemble representation. J. Exp. Psychol. Gen. 144, 432–446. doi: 10.1037/xge0000053
Haberman, J., Harp, T., and Whitney, D. (2009). Averaging facial expression over time. J. Vis. 9, 1–13. doi: 10.1167/9.11.1
Haberman, J., and Whitney, D. (2009). Seeing the mean: Ensemble coding for sets of faces. J. Exp. Psychol. Hum. Percept. Perform., 35, 718–734. doi: 10.1037/a0013899
Haberman, J., and Whitney, D. (2011). Efficient summary statistical representation when change localization fails. Psychon. Bull. Rev., 18, 855–859. doi: 10.3758/s13423-011-0125-6
Holzinger, Y., Ullman, S., Harari, D., Behrmann, M., and Avidan, G. (2019). Minimal recognizable configurations elicit category-selective responses in higher order visual cortex. J. Cogn. Neurosci. 31, 1354–1367. doi: 10.1162/jocn_a_01420
Kaltwasser, L., Hildebrandt, A., Recio, G., Wilhelm, O., and Sommer, W. (2014). Neurocognitive mechanisms of individual differences in face cognition: A replication and extension. Cogn. Affect. Behav. Neurosci. 14, 861–878. doi: 10.3758/s13415-013-0234-y
Kanwisher, N. (2000). Domain specificity in face perception. Nat. Neurosci. 3, 759–763. doi: 10.1038/77664
Kanwisher, N., McDermott, J., and Chun, M. M. (1997). The fusiform face area: a module in human extrastriate cortex specialized for face perception. J. Neurosci., 17, 4302–4311. doi: 10.1523/JNEUROSCI.17-11-04302.1997
Little, T. D., Lindenberger, U., and Nesselroade, J. R. (1999). On selecting indicators for multivariate measurement and modeling with latent variables: When “good” indicators are bad and “bad” indicators are good. Psychol. Methods 4, 192–211. doi: 10.1037/1082-989X.4.2.192
Logan, G. D. (1988). Toward an instance theory of automatization. Psychol. Rev. 95, 492–527. doi: 10.1037/0033-295X.95.4.492
Lowe, D. G. (1987). Three-dimensional object recognition from single two-dimensional images. A.I., 31, 355–395. doi: 10.1016/0004-3702(87)90070-1
Marr, D., and Nishihara, H. K. (1978). Representation and recognition of the spatial organization of three-dimensional shapes. Proc. Royal Soc. B., 200, 269–294. doi: 10.1098/rspb.1978.0020
Maurer, D., Le Grand, R., and Mondloch, C. J. (2002). The many faces of configural processing. Trends Cogn. Sci., 6, 255–260. doi: 10.1016/S1364-6613(02)01903-4
McGugin, R. W., Richler, J. J., Herzmann, G., Speegle, M., and Gauthier, I. (2012). The vanderbilt expertise test reveals domain-general and domain-specific sex effects in object recognition. Vis. Res., 69, 10–22. doi: 10.1016/j.visres.2012.07.014
McGugin, R. W., Sunday, M. A., and Gauthier, I. (2022). The neural correlates of domain-general visual ability. Cerebral Cortex. bhac342. doi: 10.1093/cercor/bhac342
Meyer, K., Sommer, W., and Hildebrandt, A. (2021). Reflections and new perspectives on face cognition as a specific socio-cognitive ability. J. Intell. 9, Article 2. doi: 10.3390/jintelligence9020030
Peter, J. P., Churchill Jr, G. A., and Brown, T. J. (1993). Caution in the use of difference scores in consumer research. J. Consum. Res. 19, 655–662.
Redick, T. S., and Lindsey, D. R. (2013). Complex span and n-back measures of working memory: a meta-analysis. Psychon. Bull. Rev., 20, 1102–1113. doi: 10.3758/s13423-013-0453-9
Rezlescu, C., Susilo, T., Wilmer, J. B., and Caramazza, A. (2017). The inversion, part-whole, and composite effects reflect distinct perceptual mechanisms with varied relationships to face recognition. J. Exp. Psychol. Hum. Percept. Perform., 43, 1961–1973. doi: 10.1037/xhp0000400
Richler, J. J., Cheung, O. S., Wong, A. C. N., and Gauthier, I. (2009). Does response interference contribute to face composite effects? Psychon. Bull. Rev., 16, 258–263. doi: 10.3758/PBR.16.2.258
Richler, J. J., and Gauthier, I. (2014). A meta-analysis and review of holistic face processing. Psychol. Bull. 140, 1281–1302. doi: 10.1037/a0037004
Richler, J. J., Mack, M. L., Palmeri, T. J., and Gauthier, I. (2011). Inverted faces are (eventually) processed holistically. Vis. Res., 51, 333–342. doi: 10.1016/j.visres.2010.11.014
Richler, J. J., Palmeri, T. J., and Gauthier, I. (2012). Meanings, mechanisms, and measures of holistic processing. Front. Psychol. 3, 553. doi: 10.3389/fpsyg.2012.00553
Richler, J. J., Tomarken, A. J., Sunday, M. A., Vickery, T. J., Ryan, K. F., Floyd, R. J., et al. (2019). Individual differences in object recognition. Psychol. Rev., 126, 226–251. doi: 10.1037/rev0000129
Richler, J. J., Wilmer, J. B., and Gauthier, I. (2017). General object recognition is specific: evidence from novel and familiar objects. Cogn. 166, 42–55. doi: 10.1016/j.cognition.2017.05.019
Riesenhuber, M., and Poggio, T. (1999). Hierarchical models of object recognition in cortex. Nat. Neurosci. 2, 1019–1025. doi: 10.1038/14819
Rosch, E., Mervis, C. B., Gray, W. D., Johnson, D. M., and Boyes-Braem, P. (1976). Basic objects in natural categories. Cogn. Psychol. 8, 382–439. doi: 10.1016/0010-0285(76)90013-X
Ross, D. A., Richler, J. J., and Gauthier, I. (2015). Reliability of composite-task measurements of holistic face processing. Behav. Res. Methods 47, 736–743. doi: 10.3758/s13428-014-0497-4
Rostami, H. N., Sommer, W., Zhou, C., Wilhelm, O., and Hildebrandt, A. (2017). Structural encoding processes contribute to individual differences in face and object cognition: inferences from psychometric test performance and event-related brain potentials. Cortex 95, 192–210. doi: 10.1016/j.cortex.2017.08.017
Russell, D. W., Kahn, J. H., Spoth, R., and Altmaier, E. M. (1998). Analyzing data from experimental studies: A latent variable structural equation modeling approach. J. Counsel. Psychol. 45, 18. doi: 10.1037/0022-0167.45.1.18
Sekuler, A. B., Gaspar, C. M., Gold, J. M., and Bennett, P. J. (2004). Inversion leads to quantitative, not qualitative, changes in face processing. Curr. Biol., 14, 391–396. doi: 10.1016/j.cub.2004.02.028
Sergent, J., Signoret, J.-., L., Bruce, V., and Rolls, E. T. (1992). Functional and anatomical decomposition of face processing: evidence from prosopagnosia and pet study of normal subjects [and discussion]. Philos. Trans. R. Soc. Lond., B, Biol. Sci., 335, 55–62. doi: 10.1098/rstb.1992.0007
Shen, J., and Palmeri, T. J. (2016). Modelling individual difference in visual categorization. Visual Cogn. 24, 260–283. doi: 10.1080/13506285.2016.1236053
Stone, C. (2019). A defense and definition of construct validity in psychology. Philos. Sci. 86, 1250–1261. doi: 10.1086/705567
Sunday, M. A., Dodd, M. D., Tomarken, A. J., and Gauthier, I. (2019). How faces (and cars) may become special. Vis. Res. 157, 202–212. doi: 10.1016/j.visres.2017.12.007
Sunday, M. A., Donnelly, E., and Gauthier, I. (2018). Both fluid intelligence and visual object recognition ability relate to nodule detection in chest radiographs. Appl. Cogn. Psychol., 32, 755–762. doi: 10.1002/acp.3460
Sunday, M. A., Tomarken, A. J., Cho, S.-., J., and Gauthier, I. (2021). Novel and familiar object recognition rely on the same ability. J. Exp. Psychol. Gen., 13, 93–110. doi: 10.1037/xge0001100
Tanaka, J. W., and Farah, M. J. (1993). Parts and wholes in face recognition. Q. J. Exp. Psychol., 46, 225–245. doi: 10.1080/14640749308401045
Tanaka, K. (1996). Inferotemporal cortex and object vision. Annu. Rev. Neurosci. 19, 109–139. doi: 10.1146/annurev.ne.19.030196.000545
Tarr, M. J., Williams, P., Hayward, W. G., and Gauthier, I. (1998). Three-dimensional object recognition is viewpoint dependent. Nat. Neurosci. 1, 275–277. doi: 10.1038/1089
Trueblood, J. S., Holmes, W. R., Seegmiller, A. C., Douds, J., Compton, M., Szentirmai, E., et al. (2018). The impact of speed and bias on the cognitive processes of experts and novices in medical image decision-making. Cogn. Res.: Princip. Implicat. 3, 28. doi: 10.1186/s41235-018-0119-2
Ullman, S., Assif, L., Fetaya, E., and Harari, D. (2016). Atoms of recognition in human and computer vision. Proc. Natl. Acad. Sci. 113, 2744–2749. doi: 10.1073/pnas.1513198113
Ullman, S., Vidal-Naquet, M., and Sali, E. (2002). Visual features of intermediate complexity and their use in classification. Nat. Neurosci. 5, 682–687. doi: 10.1038/nn870
Unsworth, N., Miller, A. L., and Robison, M. K. (2020). Are individual differences in attention control related to working memory capacity? A latent variable mega-analysis. J. Exp. Psychol. General. doi: 10.1037/xge0001000
Van Gulick, A. E., McGugin, R. W., and Gauthier, I. (2016). Measuring nonvisual knowledge about object categories: the semantic vanderbilt expertise test. Behav. Res. Methods 48, 1178–1196. doi: 10.3758/s13428-015-0637-5
Ventura, P., Ngan, V., Pereira, A., Cruz, F., Guerreiro, J. C., Rosário, V., et al. (2022). The relation between holistic processing as measured by three composite tasks and face processing: A latent variable modeling approach. Atten. Percep. Psychophys. 84, 2319–2334. doi: 10.3758/s13414-022-02543-x
Wang, C.-., C., Ross, D. A., Gauthier, I., and Richler, J. J. (2016). Validation of the Vanderbilt holistic face processing test. Front. Psychol. 7, 1837. doi: 10.3389/fpsyg.2016.01837
Whiting, B. F., and Oriet, C. (2011). Rapid averaging? Not so fast! Psychon. Bull. Rev., 18, 484–489. doi: 10.3758/s13423-011-0071-3
Whitney, D., and Yamanashi Leib, A. (2018). Ensemble Perception. Annu. Rev. Psychol. 69, 105–129. doi: 10.1146/annurev-psych-010416-044232
Wilhelm, O., Herzmann, G., Kunina, O., Danthiir, V., Schacht, A., Sommer, W., et al. (2010). Individual differences in perceiving and recognizing faces—One element of social cognition. J. Pers. Soc. Psychol., 99, 530–548. doi: 10.1037/a0019972
Wilmer, J. B. (2008). How to use individual differences to isolate functional organization, biology, and utility of visual functions; with illustrative proposals for stereopsis. Spatial Vis. 21, 561–579. doi: 10.1163/156856808786451408
Wong, A. C.-., N., Palmeri, T. J., and Gauthier, I. (2009). Conditions for Facelike Expertise With Objects: Becoming a Ziggerin Expert—but Which Type? Psychol. Sci. 20, 1108–1117. doi: 10.1111/j.1467-9280.2009.02430.x
Keywords: individual differences, visual abilities, multivariate approaches, object recognition, ensemble perception, holistic processing
Citation: Gauthier I, Cha O and Chang T-Y (2022) Mini review: Individual differences and domain-general mechanisms in object recognition. Front. Cognit. 1:1040994. doi: 10.3389/fcogn.2022.1040994
Received: 09 September 2022; Accepted: 26 October 2022;
Published: 09 November 2022.
Edited by:
Alice Mado Proverbio, University of Milano-Bicocca, ItalyReviewed by:
Hulusi Kafaligonul, Bilkent University, TurkeyCopyright © 2022 Gauthier, Cha and Chang. This is an open-access article distributed under the terms of the Creative Commons Attribution License (CC BY). The use, distribution or reproduction in other forums is permitted, provided the original author(s) and the copyright owner(s) are credited and that the original publication in this journal is cited, in accordance with accepted academic practice. No use, distribution or reproduction is permitted which does not comply with these terms.
*Correspondence: Isabel Gauthier, Isabel.gauthier@vanderbilt.edu