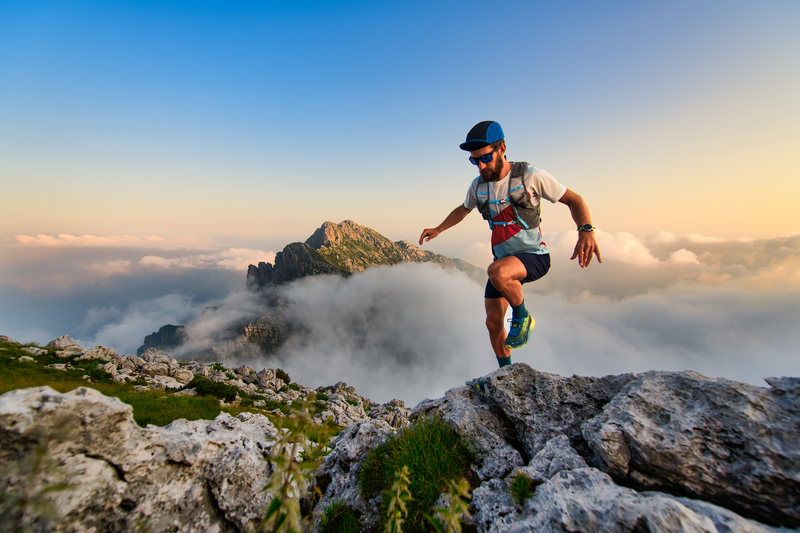
94% of researchers rate our articles as excellent or good
Learn more about the work of our research integrity team to safeguard the quality of each article we publish.
Find out more
REVIEW article
Front. Clin. Diabetes Healthc. , 21 September 2022
Sec. Diabetes and Pregnancy
Volume 3 - 2022 | https://doi.org/10.3389/fcdhc.2022.982665
This article is part of the Research Topic Frontiers in Clinical Diabetes and Healthcare - Rising Stars Africa View all 10 articles
Diabetes in pregnancy is associated with adverse pregnancy outcomes and poses a serious threat to the health of mother and child. Although the pathophysiological mechanisms that underlie the association between maternal diabetes and pregnancy complications have not yet been elucidated, it has been suggested that the frequency and severity of pregnancy complications are linked to the degree of hyperglycemia. Epigenetic mechanisms reflect gene-environment interactions and have emerged as key players in metabolic adaptation to pregnancy and the development of complications. DNA methylation, the best characterized epigenetic mechanism, has been reported to be dysregulated during various pregnancy complications, including pre-eclampsia, hypertension, diabetes, early pregnancy loss and preterm birth. The identification of altered DNA methylation patterns may serve to elucidate the pathophysiological mechanisms that underlie the different types of maternal diabetes during pregnancy. This review aims to provide a summary of existing knowledge on DNA methylation patterns in pregnancies complicated by pregestational type 1 (T1DM) and type 2 diabetes mellitus (T2DM), and gestational diabetes mellitus (GDM). Four databases, CINAHL, Scopus, PubMed and Google Scholar, were searched for studies on DNA methylation profiling in pregnancies complicated with diabetes. A total of 1985 articles were identified, of which 32 met the inclusion criteria and are included in this review. All studies profiled DNA methylation during GDM or impaired glucose tolerance (IGT), while no studies investigated T1DM or T2DM. We highlight the increased methylation of two genes, Hypoxia‐inducible Factor‐3α (HIF3α) and Peroxisome Proliferator-activated Receptor Gamma-coactivator-Alpha (PGC1-α), and the decreased methylation of one gene, Peroxisome Proliferator Activated Receptor Alpha (PPARα), in women with GDM compared to pregnant women with normoglycemia that were consistently methylated across diverse populations with varying pregnancy durations, and using different diagnostic criteria, methodologies and biological sources. These findings support the candidacy of these three differentially methylated genes as biomarkers for GDM. Furthermore, these genes may provide insight into the pathways that are epigenetically influenced during maternal diabetes and which should be prioritized and replicated in longitudinal studies and in larger populations to ensure their clinical applicability. Finally, we discuss the challenges and limitations of DNA methylation analysis, and the need for DNA methylation profiling to be conducted in different types of maternal diabetes in pregnancy.
Diabetes in pregnancy is associated with an increased risk of short- and long-term adverse outcomes, thus posing a serious health threat to both mother and offspring (1–3). The prevalence of diabetes in pregnancy is rapidly increasing and has been attributed to increasing maternal age and the rising rates of diabetes and obesity (4, 5). According to recent estimates, 21.1 million live births are affected by diabetes, of which a large portion, 80.3%, are due to gestational diabetes mellitus (GDM), a mild form of glucose intolerance that develops during pregnancy, 9.1% are due to type 1 (T1DM) or type 2 (T2DM) diabetes first detected in pregnancy and 10.6% are due to pregestational T1DM and T2DM (6). Diabetes during pregnancy has been associated with maternal (pre-eclampsia, cesarean deliveries, birth injury) and fetal (hyperbilirubinemia and polycythemia, macrosomia, large for gestational age, respiratory distress syndrome, congenital abnormalities, jaundice and perinatal mortality) adverse outcomes (7–9), while in the long-term both mothers and their babies have an increased risk of developing metabolic disease (10–12). Studies have reported that pregestational T1DM and T2DM are associated with more frequent and severe pregnancy complications compared to GDM. The more severe effects of pregestational diabetes on pregnancy are attributed to prolonged exposure to a hyperglycemic environment in the peri-conceptual period, exposure to an in utero hyperglycemic environment early during pregnancy and changes in placental structure and function, and the different pathophysiological mechanisms that underlie the different types of diabetes (13). A better understanding of the mechanisms that link the different types of diabetes in pregnancy with pregnancy complications may facilitate strategies to improve adverse pregnancy outcomes.
Epigenetics is defined as heritable alterations in gene expression that are not caused by changes in the DNA sequence (14). These processes include DNA methylation, histone and chromatin modifications, and non-coding RNAs that act as regulator molecules (15). DNA methylation is the most widely studied and best characterized epigenetic mechanism (16). It involves the covalent attachment of a methyl group to the fifth carbon position of a cytosine nucleotide to form 5-methylcytosine (5-mC). This process is catalyzed by the enzyme DNA methyltransferase (DNMT), with S-adenosyl-methionine serving as the methyl donor (16). DNA methylation mostly occurs on a cytosine base that precedes a guanine nucleotide (CpG site), which tend to cluster together to form CpG islands, and are primarily found within gene promoters, or in repeated elements such as long (LINE) and short (SINE) interspersed elements (17, 18). However, in recent years, studies have provided evidence of the importance of non-CpG and non-promoter methylation in the development of disease (19, 20). DNA methylation modifications regulate the transcriptional potential of the genome by inhibiting transcription factor binding, and is known to affect gene expression pathways associated with a range of pathophysiological processes, such as glucose and lipid homeostasis, insulin signaling and beta-cell function and, when dysregulated, contributes to metabolic disease (21–23).
DNA methylation has been shown to play a key role in regulating genes involved in metabolic adaptation during pregnancy, and aberrant DNA methylation has been demonstrated during pregnancy complications such as pre-eclampsia, hypertension, GDM, early pregnancy loss and preterm birth (24–27). Moreover, altered DNA methylation patterns have been observed in the placenta and cord blood of women with GDM, and have been identified as potential factors that mediate in utero fetal programming (26, 28–34). Thus, altered maternal DNA methylation patterns offer the potential to predict short- and long-term health complications in mothers and offspring exposed to an adverse intrauterine environment, such as hyperglycemia. This review aims to provide a summary of existing studies on DNA methylation in pregestational T1DM and T2DM, and GDM.
The inclusion criteria for this review included all studies reporting on DNA methylation profiling in women with T1DM, T2DM and GDM during pregnancy. Four databases, CINAHL, Scopus, PubMed and Google Scholar were searched to identify published studies that met the inclusion criteria No restrictions on dates were applied, and all articles until May 2022 were included. The following keywords, “pre-gestational diabetes”, OR “type 1 diabetes” OR “type 2 diabetes” OR “gestational diabetes mellitus” OR “maternal diabetes” OR hyperglycemia OR “hyperglycemia in pregnancy” OR “maternal glycemia” AND “DNA methylation” OR methylation OR epigenetics AND pregnancy OR antenatal OR prenatal OR maternal were used. Original articles profiling DNA methylation in women with diabetes in pregnancy and full-text articles published in English were included. The reference lists of included studies were searched to identify eligible articles that may have been missed in the search strategy.
Our literature search identified a total of 1985 research articles, of which 32 met the inclusion criteria and are included in this review (Figure 1). The studies that investigated DNA methylation in pregnant women with diabetes are summarized in Table 1. Of the 32 studies, the majority investigated DNA methylation in women with GDM (n=28), two studies investigated DNA methylation in pregnant women with impaired glucose tolerance (IGT), and two studies investigated DNA methylation in pregnant women with both IGT and GDM groups. GDM is a widely recognized form of IGT that develops during pregnancy (59). Six studies diagnosed IGT or GDM using the World Health Organization (WHO), 1999 criteria (75g 2-hour oral glucose tolerance test (OGTT) ≥ 7.8 mmol/L), and although GDM and IGT may thus refer to the same condition, articles in this review are summarized according to the authors’ reporting, i.e., GDM or IGT. The studies included in this review used different diagnostic criteria, including the International Association of Diabetes in Pregnancy Study Group (IADPSG) (n=12), the German Society of Gynecology and Obstetrics guidelines (n=2), the American Diabetes Association (ADA) 2004 (n=1) and 2010 (n=1), Carpenter and Coustan (n=1), National Diabetes Data Group (n=1), WHO 1999 (n=4) and 2013 (n=1), both WHO 1999 and ADA 2009 (n=1) and WHO 1999 and IADPSG (n=1), a local criteria recommended by the Royal London Hospital, UK (n=1), and six studies did not report which diagnostic criteria were used (n=6). Of these, only four studies provided fasting plasma glucose (FPG), 1-hour and 2-hour OGTT values. Studies were conducted in various countries, and included Chinese (n=8), Canadian (n=8), German (n=3), South African (n=3), American (n=3), Taiwanese (n=2), European (n=2), South Asian (n=1), Japanese (n=1) and a mixed ethnic population (n=1). The sample size varied considerably between studies, ranging from six to 1030 women. DNA methylation was profiled in various biological materials, including maternal peripheral blood, omental visceral (VAT) and subcutaneous (SAT) adipose tissue, placenta (maternal and fetal side) and cord blood. The studies included in this review quantified DNA methylation using various approaches, including global DNA methylation (n=4), genome-wide methylation (n=12) and gene-specific methylation (n=22), which will be discussed in further detail. The included studies were case-control, cross-sectional or longitudinal studies.
Figure 1 Flow diagram showing the selection of studies for inclusion in the review [adapted from (35)].
Global DNA methylation is a measure of the overall genomic methylation and is one of the earliest changes associated with the development of disease (60). Current methods to quantify global DNA methylation include direct methods such as enzyme-linked immunosorbent assays (ELISAs), liquid chromatography coupled with mass spectrometry (LC-MS/MS), high-performance capillary electrophoresis and methylation-sensitive restriction enzymes, and surrogate methods that quantify DNA methylation within repetitive elements as a marker of global DNA methylation (61). The repetitive elements LINE-1 and SINE-1 (mainly Alu) are highly represented throughout the genome and methylation of these elements have been used as a surrogate marker of global genomic DNA methylation. These repetitive elements are quantified using bisulfite pyrosequencing (62).
Four studies quantified global DNA methylation in women with GDM (Table 1). Dias et al. quantified global DNA methylation in the peripheral blood of 201 South African women with or without GDM using the Imprint Global DNA methylation ELISA (43). These authors reported no difference in global DNA methylation in the peripheral blood of women with GDM compared to pregnant women without GDM. Interestingly, this study showed higher levels of global DNA methylation in pregnant women who were obese compared to pregnant women who were not obese. Furthermore, these authors demonstrated that higher levels of global DNA methylation were associated with lower serum adiponectin levels (43), an important adipokine previously shown to be inversely associated with insulin resistance during pregnancy (63, 64). Nomura et al. measured global DNA methylation in the cord blood and placenta of 50 pregnant women with or without GDM from the USA using the Lumino-Metric methylation assay (26). The authors reported no difference in global DNA methylation levels in the cord blood of women with GDM compared to pregnant women without GDM, while lower levels of methylation were observed in the placenta of women with GDM compared to women without GDM (26). Reichetzeder et al. measured global DNA methylation in the placenta of 1030 pregnant women with mixed ethnic ancestry from Germany using LC-MS/MS. These authors reported higher levels of global DNA methylation in the placenta of women with GDM compared to pregnant women without GDM (53). El Hajj et al. assessed methylation of repetitive elements in the placenta and cord blood of 251 pregnant German women using bisulfite pyrosequencing. The authors demonstrated lower LINE-1 and Alu methylation in the cord blood of women with GDM compared to pregnant women without GDM, while an increase in LINE-1 methylation and decrease in Alu methylation was observed in the placenta of women with GDM compared to pregnant women without GDM (30). Taken together, these findings highlight the variability in assessing global DNA methylation levels in different biological sources such peripheral blood, cord blood and placenta, and using different methods of quantification.
Measurement of global DNA methylation is inexpensive and robust, yet does not have the resolution to detect DNA methylation differences within specific genes (65). The quantification of gene-specific methylation at individual CpG sites may elucidate the role of DNA methylation in regulating the expression of genes that orchestrate the development of disease. As such, gene-specific DNA methylation is increasingly being used to identify genes associated with diabetes in pregnancy. Methods to quantify gene-specific DNA methylation include bisulfite pyrosequencing, methylation-specific PCR, methylated DNA immunoprecipitation (MeDIP), direct methylation sequencing and target sequencing combined with base-specific cleavage (61).
Twenty two studies investigated gene-specific DNA methylation in pregnant women with GDM or IGT (Table 1), of which, five studies were gene-specific validation studies for genome-wide DNA methylation quantification, using BeadChip Arrays (36, 40, 46, 56, 57). Of the 22 studies, a total of 62 genes were investigated across the studies. Of these, eight of the genes investigated overlapped in two or more populations and are discussed below. These genes included adiponectin (ADIPOQ), Hypoxia Inducible Factor 3 Subunit Alpha (HIF3α), Interleukin-10 (IL-10), Leptin (LEP), Maternally Expressed 3 (MEG3), Peroxisome Proliferator-activated Receptor Gamma Coactivator 1-alpha (PGC-1α), Peroxisome Proliferator Activated Receptor Alpha (PPARα) and Small Nuclear Ribonucleoprotein Polypeptide N (SNRPN).
The three studies that quantified DNA methylation of ADIPOQ in women with GDM or IGT reported conflicting results (29, 44, 51). Dias et al. quantified DNA methylation at eight CpG sites located upstream of the ADIPOQ transcription start site (TSS) in the peripheral blood of 286 South African women with or without GDM using bisulfite pyrosequencing (44). These authors reported decreased methylation at one CpG site in the distal promoter region located -3400bp upstream of the TSS in women with GDM compared to women without GDM. Interestingly, the authors showed that DNA methylation levels were positively correlated with fasting glucose concentrations and negatively correlated with serum adiponectin concentrations (44). In line with these findings, Bouchard et al. reported lower placental DNA methylation at two CpG islands, located in the proximal promoter and between the first and second exon of ADIPOQ in 100 French-Canadian women with IGT compared to pregnant women with normoglycemia using bisulfite pyrosequencing (29). In contrast to these studies, Ott et al. demonstrated increased methylation levels at majority of the investigated CpG sites in VAT, SAT, maternal blood and cord blood of German women with GDM using bisulfite pyrosequencing (51). In SAT, DNA methylation at one CpG site located upstream of the TSS within the proximal promoter of ADIPOQ was higher in women with GDM compared to women without GDM, while DNA methylation in VAT revealed both higher and lower methylation at one CpG site located between exon 1 and 2 and one in the proximal promoter region of ADIPOQ, respectively. Moreover, in SAT and VAT, gene expression of ADIPOQ was significantly lower in women with GDM compared to women without GDM and was positively correlated with maternal circulating adiponectin levels. CpG sites investigated in SAT and VAT, were similarly hypermethylated in the maternal blood of women with GDM compared to women without GDM, while CpG sites located in the proximal promoter region or between exon 1 and 2 within ADIPOQ were either hypo- and hypermethylation in cord blood (51). ADIPOQ encodes an adipose tissue-derived hormone that plays a key role in whole-body energy homeostasis and is involved in regulating glucose and lipid metabolism and insulin sensitivity (66). Diabetes in pregnancy is characterized by insulin resistance, which increases as pregnancy progresses. Accordingly, lower adiponectin concentrations are observed with pregnancy duration (63, 64) and ADIPOQ levels are decreased in women with GDM compared to pregnant women without GDM (67). Taken together, findings from these studies showed that ADIPOQ methylation levels at specific CpG sites in SAT and VAT are correlated with lower ADIPOQ gene expression and circulating adiponectin levels, supporting the functional relevance in GDM.
Both studies profiling DNA methylation of HIF3α reported increased methylation in the omental tissue and cord blood of women with GDM compared to women without GDM (23, 46). Zhang et al. evaluated the methylation status of HIF3α in the omental tissue of Chinese women using bisulfite pyrosequencing and identified increased methylation at two CpG islands within the HIF3α promoter in women with GDM compared to women without GDM (23). In addition, the authors demonstrated that HIF3α promoter methylation was negatively correlated with HIF3α gene expression. Similarly, using bisulfite pyrosequencing, Haertle et al. reported that the average methylation levels of 11 CpG sites in the HIF3α promoter region were significantly higher in cord blood of European women with insulin and diet treated GDM compared to women without GDM (46). HIF3α is a member of the transcription factor family of hypoxia‐inducible factors, which are known to regulate a wide range of target genes related to glucose and amino acid metabolism, adipocyte differentiation, inflammation and cancer (68, 69). Recent studies have shown that HIF3α methylation is associated with adipose tissue dysfunction and insulin sensitivity, which are key factors contributing to GDM pathogenesis (70, 71). Considering the increased HIF3α promoter methylation in omental tissue and cord blood and its correlation with HIF3α gene regulation, these findings highlight the potential of HIF3α methylation as a candidate biomarker for novel therapeutic targets for GDM treatment.
The two studies quantifying DNA methylation of IL-10 in the cord blood, placenta and peripheral blood of women with GDM reported conflicting results (30, 49). Using bisulfite pyrosequencing, El Hajj et al. quantified DNA methylation of IL-10 in 251 German women with or without GDM. The authors reported an increase in IL-10 promoter methylation in the cord blood of women with GDM compared to women without GDM, while no significant difference were observed in placenta (30). Conversely, using methylation specific PCR, Kang et al. reported decreased IL-10 methylation in the peripheral blood of 32 Taiwanese women who developed GDM compared to women without GDM, while no significant differences were observed in the cord blood and placenta (49). Furthermore, the authors showed that decreased methylation of IL-10 was associated with increased serum IL-10 concentrations at the end of pregnancy. IL-10 is an anti-inflammatory cytokine, which plays an important role in regulating the innate immune system (72), and has been associated with inflammatory-associated diseases such as obesity and diabetes (73). Recently, both increased and decreased IL-10 serum levels have been reported to be associated with diabetes and GDM (74–76). Accordingly, findings from these studies demonstrate tissue and cell type specific IL-10 methylation differences and suggest that lower methylation of IL-10 at specific CpGs may play an important role in the development of GDM but needs to be explored in larger prospective studies to confirm its association.
Two studies investigating DNA methylation of LEP in the cord blood and placenta of French-Canadian and German women with GDM or IGT reported conflicting results (30, 37). Bouchard et al. assessed DNA methylation at 31 CpG sites within the LEP proximal promoter, using targeted sequencing combined with base specific cleavage. The authors reported a decrease in average CpG methylation in the cord blood of French-Canadian women with IGT compared to pregnant women with normoglycemia, while no significant differences were observed in the placenta (37). Interestingly, the authors demonstrated that average placental methylation at the LEP promoter CpG sites was significantly correlated with 2-hour OGTT glucose concentrations in women with IGT compared to pregnant women with normoglycemia. Another study using bisulfite pyrosequencing showed no significant difference in both the placenta and cord blood of German women with GDM (30). However, this study only assessed six of the 31 CpG sites investigated by Bouchard. LEP encodes an adipocytokine that is involved in energy metabolism and insulin sensitivity control, and is expressed and secreted by the placenta during pregnancy (77). Accordingly, several studies have shown that LEP expression and plasma levels are increased in obesity, diabetes and GDM, as well as during pregnancy (78–80). Taken together, these findings provide evidence that LEP DNA methylation may be influenced by glucose dysregulation during pregnancy, although further longitudinal studies are required to confirm whether LEP DNA methylation is a cause or consequence of GDM.
The two studies investigating DNA methylation of MEG3 in women with GDM showed conflicting results, with one study reporting increased methylation in the placenta of Chinese women, and the other reporting no significant change in the placenta and cord blood of German women with GDM (30, 38). Chen et al. assessed 35 CpG sites located within MEG3 in 46 Chinese women with or without GDM, using methylation specific PCR (38). The authors demonstrated increased maternal placental methylation at seven CpG sites within MEG3 in women with GDM compared to women without GDM, which was correlated with maternal hyperglycemia and neonatal birthweight. Furthermore, the authors demonstrated that increased methylation on the maternal side of the placenta was associated with decreased MEG3 gene expression, whereas no MEG3 methylation and gene expression changes were observed on the fetal side of the placenta (38). Using bisulfite pyrosequencing, Hajj et al. showed no difference in placenta and cord blood methylation at three CpG sites located in the MEG3 promoter and five CpG sites located in the MEG3 intergenic region of 251 German women with GDM compared to women without GDM. However, MEG3 methylation differed significantly between male and female cord blood samples regardless of GDM. MEG3 is a maternally expressed imprinting gene, produced by long non-coding RNA transcripts that is regulated by two differentially methylated regions (DMRs) (81, 82). Thus, results from these studies suggest that DNA methylation modifications of the imprinting gene MEG3 could potentially regulate MEG3 gene expression during GDM and may play an essential role in fetal development in response to an intrauterine hyperglycemic environment.
Three studies investigating DNA methylation of PGC-1α reported increased placental methylation in Chinese and French-Canadian women with GDM (34, 39, 55), while one of these studies also reported decreased PGC-1α methylation in cord blood of Chinese in Chinese women with GDM (34). Xie et al. quantified DNA methylation in the PGC-1α promoter region in Chinese women with GDM, using bisulfite pyrosequencing (34). The authors reported increased placental methylation and decreased cord blood methylation in women with GDM compared to women without GDM. Similarly, Côté et al. showed a trend towards increased placental methylation of PGC-1α which was correlated with higher second trimester glucose levels in French-Canadian women with GDM compared to women without GDM, using bisulfite pyrosequencing (39). In addition, these authors showed that increased methylation levels of one CpG site within PGC-1α significantly correlated with cord blood leptin levels in the offspring. Using direct methylation sequencing, Wang et al. reported an increase in DNA methylation of PGC-1α on the fetal side of the placenta in Chinese women with GDM compared women without GDM, which was significantly correlated with decreased PGC-1α gene expression levels (55). PGC-1α is a transcriptional co-activator, which plays an important regulatory role in mitochondrial biogenesis and function, oxidative stress and insulin resistance, implicating PGC-1α in the development of glucose intolerance (83, 84). Accordingly, PGC-1α methylation and mRNA expression has been shown to be altered in diabetic compared to non-diabetic patients (85). Altogether, these results indicate that increased PGC-1α methylation is associated with glucose intolerance during pregnancy and could potentially influence gene regulatory pathways involved in developmental programming and offspring health outcomes.
Two studies investigating DNA methylation of PPARα reported decreased placental methylation in women with GDM (30, 54), while no significant difference was observed in cord blood (30). Rong et al. evaluated the methylation status of PPARα in the placenta of Chinese women and reported decreased methylation levels at 2 CpG sites within the promoter region in women with GDM compared women without GDM, using bisulfite pyrosequencing (54). Moreover, the authors reported that the expression of PPARα was upregulated in the GDM group compared to controls, although, these results were not significant. In a German population, El Hajj et al. reported decreased average placental methylation across 8 CpG sites within the PPARα promoter (30), while no significant difference was observed in the cord blood of women with GDM compared to women without GDM, using bisulfite pyrosequencing. PPARα is a ligand-activated transcriptional factor, known to regulate the expression of genes involved in fatty acid oxidation, and has been shown to be associated with energy metabolism in pregnancy (86), and when dysregulated, may be involved in the molecular mechanisms postulated to underlie GDM. Thus, these findings demonstrate aberrant DNA methylation patterns of PPARα methylation in GDM which may be involved in the pathophysiology of GDM and reflect fetal development. However, future studies are required to assess the therapeutic potential of these findings.
Two studies quantified DNA methylation of SNRPN in women with GDM, and reported conflicting results (30, 36). Binder et al. reported decreased placental methylation of SNRPN in American women with GDM compared to women without GDM, which was validated in a larger sample size, using a BeadChip array and bisulfite pyrosequencing, respectively (36). However, no significant methylation differences were observed when validating these results in an independent cohort, using bisulfite pyrosequencing. Similarly, using bisulfite pyrosequencing, El Hajj et al. reported no significant SNRPN methylation differences in the cord blood and placenta of German women with GDM compared to women without GDM (30). SNRPN is a maternally imprinted gene, which is related to various neurodevelopmental disorders (87), and the lack of SNRPN expression has been linked to hyperphagia, loss of satiety, and obesity (88, 89). Furthermore, imprinted genes such as SNRPN are essential for the regulation of human fetal and placental growth (90), and abnormal methylation of SNRPN has been reported in several imprinting syndromes (91, 92). While studies linking SNRPN to GDM are still lacking, these findings demonstrate a potential role of altered placental methylation of SNRPN during GDM that needs to be explored in future studies.
Other articles in this review reported differential methylation of genes, yet these genes were identified in single studies only (32, 41, 52, 58, 93).
Due to rapid technological advances, genome-wide DNA methylation profiling has emerged as most popular platform for DNA methylation analysis. Genome-wide methylation strategies allow for a comprehensive, high-throughput quantitative approach to assess the methylation status of CpG sites for the entire genome (94). The platform provides an unbiased approach to identify both known and novel methylation sites. The techniques used to assess genome-wide methylation include various Illumina BeadChip Arrays such as the HumanMethylation27, HumanMethylation450 and the HumanMethylationEPIC array, as well as various methylation sequencing platforms such as Sanger or capillary sequencing, next-generation sequencing, whole genome bisulfite sequencing, methylated DNA immunoprecipitation, methylation sensitive restriction enzyme and Methyl-CpG-binding domain protein capture sequencing (95).
In this review, 12 studies quantified genome-wide DNA methylation using different Infinium methylation BeadChip arrays. Of the 12 studies, three studies used the HumanMethylationEPIC BeadChip, while eight studies used the older HumanMethylation450 BeadChip and one study used the HumanMethylation27 BeadChip array. Although these arrays use the same technology, they differ in the range of genomic coverage (27,000 to 850,000 CpG sites across the genome) and may lead to the identification of distinct methylation profiles (94, 96). In one of the earliest genome-wide studies, using the Illumina HumanMethylation27 BeadChip array, which interrogates approximately 27,000 CpG sites across the genome at a single-nucleotide resolution, Enquobahrie et al. reported DNA methylation changes in the peripheral blood of six American women who had two consecutive pregnancies, one of which was complicated by GDM during early pregnancy (45). The authors reported differential methylation at 27 CpG sites, of which 17 CpG sites were hypomethylated, and 10 CpG sites were hypermethylated between GDM and normal pregnancies within the same women. Novel genes related to these CpG sites were found to be associated with the cell cycle, cell morphology, cell assembly, cell organization, and cell compromise (45). These findings suggest that DNA methylation differences in peripheral blood reflect changes in GDM status in women with repeat pregnancies.
Eight of the 12 studies investigated in this review used the Illumina HumanMethylation450 BeadChip Array, which interrogates more than 480,000 methylation sites and covers 96% of CpG islands, as well as additional island shores (94). Ruchat et al. showed that CpG sites corresponding to 3271 genes in the placenta and 3758 genes in the cord blood were differentially methylated in Canadian women with GDM compared to women without GDM. Of these, 1029 differentially methylated genes were common to both tissues (33). In silico analysis revealed that these differentially methylated genes are predominantly involved in metabolic disease pathways, including glucose metabolism-related disorders which were amongst the top ranked pathways in both placenta and cord blood. Finer et al. identified 1418 methylated variable positions in cord blood and 1373 methylated variable positions in the placenta of South Asian women with GDM compared to women without GDM (31). Of these, 378 methylated variable positions were common to cord blood and placenta. Functional analysis of these methylated variable positions in placenta, cord blood and those overlapping both tissue, revealed gene pathways enriched in endocytosis, focal adhesion, chemokine signaling and ligand receptor interactions. Furthermore, these pathways display methylation differences in genes involved in key extracellular triggers to a myriad of intracellular signaling pathways involved in growth and metabolism (31). Haertle et al. reported differential methylation at 1564 CpG sites in the cord blood of middle and Southeastern European women with GDM compared to women without GDM (46). Using more robust statistical analysis, the authors reported the differential methylation of 65 CpG sites that were associated with 52 genes in women with insulin dependent GDM (I-GDM) compared to women without GDM, while no difference was observed in women with dietetically treated GDM (D-GDM) compared to women without GDM. In another study, Deng et al. quantified genome-wide DNA methylation in omental VAT of Chinese women with GDM. The authors reported differential methylation of 5910 regions targeting 1298 genes which were hypomethylated, whereas 6892 differentially methylated regions targeting 1568 genes were hypermethylated in women with GDM compared to women without GDM (40). Functional analysis showed that the differentially methylated genes were association with graft-versus-host disease, T1DM, antigen processing and presentation and allograft rejection biological pathways. Wu et al. identified 100 differentially methylated CpG sites corresponding to 66 genes in the peripheral blood of European women with GDM compared to women without GDM (56). Using a more stringent criteria to prioritize methylation sites, five CpG sites within the constitutive photomorphogenic homolog subunit 8 (COPS8), phosphoinositide 3-kinase regulatory subunit 5 (PIK3R5), 3-hydroxyanthranilate 3,4-dioxygenase (HAAO), coiled-coil domain containing 124 (CCDC124), and chromosome 5 open reading frame 34 (C5orf34), were identified and validated using bisulfite pyrosequencing. Methylation differences of these CpG sites were detected early during pregnancy and may prove useful as predictive biomarkers of GDM. However, this study included 11 women with and without GDM only, and thus, requires further validation in a larger sample size (56). Yan et al. identified 1251 differentially methylated genes in the cord blood of Chinese women with GDM compared to women without GDM (57). The authors demonstrated decreased methylation at two CpG sites in the gene body of Rho Guanine Nucleotide Exchange Factor 11 (ARHGEF11), a key gene associated with metabolic pathways, which was negatively correlated with glucose levels and neonatal birth weight (57). Using a stringent criteria, Binder et al. reported the differential methylation of 648 CpG loci in the placenta of women with GDM compared to women without GDM in an American population. Functional analysis of genes in close proximity to the differentially methylated CpG loci revealed that the most significantly enriched biological processes where associated with cellular metabolism, response to external stimuli, and immune responses (36). More recently, Awamleh et al. showed that 99 CpG sites corresponding to 49 genes in cord blood, and 662 CpG sites corresponding to 338 genes in placenta were differentially methylated in Canadian women with GDM compared to women without GDM (28). Two of these genes, aryl hydrocarbon receptor repressor (AHRR) and protein tyrosine phosphatase receptor type N2 (PTPRN2), were differentially methylated in both cord blood and placental samples. Functional analysis of the differentially methylated genes revealed that the top biological pathways were enriched for antigen processing and presentation via MHC class 1, which plays a vital role in regulating immune responses. Together, these findings indicate that differentially methylated genes, identified in the placenta, cord blood, adipose tissue, and peripheral blood of women with GDM are involved in several metabolic processes that may play an important role in the development of GDM. However, future studies should consider validation or verification of these differentially methylated genes in different populations to confirm their feasibility.
Three of the 12 studies included in this review quantified DNA methylation using the most recent Illumina HumanMethylationEPIC BeadChip array, which interrogates over 850 000 CpG sites across the genome at single-nucleotide resolution (94, 96). Dias et al. investigated DNA methylation in the peripheral blood of South African women with and without GDM, and reported differential methylation of 1046 CpG sites targeting 939 genes in women with GDM compared to women without GDM (42). Of these, 148 CpG sites (14.2%) were hypermethylated, while the 898 CpG sites (85.8%) were hypomethylated in women with GDM compared to women without GDM. Functional enrichment analysis of the differentially methylated genes revealed a significant association with pathways such as cancer, brain signaling, cell growth, proliferation, viability, and inflammatory pathways. Kang et al. showed that 200 CpG sites corresponding to 151 genes in peripheral blood and 167 genes in cord blood were differentially methylated in Taiwanese women with GDM compared to women without GDM (48). Functional analysis of differentially methylated genes demonstrated an association with pathways linked to endocrine disorders, metabolic diseases, carbohydrate metabolism, and lipid metabolism, as well as metabolically related signaling pathways such as Janus kinase 2/signal transduction and activator of transcription 3 (JAK2/STAT-3) and mitogen activated protein kinase (MAPK) in women with GDM compared to women without GDM. Conversely, in a more recent study, Kasuga et al. quantified DNA methylation at 754 255 CpG sites in the cord blood of 230 Japanese women and showed no significant differences between women with GDM compared to women without GDM (50). The identification of differentially methylated genes in peripheral and cord blood provides potential for DNA methylation as a biomarker of GDM. Although further validation using pyrosequencing and conducting longitudinal studies in large sample sizes and in different populations are required to investigate their candidacy as biomarkers of GDM.
The identification of dysregulated DNA methylation patterns may aid in elucidating the pathophysiological mechanisms that link maternal diabetes with pregnancy complications and adverse maternal and infant health outcomes. This review aimed to summarize and synthesize studies that have profiled DNA methylation in pregnancies complicated by T1DM, T2DM and GDM. The 32 studies included in this review investigated GDM or IGT and identified a total of 62 genes associated with these disorders. Eight genes including ADIPOQ, HIF3α, IL-10, LEP, MEG3, PGC-1α, PPARα and SNRPN were differentially methylated in women with GDM or IGT compared to women with normoglycemia in two or more studies. Of these, three genes, HIF3α, PGC1-α and PPARα were similarly differentially methylated in two or more studies. HIF3α and PGC1-α were hypermethylated, while PPARα was hypomethylated in women with GDM compared to pregnant women with normoglyceamia. The consistent methylation profiles of these genes across diverse populations with varying pregnancy durations, and using different diagnostic criteria, methodologies, biological material, support their candidacy as biomarkers of GDM.
Despite our search identifying 32 articles on DNA methylation profiling during maternal diabetes, none of the identified studies profiled DNA methylation in pregnant women with T1DM and T2DM. A study by Alexander et al. profiled DNA methylation in placental tissue of women with GDM (n=14) and pre-existing T2DM (n=3). However, this study correlated DNA methylation with offspring sex and did not compare DNA methylation across diabetes groups, therefore was not included in this review (97).
None of the four studies that measured global DNA methylation reported consistent associations between global DNA methylation and GDM (26, 30, 43, 53). Global DNA methylation profiling provides a robust, relatively easy and cost effective measure of assessing overall genomic DNA methylation (43). However, it may not offer the resolution required to detect CpG specific methylation, which may be more relevant to regulating the expression of genes that orchestrate the development of disease. Thus, studies using methods such as bisulfite pyrosequencing, methylation-specific PCR, methylated DNA immunoprecipitation (MeDIP), direct methylation sequencing and target sequencing combined with base-specific cleavage to assess gene-specific methylation, provided more informative data about the pathogenesis of GDM, and are discussed below.
Of the 62 genes associated with GDM and IGT, eight genes were investigated in two or more studies, of which the increased methylation of HIF3α and PGC1-α and the decreased methylation of PPARα in women with GDM compared to women without GDM were consistent across studies in diverse populations, using different measurement platforms and methodologies, biological material and diagnostic criteria, supporting their involvement in GDM. The results reported in this review showed that CpG islands in the promoter region of HIF3α were more methylated in women with GDM compared to women without GDM in European and Chinese populations (23, 46), and that higher methylation was correlated with decreased HIF3α gene expression (23). Hypoxia-inducible factors (HIFs) are transcription factors that mediate hypoxia in many tissues (98) and HIF3α has been shown to play a role in glucose metabolism, adipocyte differentiation and inflammation, which are important pathways associated with GDM (68, 99). Previous studies have reported that increased HIF3α promoter methylation is associated with obesity and higher plasma glucose levels and waist-hip ratio (71, 100) and adipose tissue dysfunction, T2DM and lower insulin sensitivity (70, 71, 101). Moreover, increased methylation of HIF3α in umbilical cord tissue was associated with higher gestational weight gain, infant birth weight and adiposity (102), suggesting that HIF3α methylation may be a potential biomarker from metabolic syndrome. In contrast, a recent study reported an association between decreased HIF3α methylation and pre-eclampsia (98), a pregnancy complication often leading to placental hypoxia (103). Given the association between GDM, pre-eclampsia and adverse maternal and offspring health outcomes, future studies to determine the functional significance of increased HIF3α methylation during GDM, and its relevance to subsequent health outcomes, are warranted.
Three studies reported increased PGC1-α methylation in the placentae of women with GDM compared to pregnant women with normoglycaemia in Canadian (39) and Chinese populations (34, 55). These results are aligned with previous findings of increased PGC1-α promoter methylation in skeletal muscle biopsies and pancreatic islets of patients with T2DM compared to normoglycemia, which were associated with decreased PGC1-α expression and insulin secretion (104, 105). PGC1-α is a transcriptional regulator of oxidative metabolism and is critical for fatty acid oxidation and mitochondrial biogenesis (106). Dysregulated mitochondrial function in placenta is thought to play a key role in the pathogenesis of GDM and its complications (107). Thus, increased PGC1-α promoter methylation and subsequent decreased PGC1-α expression may lead to mitochondrial dysfunction, oxidative stress, insulin resistance and GDM. Interestingly, increased placental PGC1-α methylation has been linked to low infant birth weight, supporting the relationship between PGC1-α methylation and fetal programming (108).
Two studies reported decreased DNA methylation of PPARα in placentae of women with GDM compared to pregnant women with normoglycaemia in German and Chinese populations (30, 54). In contrast to these findings, a recent study reported a positive association between increased PPARα methylation and metabolic syndrome, higher triglyceride levels and homeostasis model assessment of insulin resistance (HOMA-IR) (109). Similarly, studies conducted in animal models reported that increased PPARα promoter methylation was associated with decreased PPARα expression, insulin resistance and hyperlipidemia (110) and obesity (111) in offspring exposed to unhealthy diets during gestation. These findings allude to the important role of PPARα methylation in metabolic disease. PPARα is a transcription factor that regulates a variety of processes including fatty acid oxidation, inflammation and hepatic glucose production (86, 112). Thus, decreased placental DNA methylation of PPARα may be a compensatory mechanism to increase glucose and lipid metabolism during GDM. Further mechanistic studies are required to elucidate the role of PPARα methylation in the pathophysiology of GDM.
Genome-wide methylation was conducted in 12 out of the 32 studies included in this review. The number of differentially methylated CpG sites ranged between 27 and 6892, targeting several genes. The data filtering criteria used for BeadChip array analysis varied significantly across studies. For example, eight of the 12 studies used the more stringent multiple testing correction methods with adjusted p-values <0.05 for their analysis (28, 31, 36, 40, 45, 46, 48, 50), whereas four studies used the unadjusted p-value of either < 0.01 or < 0.05, since the multiple testing correction methods do not always identify significant differentially methylated loci when sample sizes are small (33, 42, 56, 57). Small sample sizes has been identified as a major limitation of genome-wide studies, which can be improved by using additional datasets and independent DNA methylation quantification methods to verify and validate study findings, although this is time consuming and costly. Indeed, only three of the 12 studies quantifying DNA methylation using BeadChip arrays verified their findings within the same study and confirmed their findings for the relevant genes of interest (36, 40, 57). Other technical differences which may influence the number of differentially methylated CpG sites identified across studies include sample preparation, loading during hybridization and batch effect bias (96, 113). These differences make it difficult to compare DNA methylation profiles across studies and has been identified as a major bottleneck for the identification of robust DNA methylation associations.
The variation in DNA methylation profiles across the studies included in this review, highlight key challenges that must be addressed before DNA methylation profiling can achieve clinical applicability. DNA methylation heterogeneity is attributed to differences in methodology, measurement platforms, normalization strategies, biological source, diagnostic criteria, and timing of methylation analysis (114, 115), thus, emphasizing the need to implement common practices and standardization of experimental approaches to facilitate reproducibility and data harmonization across studies. Studies included in this review utilized various biological material, such as placental tissue, adipose tissue, cord blood and peripheral blood, which consist of a heterogenous mixture of different cell types each possessing a unique DNA methylation signature that may have contributed to a disunited DNA methylation signal observed across studies (114).Of the 32 studies, only three studies (30, 42, 46) adjusted for cell type composition, while no studies reported utilizing the cell-sorting method to measure DNA methylation. Future DNA methylation studies should consider purification of blood cell populations to separate specific cell types, or using bioinformatic methods to adjust for cell type proportions (116). Other factors widely reported to affect DNA methylation heterogeneity include population differences such as age, ethnicity, socioeconomic status, environmental and lifestyle factors such as diet and physical activity, diabetes medication regimes and human immunodeficiency virus (HIV) infections (117–122), that could explain some of the inconsistencies in the findings reported in this review. In addition, pre-analytical factors during sample collection and transport, and analytical factors during DNA isolation and profiling procedures may also contribute the differences in DNA methylation profiling observed in these studies. Furthermore, recent evidence has suggested that DNA methylation may also be influenced by distal genetic sequence variants (123) and in this regard, the use of methylation quantitative trait loci may open exciting opportunities to better understand the complex relationship between genetics, environmental cues, and their impact on the epigenome.
While a plethora of studies investigated DNA methylation alterations in pregnancies complicated by GDM, our review highlights the lack of studies profiling DNA methylation in pregnancies with pregestational T1DM and T2DM. We propose that future studies should prioritize profiling DNA methylation in pregnancies complicated by the different types of maternal diabetes, to provide insight into their underlying molecular mechanisms, which may be related to pregnancy health outcomes. Furthermore, this review confirms the growing evidence supporting the potential of DNA methylation to serve as biomarkers of GDM. Two genes, HIF3α and PGC1-α, showing increased methylation and one gene, PPARα, showing decreased methylation in women with GDM compared to pregnant women with normoglycemia were consistently methylated across diverse populations with varying pregnancy durations and using different diagnostic criteria, methodologies and biological material. These three differentially methylated genes represent candidate biomarkers for GDM and may influence several GDM-related metabolic processes such as adipocyte differentiation, inflammation, mitochondrial function, oxidative stress, and glucose and energy metabolism (Figure 2). Furthermore, these genes may provide insight into the pathways that are epigenetically influenced during diabetes in pregnancy and should be prioritized and replicated in longitudinal studies and in larger populations to ensure their clinical applicability. Profiling DNA methylation may provide an opportunity to facilitate intervention strategies and risk assessment models to identify women at risk of GDM and thus delay or prevent its development and consequent adverse outcomes.
Figure 2 The effects of gestational diabetes on maternal DNA methylation (Image created with Biorender.com).
SD and CP conceptualized the study; SD prepared the original draft; SD, TW, SA, and CP reviewed and edited the manuscript. All authors read and approved the final draft.
This work was funded by the National Research Foundation (NRF), Thuthuka Grant No: 129844 to SD and Grant No: 129897 to TW, and the NRF Competitive Programme for Rated Researchers Grant No: 120832 to CP. Baseline funding from the South African Medical Research Council (SAMRC) is also acknowledged.
The authors declare that the research was conducted in the absence of any commercial or financial relationships that could be construed as a potential conflict of interest.
All claims expressed in this article are solely those of the authors and do not necessarily represent those of their affiliated organizations, or those of the publisher, the editors and the reviewers. Any product that may be evaluated in this article, or claim that may be made by its manufacturer, is not guaranteed or endorsed by the publisher.
The content hereof is the sole responsibility of the authors and do not represent the official views of the NRF or SAMRC.
1. Burlina S, Dalfrà MG, Lapolla A. Short- and long-term consequences for offspring exposed to maternal diabetes: A review. J. Matern Fetal Neonatal Med. (2019) 32(4):687–94. doi: 10.1080/14767058.2017.1387893
2. Johns EC, Denison FC, Norman JE, Reynolds RM. Gestational diabetes mellitus: Mechanisms, treatment, and complications. Trends Endocrinol. Metab. (2018) 29(11):743–54. doi: 10.1016/j.tem.2018.09.004
3. Sugrue R, Zera C. Pregestational diabetes in pregnancy. Obstet Gynecol Clin. North Am. (2018) 45(2):315–31. doi: 10.1016/j.ogc.2018.01.002
4. Ferrara A. Increasing prevalence of gestational diabetes mellitus: A public health perspective. Diabetes Care (2007) 30 Suppl 2:S141–146. doi: 10.2337/dc07-s206
5. Lawrence JM, Contreras R, Chen W, Sacks DA. Trends in the prevalence of preexisting diabetes and gestational diabetes mellitus among a racially/ethnically diverse population of pregnant women, 1999-2005. Diabetes Care (2008) 31(5):899–904. doi: 10.2337/dc07-2345
6. International Diabetes Federation. IDF Diabetes Atlas, 10th edn. Brussels, Belgium (2021). Available at: https://www.diabetesatlas.org.
7. Muche AA, Olayemi OO, Gete YK. Effects of gestational diabetes mellitus on risk of adverse maternal outcomes: A prospective cohort study in Northwest Ethiopia. BMC Pregnancy Childbirth (2020) 20(1):73. doi: 10.1186/s12884-020-2759-8
8. Negrato CA, Mattar R, Gomes MB. Adverse pregnancy outcomes in women with diabetes. Diabetol. Metab. Syndrome (2012) 4(1):41. doi: 10.1186/1758-5996-4-41
9. Shand AW, Bell JC, McElduff A, Morris J, Roberts CL. Outcomes of pregnancies in women with pre-gestational diabetes mellitus and gestational diabetes mellitus; a population-based study in new south Wales, Australia, 1998-2002. Diabetes Med. (2008) 25(6):708–15. doi: 10.1111/j.1464-5491.2008.02431.x
10. Burlina S, Dalfrà M, Lapolla A. Short-and long-term consequences for offspring exposed to maternal diabetes: a review. J. Maternal-Fetal Neonatal Med. (2019) 32(4):687–94. doi: 10.1080/14767058.2017.1387893
11. McCance DR. Diabetes in pregnancy. Best Pract. Research: Clin. Obstetrics Gynaecol (2015) 29(5):685–99. doi: 10.1016/j.bpobgyn.2015.04.009
12. Mitanchez D, Yzydorczyk C, Siddeek B, Boubred F, Benahmed M, Simeoni U. The offspring of the diabetic mother – short- and long-term implications. Best Pract. Res. Clin. Obstetrics Gynaecol (2015) 29(2):256–69. doi: 10.1016/j.bpobgyn.2014.08.004
13. Fong A, Serra A, Herrero T, Pan D, Ogunyemi D. Pre-gestational versus gestational diabetes: a population based study on clinical and demographic differences. J. Diabetes Complications (2014) 28(1):29–34. doi: 10.1016/j.jdiacomp.2013.08.009
14. Gibney ER, Nolan CM. Epigenetics and gene expression. Heredity. (2010) 105(1):4–13. doi: 10.1038/hdy.2010.54
15. Godfrey KM, Costello P, Lillycrop K. Development, epigenetics and metabolic programming. Nestle Nutr. Inst Workshop Ser. (2016) 85:71–80. doi: 10.1159/000439488
16. Bird AP. CpG-rich islands and the function of DNA methylation. Nature. (1986) 321(6067):209–13. doi: 10.1038/321209a0
17. Kile ML, Baccarelli A, Tarantini L, Hoffman E, Wright RO, Christiani DC. Correlation of global and gene-specific DNA methylation in maternal-infant pairs. PloS One (2010) 5(10):e13730. doi: 10.1371/journal.pone.0013730
18. Webster ALH, Yan MSC, Marsden PA. Epigenetics and cardiovascular disease. Can. J. Cardiol. (2013) 29(1):46–57. doi: 10.1016/j.cjca.2012.10.023
19. Jang HS, Shin WJ, Lee JE, Do JT. CpG and non-CpG methylation in epigenetic gene regulation and brain function. Genes (Basel) (2017) 8(6):E148. doi: 10.3390/genes8060148
20. Pheiffer C, Erasmus RT, Kengne AP, Matsha TE. Differential DNA methylation of microRNAs within promoters, intergenic and intragenic regions of type 2 diabetic, pre-diabetic and non-diabetic individuals. Clin. Biochem. (2016) 49(6):433–8. doi: 10.1016/j.clinbiochem.2015.11.021
21. Fradin D, Fur SL, Mille C, Naoui N, Groves C, Zelenika D, et al. Association of the CpG methylation pattern of the proximal insulin gene promoter with type 1 diabetes. PLoS One (2012) 7(5):e36278. doi: 10.1371/journal.pone.0036278
22. Gilbert ER, Liu D. Epigenetics: The missing link to understanding β-cell dysfunction in the pathogenesis of type 2 diabetes. Epigenetics. (2012) 7(8):841–52. doi: 10.4161/epi.21238
23. Zhang Y, Chen Y, Qu H, Wang Y. Methylation of HIF3α promoter CpG islands contributes to insulin resistance in gestational diabetes mellitus. Mol. Genet. Genomic Med. (2019) 7(4):e00583. doi: 10.1002/mgg3.583
24. Del Vecchio G, Li Q, Li W, Thamotharan S, Tosevska A, Morselli M, et al. Cell-free DNA methylation and transcriptomic signature prediction of pregnancies with adverse outcomes. Epigenetics. (2021) 16(6):642–61. doi: 10.1080/15592294.2020.1816774
25. Kazmi N, Sharp GC, Reese SE, Vehmeijer FO, Lahti J, Page CM, et al. Hypertensive disorders of pregnancy and DNA methylation in newborns. Hypertension. (2019) 74(2):375–83. doi: 10.1161/HYPERTENSIONAHA.119.12634
26. Nomura Y, Lambertini L, Rialdi A, Lee M, Mystal EY, Grabie M, et al. Global methylation in the placenta and umbilical cord blood from pregnancies with maternal gestational diabetes, preeclampsia, and obesity. Reprod. Sci. (2014) 21(1):131–7. doi: 10.1177/1933719113492206
27. Zhou Q, Xiong Y, Qu B, Bao A, Zhang Y. DNA Methylation and recurrent pregnancy loss: A mysterious compass? Front. Immunol. (2021) 12:738962. doi: 10.3389/fimmu.2021.738962
28. Awamleh Z, Butcher DT, Hanley A, Retnakaran R, Haertle L, Haaf T, et al. Exposure to gestational diabetes mellitus (GDM) alters DNA methylation in placenta and fetal cord blood. Diabetes Res. Clin. Practice (2021) 174:108690. doi: 10.1016/j.diabres.2021.108690
29. Bouchard L, Hivert MF, Guay SP, St-Pierre J, Perron P, Brisson D. Placental adiponectin gene DNA methylation levels are associated with mothers’ blood glucose concentration. Diabetes. (2012) 61(5):1272–80. doi: 10.2337/db11-1160
30. El Hajj N, Pliushch G, Schneider E, Dittrich M, Müller T, Korenkov M, et al. Metabolic programming of MEST DNA methylation by intrauterine exposure to gestational diabetes mellitus. Diabetes. (2013) 62(4):1320–8. doi: 10.2337/db12-0289
31. Finer S, Mathews C, Lowe R, Smart M, Hillman S, Foo L, et al. Maternal gestational diabetes is associated with genome-wide DNA methylation variation in placenta and cord blood of exposed offspring. Hum. Mol. Genet. (2015) 24(11):3021–9. doi: 10.1093/hmg/ddv013
32. Houde AA, Guay SP, Desgagné V, Hivert MF, Baillargeon JP, St-Pierre J, et al. Adaptations of placental and cord blood ABCA1 DNA methylation profile to maternal metabolic status. Epigenetics. (2013) 8(12):1289–302. doi: 10.4161/epi.26554
33. Ruchat SM, Houde AA, Voisin G, St-Pierre J, Perron P, Baillargeon JP, et al. Gestational diabetes mellitus epigenetically affects genes predominantly involved in metabolic diseases. Epigenetics. (2013) 8(9):935–43. doi: 10.4161/epi.25578
34. Xie X, Gao H, Zeng W, Chen S, Feng L, Deng D, et al. Placental DNA methylation of peroxisome-proliferator-activated receptor-γ co-activator-1α promoter is associated with maternal gestational glucose level. Clin. Science (2015) 129(4):385–94. doi: 10.1042/CS20140688
35. Page MJ, McKenzie JE, Bossuyt PM, Boutron I, Hoffmann TC, Mulrow CD, et al. The PRISMA 2020 statement: an updated guideline for reporting systematic reviews. BMJ (2021) 372(11):n71. doi: 10.1136/bmj.n71
36. Binder AM, LaRocca J, Lesseur C, Marsit CJ, Michels KB. Epigenome-wide and transcriptome-wide analyses reveal gestational diabetes is associated with alterations in the human leukocyte antigen complex. Clin. Epigenet (2015) 7(1):79. doi: 10.1186/s13148-015-0116-y
37. Bouchard L, Thibault S, Guay SP, Santure M, Monpetit A, St-Pierre J, et al. Leptin gene epigenetic adaptation to impaired glucose metabolism during pregnancy. Diabetes Care (2010) 33(11):2436–41. doi: 10.2337/dc10-1024
38. Chen C, Jiang Y, Yan T, Chen Y, Yang M, Lv M, et al. Placental maternally expressed gene 3 differentially methylated region methylation profile is associated with maternal glucose concentration and newborn birthweight. J. Diabetes Investig. (2021) 12(6):1074–82. doi: 10.1111/jdi.13432
39. Côté S, Gagné-Ouellet V, Guay SP, Allard C, Houde AA, Perron P, et al. PPARGC1α gene DNA methylation variations in human placenta mediate the link between maternal hyperglycemia and leptin levels in newborns. Clin. epigenetics (2016) 8:72. doi: 10.1186/s13148-016-0239-9
40. Deng X, Yang Y, Sun H, Qi W, Duan Y, Qian Y. Analysis of whole genome-wide methylation and gene expression profiles in visceral omental adipose tissue of pregnancies with gestational diabetes mellitus. J. Chin. Med. Assoc. (2018) 81(7):623–30. doi: 10.1016/j.jcma.2017.06.027
41. Desgagné V, Hivert MF, St-Pierre J, Guay SP, Baillargeon JP, Perron P, et al. Epigenetic dysregulation of the IGF system in placenta of newborns exposed to maternal impaired glucose tolerance. Epigenomics. (2014) 6(2):193–207. doi: 10.2217/epi.14.3
42. Dias S, Adam S, Rheeder P, Louw J, Pheiffer C. Altered genome-wide DNA methylation in peripheral blood of south African women with gestational diabetes mellitus. Int. J. Mol. Sci. (2019) 20(23):5828. doi: 10.3390/ijms20235828
43. Dias S, Adam S, Van Wyk N, Rheeder P, Louw J, Pheiffer C. Global DNA methylation profiling in peripheral blood cells of south African women with gestational diabetes mellitus. Biomarkers. (2019) 24(3):225–31. doi: 10.1080/1354750X.2018.1539770
44. Dias S, Adam S, Abrahams Y, Rheeder P, Pheiffer C. Adiponectin DNA methylation in south African women with gestational diabetes mellitus: Effects of HIV infection. PloS One (2021) 16(3):e0248694. doi: 10.1371/journal.pone.0248694
45. Enquobahrie DA, Moore A, Muhie S, Tadesse MG, Lin S, Williams MA. Early pregnancy maternal blood DNA methylation in repeat pregnancies and change in gestational diabetes mellitus status-a pilot study. Reprod. Sci. (2015) 22(7):904–10. doi: 10.1177/1933719115570903
46. Haertle L, El Hajj N, Dittrich M, Müller T, Nanda I, Lehnen H, et al. Epigenetic signatures of gestational diabetes mellitus on cord blood methylation. Clin. Epigenetics (2017) 9:28. doi: 10.1186/s13148-017-0329-3
47. Houde AA, St-Pierre J, Hivert MF, Baillargeon JP, Perron P, Gaudet D, et al. Placental lipoprotein lipase DNA methylation levels are associated with gestational diabetes mellitus and maternal and cord blood lipid profiles. J Developmental Origins Health Dis (2014) 5(2):132–41. doi: 10.1017/S2040174414000038
48. Kang J, Lee CN, Li HY, Hsu KH, Lin SY. Genome-wide DNA methylation variation in maternal and cord blood of gestational diabetes population. Diabetes Res. Clin. Pract. (2017) 132:127–36. doi: 10.1016/j.diabres.2017.07.034
49. Kang J, Lee CN, Li HY, Hsu KH, Wang SH, Lin SY. Association of interleukin-10 methylation levels with gestational diabetes in a Taiwanese population. Front. IN Genet. (2018) 9(JUN):222. doi: 10.3389/fgene.2018.00222
50. Kasuga Y, Kawai T, Miyakoshi K, Hori A, Tamagawa M, Hasegawa K, et al. DNA Methylation analysis of cord blood samples in neonates born to gestational diabetes mothers diagnosed before 24 gestational weeks. BMJ Open Diabetes Res. Care (2022) 10(1):e002539. doi: 10.1136/bmjdrc-2021-002539
51. Ott R, Stupin JH, Melchior K, Schellong K, Ziska T, Dudenhausen JW, et al. Alterations of adiponectin gene expression and DNA methylation in adipose tissues and blood cells are associated with gestational diabetes and neonatal outcome. Clin. Epigenetics (2018) 10(1):131. doi: 10.1186/s13148-018-0567-z
52. Rancourt RC, Ott R, Schellong K, Ziska T, Melchior K, Henrich W, et al. Altered SOCS3 DNA methylation within exon 2 is associated with increased mRNA expression in visceral adipose tissue in gestational diabetes. Epigenetics. (2021) 16(5):488–94. doi: 10.1080/15592294.2020.1805695
53. Reichetzeder C, Dwi Putra SE, Pfab T, Slowinski T, Neuber C, Kleuser B, et al. Increased global placental DNA methylation levels are associated with gestational diabetes. Clin. Epigenet. (2016) 8(1). doi: 10.1186/s13148-016-0247-9
54. Rong C, Cui X, Chen J, Qian Y, Jia R, Hu Y. DNA Methylation profiles in placenta and its association with gestational diabetes mellitus. Exp. Clin. Endocrinol. Diab (2015) 123(5):282–8. doi: 10.1055/s-0034-1398666
55. Wang L, Fan H, Zhou L, Wu Y, Lu H, Luo J. Altered expression of PGC-1alpha and PDX1 and their methylation status are associated with fetal glucose metabolism in gestational diabetes mellitus. Biochem. Biophys. Res. Commun. (2018) 501(1):300–6. doi: 10.1016/j.bbrc.2018.05.010
56. Wu P, Farrell WE, Haworth KE, Emes RD, Kitchen MO, Glossop JR, et al. Maternal genome-wide DNA methylation profiling in gestational diabetes shows distinctive disease-associated changes relative to matched healthy pregnancies. Epigenetics. (2018) 13(2):122–8. doi: 10.1080/15592294.2016.1166321
57. Yan J, Su R, Zhang W, Wei Y, Wang C, Lin L, et al. Epigenetic alteration of rho guanine nucleotide exchange factor 11 (ARHGEF11) in cord blood samples in macrosomia exposed to intrauterine hyperglycemia. J. Matern Fetal Neonatal Med. (2021) 34(3):422–31. doi: 10.1080/14767058.2019.1609929
58. Zhao BH, Jiang Y, Zhu H, Xi FF, Chen Y, Xu YT, et al. Placental delta-like 1 gene DNA methylation levels are related to mothers’ blood glucose concentration. J. Diabetes Res. (2019) 2019:e9521510. doi: 10.1155/2019/9521510
59. Metzger BE, Buchanan TA, Coustan DR, de Leiva A, DB D, DR H, et al. Summary and recommendations of the fifth international workshop-conference on gestational diabetes mellitus. Diabetes Care (2007) 30(Supplement 2):S251–60. doi: 10.2337/dc07-s225
60. Gama-Sosa MA, Slagel VA, Trewyn RW, Oxenhandler R, Kuo KC, Gehrke CW, et al. The 5-methylcytosine content of DNA from human tumors. Nucleic Acids Res. (1983) 11(19):6883–94. doi: 10.1093/nar/11.19.6883
61. Kurdyukov S, Bullock M. DNA Methylation analysis: choosing the right method. Biol. (Basel) (2016) 5(1):3. doi: 10.3390/biology5010003
62. Vryer R, Saffery R. What’s in a name? Context-dependent significance of ‘global’ methylation measures in human health and disease. Clin. Epigenetics (2017) 9(1):2. doi: 10.1186/s13148-017-0311-0
63. Bao W, Baecker A, Song Y, Kiely M, Liu S, Zhang C. Adipokine levels during the first or early second trimester of pregnancy and subsequent risk of gestational diabetes mellitus: a systematic review. Metabolism. (2015) 64(6):756–64. doi: 10.1016/j.metabol.2015.01.013
64. Catalano PM, Hoegh M, Minium J, Huston-Presley L, Bernard S, Kalhan S, et al. Adiponectin in human pregnancy: implications for regulation of glucose and lipid metabolism. Diabetologia. (2006) 49(7):1677–85. doi: 10.1007/s00125-006-0264-x
65. Dahl C, Guldberg P. DNA Methylation analysis techniques. Biogerontology. (2003) 4):233–50. doi: 10.1023/A:1025103319328
66. Brochu-Gaudreau K, Rehfeldt C, Blouin R, Bordignon V, Murphy BD, Palin MF. Adiponectin action from head to toe. Endocrine. (2010) 37(1):11–32. doi: 10.1007/s12020-009-9278-8
67. Guelfi KJ, Ong MJ, Li S, Wallman KE, Doherty DA, Fournier PA, et al. Maternal circulating adipokine profile and insulin resistance in women at high risk of developing gestational diabetes mellitus. Metab. Clin. Exp. (2017) 75:54–60. doi: 10.1016/j.metabol.2017.08.003
68. Hatanaka M, Shimba S, Sakaue M, Kondo Y, Kagechika H, Kokame K, et al. Hypoxia-inducible factor-3alpha functions as an accelerator of 3T3-L1 adipose differentiation. Biol. Pharm. Bull. (2009) 32(7):1166–72. doi: 10.1248/bpb.32.1166
69. Makino Y, Cao R, Svensson K, Bertilsson G, Asman M, Tanaka H, et al. Inhibitory PAS domain protein is a negative regulator of hypoxia-inducible gene expression. Nature. (2001) 414(6863):550–4. doi: 10.1038/35107085
70. Drevytska T, Gavenauskas B, Drozdovska S, Nosar V, Dosenko V, Mankovska I. HIF-3α mRNA expression changes in different tissues and their role in adaptation to intermittent hypoxia and physical exercise. Pathophysiology. (2012) 19(3):205–14. doi: 10.1016/j.pathophys.2012.06.002
71. Pfeiffer S, Krüger J, Maierhofer A, Böttcher Y, Klöting N, El Hajj N, et al. Hypoxia-inducible factor 3A gene expression and methylation in adipose tissue is related to adipose tissue dysfunction. Sci. Rep. (2016) 6(1):27969. doi: 10.1038/srep27969
72. Brauner H, Lüthje P, Grünler J, Ekberg NR, Dallner G, Brismar K, et al. Markers of innate immune activity in patients with type 1 and type 2 diabetes mellitus and the effect of the anti-oxidant coenzyme Q10 on inflammatory activity. Clin. Exp. Immunol. (2014) 177(2):478–82. doi: 10.1111/cei.12316
73. Canecki-Varžić S, Prpić-Križevac I, Mihaljević S, Bilić-Ćurčić I, Alkhamis T, Wagner J, et al. Association between interleukin-10 gene (-1082g/A) polymorphism and type 2 diabetes, diabetes-related traits, and microvascular complications in the Croatian population. Acta Clin. Croat (2018) 57(1):71–81. doi: 10.20471/acc.2018.57.01.08
74. Al-Shukaili A, Al-Ghafri S, Al-Marhoobi S, Al-Abri S, Al-Lawati J, Al-Maskari M. Analysis of inflammatory mediators in type 2 diabetes patients. Int. J. Endocrinol. (2013) 2013:976810. doi: 10.1155/2016/7965317
75. Atègbo JM, Grissa O, Yessoufou A, Hichami A, Dramane KL, Moutairou K, et al. Modulation of adipokines and cytokines in gestational diabetes and macrosomia. J. Clin. Endocrinol. Metab. (2006) 91(10):4137–43. doi: 10.1210/jc.2006-0980
76. van Exel E, Gussekloo J, de Craen AJM, Frölich M, Bootsma-Van Der Wiel A, Westendorp RGJ, et al. Low production capacity of interleukin-10 associates with the metabolic syndrome and type 2 diabetes: The Leiden 85-plus study. Diabetes. (2002) 51(4):1088–92. doi: 10.2337/diabetes.51.4.1088
77. Zavalza-Gómez AB, Anaya-Prado R, Rincón-Sánchez AR, Mora-Martínez JM. Adipokines and insulin resistance during pregnancy. Diabetes Res. Clin. Practice (2008) 80(1):8–15. doi: 10.1016/j.diabres.2007.12.012
78. Henson MC, Castracane VD. Leptin in pregnancy. Biol. Reproduction (2000) 63(5):1219–28. doi: 10.1095/biolreprod63.5.1219
79. Nesrine Z, Haithem H, Imen B, Fadoua N, Asma O, Fadhel NM, et al. Leptin and leptin receptor polymorphisms, plasma leptin levels and obesity in Tunisian volunteers. Int. J. Exp. Pathol. (2018) 99(3):121–30. doi: 10.1111/iep.12271
80. Yang M, Peng S, Li W, Wan Z, Fan L, Du Y. Relationships between plasma leptin levels, leptin G2548A, leptin receptor Gln223Arg polymorphisms and gestational diabetes mellitus in Chinese population. Sci. Rep. (2016) 6:23948. doi: 10.1038/srep23948
81. Miyoshi N, Wagatsuma H, Wakana S, Shiroishi T, Nomura M, Aisaka K, et al. Identification of an imprinted gene, Meg3/Gtl2 and its human homologue MEG3, first mapped on mouse distal chromosome 12 and human chromosome 14q. Genes Cells (2000) 5(3):211–20. doi: 10.1046/j.1365-2443.2000.00320.x
82. Zhang H. Mechanism associated with aberrant lncRNA MEG3 expression in gestational diabetes mellitus. Exp. Ther. Med. (2019) 18(5):3699–706. doi: 10.3892/etm.2019.8062
83. Handschin C, Spiegelman BM. Peroxisome proliferator-activated receptor gamma coactivator 1 coactivators, energy homeostasis, and metabolism. Endocr. Rev. (2006) 27(7):728–35. doi: 10.1210/er.2006-0037
84. Newsholme P, Haber EP, Hirabara SM, Rebelato ELO, Procopio J, Morgan D, et al. Diabetes associated cell stress and dysfunction: Role of mitochondrial and non-mitochondrial ROS production and activity. J. Physiol. (2007) 583(Pt 1):9–24. doi: 10.1113/jphysiol.2007.135871
85. Mensink M, Hesselink MKC, Russell AP, Schaart G, Sels JP, Schrauwen P. Improved skeletal muscle oxidative enzyme activity and restoration of PGC-1α and PPARβ/δ gene expression upon rosiglitazone treatment in obese patients with type 2 diabetes mellitus. Int. J. Obes. (2007) 31(8):1302–10. doi: 10.1038/sj.ijo.0803567
86. van Raalte DH, Li M, Pritchard PH, Wasan KM. Peroxisome proliferator-activated receptor (PPAR)-alpha: a pharmacological target with a promising future. Pharm. Res. (2004) 21(9):1531–8. doi: 10.1023/B:PHAM.0000041444.06122.8d
87. Nicholls RD, Knepper JL. Genome organization, function, and imprinting in prader-willi and angelman syndromes. Annu. Rev. Genomics Hum. Genet. (2001) 2:153–75. doi: 10.1146/annurev.genom.2.1.153
88. Bischof JM, Stewart CL, Wevrick R. Inactivation of the mouse Magel2 gene results in growth abnormalities similar to prader-willi syndrome. Hum. Mol. Genet. (2007) 16(22):2713–9. doi: 10.1093/hmg/ddm225
89. Horvath S, Erhart W, Brosch M, Ammerpohl O, von Schönfels W, Ahrens M, et al. Obesity accelerates epigenetic aging of human liver. Proc. Natl. Acad. Sci. U S A. (2014) 111(43):15538–43. doi: 10.1073/pnas.1412759111
90. Kelsey G. Genomic imprinting–roles and regulation in development. Endocr. Dev. (2007) 12:99–112. doi: 10.1159/000109637
91. Kubota T, Sutcliffe JS, Aradhya S, Gillessen-Kaesbach G, Christian SL, Horsthemke B, et al. Validation studies of SNRPN methylation as a diagnostic test for prader-willi syndrome. Am. J. Med. Genet. (1996) 66(1):77–80. doi: 10.1002/(SICI)1096-8628(19961202)66:1<77::AID-AJMG18>3.0.CO;2-N
92. van Montfoort APA, Hanssen LLP, de Sutter P, Viville S, Geraedts JPM, de Boer P. Assisted reproduction treatment and epigenetic inheritance. Hum. Reprod. Update (2012) 18(2):171–97. doi: 10.1093/humupd/dmr047
93. Houde AA, Légaré C, Biron S, Lescelleur O, Biertho L, Marceau S, et al. Leptin and adiponectin DNA methylation levels in adipose tissues and blood cells are associated with BMI, waist girth and LDL-cholesterol levels in severely obese men and women. BMC Med. Genet. (2015) 16:29. doi: 10.1186/s12881-015-0174-1
94. Bibikova M, Le J, Barnes B, Saedinia-Melnyk S, Zhou L, Shen R, et al. Genome-wide DNA methylation profiling using infinium® assay. Future Med. Ltd London UK (2009) 1(1):177 –200. doi: 10.2217/epi.09.14
95. Li S, Tollefsbol TO. DNA Methylation methods: Global DNA methylation and methylomic analyses. Methods. (2021) 187:28–43. doi: 10.1016/j.ymeth.2020.10.002
96. Nakabayashi K. Illumina HumanMethylation BeadChip for genome-wide DNA methylation profiling: advantages and limitations. In: Patel V, Preedy V, editors. Handbook of nutrition, diet, and epigenetics. Berlin, Germany: Springer International Publishing (2017). p. 1–15.
97. Alexander J, Teague AM, Chen J, Aston CE, Leung YK, Chernausek S, et al. Offspring sex impacts DNA methylation and gene expression in placentae from women with diabetes during pregnancy. PLoS One (2018) 13(2):e0190698. doi: 10.1371/journal.pone.0190698
98. Mansell T, Ponsonby AL, Januar V, Novakovic B, Collier F, Burgner D, et al. Early-life determinants of hypoxia-inducible factor 3A gene (HIF3α) methylation: A birth cohort study. Clin. Epigenetics (2019) 11:96. doi: 10.1186/s13148-019-0687-0
99. Heidbreder M, Qadri F, Jöhren O, Dendorfer A, Depping R, Fröhlich F, et al. Non-hypoxic induction of HIF-3α by 2-deoxy-D-glucose and insulin. Biochem. Biophys. Res. Commun. (2007) 352(2):437–43. doi: 10.1016/j.bbrc.2006.11.027
100. Dick KJ, Nelson CP, Tsaprouni L, Sandling JK, Aïssi D, Wahl S, et al. DNA Methylation and body-mass index: A genome-wide analysis. Lancet (2014) 383(9933):1990–8. doi: 10.1016/S0140-6736(13)62674-4
101. Main AM, Gillberg L, Jacobsen AL, Nilsson E, Gjesing AP, Hansen T, et al. DNA Methylation and gene expression of HIF3α: cross-tissue validation and associations with BMI and insulin resistance. Clin. Epigenet (2016) 8(1):89. doi: 10.1186/s13148-016-0258-6
102. Pan H, Lin X, Wu Y, Chen L, Teh AL, Soh SE, et al. HIF3α association with adiposity: The story begins before birth. Future Med. Ltd London UK (2015) 7(6):937–950. doi: 10.2217/epi.15.45
103. Zhou J, Xiao D, Hu Y, Wang Z, Paradis A, Mata-Greenwood E, et al. Gestational hypoxia induces preeclampsia-like symptoms via heightened endothelin-1 signaling in pregnant rats. Hypertension (2013) 62(3):599–607. doi: 10.1161/hypertensionaha.113.01449
104. Barres R, Zierath JR. DNA Methylation in metabolic disorders. Am. J. Clin. Nutr. (2011) 93(4):897S–900S. doi: 10.3945/ajcn.110.001933
105. Ling C, Del Guerra S, Lupi R, Rönn T, Granhall C, Luthman H, et al. Epigenetic regulation of PPARGC1A in human type 2 diabetic islets and effect on insulin secretion. Diabetologia. (2008) 51(4):615–22. doi: 10.1007/s00125-007-0916-5
106. Neidhart M. Chapter 11 - DNA methylation in metabolic diseases. In: Neidhart M, editor. DNA Methylation and complex human disease. Oxford: Academic Press (2016). p. 201–14. Available at: https://www.sciencedirect.com/science/article/pii/B9780124201941000117.
107. Ramírez-Emiliano J, Fajardo-Araujo ME, Zúñiga-Trujillo I, Pérez-Vázquez V, Sandoval-Salazar C, Órnelas-Vázquez JK. Mitochondrial content, oxidative, and nitrosative stress in human full-term placentas with gestational diabetes mellitus. Reprod. Biol. Endocrinol (2017) 15(1):26. doi: 10.1186/s12958-017-0244-7
108. Brøns C, Jacobsen S, Nilsson E, Rönn T, Jensen CB, Storgaard H, et al. Deoxyribonucleic acid methylation and gene expression of PPARGC1A in human muscle is influenced by high-fat overfeeding in a birth-weight-dependent manner. J. Clin. Endocrinol. Metab. (2010) 95(6):3048–56. doi: 10.1210/jc.2009-2413
109. Castellano-Castillo D, Moreno-Indias I, Sanchez-Alcoholado L, Ramos-Molina B, Alcaide-Torres J, Morcillo S, et al. Altered adipose tissue DNA methylation status in metabolic syndrome: Relationships between global DNA methylation and specific methylation at adipogenic, lipid metabolism and inflammatory candidate genes and metabolic variables. J. Clin. Med. (2019) 8(1):87. doi: 10.3390/jcm8010087
110. Ando Y, Yamada H, Munetsuna E, Yamazaki M, Kageyama I, Teshigawara A, et al. Maternal high-fructose corn syrup consumption causes insulin resistance and hyperlipidemia in offspring via DNA methylation of the pparα promoter region. J. Nutr. Biochem. (2022) 103:108951. doi: 10.1016/j.jnutbio.2022.108951
111. Pang H, Ling D, Cheng Y, Akbar R, Jin L, Ren J, et al. Gestational high-fat diet impaired demethylation of pparα and induced obesity of offspring. J. Cell. Mol. Med. (2021) 25(12):5404–16. doi: 10.1111/jcmm.16551
112. Contreras AV, Rangel-Escareño C, Torres N, Alemán-Escondrillas G, Ortiz V, Noriega LG, et al. PPARα via HNF4α regulates the expression of genes encoding hepatic amino acid catabolizing enzymes to maintain metabolic homeostasis. Genes Nutr. (2015) 10(2):1–16. doi: 10.1007/s12263-014-0452-0
113. Soriano-Tárraga C, Jiménez-Conde J, Giralt-Steinhauer E, Ois Á, Rodríguez-Campello A, Cuadrado-Godia E, et al. DNA Isolation method is a source of global DNA methylation variability measured with LUMA. Experimental analysis and a systematic review. PLoS One (2013) 8(4):e60750. doi: 10.1371/journal.pone.0060750
114. Rahmani E, Schweiger R, Rhead B, Criswell LA, Barcellos LF, Eskin E, et al. Cell-type-specific resolution epigenetics without the need for cell sorting or single-cell biology. Nat. Commun. (2019) 10(1):3417. doi: 10.1038/s41467-019-11052-9
115. Lisanti S, Omar WAW, Tomaszewski B, Prins SD, Jacobs G, Koppen G, et al. Comparison of methods for quantification of global DNA methylation in human cells and tissues. PLoS One (2013) 8(11):e79044. doi: 10.1371/journal.pone.0079044
116. Reinius LE, Acevedo N, Joerink M, Pershagen G, Dahlén SE, Greco D, et al. Differential DNA methylation in purified human blood cells: Implications for cell lineage and studies on disease susceptibility. PLoS One (2012) 7(7):e41361. doi: 10.1371/journal.pone.0041361
117. García-Calzón S, Perfilyev A, Männistö V, de Mello VD, Nilsson E, Pihlajamäki J, et al. Diabetes medication associates with DNA methylation of metformin transporter genes in the human liver. Clin. Epigenet. (2017) 9(1)1–9. doi: 10.1186/s13148-017-0400-0
118. Joubert BR, Håberg SE, Nilsen RM, Wang X, Vollset SE, Murphy SK, et al. 450K epigenome-wide scan identifies differential DNA methylation in newborns related to maternal smoking during pregnancy. Environ. Health Perspect. (2012) 120(10):1425–31. doi: 10.1289/ehp.1205412
119. Lim U, Song MA. Dietary and lifestyle factors of DNA methylation. Methods Mol. Biol. (2012) 863:359–76. doi: 10.1007/978-1-61779-612-8_23
120. Miyake K, Kawaguchi A, Miura R, Kobayashi S, Tran NQV, Kobayashi S, et al. Association between DNA methylation in cord blood and maternal smoking: The Hokkaido study on environment and children’s health. Sci. Rep. (2018) 8(1):5654. doi: 10.1038/s41598-018-23772-x
121. Pauwels S, Ghosh M, Duca RC, Bekaert B, Freson K, Huybrechts I, et al. Dietary and supplemental maternal methyl-group donor intake and cord blood DNA methylation. Epigenetics. (2017) 12(1):1–10. doi: 10.1080/15592294.2016.1257450
122. Pheiffer C, Dias S, Adam S. Intimate partner violence: A risk factor for gestational diabetes. Int. J. Environ. Res. Public Health (2020) 17(21):7843. doi: 10.3390/ijerph17217843
Keywords: DNA methylation, diabetes, pregnancy, gestational diabetes mellitus, type 1 diabetes mellitus, type 2 diabetes mellitus
Citation: Dias S, Willmer T, Adam S and Pheiffer C (2022) The role of maternal DNA methylation in pregnancies complicated by gestational diabetes. Front. Clin. Diabetes Healthc. 3:982665. doi: 10.3389/fcdhc.2022.982665
Received: 30 June 2022; Accepted: 22 August 2022;
Published: 21 September 2022.
Edited by:
Elisabet Børsheim, University of Arkansas for Medical Sciences, United StatesReviewed by:
Ana Laura De La Garza, Autonomous University of Nuevo León, MexicoCopyright © 2022 Dias, Willmer, Adam and Pheiffer. This is an open-access article distributed under the terms of the Creative Commons Attribution License (CC BY). The use, distribution or reproduction in other forums is permitted, provided the original author(s) and the copyright owner(s) are credited and that the original publication in this journal is cited, in accordance with accepted academic practice. No use, distribution or reproduction is permitted which does not comply with these terms.
*Correspondence: Stephanie Dias, U3RlcGhhbmllLkRpYXNAbXJjLmFjLnph
Disclaimer: All claims expressed in this article are solely those of the authors and do not necessarily represent those of their affiliated organizations, or those of the publisher, the editors and the reviewers. Any product that may be evaluated in this article or claim that may be made by its manufacturer is not guaranteed or endorsed by the publisher.
Research integrity at Frontiers
Learn more about the work of our research integrity team to safeguard the quality of each article we publish.