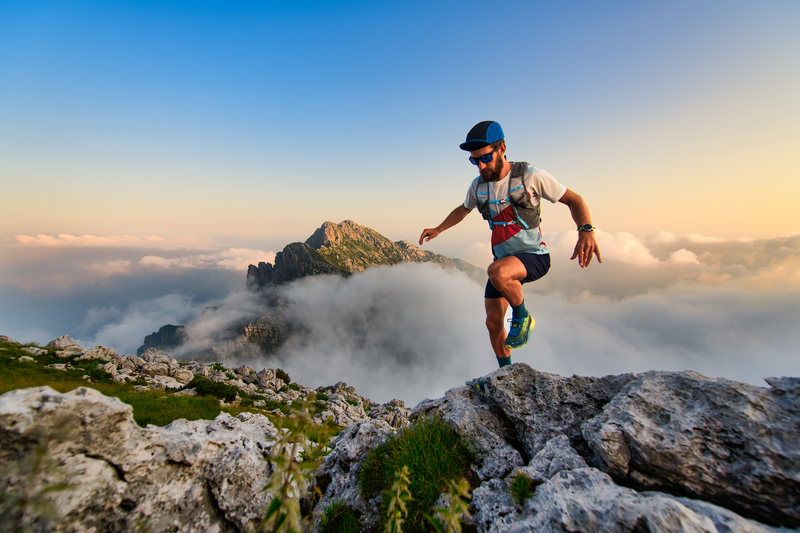
95% of researchers rate our articles as excellent or good
Learn more about the work of our research integrity team to safeguard the quality of each article we publish.
Find out more
ORIGINAL RESEARCH article
Front. Clin. Diabetes Healthc. , 09 September 2022
Sec. Diabetes Self-Management
Volume 3 - 2022 | https://doi.org/10.3389/fcdhc.2022.977216
This article is part of the Research Topic Epidemiology of Diabetes in Africa View all 4 articles
Background: Evaluating the medicine burden from the patients’ perspective is essential for getting good health outcomes of diabetes mellitus (DM) management. However, data are limited regarding this sensitive area. Thus, the study was aimed to determine the medication-related burden (MRB) and associated factors among DM patients at Felege Hiwot Comprehensive Specialized Hospital (FHCSH) in northwest Ethiopia.
Methods: A cross-sectional study was conducted on 423 systematically selected DM patients attending the DM clinic of FHCSH from June to August 2020. The medication-related burden was measured by using the Living with Medicines Questionnaire version 3 (LMQ-3). Multiple linear regression was used to identify factors associated with medication-related burden and reported with 95% confidence interval (CI). p-value <0.05 was considered as statistically significant to declare an association.
Results: The mean LMQ-3 score was 126.52 ( ± 17.39). The majority of the participants experienced moderate (58.9%, 95% CI: 53.9–63.7) to high (26.2%, 95% CI: 22.5–30.0) degrees of medication burden. Nearly half (44.9%, 95% CI: 39.9–49.7) of the participants were non-adherent to their prescribed medications. VAS score (B = 12.773, p = 0.001), ARMS score (B = 8.505, p = 0.001), and fasting blood glucose (FBS) on visit (B = 5.858, p = 0.003) were significantly associated with high medication-related burden.
Conclusion: A significant number of patients suffered from high medication-related burden and non-adherence to long-term medicine. Therefore, multidimensional intervention to decrease MRB and to upgrade adherence is required to increase patients’ quality of life.
Diabetes mellitus (DM) is a global public health problem that causes significant morbidity and mortality (1, 2). Furthermore, DM and its complications are a reason for an increased risk of hospitalization, length of hospital stay, healthcare cost, and reduced health-related quality of life (2–6). In 2019, the estimated global prevalence of DM was 9.3% (463 million people), which is expected to rise to 10.9% (700 million) by 2045. In Ethiopia, according to the International Diabetes Federation 2019 report, the prevalence of DM was 4.3% (7). A few other studies in Ethiopia also reported that the prevalence of DM ranges from 1.9% to 12.2% (3, 8–12). Based on the literature search, studies regarding the prevalence of DM in the study area were not conducted. However, a single study on medication non-adherence and associated factors among diabetic patients reported that in northwest Ethiopia specifically at Felege Hiwot Comprehensive Specialized Hospital (FHCSH) approximately 2,484 DM patients were registered for follow-up and receiving diabetic care (13).
Medication-related burden (MRB) is a recent concept concerned with the negative experiences resulting from the process of undertaking treatment. It is one aspect of treatment burden, and it includes not only the burden of the medication but also all types of healthcare intervention and patient’s perspective toward MRB (14–16). Medication-related burden can lead to non-adherence and poor clinical outcomes, as well as affecting patient satisfaction, psychological well-being, social functioning, and quality of life. It can lead to poor quality of life as patients spend more of their time, energy, and resources on staying well because they experience burden not only from their illness but also from their ever-expanding healthcare regimens. Burdened patients may struggle with adhering to prescribed medications and treatment care, but the burden may influence adherence to treatment and patients’ health. Patients with multimorbidity and an excessive burden of treatment may not adhere to the prescribed medication (17–19).
Previously conducted studies described that MRB is affected by a variety of sociodemographic, clinical, and treatment-related factors. The significant predictors of high MRB include being male, older age, unemployed, polypharmacy, number of chronic conditions, the severity of the chronic condition, visual analog scale (VAS)-burden scores, needing support, high dosing frequency, paying prescription charge, Adherence to Refills and Medication Scale (ARMS) score, duration of DM, marital status, and presence of hypertension (16, 20, 21). A study done in Qatar reported that variables such as ARMS score, duration of DM, marital status, employment status, and presence of hypertension were significantly associated with MRB (16).
Patient-reported experience measures and patient-reported outcome measures have a pivotal role in helping patients know how they feel about their own experiences and outcomes of care, including the benefits and risks of treatment (22–24).
Previous studies explained that a high burden of treatment is a reason for increased hospitalization, cognitive impairment, drug interactions, physical side effects, increased healthcare resource utilization, and higher mortality rate. Furthermore, these studies suggest that exploring new interventions to reduce the burden of treatment, ultimately moving toward minimally disruptive medicine, is necessary (22, 23, 25–30). To help patients move toward minimally disruptive medicine, the identification and targeting of risk factors for high medication-related burden is the starting point. Evidence showed that DM is one of the most common chronic illnesses associated with micro- and macrovascular complications that might result in poor clinical outcomes and increased morbidity as well as mortality. Additionally, as DM is associated with a number of comorbidities such as hypertension and dyslipidemia, DM patients might be burdened by medicines (1–4). Despite the growing burden and economic impact of treatment burden in developing countries including Ethiopia, few studies have been conducted globally and most of them were carried out in the developed world. Based on the literature search, studies regarding this sensitive issue are lacking in Ethiopia including studies conducted in this specific country. Therefore, the study was aimed to determine the MRB and associated factors among DM patients at FHCSH in northwest Ethiopia.
A cross-sectional study was conducted at FHCSH from June to August 2020, which is found in Bahir Dar City, northwest of Ethiopia. Bahir Dar is the capital city of Amhara regional state which is 564 km away from Addis Ababa. The hospital is expected to serve more than seven million people in its catchment area. It has 400 beds and 15 adult outpatient departments, one of which serves as a referral and follow-up clinic for patients with chronic diseases. The DM clinic is situated inside the outpatient department, and a large number of patients attend the follow-up clinic. Based on a previous study, around 2,484 DM patients were registered for follow-up in the clinic (31).
All adult DM patients attending the outpatient clinic of FHCSH were taken as the source population, whereas adult DM patients attending the outpatient clinic of FHCSH during the study period were considered as the study population. Adults (≥18 years) with a diagnosis of DM for at least 3 months prior to the study, with or without comorbidities, were included. However, DM patients with mental disabilities, any speech impairment, and incomplete charts were excluded.
A single population proportion formula [n = (Z α/2)2 p (1 − p)/d2] was used to calculate the sample size (32). With 95% confidence level, proportion of medication-related burden 50% because there is no previous study in Ethiopia and relative precision 5%, the total sample size was 384. The sample size was corrected for non-response rate (15%) (33), and the final calculated sample size was 442. A systematic random sampling technique was utilized to select the study participants.
The dependent variable was MRB which was measured using Living with Medicines Questionnaire version 3 (LMQ-3), while the independent variables were patients’ sociodemographic variables (age, gender), type of DM, duration of DM, presence of comorbidities, number of comorbidities, adherence to the prescribed medication, number of prescribed medication, and fasting blood glucose (FBS) level.
Data were collected by structured questionnaires via face-to-face interviews. The questionnaire has four parts. The first section contains the participants’ sociodemographic characteristics, the second part contains items related to diseases and medication, the third part contains items related to self-reported MRB, and the fourth section contains items related to medication adherence. The LMQ-3 is a 41-item questionnaire where respondents are required to indicate their level of agreement using a five-point Likert-type scale. This tool is comprised of eight domains. The LMQ-3 tool was validated in English (34) and adapted and validated in Arabic (35). The overall LMQ score was the sum of the scores of all the 41 items in the questionnaire, with scores ranging from 41 to 205 corresponding to the following: extremely high burden, 173–205; high burden, 140–172; moderate burden, 107–139; minimal burden, 74–106; and no burden at all, 41–73. The questionnaire also contained a 10-cm line VAS scale, through which respondents provided a global assessment of the overall burden they experienced (0 “no burden at all” to 10 “extreme burden at all”), with higher scores representing greater perceived burden (16).
A 12-item ARMS rating from 1 (none of the time) to 4 (all of the time) scale ranging from 12 to 48 scores was used to measure adherence. Those patients who scored ≤13 were categorized as adherent and those who scored >13 were non-adherent (20). This tool was validated in Arabic (16) and the internal consistency was correlated to the Morisky adherence (36). Other patient-related information was then obtained from medical records using the data collection form that was specifically designed for this study.
The questionnaire was translated into Amharic language and back-translated to English to check if the translated items retained the same meaning as the original items. Training was given to the data collectors by the investigator and supervisors. A pretest was done by taking 10% of the sample size to assess the understandability, internal consistency, and validity of the questionnaire. The overall LMQ-3 tool had a good internal reliability with a Cronbach’s alpha of 0.90. Each of the eight domains showed good internal consistency with Cronbach’s alpha ranging from 0.72 to 0.98. The internal consistency (Cronbach’s α coefficient) of the overall ARMS score was 0.828.
Data were checked, coded, and cleaned for inconsistencies and missing values and entered into EpiData version 4.6.0.0 (EpiData Association, Odense, Denmark) statistical software and then exported to SPSS version 21 (IBM Corporation, Armonk, NY, USA) for analysis. Descriptive, correlation, comparative, and regression analyses were conducted. Continuous variables were expressed as mean ( ± SD) when normally distributed or median (IQR) when not normally distributed. Additionally, categorical variables were summarized as frequency (percentage) of the total. The normality of data was assessed by the Shapiro–Wilk test. A Spearman’s and Pearson correlation test were used to assess the relationship between the independent variables and dependent variable. Independent t-tests or one-way analysis of variance (ANOVA) was done to examine the MRB differences among the independent variables. Since the dependent variable MRB was a continuous variable that fulfilled the normality distribution and linearity assumptions, multiple linear regression was used to identify factors associated with MRB. Multicollinearity was assessed using Pearson correlation coefficients. In the linear regression, most of the independent variables were continuous, while a few variables were dummy categorical variables. p-value <0.05 was considered as statistically significant to declare association. In the study, most of the independent variables were continuous (age, duration of DM, VAS score, ARMS score, FBS, number of comorbidities), while a few variables were dummy categorical variables which were categorized as yes/no (using tablet, using injection).
A total of 442 participants were approached and 423 of them agreed to participate in the study giving a response rate of 95.7%. Of the participants, more than half of them (234, 55.3%) were men. The mean age of the study participants was 40.04 ( ± 15.67) years. Of the study participants, 322 (76.1%) were urban dwellers and 145 (34.3%) were unable to read and write (Table 1).
Table 1 Sociodemographic characteristics of DM patients attending at Felege Hiwot Comprehensive Specialized Hospital.
Among the 423 study participants, 220 (52.0%) had type 1 DM. The median (IQR) duration of DM diagnosis was 4.0 (2.0) years. Comorbidities were present in 89 (21.0%) of the study participants, and the most commonly reported comorbidity was hypertension 65 (15.4%). Most of the participants (365, 86.3%) were prescribed with one or two medications. The median (IQR) FBS value was 198 (105) mg/dl, and 345 (81.6%) of the participants had uncontrolled DM (FBS ≥ 126 mg/dl) (Table 2).
Table 2 Clinical and medication information of DM patients attending at Felege Hiwot Comprehensive Specialized Hospital.
The mean ( ± SD) LMQ-3 score was 126.52 (17.39). The results showed that the majority (85.1%) of DM patients suffered from a moderate (58.9%, 95% CI: 53.9–63.7) to a high (26.2%, 95% CI: 22.5–30.0) degree of burden. The mean ( ± SD) VAS score was 3.83 ( ± 1.07) and the majority of the participants (60%, 95% CI: 55–65.6) were perceived to have a high global burden (Table 3).
Table 3 Descriptive summary of perceived medication-related burden by LMQ-3 and VAS score in DM patients attending at Felege Hiwot Comprehensive Specialized Hospital.
Medication-related burden showed a moderately significant positive association with VAS score (r = 0.561, p < 0.001) and ARMS score (r = 0.518, p < 0.001). Additionally, place of residence (r = 0.109, p = 0.026), duration of DM diagnosis (r = 0.151, p = 0.002), number of comorbidities (r = 0.157, p = 0.001), and number of medications (r = 0.207, p < 0.001) had a weak positive association with MRB.
Diabetic patients with FBS ≥126 mg/dl on visit showed high MRB mean score than those with FBS ≤125 mg/dl (129.19 ± 15.9 vs. 114.68 ± 18.82, p < 0.001). Patients with a high VAS score ≥4 (high global burden) showed high MRB (134.04 ± 12.49 vs. 115.21 ± 17.64, p < 0.001) than those with a VAS score ≤3 (low burden). Additionally, patients with ARMS score ≥14 (non-adherent) showed high MRB (35.16 ± 1.86 vs. 119.47 ± 18.02, p < 0.001) than those with ARMS score ≤13 (adherent).
Simple and multiple linear regression analyses were done to identify factors associated with MRB (LMQ-3) score. Simple linear regression indicated that age, having college education, being urban, practicing a healthy diet and exercise, duration of DM, absence of comorbidities, number of comorbidities, using two or more medicines, not taking both tablet and injection, FBS level, and ARMS and VAS scores were associated with MRB. However, the multiple linear regression analysis indicated that only VAS score (B = 12.773, p = 0.001), ARMS score (B = 8.505, p = 0.001), and FBS on visit (B = 5.858, p = 0.003) were significantly associated with MRB. For every unit increase in the VAS score (global burden), ARMS score, and FBS on visit, there were 12.773, 8.505, and 5.858 increases in the MRB, respectively. The model analysis showed that the independent variables explained 37% of the variability of the dependent variable MRB (R2 = 0.37), and the regression model was a good fit for the data (F16, 404 = 14.88, p < 0.001) (Table 4).
Table 4 Multiple linear regression LMQ-3 score versus sociodemographics and medicine-use characteristics in DM patients attending at Felege Hiwot Comprehensive Specialized Hospital.
The adherence to prescribed medication was measured by using the ARMS score. The median (IQR) ARMS score was 13 (3), range 12–48. The result showed that 44.9% (95% CI: 39.9–49.7) of the study participants were non-adherent to their prescribed medications and 55.1% (95% CI: 50.3–60.1) were adherent to their prescribed medications (Figure 1).
Figure 1 Adherence to prescribed medication among DM patients attending at Felege Hiwot Comprehensive Specialized Hospital.
The current findings revealed that the majority of DM patients suffered from a moderate to a high degree of burden. According to the multiple linear regression, high VAS score (perceived global burden), ARMS score, and FBS on visit were significantly associated with high MRB. In the present study, almost half of the DM patients were non-adherent to their prescribed medications.
The results of the present study showed that the majority (85.1%) of DM patients suffered from moderate (58.9%, 95% CI: 53.9–63.7) to high MRB (26.2%, 95% CI: 22.5–30.0), which is similar to the findings of a study in Qatar [minimum (66.8%) to moderate (24.1%)] (16). However, these values were higher compared to the results of the studies conducted in England [minimum (33.1%) to moderate (53.6%)] (21), New Zealand [moderate (45.1) to high (30.5)] (37), and Kuwait [minimum (35.4%) to moderate (62.0%)] (20). The possible explanation for the difference could be variations in sociodemographic characteristics, presence and number of comorbidities, number of prescribed medications, duration (years) of DM diagnosis, unavailability of better medical care, poor relationship with the healthcare providers, and inadequate knowledge of patients about their medication in developing nations which might cause higher treatment burden to the current study (15, 38).
The current findings showed that the mean LMQ-3 overall score among patients ≥65 years was higher than those aged <65 years old. This finding was in line with similar studies which reported that overall LMQ scores were related to age (16, 20, 39). A possible reason might be that age was a risk factor for comorbidities, as the number of comorbidities increases the risk of polypharmacy and the complexity of medication regimens and the passive behavior of the elderly in taking their medications also increases (40–42). In contrast, a study done in England revealed a significantly lower mean LMQ score among the elderly (≥65 years) than adult patients (18–64 years) (21). The possible explanation for the difference might be variations in educational status between developed and resource-limited settings. Participants who lived in developed nations might have a better attitude and general knowledge about medicine, know about the possible side effects of medicine, and have good communication with health professionals about medicine and the perceived effectiveness of medicine which might lower MRB in elderly participants (43–45).
The MRB was positively associated with uncontrolled DM, which is similar to the study done in Qatar (16). If blood glucose increases and is not managed properly, the risk of getting acute as well as chronic complications increases, and this might cause acute life-threatening events such as diabetic ketoacidosis and increase the frequency of hospitalization and decrease the patients’ quality of life (5, 46).
In this study, MRB had a statistically significant association with non-adherence to medication. This result agrees with the studies done in Qatar (16) and Kuwait (20). A possible explanation might be that non-adherence causes a lower serum concentration of medication which affects the effectiveness and efficacy of the medication, and this causes treatment failure which might lead to uncontrolled glycemic level. Finally, it may lead to disease progression associated with acute and chronic complications, enhancing exposure to polypharmacy (19, 47). Polypharmacy obligates patients to take multiple medications at once and apply many instructions, and it also exposes the patients to possible side effects and adverse drug reactions. In addition, non-adherence leads to a poor quality of life and frequent hospitalization, and this also leads to a high MRB (48).
In the current study, 60% of the patients had a high global perceived burden. The current findings showed a moderate positive relationship with overall LMQ-3 scores (Pearson r = 0.561) and a weak positive correlation between VAS score and ARMS score (r = 0.286). This implies that the higher the perceived global burden, the lower the medication adherence level.
The study had its limitations. The English version of the LMQ-3 tool was developed and used in a country with a different health system, which has not been used in Ethiopia before which may potentially limit the findings. The study does not include critically ill patients who do not regularly attend the follow-up clinic, and this might limit the representativeness of the findings.
The vast majority of DM patients suffered from moderate to high degree of burden and non-adherence to long-term medicine. Adherence level, perceived global burden, and FBS were the predictors of MRB in diabetic patients. Therefore, to minimize MRB, multidimensional intervention is required for DM patients with uncontrolled DM, those non-adherent to their prescribed medications, and patients with a high perceived global burden. In addition, the findings of this study would provide data for clinicians on which factors they should target in reducing the medication-related burden of their diabetic patients.
The raw data supporting the conclusions of this article will be made available by the authors, without undue reservation.
This study was reviewed and approved by the School of Pharmacy Ethical Review Committee, College of Medicine and Health Sciences, University of Gondar. To ensure confidentiality of data, study subjects were identified using codes, and only authorized persons accessed the collected data. The patients/participants provided their written informed consent to participate in this study.
AB and MB were involved in the conceptualization and design of the study and data acquisition, analysis, and interpretation and took part in drafting the initial version of the manuscript. MT and MY were responsible for the data acquisition, analysis, and interpretation and for revising and editing the initial manuscript. All authors contributed to the article and approved the submitted version.
The authors declare that the research was conducted in the absence of any commercial or financial relationships that could be construed as a potential conflict of interest.
All claims expressed in this article are solely those of the authors and do not necessarily represent those of their affiliated organizations, or those of the publisher, the editors and the reviewers. Any product that may be evaluated in this article, or claim that may be made by its manufacturer, is not guaranteed or endorsed by the publisher.
ARMS, Adherence to Refills and Medications Scale; DM, diabetes mellitus; FHCSH, Felege Hiwot Comprehensive Specialized Hospital; IQR, interquartile range; MRD, medication-related burden; MRB, perceived medication-related burden; SD, standard deviation; TBQ, Treatment Burden Questionnaire; VAS, visual analog scale; WHO, World Health Organization.
1. Foryoung JB, Ditah C, Fon PN, Mboue-Djieka Y, Nebongo DN, Mbango ND, et al. Long-term mortality in outpatients with type 2 diabetes in a reference hospital in Cameroon: a retrospective cohort study. BMJ Open (2018) 8(2):e019086. doi: 10.1136/bmjopen-2017-019086
2. Han H, Sun Y, Zhang Y, Tacke F, Zhao H, Deng H, et al. Prevalence and in-hospital outcomes of diabetes mellitus in elderly patients with liver cirrhosis. AME Med J (2017) 2(8):1–10. doi: 10.21037/amj.2017.07.14
3. Bishu KG, Jenkins C, Yebyo HG, Atsbha M, Wubayehu T, Gebregziabher M. Diabetes in Ethiopia: a systematic review of prevalence, risk factors, complications, and cost. Obes Med (2019) 15:100132. doi: 10.1016/J.OBMED.2019.100132
4. Bommer C, Sagalova V, Heesemann E, Manne-Goehler J, Atun R, Bärnighausen T, et al. Global economic burden of diabetes in adults: projections from 2015 to 2030. Diabetes Care (2018) 41(5):963–70. doi: 10.2337/dc17-1962
5. Kim S. Burden of hospitalizations primarily due to uncontrolled diabetes: implications of inadequate primary health care in the united states. Diabetes Care (2007) 30(5):1281–2. doi: 10.2337/dc06-2070
6. Zurita-Cruz JN, Manuel-Apolinar L, Arellano-Flores ML, Gutierrez-Gonzalez A, Najera-Ahumada AG, Cisneros-González N. Health and quality of life outcomes impairment of quality of life in type 2 diabetes mellitus: a cross-sectional study. Health Qual Life Outcomes (2018) 16(1):94. doi: 10.1186/s12955-018-0906-y
7. Saeedi P, Petersohn I, Salpea P, Malanda B, Karuranga S, Unwin N, et al. Global and regional diabetes prevalence estimates for 2019 and projections for 2030 and 2045: Results from the international diabetes federation diabetes atlas, 9(th) edition. Diabetes Res Clin Pract (2019) 157:107843. doi: 10.1016/j.diabres.2019.107843
8. Endris T, Worede A, Asmelash D. Prevalence of diabetes mellitus, prediabetes and its associated factors in dessie town, northeast Ethiopia: a community-based study. Diabetes Metab Syndrome Obesity: Targets Ther (2019) 12:2799. doi: 10.2147/DMSO.S225854
9. Zekewos A, Loha E, Egeno T, Wubshet K, Merga Z. Prevalence of diabetes mellitus and associated factors in southern Ethiopia: A community based study. Ethiopian J Health Sci (2018) 28(4):451–60. doi: 10.4314/ejhs.v28i4.11
10. Abebe N, Kebede T, Addise D. Diabetes in Ethiopia 2000–2016 prevalence and related acute and chronic complications; a systematic review. Afr J Diabetes Med (2017) 25(2):7–12.
11. Aynalem SB, Zeleke AJ. Prevalence of diabetes mellitus and its risk factors among individuals aged 15 years and above in mizan-aman town, southwest Ethiopia, 2016: A cross sectional study. Int J Endocrinol (2018) 2018:9317987. doi: 10.1155/2018/9317987
12. Kassa A, Woldesemayat EM. Hypertension and diabetes mellitus among patients at hawassa university comprehensive specialized hospital, hawassa, southern Ethiopia. Int J Chronic Diseases (2019) 2019:2509242. doi: 10.1155/2019/2509242
13. Abate T. Medication non-adherence and associated factors among diabetes patients in felege hiwot referral hospital, bahir dar city administration, Northwest Ethiopia. BMC Res Notes (2019) 12(1):175. doi: 10.1186/s13104-019-4205-4
14. Alsadah A, van Merode T, Alshammari R, Kleijnen J. A systematic literature review looking for the definition of treatment burden. Heliyon (2020) 6(4):e03641. doi: 10.1016/j.heliyon.2020.e03641
15. Sheehan OC, Leff B, Ritchie CS, Garrigues SK, Li L, Saliba D, et al. A systematic literature review of the assessment of treatment burden experienced by patients and their caregivers. BMC Geriatrics (2019) 19(1):262. doi: 10.1186/s12877-019-1222-z
16. Zidan A, Awaisu A, El-Hajj MS, Al-Abdulla SA, Figueroa DCR, Kheir N. Medication-related burden among patients with chronic disease conditions: perspectives of patients attending non-communicable disease clinics in a primary healthcare setting in Qatar. Pharmacy (2018) 6(3):85. doi: 10.3390/pharmacy6030085
17. Sabaté E, Sabaté E. Adherence to long-term therapies: evidence for action: World health organization. (2003). Geneva Switzerland: WHO Library Cataloguing-in-Publication Data, 71–85.
18. Mir SA, Muzamil F, Bhat M, Amin A, Shakeel D. Assessment of medication adherence among patients with chronic diseases: a descriptive cross-sectional study. Int J Basic Clin Pharmacol (2018) 8(1):115. doi: 10.18203/2319-2003.ijbcp20185168
19. Lemay J, Waheedi M, Al-Sharqawi S, Bayoud T. Medication adherence in chronic illness: do beliefs about medications play a role? Patient preference Adherence (2018) 12:1687. doi: 10.2147/PPA.S169236
20. Awad A, Alhadab A, Albassam A. Medication-related burden and medication adherence among geriatric patients in Kuwait: a cross-sectional study. Front Pharmacol (2020) 11:1296. doi: 10.3389/fphar.2020.01296
21. Krska J, Katusiime B, Corlett SA. Patient experiences of the burden of using medicines for long-term conditions and factors affecting burden: A cross-sectional survey. Health Soc Care Commun (2018) 26(6):946–59. doi: 10.1111/hsc.12624
22. Katusiime B, Corlett S, Reeve J, Krska J. Measuring medicine-related experiences from the patient perspective: a systematic review. Patient Related Outcome Measures (2016) 7:157. doi: 10.2147/PROM.S102198
23. Sav A, King MA, Whitty JA, Kendall E, McMillan SS, Kelly F, et al. Burden of treatment for chronic illness: a concept analysis and review of the literature. Health Expectations (2015) 18(3):312–24. doi: 10.1111/hex.12046
24. Mair FS, May CR. Thinking about the burden of treatment. Br. Med J Publishing Group (2014) 349:g6680–80. doi: 10.1136/bmj.g6680
25. Eton DT, Ramalho de Oliveira D, Egginton JS, Ridgeway JL, Odell L, May CR, et al. Building a measurement framework of burden of treatment in complex patients with chronic conditions: a qualitative study. Patient Relat Outcome Meas (2012) 3:39–49. doi: 10.2147/PROM.S34681
26. Tran VT, Barnes C, Montori VM, Falissard B, Ravaud P. Taxonomy of the burden of treatment: a multi-country web-based qualitative study of patients with chronic conditions. BMC Med (2015) 13:115. doi: 10.1186/s12916-015-0356-x
27. Rosbach M, Andersen JS. Patient-experienced burden of treatment in patients with multimorbidity - a systematic review of qualitative data. PloS One (2017) 12(6):e0179916. doi: 10.1371/journal.pone.0179916
28. Eton DT, Ridgeway JL, Egginton JS, Tiedje K, Linzer M, Boehm DH, et al. Finalizing a measurement framework for the burden of treatment in complex patients with chronic conditions. Patient Related Outcome Measures (2015) 6:117. doi: 10.2147/PROM.S78955
29. Krska J, Morecroft CW, Rowe PH, Poole H. Measuring the impact of long-term medicines use from the patient perspective. Int J Clin Pharmacy (2014) 36(4):675–8. doi: 10.1007/s11096-014-9970-5
30. Mohammed MA, Moles RJ, Chen TF. Medication-related burden and patients’ lived experience with medicine: a systematic review and metasynthesis of qualitative studies. BMJ Open (2016) 6(2):e010035. doi: 10.1136/bmjopen-2015-010035
31. Abate T. Medication non-adherence and associated factors among diabetes patients in felege hiwot referral hospital, bahir dar city administration, Northwest Ethiopia. BMC Res Notes (2019) 12:1–6. doi: 10.1186/s13104-019-4205-4
32. Taherdoost H. Determining sample size; how to calculate survey sample size. Int J Economics Manage. Syst (2017) 2:237–39.
33. Gary PR. Adjusting for nonresponse in surveys. In: Higher education: Handbook of theory and research. Dordrecht: Springer (2007). p. 411–49.
34. Katusiime B, Corlett SA, Krska J. Development and validation of a revised instrument to measure burden of long-term medicines use: the living with medicines questionnaire version 3. Patient Related Outcome Measures (2018) 9:155. doi: 10.2147/PROM.S151143
35. Zidan A, Awaisu A, Hasan S, Kheir N. The living with medicines questionnaire: translation and cultural adaptation into the Arabic context. Value Health Regional Issues (2016) 10:36–40. doi: 10.1016/j.vhri.2016.07.001
36. Kripalani S, Risser J, Gatti ME, Jacobson TA. Development and evaluation of the adherence to refills and medications scale (ARMS) among low-literacy patients with chronic disease. Value Health (2009) 12(1):118–23. doi: 10.1111/j.1524-4733.2008.00400.x
37. Tordoff JM, Brenkley C, Krska J, Smith A. Exploring medicines burden among adults in new Zealand: a cross-sectional survey. Patient Preference Adherence (2019) 13:2171. doi: 10.2147/PPA.S231202
38. Sav A, Salehi A, Mair FS, McMillan SS. Measuring the burden of treatment for chronic disease: implications of a scoping review of the literature. BMC Med Res Methodol (2017) 17(1):140. doi: 10.1186/s12874-017-0411-8
39. Krska J, Corlett SA, Katusiime B. Complexity of medicine regimens and patient perception of medicine burden. Pharmacy (2019) 7(1):18. doi: 10.3390/pharmacy7010018
40. Gnjidic D, Tinetti M, Allore HG. Assessing medication burden and polypharmacy: finding the perfect measure. Expert Rev Clin Pharmacol (2017) 10(4):345–7. doi: 10.1080/17512433.2017.1301206
41. Tegegn H, Sebsibe G, Gizaw B, Seifu D, Tigabe M, Ayele A. Medication related quality of life among Ethiopian elderly patients with polypharmacy: The case of north gondar zone. Value Health (2017) 20(9):A523–A4. doi: 10.1016/j.jval.2017.08.709
42. İlknur Ö, Taşkın Yılmaz F, Karakoç Kumsar A, Uyar K. Knowledge, attitude and behaviours regarding medication use among older people suffering from chronic diseases. Elderly Health Journal (2020) 6(1):43–50. doi: 10.18502/ehj.v6i1.3415
43. Bosch-Lenders D, Maessen DW, Stoffers HE, Knottnerus JA, Winkens B, van den Akker M. Factors associated with appropriate knowledge of the indications for prescribed drugs among community-dwelling older patients with polypharmacy. Age ageing (2016) 45(3):402–8. doi: 10.1093/ageing/afw045
44. O'Connell MB, Johnson JF. Evaluation of medication knowledge in elderly patients. Ann. Pharmacother (1992) 26(7-8):919–21. doi: 10.1177/106002809202600711
45. Elderly lack medication knowledge. Emergency Med. News (2002) 24(10):25. doi: 10.1097/01.EEM.0000288774.06525.66
46. Mahapatra T, Chakraborty K, Mahapatra S, Mahapatra U, Pandey N, Thomson PL, et al. Burden and socio-behavioral correlates of uncontrolled abnormal glucose metabolism in an urban population of India. PloS One (2016) 11(10):e0163891. doi: 10.1371/journal.pone.0163891
47. Heckman BW, Mathew AR, Carpenter MJ. Treatment burden and treatment fatigue as barriers to health. Curr Opin Psychol (2015) 5:31–6. doi: 10.1016/j.copsyc.2015.03.004
Keywords: medication-related burden, adherence, perceived global burden, diabetes mellitus, Ethiopia
Citation: Bekalu AF, Yenit MK, Tekile M T and Birarra MK (2022) Medication-related burden and associated factors among diabetes mellitus patients at Felege Hiwot Comprehensive Specialized Hospital in northwest Ethiopia. Front. Clin. Diabetes Healthc. 3:977216. doi: 10.3389/fcdhc.2022.977216
Received: 24 June 2022; Accepted: 11 August 2022;
Published: 09 September 2022.
Edited by:
Kourosh Zarea, Ahvaz Jundishapur University of Medical Sciences, IranReviewed by:
Sorur Javanmardifard, Ahvaz Jundishapur University of Medical Sciences, IranCopyright © 2022 Bekalu, Yenit, Tekile and Birarra. This is an open-access article distributed under the terms of the Creative Commons Attribution License (CC BY). The use, distribution or reproduction in other forums is permitted, provided the original author(s) and the copyright owner(s) are credited and that the original publication in this journal is cited, in accordance with accepted academic practice. No use, distribution or reproduction is permitted which does not comply with these terms.
*Correspondence: Abaynesh Fentahun Bekalu, YWJheWZlbnRhMjNAZ21haWwuY29t
Disclaimer: All claims expressed in this article are solely those of the authors and do not necessarily represent those of their affiliated organizations, or those of the publisher, the editors and the reviewers. Any product that may be evaluated in this article or claim that may be made by its manufacturer is not guaranteed or endorsed by the publisher.
Research integrity at Frontiers
Learn more about the work of our research integrity team to safeguard the quality of each article we publish.