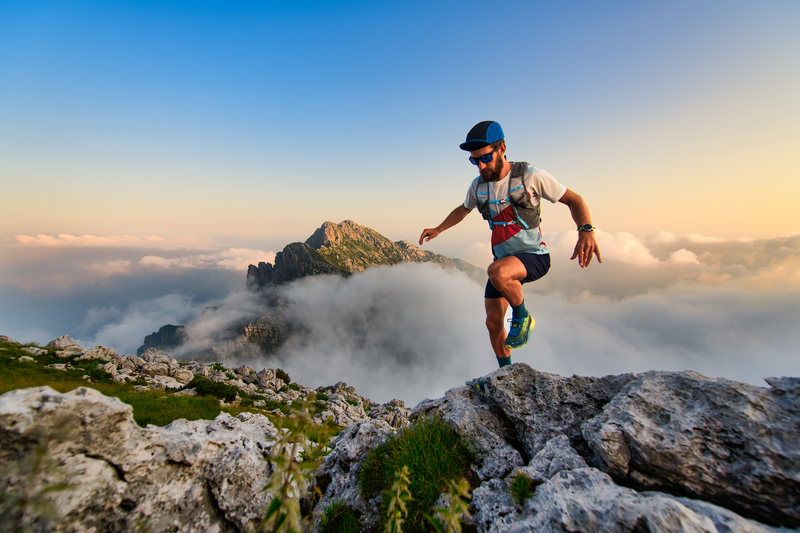
94% of researchers rate our articles as excellent or good
Learn more about the work of our research integrity team to safeguard the quality of each article we publish.
Find out more
REVIEW article
Front. Clin. Diabetes Healthc. , 19 December 2022
Sec. Diabetes Innovative Devices
Volume 3 - 2022 | https://doi.org/10.3389/fcdhc.2022.1025328
Diabetes mellitus remains the leading cause of end-stage kidney disease worldwide. Inadequate glucose monitoring has been identified as one of the gaps in care for hemodialysis patients with diabetes, and lack of reliable methods to assess glycemia has contributed to uncertainty regarding the benefit of glycemic control in these individuals. Hemoglobin A1c, the standard metric to evaluate glycemic control, is inaccurate in patients with kidney failure, and does not capture the full range of glucose values for patients with diabetes. Recent advances in continuous glucose monitoring have established this technology as the new gold standard for glucose management in diabetes. Glucose fluctuations are uniquely challenging in patients dependent on intermittent hemodialysis, and lead to clinically significant glycemic variability. This review evaluates continuous glucose monitoring technology, its validity in the setting of kidney failure, and interpretation of glucose monitoring results for the nephrologist. Continuous glucose monitoring targets for patients on dialysis have yet to be established. While continuous glucose monitoring provides a more complete picture of the glycemic profile than hemoglobin A1c and can mitigate high-risk hypoglycemia and hyperglycemia in the context of the hemodialysis procedure itself, whether the technology can improve clinical outcomes merits further investigation.
The world-wide incidence and prevalence of end-stage kidney disease (ESKD) have been increasing annually, with type 2 diabetes mellitus the leading cause of ESKD in most developed countries (1) . The global prevalence of diabetes in the ESKD population increased from 19.0% in 2000 to 29.7% in 2015, and the incident population from 22.1% to 31% (2).
Insufficient glucose monitoring has been identified as one of the gaps in care for hemodialysis patients with diabetes (3, 4). Both a previous American Diabetes Association Diabetic Kidney Disease Consensus Conference report (5) and the recent 2020 KDIGO Clinical Practice Guideline (6) have highlighted the importance of meeting recommended glucose targets. However, the benefit of glycemic control in patients with diabetes and ESKD continues to be debated (7, 8), and there is uncertainty regarding appropriate glucose targets. In the ESKD population, the lack of reliable methods to assess glycemia has hindered the attainment of optimal glycemic control (9).
The recent American Diabetes Association guidelines have highlighted the increasingly important role of continuous glucose monitoring (CGM) in glycemic management in the general diabetes population (10, 11). A recent consensus conference has recommended that CGM training programs be expanded to all healthcare professionals involved in multidisciplinary diabetes management (12). The focus of this review is to evaluate the increasing role of CGM in diabetic ESKD.
Type 2 diabetes constitutes about 90% of all cases of diabetes, and results from tissue insulin resistance with impaired peripheral glucose uptake and dysregulated hepatic glucose production, and progressive pancreatic beta-cell dysfunction (13, 14). The development of chronic kidney disease (CKD) often alters the typical natural history of diabetes. Diabetes physiology in the ESKD patient is different and more complex than in the general diabetes population, with significant alterations of both glucose and insulin homeostasis (15). The result may be wide glycemic excursions with frequent hypoglycemia and hyperglycemia (16). Factors which contribute to the complex abnormalities in glucose homeostasis include abnormal insulin metabolism with reduced renal insulin clearance, increased insulin resistance, and the effects of the dialysis procedure.
Historically, the assessment of glycemic control has relied upon two categories of testing: serum biomarkers (hemoglobin A1c [HbA1c], glycated albumin, fructosamine), and capillary blood glucose measurement (self-monitoring of blood glucose [SBGM]) (17) (Figure 1).
Figure 1 Measures for assessing glycemic control in ESKD patients with diabetes (17).
Long-term glycemic markers have limitations in end-stage kidney disease (18). The NKF-KDOQI guidelines for diabetic CKD have acknowledged that there is a deficiency in data for validating markers in patients with reduced kidney function (KDOQI) (19). As reported by the recent Kidney Disease: Improving Global Outcomes (KDIGO) guidelines, HbA1c is known to be inaccurate in patients with advanced kidney impairment (3). In the general diabetes population, a wide range of mean glucose concentrations is possible for any HbA1c value, which has been termed “the fallacy of average” (20). Furthermore, HbA1c measurements tend to run lower in advanced CKD with resultant under-estimation of hyperglycemia (15). Factors contributing to lower HbA1c values in ESKD include reduced erythrocyte lifespan/time available for glycosylation of hemoglobin, anemia, and exposure to erythropoiesis-stimulating agents (21, 22).
The role of tight glycemic control in improving clinical outcomes in ESKD remains controversial (23, 24). Using different retrospective ESKD database analyses, Kalantar-Zadeh (9) and Williams (8) found that glycemia, when considered as a continuous variable, was associated with poorer survival only at extremes of HbA1c. The optimal target HbA1c range for patients with diabetes on dialysis therefore differs from that of the general diabetes population, i.e. <7% (25). The Kidney Disease Outcome Quality Initiative (KDOQI) Clinical Practice Guidelines for Diabetes have highlighted the higher mortality risk in hemodialysis patients with HbA1c levels <6.5 or >8.0% (9). The 2020 KDIGO guidelines indicate that glycemic control must be individualized, with tighter (HbA1c < 7%) or looser (HbA1c > 8%) targets based on comorbidities, risk of hypoglycemia, life expectancy, and other factors (3, 22).
Alternative measures to HbA1c, including glycated albumin and fructosamine, have been proposed for the ESKD population. Data from Freedman et al. suggested that increasing concentrations of glycated albumin, but not of HbA1c, predicted hospitalization and survival in patients with diabetes undergoing dialysis (26). Some recent studies have shown an association between glycated albumin levels and one-year mortality, and suggested potential superiority over HbA1c (27, 28). Limited evidence suggests that the target range for glycated albumin in ESKD patients should also exceed the reference range (27).
HbA1c does not capture the full range of glucose values in patients with diabetes. Alternative established glucose metrics, including patterns of hyper/hypoglycemia and glucose variability, are known to contribute to clinical outcomes in the general diabetes population (20, 29–31). Patients with diabetes and ESKD frequently experience wide glycemic excursions, with both hyperglycemia and hypoglycemia (16). Frequent SMBG has been regarded as an essential element of diabetes management and, until the introduction of CGM, was the cornerstone of intensive diabetes management regimens. Fingerstick SMBG is associated with improvements in HbA1c, with reduced hyperglycemic excursions and rates of hypoglycemia (32). However, SBGM is limited by patient inconvenience and potential discomfort, cost, and variable device accuracy (33).
CGM has emerged as an innovative and important alternative to SBGM in diabetes management (12, 34). CGM devices measure the interstitial glucose concentration every 1-15 minutes (frequency varies depending on device type), providing a more comprehensive picture of postprandial glucose excursion and also unmasking hypoglycemia particularly in the overnight period (35). CGM devices generate a set of metrics that are valuable in assessment of glucose control, and can be used in clinical practice to complement the HbA1c measurement (36–42) (see below). Multiple subsequent randomized clinical trials have now shown unequivocal improvement in hemoglobin A1c with reduction in hypoglycemia with use of CGM by individuals with type 1 and type 2 diabetes (10, 35, 43–47). Improvements in the advances in the accuracy and ease-of-use of CGM devices have established this technology as a replacement for fingerstick SMBG and as the new gold standard in glucose monitoring (48–51).
It is widely recognized that plasma glucose levels decrease significantly during the hemodialysis procedure. Sudha et all measured capillary blood glucose levels, just before dialysis and 2 hours after dialysis, and compared with fasting and postprandial glucose levels on–off dialysis days (52). There was a significant (35.8%) decrease in blood glucose levels two hours after dialysis in comparison to predialysis levels (from mean level of 258mg/dL/14.3 mmol/L to 165 mg/dL/9.2 mmol/L). Abe et al. evaluated changes in plasma glucose levels specifically between pre- and post-dialyzer sites during hemodialysis (53). Results confirmed removal of glucose by the dialysis procedure. In hemodialysis patients with diabetes, CGM data indicate that on dialysis days mean glucose levels are lower and that glucose fluctuations, as indicated by standard deviation and mean amplitude glycemic excursion (MAGE) are greater than on days without hemodialysis (54). A component of glucose homeostasis unique to the hemodialysis procedure is the presence of dextrose, chemically identical to glucose, as a constituent of the dialysate fluid (6, 55). Addition of dextrose protects from severe hypoglycemia.
The hemodialysis procedure is known to result in removal of circulating insulin while at the same time improving insulin sensitivity. An understanding of the impact of these effects underscores the potential value of CGM in the chronic hemodialysis setting.
For patients requiring hemodialysis, insulin is frequently administered prior to the hemodialysis session. Administered insulin contributes to the intradialytic decrease in plasma glucose level noted above, but circulating insulin is removed by hemodialysis so that blood insulin levels decrease (53). The molecular size of circulating plasma insulin monomer (5.8kDa) would indicate that filtration through the membrane would contribute to its removal. Adsorption of insulin onto the dialyzer membrane is an additional factor (56).
Tissue insensitivity to insulin is a common feature of ESKD, especially in individuals with type 2 diabetes (57, 58). The mechanism remains unclear, but contributing factors may involve circulating pro-inflammatory cytokines (59). Skeletal muscle is the primary site accounting for insulin resistance in patients with uremia (60). Uremic insulin resistance is known to improve after initiation of chronic renal replacement therapy (61, 62). Using a modified euglycemic clamp methodology Sobngwi et al, evaluated the insulin requirements necessary to achieve euglycemia in the pre-, intra-, and post-hemodialysis period and noted a 25% lower exogenous insulin requirement immediately after the dialysis procedure (62).
The net effect is to make diabetes management less predictable in the ESKD patient. In general, clinical research studies suggest a prototypic peri-dialytic paradigm of hypoglycemia followed by rebound hyperglycemia shown in Figure 2 (63). In addition, circadian changes in plasma glucose levels may differ between dialysis and non-dialysis days.
Figure 2 Circadian changes in plasma glucose level in hemodialysis patients with diabetes on dialysis day (dark line) and non-dialysis day (light line). *P<0.05; **P<0.01. (Adapted with permission from (63). HD, hemodialysis; SD, standard deviation).
Of clinical relevance, the signs and symptoms of hyperglycemia will be modified, with increased thirst, fluid overload, and hyperkalemia expected but not polyuria or volume contraction. Hemodialysis patients, particularly with type 2 diabetes, rarely develop diabetic ketoacidosis (63). Development of hypoglycemia unawareness due to recurrent hypoglycemia (64) is an additional risk in the ESKD patient on dialysis. Hypoglycemia has recently been associated with the occurrence of cardiac arrhythmias in patients with diabetes and CKD (65).
ESKD patients with diabetes are known to have greater fluctuations than dialysis patients without diabetes (54). Glycemic variability – i.e., fluctuations of measures of glycemic control over a given interval of time - has been termed the “third component of dysglycemia” in diabetes (66). Accumulating data suggest that glycemic variability is an independent risk factor for diabetes complications (67, 68). Glycemic variability may be evident in both long-term measures (serial determinations of HbA1c), or in short-term measures (based on serial measures using CGM or blood glucose measurements), as shown in Figure 3 (68). Both glucose and HbA1c variability have been shown to predict hospitalization risk in ESKD (69).
Figure 3 Short-term glycemic variability, represented by within-day measures of blood glucose as determined by SBGM and CGM. Modified with permission from 68).
CGM devices measure interstitial fluid glucose. The interstitial compartment comprises approximately eighty per cent of extracellular water, the remainder comprising the plasma compartment (70). The main solute constituents (excluding glucose) of these body fluid compartments are electrolytes (71). Interstitial fluid homeostasis is considered to be regulated at the local tissue level. Fluid exchange homeostasis between the plasma and interstitium is governed by the interaction of key components of Starling’s hypothesis, such as the interstitial hydrostatic pressure (72). The pressure/volume status of the interstitium is determined by a complex interaction involving fluid inflow (blood capillary filtration), fluid outflow (lymphatics), and the interstitial compartment’s tissue compliance (73). Interstitial fluid pressures are slightly negative in healthy controls, and positive in CKD patients with edema (74). This increase in interstitial fluid pressure in CKD would be expected to result in reduced transcapillary filtration into the interstitium, preventing further fluid influx, and/or increased unidirectional outward lymphatic flow (73). Glucose dynamics between the plasma and interstitial fluid may be considered a “two-compartment” model (75). Glucose diffuses from the capillary endothelium to the interstitial compartment (76), driven by the glucose concentration gradient across capillaries, with a time lag in the passage of glucose from blood to the interstitium (77).
In individuals with kidney impairment, extracellular fluid volume, interstitial fluid volume and interstitial pressure are significantly elevated (73, 74). It is likely that there is distortion in glucose homeostasis caused by nonequilibrium conditions between the vascular compartment and the extracellular compartment (57). Although the time lag in the distribution of glucose from blood to the interstitium under normal conditions has been investigated (77, 78), changes during the hemodialysis procedure are uncertain.
In recent years,advances in sensor technology have set the stage for more widespread adoption of CGM into diabetes care (79). Among Current FDA-approved CGM devices are the Abbott Freestyle Libre (Abbott Diabetes Care, Alameda CA), Dexcom G6 (Dexcom, San Diego CA) and Medtronic Guardian (Medtronic, Northridge CA) CGM devices. These devices typically consist of a transcutaneous sensor probe that is inserted into subcutaneous tissue and measures glucose concentrations in the interstitial fluid; the glucose measurement technology in these sensor probes is electrochemical and utilizes the enzyme glucose oxidase, which generates hydrogen peroxide and an electron flow proportional to the glucose concentration. Because of the specificity of glucose oxidase for beta-D-glucose, these sensors are not expected to be subject to interference by other monosaccharides and polysaccharides [80. However, acetaminophen at doses in excess of 1gram every 6 hours and high dose ascorbic acid can affect performance of the Abbott Freestyle Libre (81). Local skin reactions from adhesive tape that secures the transmitter to the skin can occur (82, 83). Another approved device, the Eversense CGM (Senseonics, Germantown MD) is clinician-implanted, with need for an office-based procedure for insertion/removal every 90 days (84), and functions non-enzymatically, utilizing a hydrogel that fluoresces on glucose binding. Studies indicate that mannitol and tetracycline can interfere with its accuracy (85).
Traditionally, CGM devices required that the patients perform fingerstick capillary blood glucose measurements for calibration (86). However, the Abbott Freestyle Libre and Dexcom G6 are factory-calibrated, and there is no need for regular calibration to ensure CGM accuracy.
The measurement accuracy of CGM devices is assessed using plasma glucose as the reference standard (Figure 4). The mean absolute relative difference (MARD) between CGM measurements of and matched reference values is the commonly used metric to evaluate device accuracy. A MARD ¾ 10% is widely cited as the desired goal in CGM device accuracy (30, 88). However, it is noteworthy that many of the studies demonstrating the clinical benefits of CGM were conducted with devices with MARD that did not meet this cut-point (35, 89–91).
Figure 4 Measured CGM data and paired blood glucose measurements (BG profile) in a patient with diabetes (Adapted with permission from Ref (87).
Research has revealed that paired glucose concentrations in the capillary and venous compartments and the interstitial space will differ when the glucose is changing rapidly (78). Because of this, apparent accuracy of CGM devices tends to be lower in individuals with high glucose variability and at the extremes of the glucose range (92, 93). This is an important consideration in the evaluation of studies of device accuracy. In patients with chronic kidney disease, data correlating CGM and plasma glucose to validate the accuracy of CGM are limited. Riveline evaluated the relationship between CGM and blood glucose measurements in nineteen hemodialysis patients on hemodialysis studied over four days (94). There was a high correlation between mean values using the two techniques (Figure 5), and the correlations were similar in dialysis and non-dialysis patients.
Figure 5 Correlation between mean glucose levels obtained through glucose meters and CGM in hemodialysis type 2 diabetes (dark circles) and nondialysis type 2 diabetes (light circles) (Ref (94).). Correlation in the entire patient group (r = 0.84; P<0.00001). Correlation in hemodialysis type 2 patients (dark line) (r = 0.90; P<0.0001). Correlation in nondialysis type 2 patients (light line) (r = 0.81; P<0.0001).
Mambelli et al. found very good accuracy of the Abbott Libre CGM in patients with type 2 diabetes on hemodialysis, with 97% of measurements in A+B regions of the Clarke error grid (95). In contrast, another study in patients with type 2 diabetes on hemodialysis by Yajima et al. showed poorer accuracy with the Abbott Libre CGM (96). The accuracy in patients on hemodialysis is not affected by hydration status measured via impedance spectroscopy (97). A small study in twelve patients (98) showed better MARD at the beginning of the dialysis treatment (r=0.731) compared to the end of the treatment session (r=0.513), and overall 90% of the paired measurments were in the zones A/B (clinically accurate or no risk from error) of the Clarke error grid. A recent study evaluating the Dexcom G6 CGM in patients with type 1 and type 2 diabetes during dialysis showed an overall MARD 13.8% (interquartile range 4.9-18.2%) when CGM was compared to matched SBGM measurements (99). In a meta-analysis of 524 routine dialysis patients with diabetes from 23 studies, Wang et al. confirmed a good correlation of CGM and BGM values (r=0.837) (100).
The latest international guidelines on CGM for analysis and treatment goals were published in 2019 (36). “Time-in-range” describes the percentage of time spent with CGM reading between 70-180mg/dL/3.9-10.0 mmol/L). For most adults with diabetes, the consensus recommendations suggest that an appropriate goal is to achieve a percent time-in-range goal of over 70 percent, if achievable without undue risk of hypoglycemia (36, 101). Recommended treatment goals for patients with diabetes and comorbid conditions, such as chronic kidney disease, includes maintaining time-in-range >50% and time-below-range (<70 mg/dL3.9 mmol/L) <1% (36),. Several metrics exist for assessment of short-term, glucose variability (39), including the percent coefficient of variation (%CV), which is predictive of risk for severe hypoglycemia. In the past, CGM data were used to derive an “estimated A1c” calculated from mean glucose levels. However, in order to reduce confusion with measured HbA1c values, this predicted value of HbA1c is now referred to as a glucose management indicator (GMI) (31). CGM data indicate that the laboratory HbA1c may over-estimate the mean glucose in black persons compared to white persons (102).
Clinical studies using CGM in the ESKD population have explored: (1) changes in glucose levels with hemodialysis; (2) comparison of CGM to standard glycemic markers in the setting of ESKD; and, (3) clinical benefit to be derived from use of CGM in diabetes management in the ESKD population.
(1) The advent of CGM has confirmed two known glycemic patterns in patients undergoing hemodialysis treatments. Several studies have demonstrated that glucose levels overall are lower on the day of the dialysis treatment. Using 24-hour CGM data, Kazempour-Ardebili et al. reported roughly 25% lower mean glucose values in type 2 patients during the day of dialysis (103). The average 24-hour glucose levels on nondialysis days was 38 to 187mg/dL (2.1 to 10.4 mmol/L) higher, compared to the average on dialysis days. The most common glucose nadir and risk of asymptomatic hypoglycemia was within 24 hours following dialysis. Euglycemic clamp data indicate a 25% reduction in basal insulin requirements the day after dialysis compared to the day before (62). In the meta-analysis by Wang et al, the average CGM values were significantly lower during dialysis in comparison with predialysis CGM values in patients with diabetes (MD: -2.11mmol/L or -40.3mg/dL, p=0.0003) (100). Divani et al. have shown using CGM in 36 diabetic hemodialysis patients the glucose variability as determined by coefficient of variation and hypoglycemia are higher on dialysis days (104).
(2) Several studies have evaluated the comparative clinical performance of CGM with HbA1c in diabetic patients with chronic kidney disease, including patients on hemodialysis. Two recent reports on predialysis patients have addressed the question of accuracy of long-term markers such as HbA1c, using its correlation with CGM as the primary outcome measure. Presswala evaluated CGM and HbA1c in CKD patients with type 2 diabetes and eGFR levels 78-45mL/min, using mean glucose levels over 14 days and mean HbA1c levels at the end of the CGM period. The Pearson correlation coefficient was 0.82, and the correlation was not affected by the severity of CKD (105). In a similar clinical study, Zelnick reported an overall Pearson coefficient of 0.78 (28). In contrast, a much weaker correlation in ESKD was reported by Riveline et al, who compared mean CGM values and hemoglobin A1c in dialysis and nondialysis patients with diabetes. The correlation between mean CGM and hemoglobin A1c values in hemodialysis patients was only 0.47, p=0.042. Poor metabolic control was identified using CGM data, which was not suspected based on HbA1c levels, even after correction for anemia (94).
The longitudinal association of glucose levels with the HbA1c was reported in 37 diabetic hemodialysis patients who used CGM over seven days to assess glycemic control (106). The area under ROC curve (AUC) for HbA1c to detect poor glycemic control was 0.776, indicating a fair association of HbA1c with average glucose levels. In the meta-analysis by Wang et al, the correlation coefficient between CGM and HbA1c in diabetes patients on dialysis (converting values from individual studies to Fisher’s z-scores) was =0.523 (100).
(3) To date there has been limited investigation on the potential utility of CGM in the management of diabetes in patients with ESKD. In the DIALYDAB pilot study reported by Joubert et al. (107), fifteen hemodialysis patients with diabetes used two different glucose measurements – SBGM (three per day) and CGM – each for a period of six weeks, to guide their insulin therapy. SBGM and CGM profiles were remotely analyzed by a diabetes specialist, who then provided therapeutic input to dialysis physicians. Use of CGM was associated with a greater number of alterations in the insulin regimen, improved glycemic control, and fewer episodes of hypoglycemia. Kepenekian et al. evaluated the use of CGM as a tool to guide treatment decisions in a multi-center study of 28 diabetic hemodialysis patients (108). Use of CGM allowed intensification of CGM-adapted insulin therapy over three months, with significant reductions in CGM-derived glucose levels and Hb A1c, despite no increase in symptomatic hypoglycemia. In a randomized crossover trial in adults with type 2 diabetes requiring dialysis, Boughton et al. have demonstrated that use closed loop insulin therapy - with an insulin pump, CGM and control algorithm to adjust insulin delivery is associated with improved time-in-range (3.9-10.0 mmol/L) and less hypoglycemia (109)
Available guideline recommendations designed to address the use of CGM data to optimize clinical outcomes do not specifically include patients on dialysis (36). The consensus recommendations for lower risk patients with diabetes are shown in Figure 6. In comparison, it is recommended that patients with diabetes and higher risk due to comorbid conditions such as renal disease maintain time-in-range (70-180 mg/dL/3.9-10.0 mmol/L) >50% and time-below-range (<70 mg/dL/3.9 mmol/L) <1%, (Figure 6) (36).
Figure 6 Consensus recommendations for CGM-based targets in different diabetes populations (see text). Modified with permission from Ref (36)..
Despite the growing body of evidence supporting CGM use in type 1 diabetes and, more recently, the broader type 2 diabetes population, current eligibility criteria required by public and many private insurers are making access to this technology difficult for the ESKD diabetic population (36). There is a need for outcome studies demonstrating the potential benefit of CGM to provide the evidence base to support broader coverage of this technology by payers (110).
Glucose management in ESKD patients with diabetes mellitus imposes challenges on both the patient and the dialysis provider. Evidence that current Hb A1c-based indicators of glycemic control adequately predict outcomes related to morbidity and mortality in diabetic ESRD patients remains insufficient. Closer glucose monitoring of glycemia would appear to be essential in ESKD because of various factors that impact glucose metabolism, related to abnormal glucose and insulin homeostasis. The more intensive and automated glucose monitoring facilitated by CGM technology may be of particular value in hemodialysis patients, insofar as hemodialysis exaggerates the state of glycemic variability. Until recently, CGM has been inadequately studied in the ESKD population. As a result, it is important that the CGM innovation be validated and its utility determined in the ESKD population. There is a need to better understand how CGM metrics can impact clinical outcomes in the hemodialysis population. In the meantime, CGM is an accurate diagnostic tool that should empower hemodialysis care providers to recognize and mitigate high-risk hypoglycemia and hyperglycemia in the context of the hemodialysis procedure itself.
MW conceived of the review topic and was the primary author in the manuscript. Except for sections on glucose sensor technology, MW was the primary author. MW edited the entire manuscript. HW and DS are recognized experts in diabetes technology; they reviewed the content of the entire manuscript and were primary authors of the section(s) on glucose sensor technology. All authors contributed to the article and approved the submitted version.
The authors declare that the research was conducted in the absence of any commercial or financial relationships that could be construed as a potential conflict of interest.
All claims expressed in this article are solely those of the authors and do not necessarily represent those of their affiliated organizations, or those of the publisher, the editors and the reviewers. Any product that may be evaluated in this article, or claim that may be made by its manufacturer, is not guaranteed or endorsed by the publisher.
1. Johansen KL, Chertow GM, Foley RN, Gilbertson DT, Herzog CA, Ishani A, et al. US Renal data system 2020 annual data report: Epidemiology of kidney disease in the united states. Am. J. Kidney Dis. (2021) 77(4 Suppl 1):A7–8. doi: 10.1053/j.ajkd.2021.01.002
2. Cheng HT, Xu X, Lim PS, Hung KY. Worldwide epidemiology of diabetes-related end-stage renal disease, 2000-2015. Diabetes Care (2021) 44(1):89–97. doi: 10.2337/dc20-1913
3. de Boer IH, Caramori ML, Chan JCN, Heerspink HJL, Hurst C, Khunti K, et al. Executive summary of the 2020 KDIGO diabetes management in CKD guideline: evidence-based advances in monitoring and treatment. Kidney Int. (2020) 98(4):839–48. doi: 10.1016/j.kint.2020.06.024
4. Fang M, Wang D, Coresh J, Selvin E. Trends in diabetes treatment and control in U.S. adults, 1999-2018. N Engl. J. Med. (2021) 384(23):2219–28. doi: 10.1056/NEJMsa2032271
5. Tuttle KR, Bakris GL, Bilous RW, Chiang JL, de Boer IH, Goldstein-Fuchs J, et al. Diabetic kidney disease: a report from an ADA consensus conference. Am. J. Kidney Dis. (2014) 64(4):510–33. doi: 10.1053/j.ajkd.2014.08.001
6. Navaneethan SD, Zoungas S, Caramori ML, Chan JCN, Heerspink HJL, Hurst C. Diabetes management in chronic kidney disease: Synopsis of the 2020 KDIGO clinical practice guideline. Ann. Intern. Med. (2021) 174(3):385–94. doi: 10.7326/M20-5938
7. Williams ME, Lacson E Jr, Teng M, Ofsthun N, Lazarus JM. Hemodialyzed type I and type II diabetic patients in the US: Characteristics, glycemic control, and survival. Kidney Int. (2006) 70(8):1503–9. doi: 10.1038/sj.ki.5001789
8. Williams ME, Lacson E Jr, Wang W, Lazarus JM, Hakim R. Glycemic control and extended hemodialysis survival in patients with diabetes mellitus: comparative results of traditional and time-dependent cox model analyses. Clin. J. Am. Soc. Nephrol. (2010) 5(9):1595–601. doi: 10.2215/CJN.09301209
9. Kalantar-Zadeh K, Kopple JD, Regidor DL, Jing J, Shinaberger CS, Aronovitz J, et al. A1C and survival in maintenance hemodialysis patients. Diabetes Care (2007) 30(5):1049–55. doi: 10.2337/dc06-2127
10. Practice C, Draznin B, Aroda VR, Bakris G, Benson G, Brown FM, et al. 7. diabetes technology: Standards of medical care in diabetes-2022. Diabetes Care (2022) 45(Suppl 1):S97–S112. doi: 10.2337/dc22-S007
11. Practice C, Draznin B, Aroda VR, Bakris G, Benson G, Brown FM, et al. 6. glycemic targets: Standards of medical care in diabetes-2022. Diabetes Care (2022) 45(Suppl 1):S83–96. doi: 10.2337/dc22-S006
12. Fonseca VA, Grunberger G, Anhalt H, Bailey TS, Blevins T, Garg SK, et al. Continuous glucose monitoring: A consensus conference of the American association of clinical endocrinologists and American college of endocrinology. Endocr. Pract. (2016) 22(8):1008–21. doi: 10.4158/EP161392.CS
13. Reed J, Bain S, Kanamarlapudi V. A review of current trends with type 2 diabetes epidemiology, aetiology, pathogenesis, treatments and future perspectives. Diabetes Metab. Syndr. Obes. (2021) 14:3567–602. doi: 10.2147/DMSO.S319895
14. Lin Y, Sun Z. Current views on type 2 diabetes. J. Endocrinol. (2010) 204(1):1–11. doi: 10.1677/JOE-09-0260
15. Williams ME, Garg R. Glycemic management in ESRD and earlier stages of CKD. Am. J. Kidney Dis. (2014) 63(2 Suppl 2):S22–38. doi: 10.1053/j.ajkd.2013.10.049
16. Galindo RJ, Beck RW, Scioscia MF, Umpierrez GE, Tuttle KR. Glycemic monitoring and management in advanced chronic kidney disease. Endocr. Rev. (2020) 41(5):756–74. doi: 10.1210/endrev/bnaa017
17. Garg R, Williams ME. Diabetes management in the kidney patient. Med. Clin. North Am. (2013) 97(1):135–56. doi: 10.1016/j.mcna.2012.11.001
18. Bomholt T, Adrian T, Nørgaard K, Ranjan AG, Almdal T, Larsson A, et al. The use of HbA1c, glycated albumin and continuous glucose monitoring to assess glucose control in the chronic kidney disease population including dialysis. Nephron (2021) 145(1):14–9. doi: 10.1159/000511614
19. National Kidney F. KDOQI clinical practice guideline for diabetes and CKD: 2012 update. Am. J. Kidney Dis. (2012) 60(5):850–86. doi: 10.1053/j.ajkd.2012.07.005
20. Beck RW, Connor CG, Mullen DM, Wesley DM, Bergenstal RM. The fallacy of average: How using HbA1c alone to assess glycemic control can be misleading. Diabetes Care (2017) 40(8):994–9. doi: 10.2337/dc17-0636
21. Peacock TP, Shihabi ZK, Bleyer AJ, Dolbare EL, Byers JR, Knovich MA, et al. Comparison of glycated albumin and hemoglobin A(1c) levels in diabetic subjects on hemodialysis. Kidney Int. (2008) 73(9):1062–8. doi: 10.1038/ki.2008.25
22. Chen HS, Wu TE, Lin HD, Jap TS, Hsiao LC, Lee SH, et al. Hemoglobin A(1c) and fructosamine for assessing glycemic control in diabetic patients with CKD stages 3 and 4. Am. J. Kidney Dis. (2010) 55(5):867–74. doi: 10.1053/j.ajkd.2009.10.064
23. Ou SH, Chen HY, Fang NW, Yin CH, Chen CL, Chen JS. Effect of anti-diabetic drugs in dialysis patients with diabetes: a nationwide retrospective cohort study. Cardiovasc. Diabetol. (2021) 20(1):179. doi: 10.1186/s12933-021-01364-w
24. Shurraw S, Majumdar SR, Thadhani R, Wiebe N, Tonelli M, Alberta Kidney Disease Network. Glycemic control and the risk of death in 1,484 patients receiving maintenance hemodialysis. Am. J. Kidney Dis. (2010) 55(5):875–84. doi: 10.1053/j.ajkd.2009.12.038
25. Hill CJ, Maxwell AP, Cardwell CR, Freedman BI, Tonelli M, Emoto M, et al. Glycated hemoglobin and risk of death in diabetic patients treated with hemodialysis: a meta-analysis. Am. J. Kidney Dis. (2014) 63(1):84–94. doi: 10.1053/j.ajkd.2013.06.020
26. Freedman BI, Andries L, Shihabi ZK, Rocco MV, Byers JR, Cardona CY, et al. Glycated albumin and risk of death and hospitalizations in diabetic dialysis patients. Clin. J. Am. Soc. Nephrol. (2011) 6(7):1635–43. doi: 10.2215/CJN.11491210
27. Hoshino J, Hamano T, Abe M, Hasegawa T, Wada A, Ubara Y, et al. Glycated albumin versus hemoglobin A1c and mortality in diabetic hemodialysis patients: a cohort study. Nephrol. Dial Transplant. (2018) 33(7):1150–8. doi: 10.1093/ndt/gfy014
28. Zelnick LR, Batacchi ZO, Ahmad I, Dighe A, Little RR, Trence DL, et al. Continuous glucose monitoring and use of alternative markers to assess glycemia in chronic kidney disease. Diabetes Care (2020) 43(10):2379–87. doi: 10.2337/dc20-0915
29. Rhee CM, Leung AM, Kovesdy CP, Lynch KE, Brent GA, et al. Updates on the management of diabetes in dialysis patients. Semin. Dial (2014) 27(2):135–45. doi: 10.1111/sdi.12198
30. Danne T, Nimri R, Battelino T, Bergenstal RM, Close KL, Devries JH, et al. International consensus on use of continuous glucose monitoring. Diabetes Care (2017) 40(12):1631–40. doi: 10.2337/dc17-1600
31. Bergenstal RM, Beck RW, Close KL, Grunberger G, Sacks DB, Kowalski A, et al. Glucose management indicator (GMI): A new term for estimating A1C from continuous glucose monitoring. Diabetes Care (2018) 41(11):2275–80. doi: 10.2337/dc18-1581
32. Szymborska-Kajanek A, Psurek A, Hese R, Strojek K. Self-monitoring of blood glucose in treatment of type 2 diabetes. Diabetes Res. Clin. Pract. (2009) 86 Suppl 1:S49–52. doi: 10.1016/S0168-8227(09)70009-9
33. Klonoff DC, Parkes JL, Kovatchev BP, Kerr D, Bevier WC, Brazg RL, et al. Investigation of the accuracy of 18 marketed blood glucose monitors. Diabetes Care (2018) 41(8):1681–8. doi: 10.2337/dc17-1960
34. Peters AL, Ahmann AJ, Battelino T, Evert A, Hirsch IB, Murad MH, et al. Diabetes technology-continuous subcutaneous insulin infusion therapy and continuous glucose monitoring in adults: An endocrine society clinical practice guideline. J. Clin. Endocrinol. Metab. (2016) 101(11):3922–37. doi: 10.1210/jc.2016-2534
35. Juvenile Diabetes Research Foundation Continuous Glucose Monitoring study group, Tamborlane WV, Beck RW, Bode BW, Buckingham B, Chase HP, et al. Continuous glucose monitoring and intensive treatment of type 1 diabetes. N Engl. J. Med. (2008) 359(14):1464–76. doi: 10.1056/NEJMoa0805017
36. Battelino T, Danne T, Bergenstal RM, Amiel SA, Beck R, Biester T, et al. Clinical targets for continuous glucose monitoring data interpretation: Recommendations from the international consensus on time in range. Diabetes Care (2019) 42(8):1593–603. doi: 10.2337/dci19-0028
37. Beck RW, Bergenstal RM, Cheng P, Kollman C, Carlson AL, Johnson ML, et al. The relationships between time in range, hyperglycemia metrics, and HbA1c. J. Diabetes Sci. Technol. (2019) 13(4):614–26. doi: 10.1177/1932296818822496
38. Rodbard D. Glucose time in range, time above range, and time below range depend on mean or median glucose or HbA1c, glucose coefficient of variation, and shape of the glucose distribution. Diabetes Technol. Ther. (2020) 22(7):492–500. doi: 10.1089/dia.2019.0440
39. Rodbard D. Glucose variability: A review of clinical applications and research developments. Diabetes Technol. Ther. (2018) 20(S2):S25–S215. doi: 10.1089/dia.2018.0092
40. Julla JB, Jacquemier P, Fagherazzi G, Vidal-Trecan T, Juddoo V, Jaziri A, et al. Is the consensual threshold for defining high glucose variability implementable in clinical practice? Diabetes Care (2021) 44(7):1722–5. doi: 10.2337/dc20-1847
41. Boland E, Monsod T, Delucia M, Brandt CA, Fernando S, Tamborlane WV, et al. Limitations of conventional methods of self-monitoring of blood glucose: lessons learned from 3 days of continuous glucose sensing in pediatric patients with type 1 diabetes. Diabetes Care (2001) 24(11):1858–62. doi: 10.2337/diacare.24.11.1858
42. Kaufman FR, Austin J, Neinstein A, Jeng L, Halvorson M, Devoe DJ, et al. Nocturnal hypoglycemia detected with the continuous glucose monitoring system in pediatric patients with type 1 diabetes. J. Pediatr. (2002) 141(5):625–30. doi: 10.1067/mpd.2002.129175
43. Beck RW, Riddlesworth T, Ruedy K, Ahmann A, Bergenstal R, Haller S, et al. Effect of continuous glucose monitoring on glycemic control in adults with type 1 diabetes using insulin injections: The DIAMOND randomized clinical trial. JAMA (2017) 317(4):371–8. doi: 10.1001/jama.2016.19975
44. Laffel LM, Kanapka LG, Beck RW, Bergamo K, Clements MA, Criego A, et al. Effect of continuous glucose monitoring on glycemic control in adolescents and young adults with type 1 diabetes: A randomized clinical trial. JAMA (2020) 323(23):2388–96. doi: 10.1001/jama.2020.6940
45. Beck RW, Riddlesworth TD, Ruedy K, Ahmann A, Haller S, Kruger D, et al. Continuous glucose monitoring versus usual care in patients with type 2 diabetes receiving multiple daily insulin injections: A randomized trial. Ann. Intern. Med. (2017) 167(6):365–74. doi: 10.7326/M16-2855
46. Vigersky RA, Fonda SJ, Chellappa M, Walker MS, Ehrhardt NM. Short- and long-term effects of real-time continuous glucose monitoring in patients with type 2 diabetes. Diabetes Care (2012) 35(1):32–8. doi: 10.2337/dc11-1438
47. Martens T, Beck RW, Bailey R, Ruedy KJ, Calhoun P, Peters AL, et al. Effect of continuous glucose monitoring on glycemic control in patients with type 2 diabetes treated with basal insulin: A randomized clinical trial. JAMA (2021) 325(22):2262–72. doi: 10.1001/jama.2021.7444
48. Bronstone A, Graham C. The potential cost implications of averting severe hypoglycemic events requiring hospitalization in high-risk adults with type 1 diabetes using real-time continuous glucose monitoring. J. Diabetes Sci. Technol. (2016) 10(4):905–13. doi: 10.1177/1932296816633233
49. Fonda SJ, Graham G, Munakata J, Powers JM, Price D, Vigersky RA. The cost-effectiveness of real-time continuous glucose monitoring (RT-CGM) in type 2 diabetes. J. Diabetes Sci. Technol. (2016) 10(4):898–904. doi: 10.1177/1932296816628547
50. Parkin CG, Graham C, Smolskis J. Continuous glucose monitoring use in type 1 diabetes: Longitudinal analysis demonstrates meaningful improvements in HbA1c and reductions in health care utilization. J. Diabetes Sci. Technol. (2017) 11(3):522–8. doi: 10.1177/1932296817693253
51. Freckmann G, Pleus S, Grady M, Setford S, Levy B. Measures of accuracy for continuous glucose monitoring and blood glucose monitoring devices. J. Diabetes Sci. Technol. (2019) 13(3):575–83. doi: 10.1177/1932296818812062
52. Sudha MJ, Salam HS, Viveka S, Udupa AL. Assessment of changes in insulin requirement in patients of type 2 diabetes mellitus on maintenance hemodialysis. J. Nat. Sci. Biol. Med. (2017) 8(1):64–8. doi: 10.4103/0976-9668.198348
53. Abe M, Kaizu K, Matsumoto K. Plasma insulin is removed by hemodialysis: evaluation of the relation between plasma insulin and glucose by using a dialysate with or without glucose. Ther. Apher Dial (2007) 11(4):280–7. doi: 10.1111/j.1744-9987.2007.00491.x
54. Jin YP, Su XF, Yin GP, Xu XH, Lou JZ, Chen JJ, et al. Blood glucose fluctuations in hemodialysis patients with end stage diabetic nephropathy. J. Diabetes Complications (2015) 29(3):395–9. doi: 10.1016/j.jdiacomp.2014.12.015
55. Wathen RL, Keshaviah P, Hommeyer P, Cadwell K, Comty CM. The metabolic effects of hemodialysis with and without glucose in the dialysate. Am. J. Clin. Nutr. (1978) 31(10):1870–5. doi: 10.1093/ajcn/31.10.1870
56. Abe M, Okada K, Ikeda K, Matsumoto S, Soma M, Matsumoto K. Characterization of insulin adsorption behavior of dialyzer membranes used in hemodialysis. Artif. Organs (2011) 35(4):398–403. doi: 10.1111/j.1525-1594.2010.01112.x
57. Rahhal MN, Gharaibeh NE, Rahimi L, Ismail-Beigi F. Disturbances in insulin-glucose metabolism in patients with advanced renal disease with and without diabetes. J. Clin. Endocrinol. Metab. (2019) 104(11):4949–66. doi: 10.1210/jc.2019-00286
58. Mak RH, DeFronzo RA. Glucose and insulin metabolism in uremia. Nephron (1992) 61(4):377–82. doi: 10.1159/000186953
59. Shoelson SE, Lee J, Goldfine AB. Inflammation and insulin resistance. J. Clin. Invest. (2006) 116(7):1793–801. doi: 10.1172/JCI29069
60. Friedman JE, Dohm GL, Elton CW, Rovira A, Chen JJ, Leggett-Frazier N, et al. Muscle insulin resistance in uremic humans: glucose transport, glucose transporters, and insulin receptors. Am. J. Physiol. (1991) 261(1 Pt 1):E87–94. doi: 10.1152/ajpendo.1991.261.1.E87
61. Kobayashi S, Maejima S, Ikeda T, Nagase M. Impact of dialysis therapy on insulin resistance in end-stage renal disease: comparison of haemodialysis and continuous ambulatory peritoneal dialysis. Nephrol. Dial Transplant. (2000) 15(1):65–70. doi: 10.1093/ndt/15.1.65
62. Sobngwi E, Enoru S, Ashuntantang G, Azabji-Kenfack M, Dehayem M, Onana A, et al. Day-to-day variation of insulin requirements of patients with type 2 diabetes and end-stage renal disease undergoing maintenance hemodialysis. Diabetes Care (2010) 33(7):1409–12. doi: 10.2337/dc09-2176
63. Nakao T, Inaba M, Abe M, Kaizu K, Shima K, Babazono T, et al. Best practice for diabetic patients on hemodialysis 2012. Ther. Apher Dial (2015) 19 Suppl 1:40–66. doi: 10.1111/1744-9987.12299
64. Cryer PE. Mechanisms of hypoglycemia-associated autonomic failure in diabetes. N Engl. J. Med. (2013) 369(4):362–72. doi: 10.1056/NEJMra1215228
65. Bansal N, Zelnick LR, Hirsch IB, Trence D, Akoum N, de Boer IH. Temporal relationship of glycemia with cardiac arrhythmias in patients with type 2 diabetes and CKD. Am. J. Kidney Dis. (2021) 77(6):988–90. doi: 10.1053/j.ajkd.2020.08.008
66. Monnier L, Colette C, Owens DR. Glycemic variability: the third component of the dysglycemia in diabetes. is it important? how to measure it? J. Diabetes Sci. Technol. (2008) 2(6):1094–100. doi: 10.1177/193229680800200618
67. Gorst C, Kwok CS, Aslam S, Buchan I, Kontopantelis E, Myint PK, et al. Long-term glycemic variability: adverse clinical outcomes and how to improve it? Diabetes Care (2015) 38(12):2354–69. doi: 10.2337/dc15-1188
68. Zhou Z, Sun B, Huang S, Zhu C, Bian M. Glycaemic variability in diabetes: clinical and therapeutic implications. Cardiovasc. Diabetol. (2020) 19:102–16. doi: 10.1186/512933–020–1085-6
69. Williams ME, Lacson JRE, Teng M, Hakim RM, Lazarus JM. Extremes of glycemic control (HbA1c) increase hospitalization risk in diabetic hemodialysis patients in the USA. Am. J. Nephrol. (2009) 29(1):54–61. doi: 10.1159/000151276
70. Valtin H. Renal dysfunction: Mechanisms inolved in fluid and solute balance. Boston: Little, Brown (1973).
71. Fogh-Andersen N, Altura BM, Altura BT, Siggaard-Andersen O. Composition of interstitial fluid. Clin. Chem. (1995) 41(10):1522–5. doi: 10.1093/clinchem/41.10.1522
72. Starling EH. On the absorption of fluids from the connective tissue spaces. J. Physiol. (1896) 19(4):312–26. doi: 10.1113/jphysiol.1896.sp000596
73. Titze J. Interstitial fluid homeostasis and pressure: news from the black box. Kidney Int. (2013) 84(5):869–71. doi: 10.1038/ki.2013.287
74. Ebah LM, Wiig H, Dawidowska I, O’Toole C, Summers A, Nikam M, et al. Subcutaneous interstitial pressure and volume characteristics in renal impairment associated with edema. Kidney Int. (2013) 84(5):980–8. doi: 10.1038/ki.2013.208
75. Youn JH, Kim JK, Steil GM. Assessment of extracellular glucose distribution and glucose transport activity in conscious rats. Am. J. Physiol. (1995) 268(4 Pt 1):E712–21. doi: 10.1152/ajpendo.1995.268.4.E712
76. Zierler K. Whole body glucose metabolism. Am. J. Physiol. (1999) 276(3):E409–26. doi: 10.1152/ajpendo.1999.276.3.E409
77. Basu A, Dube S, Veettil S, Slama M, Kudva YC, Peyser T, et al. Time lag of glucose from intravascular to interstitial compartment in type 1 diabetes. J. Diabetes Sci. Technol. (2015) 9(1):63–8. doi: 10.1177/1932296814554797
78. Schiavon M, Dalla Man C, Dube S, Slama M, Kudva YC, Peyser T, et al. Modeling plasma-to-Interstitium glucose kinetics from multitracer plasma and microdialysis data. Diabetes Technol. Ther. (2015) 17(11):825–31. doi: 10.1089/dia.2015.0119
79. Rodbard D. Continuous glucose monitoring: A review of recent studies demonstrating improved glycemic outcomes. Diabetes Technol. Ther. (2017) 19(S3):S25–37. doi: 10.1089/dia.2017.0035
80. Calhoun P, Johnson TK, Hughes J, Price D, Balo AK. Resistance to acetaminophen interference in a novel continuous glucose monitoring system. J. Diabetes Sci. Technol. (2018) 12(2):393–6. doi: 10.1177/1932296818755797
81. Feldman B, Brazg R, Schwartz S, Weinstein R. A continuous glucose sensor based on wired enzyme technology – results from a 3-day trial in patients with type 1 diabetes. Diabetes Technol. Ther. (2003) 5(5):769–79. doi: 10.1089/152091503322526978
82. Pyl J, Dendooven E, Eekelen IV, Den Brinker M, Dotremont H, France A, et al. Prevalence and prevention of contact dermatitis caused by FreeStyle libre: A monocentric experience. Diabetes Care (2020) 43(4):918–20. doi: 10.2337/dc19-1354
83. Asarani NAM, Reynolds AN, Boucher SE, de Bock M, Wheeler BJ. Cutaneous complications with continuous or flash glucose monitoring use: Systematic review of trials and observational studies. J. Diabetes Sci. Technol. (2020) 14(2):328–37. doi: 10.1177/1932296819870849
84. Joseph JI. Review of the long-term implantable senseonics continuous glucose monitoring system and other continuous glucose monitoring systems. J. Diabetes Sci. Technol. (2021) 15(1):167–73. doi: 10.1177/1932296820911919
85. Lorenz C, Sandoval W, Mortellaro M. Interference assessment of various endogenous and exogenous substances on the performance of the eversense long-term implantable continuous glucose monitoring system. Diabetes Technol. Ther. (2018) 20(5):344–52. doi: 10.1089/dia.2018.0028
86. Wolpert H. Establishing a continuous glucose monitoring program. J. Diabetes Sci. Technol. (2008) 2(2):307–10. doi: 10.1177/193229680800200224
87. Reiterer F, Polterauer P, Schoemaker M, Schmelzeisen-Redecker G, Freckmann G, Heinemann L, et al. Significance and reliability of MARD for the accuracy of CGM systems. J. Diabetes Sci. Technol. (2017) 11(1):59–67. doi: 10.1177/1932296816662047
88. Kovatchev BP, Patek SD, Ortiz EA, Breton MD. Assessing sensor accuracy for non-adjunct use of continuous glucose monitoring. Diabetes Technol. Ther. (2015) 17(3):177–86. doi: 10.1089/dia.2014.0272
89. Ruedy KJ, Parkin CG, Riddlesworth TD, Graham C., DIAMOND Study Group. Continuous glucose monitoring in older adults with type 1 and type 2 diabetes using multiple daily injections of insulin: Results from the DIAMOND trial. J. Diabetes Sci. Technol. (2017) 11(6):1138–46. doi: 10.1177/1932296817704445
90. Nakamura K, Balo A. The accuracy and efficacy of the dexcom G4 platinum continuous glucose monitoring system. J. Diabetes Sci. Technol. (2015) 9(5):1021–6. doi: 10.1177/1932296815577812
91. Boscari F, Galasso S, Facchinetti A, Marescotti MC, Vallone V, Amato AML, et al. FreeStyle libre and dexcom G4 platinum sensors: Accuracy comparisons during two weeks of home use and use during experimentally induced glucose excursions. Nutr. Metab. Cardiovasc. Dis. (2018) 28(2):180–6. doi: 10.1016/j.numecd.2017.10.023
92. Bailey T, Bode BW, Christiansen MP, Klaff LJ, Alva S. The performance and usability of a factory-calibrated flash glucose monitoring system. Diabetes Technol. Ther. (2015) 17(11):787–94. doi: 10.1089/dia.2014.0378
93. Wadwa RP, Laffel LM, Shah VN, Garg SK. Accuracy of a factory-calibrated, real-time continuous glucose monitoring system during 10 days of use in youth and adults with diabetes. Diabetes Technol. Ther. (2018) 20(6):395–402. doi: 10.1089/dia.2018.0150
94. Riveline JP, Teynie J, Belmouaz S, Franc S, Dardari D, Bauwens M, et al. Glycaemic control in type 2 diabetic patients on chronic haemodialysis: use of a continuous glucose monitoring system. Nephrol. Dial Transplant. (2009) 24(9):2866–71. doi: 10.1093/ndt/gfp181
95. Mambelli E, Cristino S, Mosconi G, Göbl C, Tura A. Flash glucose monitoring to assess glycemic control and variability in hemodialysis patients: The GIOTTO study. Front. Med. (Lausanne) (2021) 8:617891. doi: 10.3389/fmed.2021.617891
96. Yajima T, Takahashi H, Yasuda K. Comparison of interstitial fluid glucose levels obtained by continuous glucose monitoring and flash glucose monitoring in patients with type 2 diabetes mellitus undergoing hemodialysis. J. Diabetes Sci. Technol. (2020) 14(6):1088–94. doi: 10.1177/1932296819882690
97. Genua I, Sanchez-Hernandez J, Martinez MJ, Pujol I, Places J, Gonzalez C, et al. Accuracy of flash glucose monitoring in patients with diabetes mellitus on hemodialysis and its relationship with hydration status. J. Diabetes Sci. Technol. (2021) 15(6):1308–12. doi: 10.1177/1932296820975057
98. Hissa MRN, Hissa PNG, Guimaraes SB, Hissa MN. Use of continuous glucose monitoring system in patients with type 2 mellitus diabetic during hemodialysis treatment. Diabetol. Metab. Syndr. (2021) 13(1):104. doi: 10.1186/s13098-021-00722-8
99. Villard O, Breton MD, Rao S, Voelmle MK, Fuller MR, Myers HE, et al. Accuracy of a factory-calibrated continuous glucose monitor in individuals with diabetes on hemodialysis. Diabetes Care (2022) 45(7):1666–9. doi: 10.2337/figshare.19491329
100. Wang F, Wang D, Lu XL, Sun XM, Duan BH. Continuous glucose monitoring in diabetes patients with chronic kidney disease on dialysis: a meta-analysis. Minerva Endocrinol. (2020) 47(3):325–33. doi: 10.23736/S0391-1977.20.03284-8
101. Grunberger G, Sherr J, Allende M, Blevins T, Bode B, Handelsman Y, et al. American Association of clinical endocrinology clinical practice guideline: The use of advanced technology in the management of persons with diabetes mellitus. Endocr. Pract. (2021) 27(6):505–37. doi: 10.1016/j.eprac.2021.04.008
102. Bergenstal RM, Gal RL, Connor CG, Gubitosi-Klug R, Kruger D, Olson BA, et al. Racial differences in the relationship of glucose concentrations and hemoglobin A1c levels. Ann. Intern. Med. (2017) 167(2):95–102. doi: 10.7326/M16-2596
103. Kazempour-Ardebili S, Lecamwasam VL, Dassanyake T, Frankel AH, Tam FW, Dornhorst A, et al. Assessing glycemic control in maintenance hemodialysis patients with type 2 diabetes. Diabetes Care (2009) 32(7):1137–42. doi: 10.2337/dc08-1688
104. Divani M, Georgianos PI, Didangelos T, Liakopoulos V, Makedou K, Iliadis F, et al. Assessment of hyperglycemia, hypoglycemia and inter-day glucose variability using continuous glucose monitoring among diabetic patients on chronic hemodialysis. J. Clin. Med. (2021) 10(18):4116. doi: 10.3390/jcm10184116
105. Presswala L, Hong S, Harris Y, Romao I, Zhang M, Jhaveri KD, et al. Continuous glucose monitoring and glycemic control in patients with type 2 diabetes mellitus and CKD. Kidney Med. (2019) 1(5):281–7. doi: 10.1016/j.xkme.2019.07.006
106. Divani M, Georgianos PI, Didangelos T, Iliadis F, Makedou A, Hatzitolios A, et al. Comparison of glycemic markers in chronic hemodialysis using continuous glucose monitoring. Am. J. Nephrol. (2018) 47(1):21–9. doi: 10.1159/000485843
107. Joubert M, Fourmy C, Henri P, Ficheux M, Lobbedez T, Reznik Y. Effectiveness of continuous glucose monitoring in dialysis patients with diabetes: the DIALYDIAB pilot study. Diabetes Res. Clin. Pract. (2015) 107(3):348–54. doi: 10.1016/j.diabres.2015.01.026
108. Kepenekian L, Smagala A, Meyer L, Imhoff O, Alenabi F, Serb L, et al. Continuous glucose monitoring in hemodialyzed patients with type 2 diabetes: a multicenter pilot study. Clin. Nephrol. (2014) 82(4):240–6. doi: 10.5414/CN108280
109. Boughton CK, Tripyla A, Hartnell S, Daly A, Herzig D, Wilinska ME, et al. Fully automated closed-loop glucose control compared with standard insulin therapy in adults with type 2 diabetes requiring dialysis: an open-label, randomized crossover trial. Nat. Med. (2021) 27(8):1471–6. doi: 10.1038/s41591-021-01453-z
110. Petrie JR, Peters AL, Bergenstal RM, Holl RW, Fleming GA, Heinemann L. Improving the clinical value and utility of CGM systems: issues and recommendations : A joint statement of the European association for the study of diabetes and the American diabetes association diabetes technology working group. Diabetologia (2017) 60(12):2319–28. doi: 10.1007/s00125-017-4463-4
Keywords: Diabetes, glucose sensors, hemodialysis, glycemic control, complications
Citation: Williams ME, Steenkamp D and Wolpert H (2022) Making sense of glucose sensors in end-stage kidney disease: A review. Front. Clin. Diabetes Healthc. 3:1025328. doi: 10.3389/fcdhc.2022.1025328
Received: 22 August 2022; Accepted: 27 October 2022;
Published: 19 December 2022.
Edited by:
Mudassir Mohammad Rashid, Illinois Institute of Technology, United StatesReviewed by:
Richard Ephraim, University of Cape Coast, GhanaCopyright © 2022 Williams, Steenkamp and Wolpert. This is an open-access article distributed under the terms of the Creative Commons Attribution License (CC BY). The use, distribution or reproduction in other forums is permitted, provided the original author(s) and the copyright owner(s) are credited and that the original publication in this journal is cited, in accordance with accepted academic practice. No use, distribution or reproduction is permitted which does not comply with these terms.
*Correspondence: Mark E. Williams, bWFyay53aWxsaWFtc0Bqb3NsaW4uaGFydmFyZC5lZHU=
Disclaimer: All claims expressed in this article are solely those of the authors and do not necessarily represent those of their affiliated organizations, or those of the publisher, the editors and the reviewers. Any product that may be evaluated in this article or claim that may be made by its manufacturer is not guaranteed or endorsed by the publisher.
Research integrity at Frontiers
Learn more about the work of our research integrity team to safeguard the quality of each article we publish.