- 1Peers for Progress, Department of Health Behavior, Gillings School of Global Public Health, University of North Carolina at Chapel Hill, Chapel Hill, NC, United States
- 2Population Health Team, Vanguard Medical Group, Verona, NJ, United States
- 3Clinical Services and Research, WellDoc, Inc., Columbia, MD, United States
Purpose: This study examined integration of peer support and a Food and Drug Administration-cleared, diabetes management app (DMA) in diabetes self-management support as a scalable model for those with type 2 diabetes mellitus (T2DM).
Methods: Two lay health Coaches delivered telephone-based self-management support to adults (N = 43) with T2DM recruited through a primary group practice. Those eligible were offered no-cost access to DMA for the entire 6-month study. Coaches introduced DMA and contacted individuals by phone and text with frequency dependent on participant needs/preferences. DMA supported monitoring of blood glucose, carbohydrate intake, and medication use, as well as messaging personalized to participants’ medication regimens. Clinical data were extracted from DMA, electronic medical records, and Coaches’ records. Structured interviews of 12 participants, 2 Coaches, and 5 project staff were analyzed using deductive pre-identified codes (regarding adoptability, patterns of use, value added, complementarity, and sustainability) utilizing standard procedures for qualitative analysis.
Results: Of the 43 participants, 38 (88.4%) enrolled in DMA. In general, participants used both DMA and lay health coaches, averaging 144.14 DMA entries (structured, e.g., medications, and free form, e.g., “ate at a restaurant” and “stressed”) and 5.86 coach contacts over the 6-month intervention. Correlation between DMA entries and coach contacts (r = .613, p < 0.001) was consistent with complementarity as were participants’ and coaches’ observations that (a) DMA facilitated recognition of patterns and provided reminders and suggestions to achieve self-management plans, whereas (b) coaching provided motivation and addressed challenges that emerged. Mean hemoglobin A1c (A1c) declined from 9.93% to 8.86% (p < 0.001), with no pattern of coaching or DMA use significantly related to reductions. Staff identified resources to coordinate coach/DMA interventions as a major sustainability challenge.
Conclusions: DMA and peer support for diabetes management are compatible and complementary. Additional practice integration research is needed for adoption and scale-up.
Introduction
Integration is important across many dimensions of diabetes care, such as individual self-management behaviors according to the ADCES7 Self-Care Behaviors™ (1) as well as across varied sources of care (2). This project developed and tested the integration of two sources of support: lay health coaching, idiomatically “soft touch,” and digital health support—“high tech”—for individuals with type 2 diabetes mellitus (T2DM).
Systematic reviews have documented the benefits of peer support for people with diabetes (primarily T2DM) (3–8), as well as many other health concerns (9, 10). Given effectiveness, the challenge for peer support in diabetes care is to develop models that are feasible, scalable, and cost-effective in real-world settings. At the same time, digital health platforms such as smartphone apps have shown effectiveness in diabetes prevention (11–13) and management (14–17), including a review of reviews that found average reductions of 0.5 points in glycated hemoglobin (A1c), mostly among those with T2DM (18). Digital health solutions support individuals in their daily self-management activities and provide valuable patient-generated data to providers to enhance shared decision-making regarding treatment decisions (19). The convenience and low cost of digital health appeal to people that might otherwise face financial, logistical, or communication barriers when participating in face-to-face interventions. The challenges for digital health include engaging and retaining users, and addressing complex needs (12, 20–22).
Evidence from these two areas of study point to the unrealized potential of well-integrated peer support and digital health. A systematic review of web-based strategies in diabetes self-management (20) noted that interventions incorporating peer support were more likely to be effective than those that did not. Furthermore, a meta-analysis found that diabetes mobile phone apps reduced A1c among individuals with type 2 diabetes and the effect size was associated with the amount of feedback from healthcare professionals (23), suggesting that apps can be more effective when there is someone to provide live support. Peer support can provide grounding for digital apps, promoting uptake and continued use by incorporating those tools into routine contacts. Reciprocally, digital health may provide data to prompt and guide the work of peer supporters, while also reducing the burden of routine tasks such as monitoring key behaviors and indicators like blood glucose. Indeed, a 2020 review of reviews and gap analysis examining in-person and technology mediated peer support (8) identified as a gap exploration of “technology-mediated peer support, beyond voice-based telephones … [and including] … technology-mediated peer support modalities (i.e., video conferencing, SMS text message, social media).”
This study focused on a diabetes management app (DMA) for T2DM developed by WellDoc, Inc., a collaborator in the present project. The DMA had been cleared by the Food and Drug Administration. Using individuals’ own data (structured and free form, e.g., medications, blood glucose readings, carbohydrates, exercise, or “ate at a restaurant,” “stressed,” and “felt sick”), the DMA responds with adaptive messaging that is personalized to individuals’ medication regimens, and aligned with standards of care of the American Diabetes Association and the Association of Diabetes Care & Education Specialists (ADCES, formerly the American Association of Diabetes Educators) (24, 25). It also offers messaging and sharing clinical reports with individuals’ providers to enhance patient-provider communications and shared decision-making. Randomized controlled trials of the DMA software demonstrated significant reductions in A1c of 1.9 and 2.0 points in intervention groups (26, 27). At the time of the present project, the DMA was available by prescription but provided free to participants in the study. It is now also available without prescription.
The present project developed and assessed the offer of peer support and the DMA for self-management education and support for adults with T2DM in a primary care group practice setting. Drawing from the expanding literatures in implementation and dissemination research (28–30), key points of evaluation included (a) patterns of use, including how much participants would utilize each of peer support and DMA, (b) clinical changes and participants’ as well as staff observations regarding the value added by the combination, (c) participant and staff observations as well as patterns of use regarding the complementarity of peer support and DMA, and (d) staff comments regarding the sustainability of the combination within their clinical setting.
Complementarity has many meanings including in common language in which, say, one item of clothing is said to complement, that is, go well with, another. In behavioral economics (31), complementarity refers to a positive association between consumption of two commodities, e.g., bread and butter, in response to price changes for either. As price changes alter the consumption of one, the consumption of the other will change in the same direction. That is, reducing the price of bread leads to increased consumption of not only bread but also butter. Both of these share the sense that things “go together.” It is in that sense that we consider complementarity here, that is that use of the DMA and engagement with the Coaches might tend to go together, in contrast to one replacing the other, “substitutability” in behavioral economics terms.
Methods
Research Setting
In 2015, Peers for Progress in the Gillings School of Global Public Health at the University of North Carolina-Chapel Hill (UNC-CH), WellDoc, Inc., and Vanguard Medical Group in New Jersey (Vanguard) joined to pursue this project. Vanguard’s eight primary care sites are recognized patient-centered medical homes with care coordination processes to manage high-risk individuals, electronic medical records (EMRs), disease registries, quality metrics tracking, patient portal, and online appointment scheduling. Vanguard’s clinical staff were involved throughout the project to ensure feasibility. The study was approved by the Institutional Review Board at UNC-CH. Vanguard, WellDoc, and UNC-CH entered into a data use agreement to facilitate data sharing and analysis.
Formative Evaluation and Intervention Development
UNC-CH researchers conducted a formative evaluation in June–July 2015, following standard health education methods (32), to determine the feasibility of combining peer support and digital health. Telephone interviews were conducted with 4 care coordinators, 1 physician’s assistant, and 4 patients with T2DM at Vanguard (33). Vanguard patients each received a $30 gift card for their participation in the interviews. They ranged in age from 31 to 73.
As previously described (33), participants noted potential value in a diabetes self-management intervention that employed some combination of DMA and live peer support. Contrary to possible expectations that individuals might object to having their choices and activities guided by an automated or artificial system, no one expressed such objections to using the DMA. Clinical staff saw value in the proposed intervention but expressed concerns about the potential of lay health coaches (Coaches) promoting inaccurate medical information and the lack of coordination with clinical care. The concerns of clinical staff were addressed by employing a certified diabetes educator to assist in training the Coaches, conducting weekly supervisory meetings with the entire study team, and coordinating the activity of Coaches through the nurse care coordinator (JD).
Following the formative evaluation, the study team developed the intervention according to the following principles: (1) the DMA would assist individuals with daily self-management and provide regular encouragement and praise, (2) the Coaches would work with participants to provide social support and assist with problem-solving and overcoming challenges, (3) the Coaches would also encourage participants to utilize a variety of DMA features, (4) participants were free to determine the extent to which they utilized health coaching and the DMA, and (5) data entered by participants into the DMA would prompt timely follow-up from the coaches.
Recruitment and Training of Lay Health Coaches
Two lay health coaches (Coaches) were recruited through the department of nutrition at a local university. Both had bachelor’s degrees but had not been previously employed in healthcare. The Coaches were employed through UNC-CH with paid, part-time positions (15–20 h per week).
The initial two-day training (18h) was held onsite at Vanguard, covering basic diabetes education, self-management support, effective peer support, communication skills, the DMA, research ethics, coaching protocol, and study documentation. The training was led by a certified diabetes educator and nurse coordinator from Vanguard, a DMA trainer from WellDoc, and trainers from Peers for Progress. The Coaches each received a smartphone on a prepaid plan with a local number to carry out the intervention.
Participant Recruitment and DMA Onboarding
Vanguard identified 203 potential participants from four of its eight primary care sites according to the following criteria: T2DM, 30–75 years of age, and at least one A1c value ≥ 7.5% in the previous 12 months. Although identified by A1c ≥ 7.5%, individuals were contacted in descending order of A1c values in order to recruit a sample of greatest clinical need. During recruitment, Coaches confirmed that participants were fluent in English and had regular access to a web-enabled device (smartphone or computer).
Each potential participant received a mailing containing a signed invitation letter from their primary care provider, HIPAA and consent forms, and a stamped return envelope. Coaches commenced telephone recruitment 2 weeks after the mailings were sent out unless individuals had opted out. Coaches attempted a total of seven contacts, including voicemails and a personalized follow-up letter, before designating individuals unable to be reached. Upon successful contact, Coaches introduced the program, obtained verbal HIPAA authorization and consent, and conducted a health assessment. Participants were asked to return signed HIPAA and consent forms using the stamped return envelopes. Following successful recruitment, Coaches set up a second call to assist with DMA installation and registration with DMA customer care for further technical support.
Coach Intervention
Beginning with the third call, Coaches began to build rapport with the participants and initiated substantive discussions around self-management. They inquired about the participant’s general health status, answered questions about using the DMA, and assisted participants with entering data into the DMA. Coaches probed emotional status using items from the Diabetes Distress Scale (34, 35) and reviewed individuals’ diabetes self-management, such as their familiarity with A1c and fasting blood glucose measures, self-monitoring blood glucose, medication adherence, diet and exercise, and adherence to recommended clinic visits and examinations. If neither the participant nor DMA data suggested a self-management target, Coaches reviewed the ADCES7 Self-Care Behaviors™ with participants to help set goals. Coaches encouraged the participant to attend diabetes self-management education if they had not already done so, and checked that the participant had a glucometer, testing strips, and prescribed medications.
Frequency of ongoing coaching calls ranged from biweekly to monthly according to participants’ needs and preferences. Each call began with follow-up on matters raised in the previous call as Coaches tailored discussions to individual participants. A protocol guided notification of the nurse coordinator in response to urgent medical issues or emotional distress. The intervention lasted up to 6 months, with individuals participating for 5–6 months depending on latency of enrollment.
The Coaches conducted their telephone calls from the care coordination office at Vanguard. This colocation facilitated back-up and coordination with the clinical team. Additionally, Vanguard, WellDoc, and UNC-CH staff held weekly calls with Coaches to address emergent issues, hot cases, and questions around protocols. This process led to improvements such as streamlined DMA onboarding, better incorporation of diabetes care standards, development of protocols for addressing urgent needs and strategies for motivating participants after the New Year, and guidance on generating discussions around participants’ own DMA data.
Fidelity of Implementation
Fidelity of implementation was monitored and encouraged in several ways. As noted above, staff (JD, EF, MP, and PT) met weekly with the Coaches to review progress and discuss any adjustments to the protocol as well as emergent clinical concerns. Coaches followed detailed protocols and scripts for initial contact of potential participants including, e.g., number and timing of recruitment calls. As noted in Results, Coaches succeeded in onboarding to the DMA 38 of the 43 participants for whom this was intended (88.4%). Per protocol, however, contacts with the Coaches and DMA entries were left free to vary in order to assess participants’ utilization of these. Project staff monitored coaches’ timely submission of contact notes.
Evaluation
Evaluation drew from established approaches to implementation and dissemination research including Glasgow’s RE-AIM model, Proctor’s implementation model, and the PRISM model (28–30). It also followed a triangulation design (36) that combined several investigators and interviewers (investigator triangulation) as well as both qualitative and quantitative data (methodological triangulation) (37) to address research questions comprehensively and to validate findings generated by each approach.
Qualitative Data Collection and Analysis
After the conclusion of the intervention, semi-structured interviews were conducted with 12 participants, 2 Coaches, and 5 project staff from UNC-CH, Vanguard, and WellDoc. Twelve participants, representing different patterns of participation in the program (both coaching and DMA, DMA with little coaching, and coaching with little DMA use), were recruited to participate in these interviews through their Coaches. Vanguard patients each received a $30 gift card for participating in the interviews.
UNC-CH graduate research assistants conducted the interviews by phone or in-person. Interviews probed strengths and challenges of the program, and solicited recommendations for dissemination, scale-up, and tailoring (28, 38). Interviews lasted 20–70 min, and were audiotaped, transcribed, and imported into Atlas.ti for analysis. Interviews were coded by three graduate research assistants according to deductive pre-identified codes and inductive emergent themes utilizing standard procedures for qualitative analysis (39).
Quantitative Data and Analysis
Baseline data included age, whether prescribed insulin, most recent A1c, blood pressure, and lipid values from EMR up to 10 months prior to initiation of the study. End-of-treatment values were the last available, up to 1 month following intervention termination. Data from DMA included blood glucose readings, carbohydrate counts, medication adherence, hypoglycemic events, and free text notes/diaries. Coaches’ records included dates of contacts and topics discussed.
Statistical methods used to describe the sample, patterns of use, and value added included descriptive statistics, and t-tests to assess differences between those who participated and those who were eligible but did not participate. Other differences are detailed in the Results, such as participation by sex. Principal components analysis examined the factorial structure among the number of different types of entries to the DMA. Analyses also included correlations among participation variables and between those variables and clinical indicators, and within-group t-tests of changes in clinical indicators.
Results
Following methodological triangulation’s (36) inclusion of both qualitative and quantitative data (37), the quantitative and qualitative data are integrated in answering each of the research questions. Table 1 includes illustrative quotations from the interviews, organized according to the key sections of the Results: (a) patterns of use, (b) the value added by the coach and DMA support, (c) the complementarity of coach support and DMA, and (d) sustainability within the clinical setting. Each section of the Results draws also from the interview results, including illustrative quotations from Table 1, indicated as from participants—“PQ”; Coaches—“CQ”; or staff—“SQ” and numbered consecutively.
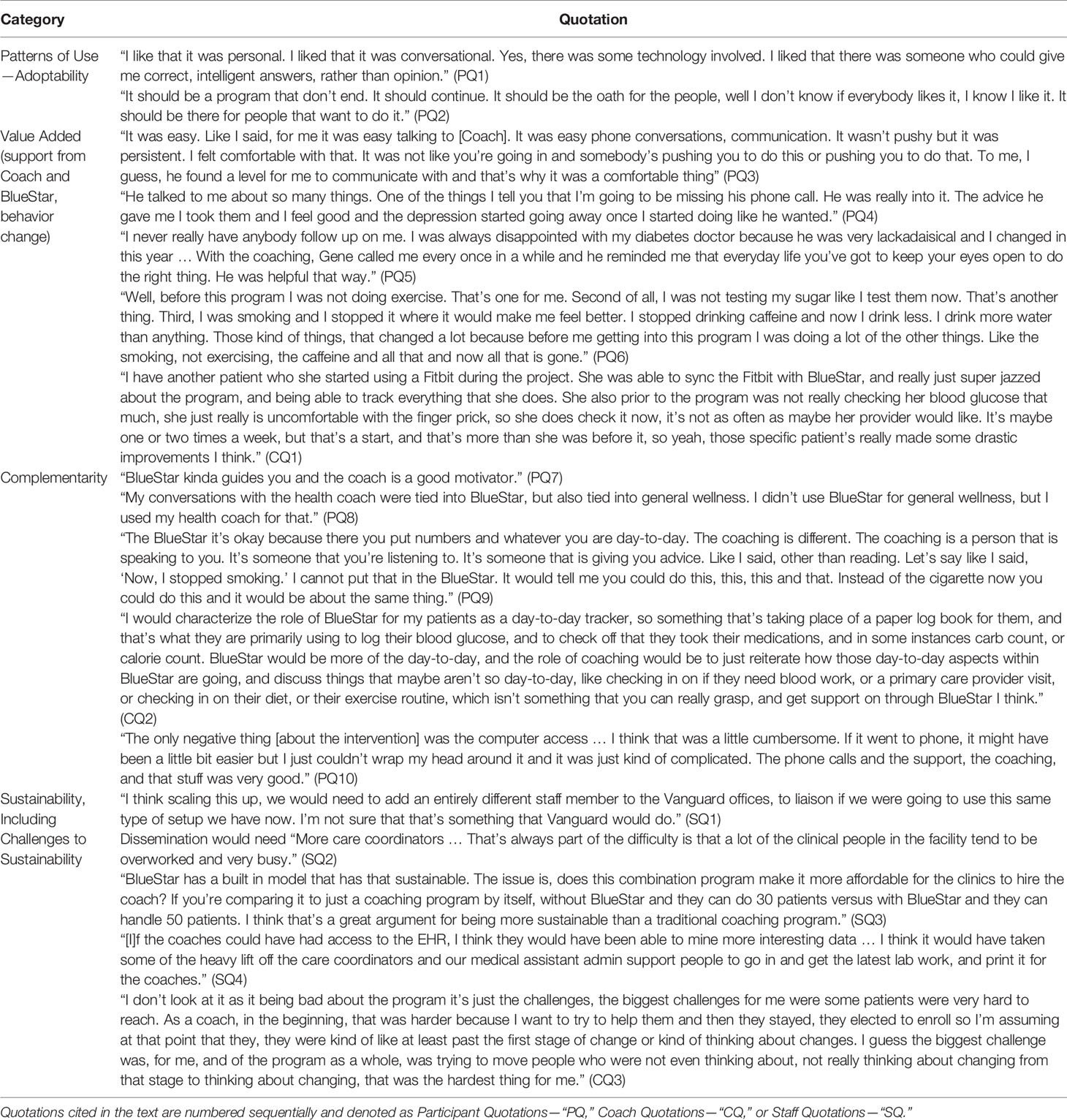
Table 1 Quotations illustrating patterns of use and adoptability, value added, complementarity, and sustainability challenges from participants, coaches, and staff.
Recruitment and Sample Characteristics
Of the 203 potential participants identified from EMR data, 140 were contacted for participation, of whom 46 were found to be ineligible. Of the 94 eligible, 47 declined and 47 (50%) consented to participate. This is a common rate of acceptance. For example, recruitment was 51.8% (240 of 463) in a pragmatic trial of the same DMA as used here that was also restricted to those with A1c levels at or above 8% and that included in-person identification by clinicians during regular clinic visits (40).
It should also be noted that individuals were approached for participation starting with those with the highest A1c values. Relative to the baseline A1c of 8.96 in the aforementioned study (40), this resulted in mean baseline A1c of 9.93% in each of the current groups that participated and that declined participation.
The study objective was to examine use of and benefits from the availability of both live coaching and DMA. Availability was operationalized as minimal exposure to each, leaving unconstrained variation in subsequent use. Minimal exposure was defined as two coach contacts in the second of which DMA onboarding was planned. Of the 47 who consented to participate, 43 (91.5%) met this criterion of two coach contacts, among whom 38 (88.4%) enrolled in DMA.
Table 2 compares pre-intervention values for these 43 participants with those of 114 from among the 203 identified from EMR as eligible but excluding the 46 confirmed to be ineligible during initial recruitment. Thus, the 114 to whom the 43 participants are compared includes four who agreed to participate but did not meet the criterion of two coach contacts, 47 who were reached but declined, and 63 who were not reached, some of whom might have been found ineligible had they been reached. There were no appreciable differences between the groups (ps for t-tests ranged from 0.365–0.993).
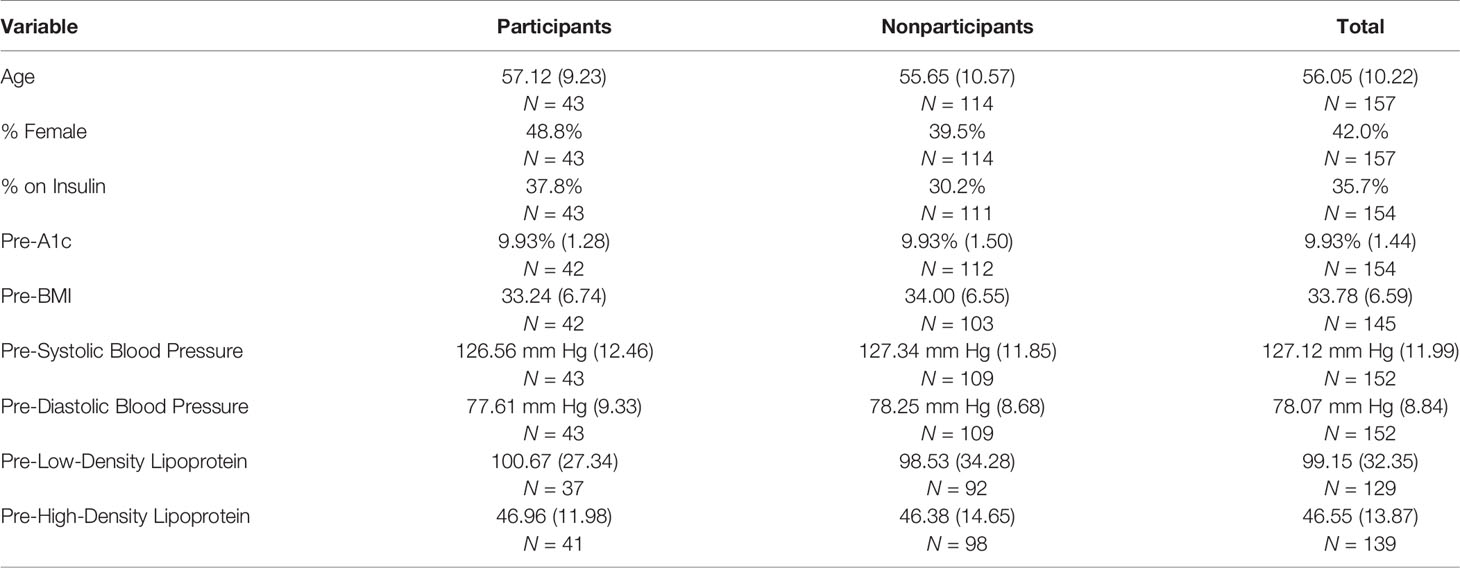
Table 2 Comparisons of participants and nonparticipants: percentages or means (standard deviations) on key variables pre-intervention.
Participants’ healthcare was covered by commercial insurance or Medicare. From zip codes of participants, estimated per-capita income was $42,126. In the third quarter of 2015 when participants were recruited, the per-capita income in New Jersey was $61,136 (41), 45.1% or almost $20,000 higher than that of participants.
Patterns of Use
Among the 43 participants, coach contacts and DMA entries are described in Table 3. Coach contacts ranged from 1 to 16 with an average of 5.86. Of the 38 who enrolled in DMA, 29% utilized the DMA with smartphone only, 29% with computer only, and 42% with both. Six participants (16%) entered at least one hypoglycemic value (BG < 70 mg/dl) and 8 (21.1%) entered at least one hyperglycemic value (>300 mg/dl), both of which triggered real-time feedback within the DMA. Fourteen participants (36.8%) transmitted at least one “SMART Visit Report” (integrated DMA data including glucose control and self-management behaviors) to their Vanguard PCP for review at their office visit.
Ninety-three percent of coach contacts were initiated by the Coaches. Their contact notes provided data on the topics of contacts, including the percentage of contacts that included discussion of each of the ADCES7 Self-Care Behaviors™ (1). These were healthy eating—65.6% of contacts, physical activity—58.2% of contacts, glucose monitoring—67.7%, medication adherence—69.5%, reducing risks—62.8%, problem solving—65.3%, and healthy coping—63.5% of contacts. Turning to types of coaching support, the percentages of calls in which each of the following types of support were provided were as follows: emotional support—64.9%, encouragement or motivational support—82.1%, problem solving—42.5%, new goal(s) set—31.2%, and review of goal progress—46.7%. Support for PCP visits was included in 24.6% of contacts and miscellaneous topics (economic, legal, social, and health services) in 5.3%.
Table 3 also provides the ranges and means of blood glucose, carbohydrate, and medication entries and numbers of notes into DMA. Principal components analysis indicated that they were best characterized as a single factor (Eigen value = 2.792, loadings ranging from .707 to .926). Consequently, these four types of entries were summed to create “total entries” that ranged from 0 to 1,443 with a mean of 144.140 (SD = 270.114).
There were no significant relationships between participant characteristics (age, baseline SBP, DBP, and A1c) and number of either DMA entries or coach contacts (ps range from 0.372 to 0.993). The 13 participants with prescriptions for insulin averaged twice as many total entries into DMA compared to the 30 without insulin prescriptions, 225.23 vs. 110.43, but this was not significant (p = 0.204) because of large variability (SD = 270.11). Similarly, those with insulin prescriptions averaged 6.846 coach contacts versus 5.433 for those without insulin prescriptions, but this also was not significant (p = 0.161). There were no significant differences by sex in DMA entries (p = 0.789) or coach contacts (p = 0.624).
Participants, Coaches, and study staff spoke positively about the program. Participants mentioned the flexibility and user-friendliness of DMA and that there was live coaching from “someone who could give me correct, intelligent answers” (PQ1) and of the value of ongoing support, “It should be a program that don’t end” (PQ2).
Value Added
Among the 43 participants, pre- and post-A1c values were missing for one participant and post values were missing for an additional four. For these four, post values were conservatively imputed as equal to pre values, resulting in an analytic sample of 42. A1c declined from 9.93% (SD = 1.28), reflecting the selection of participants on the basis of high A1c scores, to 8.86% (SD = 1.84) after the end of the coach intervention (t = 4.09, df = 41, p < 0.001). Among the 37 who both enrolled in DMA and had at least two coach contacts, the change was similar (9.99, SD = 1.32, to 8.80%, SD = 1.93, t = 4.21, df = 36, p < 0.001). Using categories from the National Committee for Quality Assurance (42), the percentage with A1c below 9% increased from 27.9 to 55.8% and that below 8% increased from 0 to 33.3%.
We divided the 43 participants at/above or below the median for coach contacts (median = 6) and total DMA entries (median = 21). Table 4 shows that for each combination of high or low use of Coach and DMA, A1c values declined a minimum of 0.49 points (9.69% to 9.20%). Although not significant because of small sample sizes, the smallest reduction, 0.49 points, was among those relatively low on both DMA entries and coach contacts.
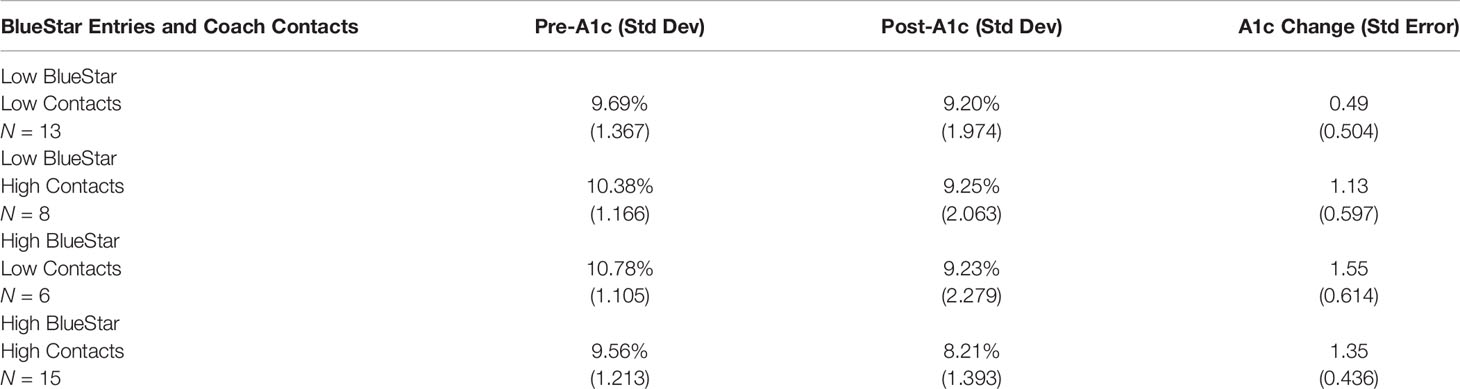
Table 4 A1c means and mean changes from pre- to post-assessment disaggregated by level of BlueStar entries (low/high) and numbers of coach contacts (low/high).
Participants cited the support, guidance, knowledge, and motivation received from their Coach and overall motivation that the program provided. When describing support received from the Coaches, participants described it as “wasn’t pushy but it was persistent” (PQ3), “someone who was there for me when I needed them,” and “friendly, comfortable, personalized” attention. They praised the attention and engagement of the Coaches, “he was really into it” (PQ4), and mentioned how the Coaches made it easy to talk about diabetes, and could help them identify what issues they needed to discuss with a healthcare professional. Participants also spoke about their Coach remaining sensitive to their situations. For instance, participants noted that Coaches were able to adjust recommendations in the face of obstacles such as in the case of a toe complication that made it difficult to walk.
Participants noted the importance of follow-up, “I never really have anybody follow up on me. I was always disappointed with my diabetes doctor because he was very lackadaisical” (PQ5) and expressed disappointment that their healthcare providers did not review their data contained in DMA Smart Visit Reports, thereby underscoring the need to encourage clinicians to utilize DMA generated data.
As also noted in Table 1, participants mentioned specific behavioral changes made through being in the program (PQ6, CQ1). These fell into three categories: improving general health behaviors (e.g., quitting smoking), monitoring data (e.g., blood glucose values), and medication adherence. When explaining these behavioral changes, participants indicated that the DMA and/or the Coach helped them follow their self-management regimen.
Complementarity
Overall, participants tended to use both DMA and Coaches as indicated by the correlation, r = .613 (p < 0.001) between DMA entries and coach contacts. However, dividing the 43 participants into those above/below the median for DMA entries and coach contacts identified several different patterns. Fourteen favored one or the other but not both of coaches or DMA (8 high coach/low DMA, 6 high DMA/low coach). Complementarity, tending to use or not use both if using or not using either, was indicated by 16 being high on both (including one missing both pre- and post-A1c values and therefore not included in Table 4) and, also, by 13 low on both.
All 38 participants enrolled in DMA had at least some coach contacts because of the introduction of DMA through the Coaches. Among three participants with two or fewer contacts, total DMA entries averaged 6, far below the average across all 38 DMA users of 144.14. Among four who enrolled in DMA but never entered data into it, total coach contacts averaged 3.75 with none reaching the average of 5.86 among the other 34 DMA enrollees. Thus, those with very low engagement in one of Coach or DMA tended to be low in use of the other.
Interviews also indicated complementarity of coaching and DMA: DMA “kinda guides you and the coach is a good motivator” (PQ7), “I didn’t use [DMA] for general wellness, but I used my health coach for that” (PQ8), “it’s okay [DMA] because there you put numbers and whatever you are day-to-day. The coaching is different … It’s someone that you’re listening to” (PQ9), DMA “would be more of the day-to-day, and the role of the coaching would be … discuss things that maybe aren’t so day-to-day” (CQ2). However, as 14 participants favored one of coach or DMA over the other, participants’ comments also reflected preference for one or the other as with “The only negative thing was the computer access … I think that was a little cumbersome … I just couldn’t wrap my head around it … The phone calls and the support, the coaching, and that stuff was very good” (PQ10).
DMA data reports allowed Coaches to identify participants’ self-management challenges and provided a basis to initiate conversations about those issues. Coaches noted that they tailored recommendations to participants based on data from the DMA and their conversations. Participants mentioned how Coaches helped them make sense of trends in their blood glucose and in their medication adherence, as displayed in the DMA. Furthermore, participants reported sharing reports with their Coach and discussing recipes suggested by DMA.
These quantitative and qualitative findings support the complementarity of the DMA and coaching in that use of one tended to vary in parallel with use of the other, that features of one tended to “go well with” features of the other, that they tended to go together. An alternative explanation is that individual differences such as in motivation may lead some people to do more or less of both coach contacts and DMA entries, accounting for their correlation.
Sustainability
A major challenge for sustainability identified was the importance of the nurse coordinator or other staff to coordinate Coaches and serve as liaison to the clinical team (SQ1,2). Clearly, coaching and DMA are not self-implementing. However, one project staff member noted potential efficiency with the DMA that “has a built-in model that is sustainable.” She noted that, if Coaches could serve, e.g., 30 participants without but 50 with the DMA, “I think that’s a great argument for being more sustainable than a traditional coaching program” (SQ3). Staff also noted that efficiency would be enhanced and the burden on the care coordinator would be reduced if Coaches had direct access to EHR data (SQ4). Additional observations regarding sustainability included (a) potential to enhance integrated, value-based care with less siloing; (b) need for protocols to hire, support, and integrate Coaches into primary care practice; (c) hiring Coaches with more clinical or technical knowledge; and (d) a Coach’s observation that patient motivation remained a challenge, “trying to move people who were … not really thinking about changing from that stage to thinking about changing” (CQ3).
Discussion
Integration of telephone-based lay health coaching with a DMA in a primary care setting was able to be implemented, accepted by individuals with T2DM, and, in uncontrolled analyses, associated with reductions in A1c. Forty-seven (50%) of 94 eligible individuals agreed to participate in the study, of whom 43 participated at least minimally. Utilization of both live coaching and DMA was frequent and positively associated, suggesting the complementarity of live and automated support—”soft touch” and “high tech.” With all participants initially selected on the basis of elevated A1c and using National Committee for Quality Assurance (42) criteria, the percentage of participants with A1c below 9% increased from 27.9 to 55.8% and below 8% from 0 to 33.3%. Additionally, those electing to participate were almost equally divided by gender (48.8% female).
In post-intervention interviews, participants expressed that they accepted the interface between participant, coach, and DMA. They expected Coaches to have access to their DMA data and use those data as part of the coaching, viewing this as a strength of the intervention. No participant mentioned feeling surveilled or disliking the monitoring by DMA. Instead, several reported liking the accountability from entering blood glucose levels in the DMA and Coaches having access to the data. As one participant put it, “… it was like someone was watching over me or I was answerable to someone. That helped me in putting in the numbers.” These comments mirror those of users of an automated telephone support intervention for diabetes management developed by Oldenburg and his colleagues (43) in Australia: “It is good to know ‘someone’ is keeping an eye (on my management).” (44)
In order to facilitate speedy training and initiation of the intervention, this study recruited lay health coaches that had prior education in promoting healthy lifestyles, behavior change, and managing chronic diseases. However, research on peer support indicates that even nonprofessionals with simple training can be effective in supporting diabetes management (5, 45). It is important to note that clinical staff perceived the Coaches having completed bachelors’ training and being enrolled in graduate nutrition programs as a strength of the intervention, linked to Coaches’ ability to be quickly deployed and capable in working with clinical staff. Though there are operational considerations that favor the recruitment of Coaches that are younger, have higher levels of formal education, and perceived to be more facile with digital health technologies, future research should explore the feasibility of using different lay health workers across the continuum of peer support (46, 47), from part-time volunteers to state-credentialed Community Health Workers.
The task of incorporating health coaches into routine clinical care may pose challenges to healthcare organizations that do not follow the patient-centered medical home model. In this study, an experienced nurse care coordinator served as bridge and coordinator among participants, Coaches, and the clinical team. This role could be standardized so that nurse care coordinators or other care managers might have the capacity to coordinate several Coaches (48–50).
The complementarity of peer support and digital health reflects a 2017 systematic review of “technology-enabled diabetes self-management” that found that the most effective interventions (a) connected people with diabetes with their healthcare teams using two-way communication, (b) analyzed participant-generated health data, (c) tailored education, and (d) individualized feedback (18). These features share much with five key functions of peer support, outlined in Table 5, that Peers for Progress has noted as a template for standardization and dissemination (45, 51, 52). Indeed, Table 5 documents how digital health and live peer support complement the contributions of each to these five key functions. The key functions then provide a template for self-management support through interpersonal or technological modalities or combinations of the two.
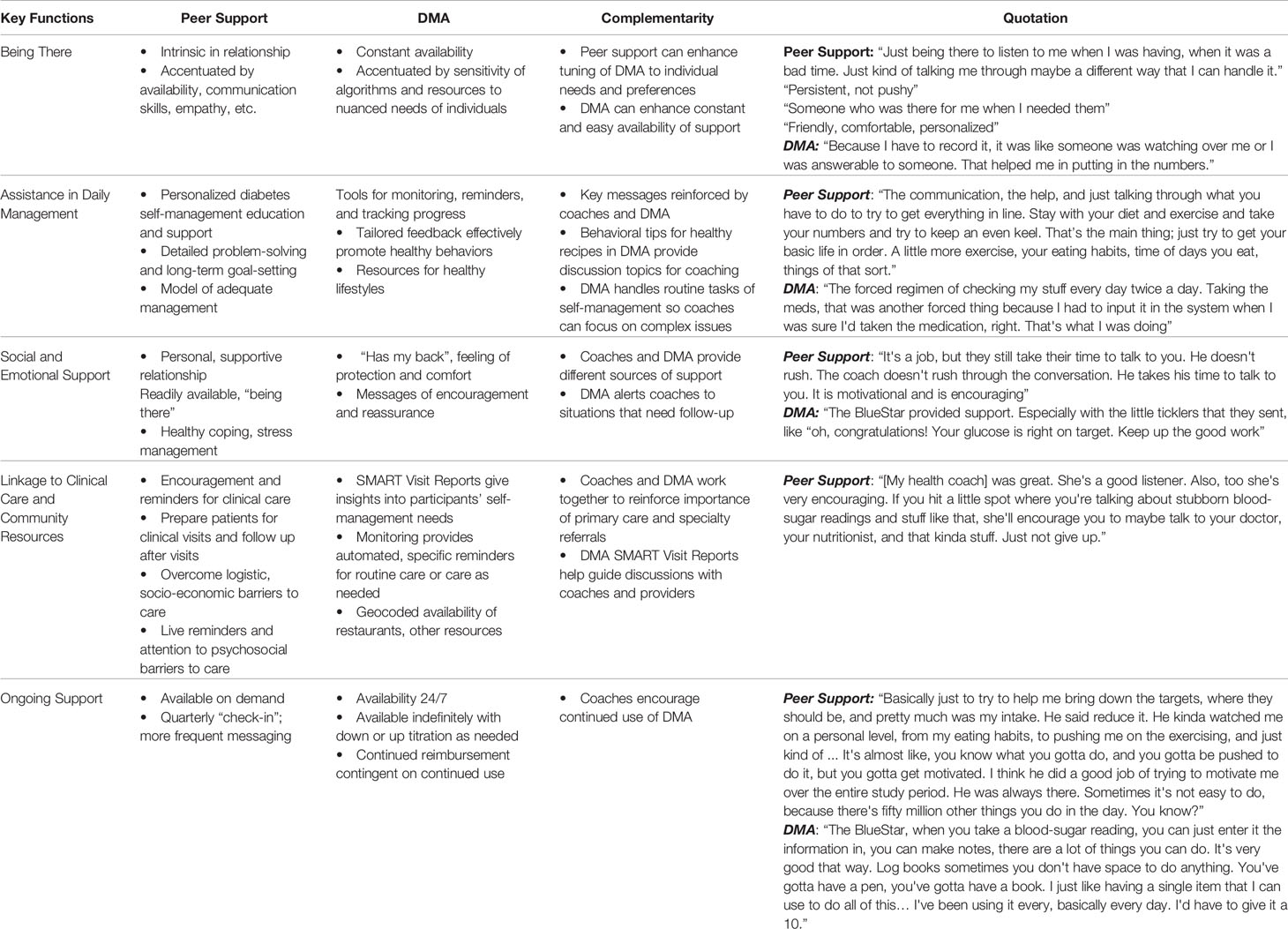
Table 5 Features of peer support and Diabetes Management App (DMA) corresponding to each of the five key functions of peer support, aspects of complementarity of peer support and DMA, and quotations from participants illustrating these features.
The present observations that participants saw the DMA and live coaching as fitting well together suggest that digital health apps may be considered a form of automated, resource-saving, low-cost support, ready to be paired with live peer support for those with greater need, e.g., suboptimal self-management, complex multi-morbidities, or psychosocial concerns. Extending such thinking beyond the details of this study, population management might titrate app use and live coaching (53). As an example of this kind or approach, a simple model might include Level One: Doing well (e.g., A1c < 7%, no psychosocial concerns)—Routine availability of app; Level Two: Little clinical or psychosocial concern (e.g., A1c < 7.5%, no pronounced psychosocial concerns)—Routine encouragement of app through primary care visits; Level Three: Moderate clinical concern (e.g., A1c > 7.5%)—Limited coach contacts to support self-management and encourage use of app; and Level Four: Substantial clinical concern (e.g., A1c > 8% and/or appreciable psychosocial distress)—Live coaching for 6 months, renewable as needed, with incorporation of app as acceptable or helpful. Although they surely do not provide adequate data for concluding that such a model would be effective, the present study results suggest that this type of titration of interventions may be realistic and worthy of future research.
Digital health is “what’s coming” across a range of functions and services. With mobile health apps available not only for wellness support but also for most common conditions, there are solutions available to support each step of the journey of those with diabetes on devices that people already own. Experts have noted the remarkable market-driven growth of mobile health apps for diabetes (54, 55). People with diabetes, clinicians, investors, and tech developers all seem to recognize the promise of digital health in diabetes care, and regulators are encouraging growth in this field by lowering barriers to accessing new technologies (56).
The issue is not if but how to deliver digital health to individuals and integrate it with clinical care. Health coaches may be a valuable strategy in rolling out digital health to patient populations. From the perspective of digital health, an important contribution of health coaching is how it promoted the uptake and continued use of DMA. From the perspective of the health coaches, DMA provided a focal point for participant monitoring and generating discussion, which helped to shape participant encounters and pinpoint key areas of improvement for each individual participant. In addition to more rigorous, controlled tests of efficacy and effectiveness, follow-up studies might examine how digital health solutions impact the ways in which Coaches deliver diabetes support, such as by comparing how Coaches adjust their counseling strategies when assisted by digital health solutions versus when using traditional strategies. In addition, future developments might involve adding in-person coaching, in either individual or group formats.
Limitations
In this study, participants were encouraged to engage with service offerings as they wished, similar to the way in which comprehensive chronic disease programs might be implemented under real-world conditions. One limitation of the study is the selection bias for participants who are interested in digital health and have the means and skills to use such tools.
The intervention was planned to meet the needs of those having trouble managing their diabetes (mean baseline A1c = 9.93%) and the sample had, on average, incomes estimated at 31.1% below the statewide average. However, the intervention was not tailored to any specific racial or ethnic group, perhaps limiting generality to some groups with disproportionate diabetes burden. At the time of this study, the DMA was only available in English, which excluded individuals not fluent in English.
Other limitations of the project include the relatively small sample size and the lack of controls. Additionally, participant data were drawn from EMR records as part of routine care instead of being gathered as part of an independent collection of evaluation data.
Conclusions
Contributing to the growing research around digital health for diabetes management, this study shows that integrated health coaching/DMA is feasible and acceptable for individuals with diabetes, coaches, and clinicians. The findings suggest that these two approaches complement each other, meriting additional studies to test integrated models at scale and address implementation challenges for clinical and organizational adoption.
Data Availability Statement
The datasets presented in this article are not readily available because of the modest size of the sample, and because the data consist largely of clinical data, we would anticipate problems in assuring confidentiality of participants’ data and, so, have decided not to make the data available. Questions about details of the data may be directed to PT (cHRhbmdAdW5jLmVkdQ==) or EF (edfisher@unc.edu). Requests to access the datasets should be directed to PT (cHRhbmdAdW5jLmVkdQ==) and EF (ZWRmaXNoZXJAdW5jLmVkdQ==).
Ethics Statement
The studies involving human participants were reviewed and approved by the Institutional Review Board at the University of North Carolina at Chapel Hill. The patients/participants provided their written informed consent to participate in this study.
Author Contributions
PT, JD, MP, and EF led the development, implementation, and evaluation of the project and the preparation of the manuscript. SK, NB, and RS contributed to planning the implementation and evaluation of the project, and contributed to conducting evaluation including data management, data analysis, and coding and interpretation of interviews. All authors contributed to the article and approved the submitted version.
Funding
Funding for this project was provided by a Gillings Innovation Laboratory award funded by the 2007 Gillings Gift to UNC-Chapel Hill’s Gillings School of Global Public Health. The funding source had no role in the study design; data collection; administration of the interventions; analysis, interpretation, reporting of data; or decision to submit the findings for publication.
Conflict of Interest
Subsequent to completion of this study, EF and PT became part of a contract with WellDoc that provided BlueStar® Diabetes for the present study. The contract was for development of programs in areas other than diabetes. MP is an employee of WellDoc that provided the BlueStar® Diabetes for the study. She collaborated throughout planning, implementation, and preparation of this manuscript, and specifically contributed to the description of BlueStar® Diabetes and product user data.
The remaining authors declare that the research was conducted in the absence of any commercial or financial relationships that could be construed as a potential conflict of interest.
Publisher’s Note
All claims expressed in this article are solely those of the authors and do not necessarily represent those of their affiliated organizations, or those of the publisher, the editors and the reviewers. Any product that may be evaluated in this article, or claim that may be made by its manufacturer, is not guaranteed or endorsed by the publisher.
Acknowledgments
Emily Berlin and Eugene Arnold served with great thoughtfulness and dedication as Coaches for the project. Nicole Gyamfi at Vanguard Medical Group was extremely helpful in recruitment and early stages of the intervention. Thomas McCarrick, Medical Director of Vanguard, provided valuable support and guidance for the overall project. At WellDoc, Janice MacLeod and Ashley Naumann provided extensive assistance in participants’ access to BlueStar® and in extracting data from participants’ use of the DMA. Steven Peskin of Horizon Blue Cross Blue Shield of New Jersey helped bring together Vanguard, WellDoc, and Peers for Progress and encouraged the project throughout. The strong partnership among Vanguard, WellDoc, and UNC-CH Peers for Progress deserves attention as an unusual collaboration among the worlds of academic research, clinical care, commercial health technology. The content is solely the responsibility of the authors. The project was also assisted by the UNC-Michigan Peer Support Core of the Michigan Center for Diabetes Translational Research, P30 DK092926, William Herman, PI.
References
1. Association of Diabetes Care and Education Specialists, Kolb L. An Effective Model of Diabetes Care and Education: The ADCES7 Self-Care Behaviors™. Sci Diabetes Self-Manag Care (2021) 47(1):30–53. doi: 10.1177/0145721720978154
2. Marrero DG, Ard J, Delamater AM, Peragallo-Dittko V, Mayer-Davis EJ, Nwankwo R, et al. Twenty-First Century Behavioral Medicine: A Context for Empowering Clinicians and Patients With Diabetes: A Consensus Report. Diabetes Care (2013) 36(2):463–70. doi: 10.2337/dc12-2305
3. Fisher EB, Boothroyd RI, Elstad EA, Hays L, Henes A, Maslow GR, et al. Peer Support of Complex Health Behaviors in Prevention and Disease Management With Special Reference to Diabetes: Systematic Reviews. Clin Diabetes Endocrinol (2017) 3:4. doi: 10.1186/s40842-017-0042-3
4. Fisher EB, Ballesteros J, Bhushan N, Coufal MM, Kowitt SD, McDonough AM, et al. Key Features of Peer Support in Chronic Disease Prevention and Management. Health Aff (Millwood) (2015) 34(9):1523–30. doi: 10.1377/hlthaff.2015.0365
5. Fisher EB, Coufal MM, Parada H, Robinette JB, Tang PY, Urlaub DM, et al. Peer Support in Health Care and Prevention: Cultural, Organizational and Dissemination Issues. In: Fielding J, Brownson RC, Green L, editors. Annual Review of Public Health, vol. 35 . Palo Alto: Annual Reviews (2014). p. 363–83.
6. Trump LJ, Mendenhall TJ. Community Health Workers in Diabetes Care: A Systematic Review of Randomized Controlled Trials. Families Syst Health: J Collab Family Healthcare (2017) 35(3):320–40. doi: 10.1037/fsh0000283
7. Kim K, Choi JS, Choi E, Nieman CL, Joo JH, Lin FR, et al. Effects of Community-Based Health Worker Interventions to Improve Chronic Disease Management and Care Among Vulnerable Populations: A Systematic Review. Am J Public Health (2016) 106(4):e3–e28. doi: 10.2105/AJPH.2015.302987
8. Litchman ML, Oser TK, Hodgson L, Heyman M, Walker HR, Deroze P, et al. In-Person and Technology-Mediated Peer Support in Diabetes Care: A Systematic Review of Reviews and Gap Analysis. Diabetes Educ (2020) 46(3):230–41. doi: 10.1177/0145721720913275
9. Golden SH, Maruthur N, Mathioudakis N, Spanakis E, Rubin D, Zilbermint M, et al. The Case for Diabetes Population Health Improvement: Evidence-Based Programming for Population Outcomes in Diabetes. Curr Diabetes Rep (2017) 17(7):51. doi: 10.1007/s11892-017-0875-2
10. Jack HE, Arabadjis SD, Sun L, Sullivan EE, Phillips RS. Impact of Community Health Workers on Use of Healthcare Services in the United States: A Systematic Review. J Gen Intern Med (2017) 32(3):325–44. doi: 10.1007/s11606-016-3922-9
11. Michaelides A, Major J, Pienkosz E Jr., Wood M, Kim Y, Toro-Ramos T. Usefulness of a Novel Mobile Diabetes Prevention Program Delivery Platform With Human Coaching: 65-Week Observational Follow-Up. JMIR Mhealth Uhealth (2018) 6(5):e93. doi: 10.2196/mhealth.9161
12. Joiner KL, Nam S, Whittemore R. Lifestyle Interventions Based on the Diabetes Prevention Program Delivered via Ehealth: A Systematic Review and Meta-Analysis. Prev Med (2017) 100:194–207. doi: 10.1016/j.ypmed.2017.04.033
13. Kirley K, Sachdev N. Digital Health-Supported Lifestyle Change Programs to Prevent Type 2 Diabetes. Diabetes Spectr (2018) 31(4):303–9. doi: 10.2337/ds18-0019
14. Lunde P, Nilsson BB, Bergland A, Kvaerner KJ, Bye A. The Effectiveness of Smartphone Apps for Lifestyle Improvement in Noncommunicable Diseases: Systematic Review and Meta-Analyses. J Med Internet Res (2018) 20(5):e162. doi: 10.2196/jmir.9751
15. Cui M, Wu X, Mao J, Wang X, Nie M. T2DM Self-Management via Smartphone Applications: A Systematic Review and Meta-Analysis. PLoS One (2016) 11(11):e0166718. doi: 10.1371/journal.pone.0166718
16. Eberle C, Lohnert M, Stichling S. Effectiveness of Disease-Specific Mhealth Apps in Patients With Diabetes Mellitus: Scoping Review. JMIR Mhealth Uhealth (2021) 9(2):e23477. doi: 10.2196/23477
17. Shan R, Sarkar S, Martin SS. Digital Health Technology and Mobile Devices for the Management of Diabetes Mellitus: State of the Art. Diabetologia (2019) 62(6):877–87. doi: 10.1007/s00125-019-4864-7
18. Greenwood DA, Gee PM, Fatkin KJ, Peeples M. A Systematic Review of Reviews Evaluating Technology-Enabled Diabetes Self-Management Education and Support. J Diabetes Sci Technol (2017) 11(5):1015–27. doi: 10.1177/1932296817713506
19. Levine BJ, Close KL, Gabbay RA. Reviewing U.s. Connected Diabetes Care: The Newest Member of the Team. Diabetes Technol Ther (2020) 22(1):1–9. doi: 10.1089/dia.2019.0273
20. Cotter AP, Durant N, Agne AA, Cherrington AL. Internet Interventions to Support Lifestyle Modification for Diabetes Management: A Systematic Review of the Evidence. J Diabetes Complications (2014) 28(2):243–51. doi: 10.1016/j.jdiacomp.2013.07.003
21. Lie SS, Karlsen B, Oord ER, Graue M, Oftedal B. Dropout From an Ehealth Intervention for Adults With Type 2 Diabetes: A Qualitative Study. J Med Internet Res (2017) 19(5):e187. doi: 10.2196/jmir.7479
22. Graffigna G, Barello S, Bonanomi A, Menichetti J. The Motivating Function of Healthcare Professional in Ehealth and Mhealth Interventions for Type 2 Diabetes Patients and the Mediating Role of Patient Engagement. J Diabetes Res (2016) 2016:2974521. doi: 10.1155/2016/2974521
23. Hou C, Xu Q, Diao S, Hewitt J, Li J, Carter B. Mobile Phone Applications and Self-Management of Diabetes: A Systematic Review With Meta-Analysis, Meta-Regression of 21 Randomized Trials and GRADE. Diabetes Obes Metab (2018) 20(8):2009–13. doi: 10.1111/dom.13307
24. Beck J, Greenwood DA, Blanton L, Bollinger ST, Butcher MK, Condon JE, et al. 2017 National Standards for Diabetes Self-Management Education and Support. Diabetes Care (2017) 40(10):1409–19. doi: 10.2337/dci17-0025
25. Introduction: Standards of Medical Care in Diabetes-2018. Diabetes Care (2018) 41(Suppl 1):S1–2. doi: 10.2337/dc18-Sint01
26. Quinn CC, Clough SS, Minor JM, Lender D, Okafor MC, Gruber-Baldini A. Welldoc Mobile Diabetes Management Randomized Controlled Trial: Change in Clinical and Behavioral Outcomes and Patient and Physician Satisfaction. Diabetes Technol Ther (2008) 10(3):160–8. doi: 10.1089/dia.2008.0283
27. Quinn CC, Shardell MD, Terrin ML, Barr EA, Ballew SH, Gruber-Baldini AL. Cluster-Randomized Trial of a Mobile Phone Personalized Behavioral Intervention for Blood Glucose Control. Diabetes Care (2011) 34(9):1934–42. doi: 10.2337/dc11-0366
28. Feldstein AC, Glasgow RE. A Practical, Robust Implementation and Sustainability Model (PRISM) for Integrating Research Findings Into Practice. Joint Commission J Qual Patient Safety/Joint Commission Resources (2008) 34(4):228–43. doi: 10.1016/S1553-7250(08)34030-6
29. Proctor EK, Landsverk J, Aarons G, Chambers D, Glisson C, Mittman B. Implementation Research in Mental Health Services: An Emerging Science With Conceptual, Methodological, and Training Challenges. Adm Policy Ment Health (2009) 36(1):24–34. doi: 10.1007/s10488-008-0197-4
30. Glasgow RE, Estabrooks PE. Pragmatic Applications of RE-AIM for Health Care Initiatives in Community and Clinical Settings. Prev Chronic Dis (2018) 15:E02. doi: 10.5888/pcd15.170271
31. Rachlin H, Green L, Vanderveldt A, Fisher EB. Behavioral Medicine’s Roots in Behaviorism: Concepts and Applications. In: Fisher EB, Cameron LD, Christensen AJ, Ehlert U, Guo Y, Oldenburg B, et al, editors. Principles and Concepts of Behavioral Medicine: A Global Handbook. New York: Springer (2018). p. 241–75.
32. Allegrante JP, Hyden C, Kristjansson AL. Research Approaches of Education, Applied Psychology, and Behavioral Science and Their Application to Behavioral Medicine. In: Fisher EB, Cameron LD, Christensen AJ, Ehlert U, Guo Y, Oldenburg B, et al, editors. Principles and Concepts 9 of Behavioral Medicine: A Global Handbook. New York: Springer (2018).
33. Kowitt SD, Tang PY, Peeples M, Duni J, Peskin S, Fisher EB. Combining the High Tech With the Soft Touch: Population Health Management Using Ehealth and Peer Support. Popul Health Manag (2016) 20(1):3–5. doi: 10.1089/pop.2016.0027
34. Peers for Progress. Consensus Evaluation for Research on Self Management and Peer Support in Diabetes. Leawood, Kansas: American Academy of Family Physicians Foundation (2010).
35. Fisher L, Glasgow RE, Mullan JT, Skaff MM, Polonsky WH. Development of a Brief Diabetes Distress Screening Instrument. Ann Family Med (2008) 6(3):246. doi: 10.1370/afm.842
36. Creswell JW, Plano Clark VL, Gutmann ML, Hanson WE. Advances in Mixed Methods Research Designs. In: Tashakkori A, Teddlie C, editors. Handbook of Mixed Methods in Social and Behavioral Research. Thousand Oaks, CA: SAGE (2003). p. 209–40.
37. Noble H, Heale R. Triangulation in Research, With Examples. Evid Based Nurs (2019) 22(3):67–8. doi: 10.1136/ebnurs-2019-103145
38. Shea CM, Jacobs SR, Esserman DA, Bruce K, Weiner BJ. Organizational Readiness for Implementing Change: A Psychometric Assessment of a New Measure. Implement Sci (2014) 9(1):7. doi: 10.1186/1748-5908-9-7
40. Agarwal P, Mukerji G, Desveaux L, Ivers NM, Bhattacharyya O, Hensel JM, et al. Mobile App for Improved Self-Management of Type 2 Diabetes: Multicenter Pragmatic Randomized Controlled Trial. JMIR Mhealth Uhealth (2019) 7(1):e10321. doi: 10.2196/10321
41. Bureau of Economic Analysis. Regional Data: GCP and Personal Income. Available at: https://apps.bea.gov/iTable/iTable.cfm?reqid=70&step=1#reqid=70&step=1 (Accessed May 17, 2021).
42. National Committee for Quality Assurance. Comprehensive Diabetes Care (Cdc). Available at: https://www.ncqa.org/hedis/measures/comprehensive-diabetes-care/ (Accessed October 29, 2018).
43. Williams ED, Bird D, Forbes AW, Russell A, Ash S, Friedman R, et al. Randomised Controlled Trial of an Automated, Interactive Telephone Intervention (TLC Diabetes) to Improve Type 2 Diabetes Management: Baseline Findings and Six-Month Outcomes. BMC Public Health (2012) 12:602. doi: 10.1186/1471-2458-12-602
44. Peers for Progress. Global Evidence for Peer Support: Humanizing Health Care. In: Report From an International Conference. Leawood, Kansas: Peers for Progress, American Academy of Family Physicians Foundation (2014).
45. Fisher EB, Bhushan N, Coufal MM, et al. Peer Support in Prevention, Chronic Disease Management, and Well Being. In: Fisher EB, Cameron LD, Christensen AJ, Ehlert U, Guo Y, Oldenburg B, et al, editors. Principles and Concepts of Behavioral Medicine: A Global Handbook. New York: Springer (2018).
46. Eng E, Parker E, Harlan C. Lay Health Advisor Intervention Strategies: A Continuum From Natural Helping to Paraprofessional Helping. Health Educ Behav (1997) 24(4):413–7. doi: 10.1177/109019819702400402
47. Center for Health Law and Policy Innovation at Harvard Law School, National Council of La Raza (NCLR), Peers for Progress. Recommended Model Guidelines for Credentialing Community Health Worker Programs and Community Health Workers. (2015). Available at: http://peersforprogress.org/wp-content/uploads/2020/07/200707-chw-credentialing-guidelines.pdf.
48. Peers for Progress, American Academy of Family Physicians Foundation, National Council of La Raza, Patient-Centered Primary Care Collaborative. Peer Support in the Patient-Centered Medical Home and Primary Care (2015). Available at: http://peersforprogress.org/wp-content/uploads/2015/05/150526-peer-support-in-the-pcmh-and-primary-care-report.pdf.
49. Daaleman TP, Fisher EB. Enriching Patient-Centered Medical Homes Through Peer Support. Ann Fam Med (2015) 13(Suppl 1):S73–78. doi: 10.1370/afm.1761
50. Fisher EB, Tang PY, Coufal M, Liu Y, Jia W. Peer Support. In: Dalleman T, Helton M, editors. Chronic Illness Care: Principles and Practice. New York: Springer (2018).
51. Fisher EB, Boothroyd RI, Coufal MM, Baumann LC, Mbanya JC, Rotheram-Borus MJ, et al. Peer Support for Self-Management of Diabetes Improved Outcomes in International Settings. Health Aff (Millwood) (2012) 31(1):130–9. doi: 10.1377/hlthaff.2011.0914
52. Evans M, Daaleman TP, Fisher EB. Peer Support for Chronic Medical Conditions. In: Avery J, editor. Peer Support in Medicine: A Quick Guide. New York: Springer (2020).
53. Desveaux L, Shaw J, Saragosa M, Soobiah C, Marani H, Hensel J, et al. A Mobile App to Improve Self-Management of Individuals With Type 2 Diabetes: Qualitative Realist Evaluation. J Med Internet Res (2018) 20(3):e81. doi: 10.2196/jmir.8712
54. Fleming GA, Petrie JR, Bergenstal RM, Holl RW, Peters AL, Heinemann L. Diabetes Digital App Technology: Benefits, Challenges, and Recommendations. A Consensus Report by the European Association for the Study of Diabetes (EASD) and the American Diabetes Association (ADA) Diabetes Technology Working Group. Diabetes Care (2020) 43(1):250–60. doi: 10.2337/dci19-0062
55. Kaufman N, Mel E. Using Digital Health Technology to Prevent and Treat Diabetes. Diabetes Technol Ther (2020) 22(S1):S63–78. doi: 10.1089/dia.2020.2505
Keywords: diabetes, digital health, peer support, coach, self-management, primary care, app, telephone
Citation: Tang PY, Duni J, Peeples MM, Kowitt SD, Bhushan NL, Sokol RL and Fisher EB (2021) Complementarity of Digital Health and Peer Support: “This Is What’s Coming”. Front. Clin. Diabetes Healthc. 2:646963. doi: 10.3389/fcdhc.2021.646963
Received: 28 December 2020; Accepted: 31 August 2021;
Published: 24 September 2021.
Edited by:
Heather L. Stuckey, The Pennsylvania State University (PSU), United StatesReviewed by:
Rebecca Upsher, King’s College London, United KingdomMark Peyrot, Loyola University Maryland, United States
Copyright © 2021 Tang, Duni, Peeples, Kowitt, Bhushan, Sokol and Fisher. This is an open-access article distributed under the terms of the Creative Commons Attribution License (CC BY). The use, distribution or reproduction in other forums is permitted, provided the original author(s) and the copyright owner(s) are credited and that the original publication in this journal is cited, in accordance with accepted academic practice. No use, distribution or reproduction is permitted which does not comply with these terms.
*Correspondence: Edwin B. Fisher, ZWRmaXNoZXJAdW5jLmVkdQ==