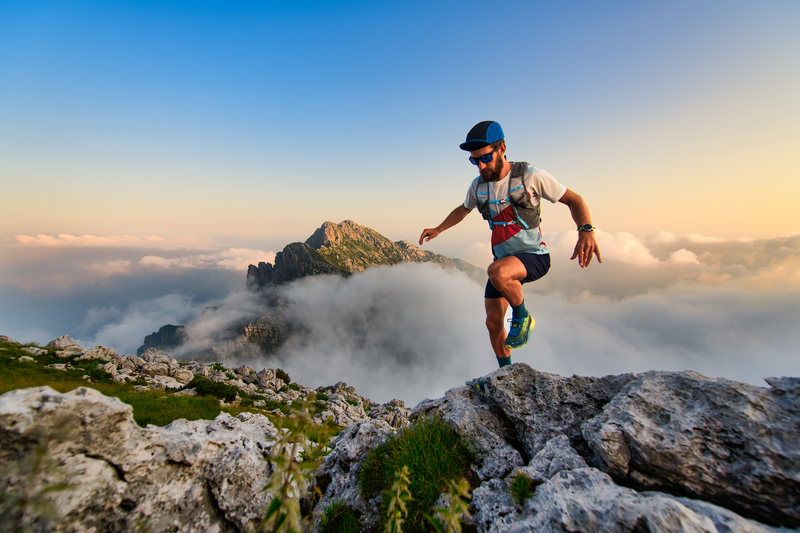
94% of researchers rate our articles as excellent or good
Learn more about the work of our research integrity team to safeguard the quality of each article we publish.
Find out more
ORIGINAL RESEARCH article
Front. Clim.
Sec. Climate Adaptation
Volume 7 - 2025 | doi: 10.3389/fclim.2025.1541228
This article is part of the Research Topic Addressing climate change in agriculture and natural resources with a focus on adaptation and extreme events View all articles
The final, formatted version of the article will be published soon.
You have multiple emails registered with Frontiers:
Please enter your email address:
If you already have an account, please login
You don't have a Frontiers account ? You can register here
Globally, climate change (CC) is a widely recognized fact, particularly in the past few decades. Developing nations like Ethiopia has been experiencing climate change and its effects on their agriculture. In this regard, urban agriculture is one of the sectors significantly impacted by adverse change in climate. However, there is scant empirical evidence on factors influencing urban farmers' intention to adapt to climate change in urban centers of Ethiopia. To fill the research gap, this study aimed at identifying factors influencing urban farmers' intention to adapt to climate change in Addis Ababa, Ethiopia. In doing so, a quantitative research approach with random sampling technique was applied. Based on the protection motivation theory (PMT), primary data were collected from 364 respondents using a survey questionnaire. Accordingly, a structural equation modeling (SEM) was used to identify and analyze the underlying relationships among nine constructs used in this study. Hence, the results revealed that selection of new crop varieties, water management practices, adjusting dates of sowing crops with changing local climatic conditions and sowing of drought-resistant cops were major adaptation practices. Hence, perception of CC indicators, the perceived effects of CC, incentives, subjective norms, adaptation efficacy, and self-efficacy were found positively and significantly influencing urban farmers' intention to adapt. In contrast, urban farmers (UFs) shows less intension to adapt to CC when subjected to maladaptive behaviors. Based on this, the study recommends strengthening the capacity of local institutions, farmer training and education, climate information dissemination such as early warning systems, access to extension services and access to finance to equip urban farmers to undertake practical adaptation strategies in the face of climate change.
Keywords: Climate Change, intention to adapt, Urban agriculture, Urban farmers, PMT, SEM
Received: 07 Dec 2024; Accepted: 03 Mar 2025.
Copyright: © 2025 Getu, Ayenew, Singh and Worku. This is an open-access article distributed under the terms of the Creative Commons Attribution License (CC BY). The use, distribution or reproduction in other forums is permitted, provided the original author(s) or licensor are credited and that the original publication in this journal is cited, in accordance with accepted academic practice. No use, distribution or reproduction is permitted which does not comply with these terms.
* Correspondence:
Mitiku Adisu Worku, Ethiopian Civil Service University, Addis Ababa, 5648, Addis Ababa, Ethiopia
Disclaimer: All claims expressed in this article are solely those of the authors and do not necessarily represent those of their affiliated organizations, or those of the publisher, the editors and the reviewers. Any product that may be evaluated in this article or claim that may be made by its manufacturer is not guaranteed or endorsed by the publisher.
Research integrity at Frontiers
Learn more about the work of our research integrity team to safeguard the quality of each article we publish.