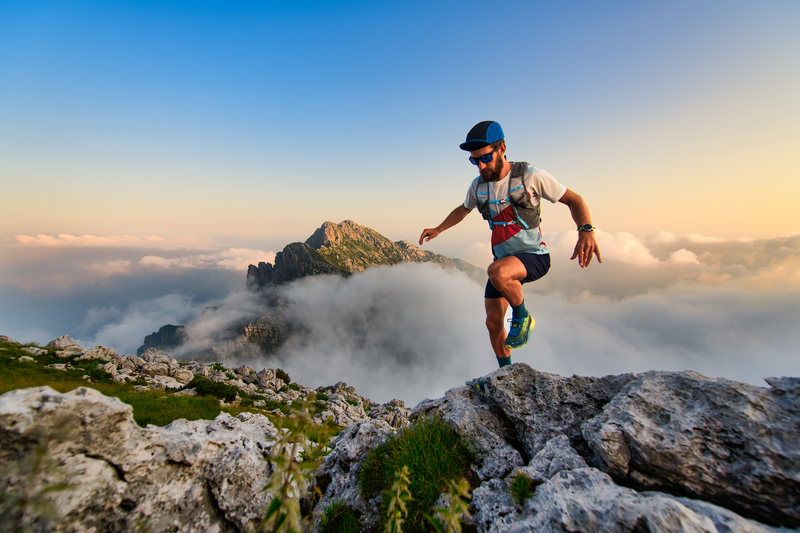
95% of researchers rate our articles as excellent or good
Learn more about the work of our research integrity team to safeguard the quality of each article we publish.
Find out more
ORIGINAL RESEARCH article
Front. Clim. , 10 December 2024
Sec. Predictions and Projections
Volume 6 - 2024 | https://doi.org/10.3389/fclim.2024.1511608
This study employs the Weather Research and Forecasting (WRF) model to dynamically downscale climate projections from the Community Climate System Model version 4 (CCSM4) using 12 km and 4 km high grid resolutions. The performance of the WRF-CCSM4 configuration is evaluated against observational data from the automated weather observing systems of Kuwait (AWOSK). The analysis focuses on future predictions for maximum soil temperature during the summer months from May to September for the period 2050–2060. The findings indicate a projected increase in average soil temperatures of 1–3°C across the Arabian Peninsula and Kuwait. Notably, the results demonstrate that the 4-km high-resolution WRF domain, is more effective framework for accurate weather and climate predictions in this region. These insights underscore the importance of high-resolution modeling in understanding and mitigating the impacts of climate change, particularly in arid environments like Kuwait and the broader Arabian Peninsula.
Soil temperature is a critical variable that influences a wide range of environmental and climate-related processes, such as plant growth, soil moisture retention, microbial activity, and land-atmosphere interactions. Accurate predictions of soil temperature are essential for applications in agriculture, ecosystem modeling, urban planning, and climate impact assessments. With the increasing effects of climate change, there is growing interest in forecasting how soil temperature will evolve over time, especially in regions sensitive to temperature fluctuations, such as deserts, permafrost areas, and agricultural zones.
This study involves using the Weather Research and Forecasting (WRF) model as a regional climate model (RCM) to dynamically downscale output from the Community Climate System Model (CCSM4) for the Arabian Peninsula, with a focus on Kuwait. The objective is to investigate how fine-scale processes like local terrain and land cover influence weather systems, particularly Soil temperatures during summer. The study spans two different 10-year periods: 2010–2020 and 2050–2060, providing insights into past and potential future summer climate conditions in the region. The GCMs are capable of simulating large-scale weather systems that affect the Arabian Peninsula. However, they lack the spatial resolution to capture fine-scale processes influenced by local terrain and land cover, which are essential for understanding regional climate dynamics. Ma et al. (2023) explored soil temperature responses in the Tibetan Plateau using the WRF model under different climate scenarios. The study found that soil temperature is highly sensitive to both atmospheric warming and snow cover variations. Yuan et al. (2016) applied WRF with the Noah LSM to study soil temperature trends in the North China Plain. The study highlighted that soil temperature will increase significantly under future climate scenarios, with implications for water use and agricultural productivity. The study used dynamic downscaling from global climate models (GCMs) and projected an increase in soil temperature by up to 3°C by 2050. Ju et al. (2022) used WRF model to predict soil temperature changes in Central Asia Arid Region. Their findings showed that WRF with high spatial resolution was able to capture soil temperature dynamics with high accuracy, particularly in agricultural and semi-arid regions. According to studies like Alpert et al. (2008) and Jiménez et al. (2012), there is a need to move beyond GCMs and adopt high-resolution dynamical downscaling models to predict future regional climates more accurately. This allows for capturing interactions among land, atmosphere, and ocean as well as microphysics and convection. The use of dynamical downscaling with a higher-resolution regional model such as WRF can improve the representation of regional climate processes (Alsarraf et al., 2019). This includes interactions between clouds, aerosols, radiation, and surface features like topography and land use. Sunyer et al. (2011) emphasized that this method provides enhanced results for studying localized climate impacts. Almazroui et al. (2020) focused on soil temperature predictions in desert environments, particularly the Arabian Peninsula. The study found that soil temperatures in the region are highly sensitive to changes in air temperature and soil moisture, with an expected increase of 1–2°C by mid-century. The results indicated that the Noah model outperformed others in capturing the extreme temperature variability typical of desert regions. Sándor and Fodor (2012) used model simulations to study the soil temperature dynamics in the semi-arid Horn of Africa. They found that climate change will exacerbate the already high soil temperatures in the region, reducing soil moisture and impacting crop yields. The Noah-MP model was instrumental in capturing the effects of soil moisture depletion on soil temperature rise. The High-resolution simulations provide more detailed soil temperature predictions, but they are computationally expensive.
The Community Climate System Model version 4 (CCSM4) output serves as the boundary conditions for the WRF simulations. CCSM4 is a global climate model that captures large-scale atmospheric processes, but its coarse resolution necessitates downscaling to capture regional and local dynamics. The WRF model was chosen for its capability to simulate weather and climate at regional scales. The WRF simulation is set up with two nested domains at resolutions of 12 km, and 4 km, focusing on progressively smaller regions and providing increasing detail. The 12 km domain focuses on a smaller regional area, and The 4 km domain zeroes in on Kuwait for a detailed study of localized effects. WRF allows for regional climate modeling at high spatial resolutions which is crucial for capturing the heterogeneity of soil temperature across different land surfaces. The simulations cover two 10-year summer periods from May to September 2010–2020: This period represents past climate conditions, providing a baseline for evaluating how well the model simulates current temperatures. The 2050–2060 period represents future climate projections, allowing for an examination of potential Soil temperature changes under future climate scenarios.
Dynamically downscaling CCSM4 using the higher-resolution WRF allows for an evaluation of the sensitivity of model performance when using high-resolution grids. By running WRF with three nested domains at grid resolutions of 12 and 4 km (Figure 1), the simulation captures both large-scale atmospheric conditions and localized small-scale features. The purpose of using nested domains is to maintain the consistency between the simulated states and the driving large-scale conditions provided by the CCSM4 model, while still allowing WRF to generate fine-scale regional dynamics that reflect the local terrain, land cover, and other small-scale processes. This hierarchical approach enables the model to downscale global data more effectively, ensuring that regional climate characteristics, such as extreme temperatures, wind patterns, or precipitation, are accurately represented in the localized simulations. By keeping the model setup similar to previous reanalysis runs, the simulation results can remain close to the observed or reanalysed state at large scales. This is important to ensure that the model does not drift away from the real-world large-scale climate drivers while allowing for high-resolution features like convection, land-ocean interactions, and complex topography to emerge in the nested WRF domains. These small-scale features are particularly important for regions with varied terrain, such as the Arabian Peninsula, where localized conditions like coastal effects and topographical variability can significantly impact climate processes.
Figure 1. High resolution WRF high resolution domains, (D02) 12-km grid resolution, (D03) 4-km grid resolution.
In this study, dynamical downscaling was performed using data generated from 10-year climate simulations covering the months of May to September for the period 2010–2020. These simulations were conducted with the CCSM4 model under the RCP8.5 scenario (a high-emission climate change scenario). Additionally, a 10-year simulation was run for the future climate period of 2050–2060 to assess how climate patterns may evolve mid-century.
The WRF model was employed to downscale the coarse-resolution CCSM4 output for both historical (2010–2020) and future (2050–2060) periods, specifically focusing on the summer months. The objective was to evaluate the sensitivity of the high-resolution WRF model (with nested domains 12, and 4 km resolution) to capture local climate features and to predict changes in the Soil Temperature (ST) during the dry summer season. The first WRF run simulated summer weather patterns over a 10-year period (May to September) to assess how well the model performs at high resolutions, with a focus on (ST) during the summer dry season, the second WRF run simulated summer weather patterns for the same months in the mid-century (2050–2060), under the RCP8.5 scenario, to predict how (ST) is likely to change in the future, accounting for the impacts of climate change. This approach enables a more precise prediction of (ST) changes during the critical dry summer season, which is especially important for regions like the Arabian Peninsula, where extreme temperatures can have severe environmental impacts.
The WRF microphysics scheme plays a crucial role in simulating the physical processes related to clouds, precipitation, and moisture within the model. In your study, where the WRF model was used for dynamical downscaling, selecting the appropriate microphysics scheme is important because it affects how the model handles cloud formation, rainfall, and other atmospheric moisture processes, which are key to accurately simulating (ST) during the summer season. The WRF Single-Moment 6-Class Scheme (WSM6) is a commonly used microphysics scheme in the WRF (Weather Research and Forecasting) model. It is designed to simulate cloud and precipitation processes by representing key microphysical variables such as water vapor, cloud water, rain, ice, snow, and graupel (soft hail). This scheme strikes a balance between accuracy and computational efficiency, making it well-suited for regional climate and weather forecasting applications, including downscaling simulations like yours. The WSM6 is a single-moment scheme, meaning that it predicts only the mass mixing ratios of hydrometeors (cloud water, rain, snow, ice, and graupel). The WSM6 scheme is computationally efficient, making it an appropriate choice for long-term climate simulations and high-resolution regional climate modeling like your study, where multiple nested domains are used. The WSM6 scheme is robust enough to be applied in arid regions like the Arabian Peninsula. Its handling of dry conditions with occasional convective storms makes it suitable for capturing cloud formation and limited precipitation processes, which affect local dynamics, particularly in regions like Kuwait (Hong et al., 2004). The Kain-Fritsch (KF) cumulus parameterization scheme is used in this study, the scheme in the WRF model to represent the effects of convection (the vertical transport of heat, moisture, and momentum) on the atmosphere. Since convection occurs on scales smaller than the grid resolution, cumulus parameterization schemes are essential in models like WRF to simulate these processes at coarser resolutions, especially in regional climate modeling. In arid regions like the Arabian Peninsula and Kuwait, where convection is less frequent but can lead to intense thunderstorms and significant temperature shifts, the Kain-Fritsch scheme helps to simulate the impact of occasional convective events on local weather patterns. Even though the Arabian Peninsula experiences dry, arid conditions during the summer, convective processes still play a role in shaping regional weather, particularly in areas near the coast or during occasional storms. Coupling with Microphysics: In conjunction with the WSM6 microphysics scheme, the KF scheme helps ensure that convective clouds, precipitation, and their impacts on local temperature and moisture distribution are accurately represented by Kain (2004). The Dudhia Shortwave Radiation (SW) scheme is used radiation parameterization in the WRF model, designed to simulate the effects of solar radiation on the atmosphere, surface, and clouds. It is an important component in climate simulations, especially in regions like the Arabian Peninsula, where solar radiation plays a crucial role in determining temperature extremes, particularly during the summer months. The Dudhia SW scheme is computationally efficient, making it ideal for long-term climate simulations where radiation processes need to be represented without overwhelming computational resources (Dudhia, 1989). The Dudhia SW scheme interacts well with the Kain-Fritsch cumulus parameterization and the WSM6 microphysics scheme, ensuring a comprehensive representation of the atmosphere (Dudhia, 1989). Together, these schemes simulate how solar radiation, convection, and cloud processes affect temperature and weather patterns at different scales. Given the 10-year climate simulations you are conducting, the Dudhia scheme’s computational efficiency makes it a practical choice, allowing you to balance between detail and computational resource use, while still capturing the most important shortwave radiation effects. The Dudhia Shortwave Radiation scheme is a suitable choice for simulating solar radiation effects in your high-resolution WRF simulations of the Arabian Peninsula. Its efficient handling of shortwave radiation, particularly in clear-sky conditions common during summer in this region, ensures accurate representation of temperature extremes and surface heating. In this study the Rapid Radiative Transfer Model (RRTM) is used in WRF model for longwave radiation (LW) and shortwave radiation (SW). It calculates the transfer of longwave radiation, which is essential for simulating the cooling of the Earth’s surface and atmosphere through the emission of infrared radiation. It also handles shortwave radiation, simulating how solar energy is absorbed, scattered, and transmitted through the atmosphere. The Rapid Radiative Transfer Model (RRTM) provides a high level of accuracy for simulating radiative transfer in the atmosphere, including the effects of greenhouse gases, clouds, and aerosols. It is particularly well-suited for climate simulations, especially in the dynamical downscaling of CCSM4 output, where capturing changes in longwave and shortwave radiation is crucial for predicting changes under future climate conditions (Mlawer et al., 1997). The Yonsei University (YSU) planetary boundary layer (PBL) scheme is a scheme in the WRF model for simulating the planetary boundary layer processes. In this study is used because It plays a critical role in representing the vertical mixing of heat, moisture, momentum, and other tracers between the Earth’s surface and the atmosphere, particularly in the lower atmosphere (up to about 1–2 km in height). It is more physically realistic for simulating large eddies, which dominate the convective mixing process in the boundary layer, particularly during the daytime when the boundary layer is well-mixed. The Yonsei University (YSU) PBL scheme is an excellent choice for simulating the boundary layer processes in your high-resolution downscaling of CCSM4 data. Its ability to handle non-local mixing, the interaction between the surface and atmosphere, and both stable and unstable conditions ensures that you can accurately simulate (ST) and other critical atmospheric parameters in Kuwait and the broader Arabian Peninsula (Hong et al., 2006).
In regions like Kuwait, with intense solar radiation and high surface temperatures during the summer, the MOST surface layer scheme plays a crucial role in simulating how heat is transferred from the surface to the atmosphere. During the day, convective turbulence drives strong upward heat fluxes, while at night, radiative cooling leads to temperature inversions and a stable boundary layer. The Monin–Obukhov Similarity Theory (MOST) is a used in the WRF model for representing the surface layer the lowest part of the atmosphere where surface-atmosphere interactions occur. The surface layer scheme based on Monin–Obukhov similarity theory provides the framework for calculating the exchange of heat, momentum, and moisture between the surface (land, water, or vegetation) and the atmosphere. In the downscaling study over the Arabian Peninsula, particularly in Kuwait, the Monin–Obukhov surface layer scheme captures critical interactions between the hot, dry land surface and the atmosphere during the summer months, significantly impacting (ST) simulations. The Monin–Obukhov similarity theory provides a robust framework for calculating surface fluxes of heat, moisture, and momentum in the WRF model. Its role in determining the surface layer stability and linking surface conditions with atmospheric turbulence makes it essential for simulating (ST) and other temperature dynamics in this downscaling study over Kuwait and the Arabian Peninsula. The Noah Land Surface Model (Noah LSM) is used in this study. The (Noah LSM) land surface model in the WRF model that simulates the interaction between the land surface and the atmosphere. It represents processes such as surface energy and water fluxes, soil temperature, and moisture dynamics. Noah LSM is crucial in determining how land surface properties influence weather and climate patterns, particularly near the surface. Noah LSM simulates soil temperature and soil moisture in multiple layers, typically ranging from the surface to a depth of about 2 meters. These layers are important for understanding how heat and moisture are stored in and transferred through the soil. Noah LSM divides the soil into four layers to simulate temperature and moisture dynamics at different depths, ranging from the surface to deeper layers. These layers help capture diurnal variations in soil temperature and the slower response of deeper soil to surface conditions. The soil moisture content affects evapotranspiration rates, which, in turn, influence latent heat fluxes. In dry, arid regions like Kuwait, where soil moisture is low, sensible heat fluxes dominate, contributing to extreme surface temperatures. In Kuwait and the Arabian Peninsula, where soil temperature is scarce and surface heating is intense, Noah LSM is particularly useful for simulating how dry soils and low vegetation cover contribute to extreme temperatures. The Noah Land Surface Model (Noah LSM) is a vital component of the WRF model for simulating land-atmosphere interactions. Its detailed treatment of surface energy fluxes, soil moisture, vegetation, and urban processes makes it essential for accurately predicting (ST) and other key variables in arid regions like Kuwait. It enhances the realism of your dynamical downscaling, ensuring that surface conditions are properly represented in both historical and future climate simulations (Chen and Dudhia, 2001) (Table 1).
In this study the WRF model’s performance using the results from the 10-year summer simulation (2010–2020), observations from Automated Weather Observing Systems of Kuwait (AWOSK) can be analysed. The model evaluation is typically done for the annual hot–dry season, focusing on daily maximum surface (TS) over the months of May, June, July, August, and September. The WRF (ST) 0–10 cm the top layer of the soil is used in this study, which interacts most directly with the atmosphere. It is sensitive to daily temperature variations and solar radiation. The (ST) from AWOS Kuwait used the 5 cm depth. The nearest grid point Comparison: The model results (WRF) are compared with the observations by extracting the grid point closest to the AWOS station. This method ensures that the spatial variation is minimized, and the comparison focuses on the same location. Several statistical measures are used to assess model performance, The Bias (1): this represents the average difference between WRF predictions and AWOS observations. A negative bias, in this case, indicates a cold bias, meaning that the WRF model is predicting lower temperatures than observed, the Root Mean Square Error (RMSE) (2): RMSE is used to quantify the magnitude of the error in the model, the Mean Absolute Error (MAE) (3): this measures the average magnitude of the errors in the WRF simulations without considering their direction, Correlation Coefficient (R): This shows the strength of the relationship between WRF predictions and AWOS observations (Tables 2, 3 and Figure 2).
Table 2. Comparing 12 km WRF with automatic weather observation system Kuwait (AWOSK) in degrees Celsius.
Table 3. Comparing 4 km WRF with Automatic weather observation system Kuwait (AWOSK) in degrees Celsius.
Figure 2. Kuwait map, (A) Abraque Alhabari (29.3703, 46.9686). (B) Jal Aliyaah (29.6124, 47.5767), (C) Managish (29.0670, 47.539), (D) Wafra (28.5930, 48.1049), (E) Abdaly (30.0235, 47.7046), (F) Kuwait International Airport (29.224, 47.9679).
1- Bias
2- RMSE
3-MAE
This Evaluation highlights the benefits of using the high-resolution WRF model for (ST) In simulations in complex terrain, such as arid and coastal areas near the gulf. The reduced relative Mean Absolute Error (MAE) and bias in the downscaled (ST) indicate that the WRF model is better able to capture both the spatial and temporal variations of (ST) in the 4 km grid resolution in this region compared to other lower-resolution models the 12 km grid resolution or global models.
By using a higher resolution, WRF can account for the detailed topography and local climatic processes in each of the seven sub-regions of the Arabian Peninsula, and Kuwait, leading to significant improvements in simulating the summer (ST). This suggests that WRF, when used for dynamical downscaling, enhances the accuracy of regional climate predictions, especially in areas with complex terrain where local weather patterns are strongly influenced by altitude, slope, and landforms.
The WRF-CCSM4 12-km high-resolution simulations reveal significant spatial variations in maximum (ST) increases across the Arabian Peninsula during the summer months (May to September). These temperature increases reflect the warming trends projected by the WRF-CCSM4 model at a finer 12-km resolution, highlighting specific regional variations across the Arabian Peninsula during the summer. The WRF-CCSM4 results suggest that the northwest region experiences the most significant warming, in May with a peak increase of 5°C, likely influenced by the local terrain, atmospheric conditions, and land surface characteristics. This warming could have critical implications for the region’s agriculture, water resources, and energy demands during the summer months, while the southern region shows a significant increase of 3°C. The northeast region, including Kuwait, experiences a more moderate warming with increase of 1°C compared to the central region, where the temperature rise 2°C is more pronounced (Figure 3A). The WRF-CCSM4 suggests that in June, the northwest, central, and southern regions experience more intense warming with a 3°C rise, while the northeast sees a smaller but still significant 2°C increase in maximum soil temperature (Figure 3B). In July, the WRF-CCSM4 model indicates that July experiences widespread warming across the region, with most areas seeing a significant 3°C rise, while the southern part of the Arabian Peninsula experiences a somewhat more moderate increase of 2°C (Figure 3C). In August the model sees significant warming across much of the Arabian Peninsula, especially in the east and most of the region, while the southeast experiences a more moderate warming. Interestingly, the southwest shows a slight cooling trend with a 1°C decrease, which could be due to local atmospheric or land-surface interactions (Figure 3D). A decrease in soil temperature could indicate shifts in regional climate patterns, such as increased rainfall or altered atmospheric conditions. In September, the WRF-CCSM4 most regions of the Arabian Peninsula: An increase of 1°C to 2°C (Figure 3E). This indicates that September continues the trend of warming seen throughout the summer months, albeit at a slightly lower intensity compared to the peak temperatures observed in the earlier summer months.
Figure 3. WRF-CCSM4 12 km by 12 km grid resolution: maps showing differences in monthly average maximum (ST) between (2010–2020) and Future projections (2050–2060). (A) May, (B) June, (C) July, (D) August, (E) September.
The WRF-CCSM4 4 -km high-resolution in May The increase of 1°C over Kuwait suggests a localized warming effect, while the 2°C increase in most other areas reflects a broader trend of rising temperatures across the region (Figure 4A). In June the 2°C increase in Kuwait and surrounding Gulf countries suggests a continuation of warming trends, impacting local agriculture, water resources, and ecosystems. The 3°C increase in certain areas of Saudi Arabia indicates a more intense warming effect in those regions (Figure 4B). In July The 2°C increase near the coastal areas may be influenced by the moderating effect of the sea breeze, which can help regulate temperatures somewhat compared to inland areas (Figure 4C). The 3°C increase further west indicates a more significant warming trend, and less sea breeze effect (Alsarraf et al., 2019). In August the 3°C increase across Kuwait and the broader region indicates a significant rise in temperatures, the 2°C increase near the coastal areas suggests some moderating influence from the sea. WRF projected 4°C increase in some regions of the United Arab Emirates (UAE) in August (Figure 4D). In September, the WRF-CCSM4 model projects an overall increase of 1°C across most regions including Kuwait, and 2°C increase in certain areas suggests localized hotspots where temperatures may rise more significantly (Figure 4E).
Figure 4. WRF-CCSM4 4 km by 4 km grid resolution: maps showing differences in monthly average maximum (ST) between (2010–2020) and future projections (2050–2060). (A) May, (B) June, (C) July, (D) August, (E) September.
Utilizing a high-resolution WRF model is vital for capturing the complexities of soil temperature dynamics in the Arabian Peninsula. The significant benefits derived from finer resolutions not only improve the accuracy of temperature predictions but also enhance the understanding of local climatic impacts, which is crucial for effective climate adaptation and mitigation strategies. The findings underscore the need for continued investment in high-resolution modeling to address the unique climatic challenges faced by this region. The WRF model at high resolutions is crucial for accurately predicting soil temperature (ST) across the Arabian Peninsula and Kuwait. Higher spatial resolution allows for more precise representation of local climatic conditions and interactions. Experimental results demonstrate that a 4-km resolution WRF model outperforms the 12-km resolution version in simulating soil temperatures. This improvement can be attributed to the model’s enhanced ability to capture fine-scale features and local dynamics. The use of detailed parameterizations, including sophisticated land surface models, significantly improves the simulation of extreme climatic events, such as heat waves. These improvements are essential for accurate future climate projections, as highlighted by Schneider et al. (2015). In regions like Kuwait, where mesoscale circulations have a pronounced effect on local weather, high resolution is especially important. Factors such as the sea breeze circulation and lee-side northerly dry winds during summer substantially influence the surface temperature gradient. The detailed representation of these phenomena in a high-resolution WRF model enhances the accuracy of simulations, as noted in studies by Alsarraf and Van Den Broeke (2016) (Jiang et al., 2018, Zhang et al., 2016).
This preliminary study investigates the fine-scale patterns of soil temperature (ST) changes over the Arabian Peninsula and Kuwait, employing a dynamical downscaling method using the WRF model with both 12-km and 4-km grid spacing. The simulations were conducted with the WRF model, utilizing outputs from the Community Climate System Model version 4 (CCSM4) as boundary conditions. The 4-km high-resolution WRF significantly outperformed the 12-km resolution in capturing temperature dynamics over the dry summer regions. This improvement is attributed to the model’s enhanced capability to resolve local climatic features and mesoscale processes. The results provide substantial evidence that mesoscale processes play a critical role in influencing soil temperature changes. These processes are particularly important for understanding the nuances of temperature variation and predicting extreme climatic events. Utilizing a high-resolution WRF model improves the accuracy of future predictions regarding soil temperature in the context of climate change. The model minimizes prediction errors, offering a more reliable forecast for stakeholders.
In conclusion, the preliminary findings underscore the importance of high-resolution modeling in understanding and predicting soil temperature changes due to climate change in arid regions. The insights gained from this research can inform climate adaptation strategies, improve resource management, and enhance preparedness for extreme weather events in the Arabian Peninsula. This work sets the foundation for future studies aimed at refining climate predictions and mitigating the impacts of climate change.
The original contributions presented in the study are included in the article/supplementary material, further inquiries can be directed to the corresponding author.
HA: Conceptualization, Data curation, Formal analysis, Funding acquisition, Investigation, Methodology, Project administration, Resources, Software, Supervision, Validation, Visualization, Writing – original draft, Writing – review & editing.
The author(s) declare that financial support was received for the research, authorship, and/or publication of this article. This publication was made possible by the support of the AUK Open Access Publishing Fund.
I would like to express my sincere gratitude to the American University of Kuwait for their unwavering support throughout this project. The institution’s commitment to fostering academic excellence and providing resources has been instrumental in the successful completion of this study. I deeply appreciate the collaborative environment and encouragement that have significantly contributed to my professional and academic growth.
The author declares that the research was conducted in the absence of any commercial or financial relationships that could be construed as a potential conflict of interest.
The author(s) declare that no Generative AI was used in the creation of this manuscript.
All claims expressed in this article are solely those of the authors and do not necessarily represent those of their affiliated organizations, or those of the publisher, the editors and the reviewers. Any product that may be evaluated in this article, or claim that may be made by its manufacturer, is not guaranteed or endorsed by the publisher.
Almazroui, M., Saeed, F., Saeed, S., Islam, M. N., Ismail, M., Klutse, N. A. B., et al. (2020). Projected change in temperature and precipitation over Africa from CMIP6. Earth Syst. Environ. 4, 455–475. doi: 10.1007/s41748-020-00161-x
Alpert, P., Kishcha, P., Kaufman, Y. J., and Schwarzbard, R. (2008). Global dimming or local dimming? Effect of urbanization on sunlight availability. Geophys. Res. Lett. 32:L17802. doi: 10.1029/2005GL023320
Alsarraf, H., and Van Den Broeke, M. (2016). Using high-resolution WRF model simulations to investigate the relationship between mesoscale circulations and aerosol transport over Kuwait. J. Climatol. Weather Forecast. 3, 1–12. doi: 10.4172/2332-2594.1000126
Alsarraf, H., Van Den Broeke, M., and Aljassar, H. (2019). Effects of the sea breeze circulation on soil temperature over Kuwait using in situ observations and the ECMWF model. Open Atmo. Sci. J. 13, 29–42. doi: 10.2174/1874282301913010029
Chen, F., and Dudhia, J. (2001). Coupling an advanced land surface–hydrology model with the Penn State–NCAR MM5 modeling system. Part I: model implementation and sensitivity. Mon. Weather Rev. 129, 569–585. doi: 10.1175/1520-0493(2001)129<0569:CAALSH>2.0.CO;2
Dudhia, J. (1989). Numerical study of convection observed during the winter monsoon experiment using a mesoscale two-dimensional model. J. Atmos. Sci. 46, 3077–3107. doi: 10.1175/1520-0469(1989)046<3077:NSOCOD>2.0.CO;2
Hong, S.-Y., Dudhia, J., and Chen, S.-H. (2004). A revised approach to ice microphysical processes for the bulk parameterization of clouds and precipitation. Mon. Weather Rev. 132, 103–120. doi: 10.1175/1520-0493(2004)132<0103:ARATIM>2.0.CO;2
Hong, S. Y., Noh, Y., and Dudhia, J. (2006). A new vertical diffusion package with an explicit treatment of entrainment processes. Mon. Weather Rev. 134, 2318–2341. doi: 10.1175/MWR3199.1
Jiang, Z., Li, J., Huang, G., Ma, J., and Wang, S. (2018). Effects of the sea breeze circulation on regional climate: a review. Atmos. Res. 209, 134–142.
Jiménez, P. A., Dudhia, J., González-Rouco, J. F., Navarro, J., Montávez, J. P., and García-Bustamante, E. (2012). A revised scheme for the WRF surface layer formulation. Mon. Weather Rev. 140, 898–918. doi: 10.1175/MWR-D-11-00056.1
Ju, C., Li, H., Li, M., Liu, Z., Ma, Y., Mamtimin, A., et al. (2022). Comparison of the forecast performance of WRF using Noah and NOAH-MP land surface schemes in Central Asia arid region. Atmos. 13:927. doi: 10.3390/atmos13060927
Kain, J. S. (2004). The Kain-Fritsch convective parameterization: an update. J. Appl. Meteorol. 43, 170–181. doi: 10.1175/1520-0450(2004)043<0170:TKCPAU>2.0.CO;2
Ma, M., Tang, J., Ou, T., and Zhou, P. (2023). High-resolution climate projection over the Tibetan plateau using WRF forced by bias-corrected CESM. Atmos. Res. 286:106670. doi: 10.1016/j.atmosres.2023.106670
Mlawer, E. J., Taubman, S. J., Brown, P. D., Iacono, M. J., and Clough, S. A. (1997). Radiative transfer for inhomogeneous atmospheres: RRTM, a validated correlated-k model for the longwave. J. Geophys. Res. Atmos. 102, 16663–16682. doi: 10.1029/97JD00237
Sándor, R., and Fodor, N. (2012). Simulation of soil temperature dynamics with models using different concepts. Sci. World J. 2012:590287. doi: 10.1100/2012/590287
Schneider, U., Becker, A., Finger, P., Meyer-Christoffer, A., and Ziese, M. (2015). “GPCC full data reanalysis version 7.0 at 0.5°: monthly land-surface precipitation from rain gauges built on GTS-based and historical data,” in Global Precipitation Climatology Centre (GPCC), Offenbach am Main: Deutscher Wetterdienst.
Sunyer, M. A., Madsen, H., and Ang, P. H. (2011). A comparison of different regional climate models and statistical downscaling methods for extreme rainfall estimation under climate change. Atmos. Res. 103, 119–128.
Yuan, C., Ng, E., and Norford, L. (2016). Improving air quality in high-density cities by understanding the relationship between air pollutant dispersion and urban morphologies. Build. Environ. 71, 245–258. doi: 10.1016/j.buildenv.2013.10.008
Keywords: WRF, CCSM4, future climate predictions, soil temperature, climate change
Citation: Alsarraf H (2024) Dynamic downscaling of climate projections using WRF-CCSM4: future soil temperature predictions for the Arabian Peninsula and Kuwait. Front. Clim. 6:1511608. doi: 10.3389/fclim.2024.1511608
Received: 15 October 2024; Accepted: 18 November 2024;
Published: 10 December 2024.
Edited by:
Matthew Collins, University of Exeter, United KingdomReviewed by:
Goran Trbic, University of Banjaluka, Bosnia and HerzegovinaCopyright © 2024 Alsarraf. This is an open-access article distributed under the terms of the Creative Commons Attribution License (CC BY). The use, distribution or reproduction in other forums is permitted, provided the original author(s) and the copyright owner(s) are credited and that the original publication in this journal is cited, in accordance with accepted academic practice. No use, distribution or reproduction is permitted which does not comply with these terms.
*Correspondence: Hussain Alsarraf, ZHIuaHVzc2Fpbi5hbHNhcnJhZkBnbWFpbC5jb20=
Disclaimer: All claims expressed in this article are solely those of the authors and do not necessarily represent those of their affiliated organizations, or those of the publisher, the editors and the reviewers. Any product that may be evaluated in this article or claim that may be made by its manufacturer is not guaranteed or endorsed by the publisher.
Research integrity at Frontiers
Learn more about the work of our research integrity team to safeguard the quality of each article we publish.