- 1Department of Geography, Philipps-Universität Marburg, Marburg, Germany
- 2Institute for Lung Research, German Center for Lung Research (DZL), Universities of Giessen and Marburg Lung Centre, Philipps-Universität Marburg, Marburg, Germany
- 3Department of Medicine, Pulmonary and Critical Care Medicine, German Center for Lung Research (DZL), University Medical Center Giessen and Marburg, Marburg, Germany
- 4CAPNETZ STIFTUNG, Hannover, Germany
- 5Department of Infectious Diseases and Microbiology, University Hospital Schleswig-Holstein, Lübeck, Germany
- 6Department of Infectious Diseases, Respiratory Medicine and Critical Care, Charité-Universitätsmedizin Berlin, Freie Universität Berlin, Humboldt-Universität zu Berlin, and Berlin Institute of Health, Berlin, Germany
- 7Department of Respiratory Medicine, Goethe University Frankfurt, University Hospital, Medical Clinic I, Frankfurt, Germany
- 8Biomedical Research in Endstage and Obstructive Lung Disease Hannover (BREATH), Member of the German Center for Lung Research, Hannover, Germany
- 9Institute for Infectious Diseases and Infection Control, Jena University Hospital, Jena, Germany
- 10German Center for Infection Research (DZIF), The Center for Synthetic Microbiology (SYNMIKRO), Marburg, Germany
- 11Institute for Lung Health (ILH), Giessen, Germany
Community-acquired pneumonia (CAP) is one of the most frequent causes of death among infectious diseases worldwide. There is a growing concern about weather impacts on CAP. However, no studies have examined the effects of comorbidities and personal characteristics alongside the twofold impact of weather conditions (meteorological and air quality) on CAP. Our study investigates how personal characteristics (age, sex, and BMI) and comorbidities (asthma, chronic heart disease, COPD, diabetes, heart insufficiency, smoking, and tumor) and care influence the twofold compound impact of weather on CAP admissions. We match medical data from a German multicentre cohort of 10,660 CAP patients with daily regional weather data, using logistic regressions to calculate the “Pneumonia Risk Increase Factor” (PRIF). This factor quantifies the heightened risk of CAP admissions due to weather conditions. We demonstrate that individuals with specific personal characteristics and those with comorbidities are more susceptible to weather impacts in the context of CAP than their counterparts. People with COPD have a PRIF of 5.28, followed by people in care (5.23) and people with a high BMI (4.02). Air pollutants, particularly CO and PM2.5, play a significant role in increasing CAP hospitalizations. For meteorological conditions, air pressure and lower temperatures, combined with air pollutants, lead to high PRIFs. Our findings emphasize the increased weather vulnerability of old, high BMI, and males and people with comorbidities. This provides invaluable information to support at-risk individuals through protective measures and provides healthcare providers as well as health policymakers with insights for resource planning before and during pneumonia-contributing weather conditions.
1 Introduction
Community-acquired pneumonia (CAP) poses a substantial public health challenge, marked by its significant impact on morbidity, mortality, and healthcare expenditures worldwide (Kyu, 2022) but also within Germany (Theilacker et al., 2021). In Germany, hospitalizations for this condition have ranged from 250,000 to 290,000, with an associated mortality rate of approximately 13% (Institut für Qualitätssicherung, 2020). A study from 2016 to 2019 examined the medical costs associated with all-cause pneumonia among individuals aged 16 or older in the German InGef database, which estimated a yearly direct medical expense of about €83 million (Deb et al., 2022).
Comorbidities and personal characteristics in the context of CAP significantly influence both the susceptibility (Molinos et al., 2009) to and severity of the disease (Hespanhol and Bárbara, 2020). Individuals with comorbidities, such as respiratory diseases, cardiovascular diseases, diabetes, or compromised immune systems, are often at heightened risk of developing CAP. Moreover, personal characteristics, including age, sex, socioeconomic status, and lifestyle factors, can further modulate this risk (Cillóniz et al., 2013). For instance, advanced age and male gender have been associated with increased susceptibility to CAP, while socioeconomic disparities may impact access to healthcare and predispose specific populations to a higher burden of disease (Luna et al., 2016). Understanding the interplay between comorbidities, personal characteristics, and CAP risk is essential for targeted prevention strategies, early detection, and tailored management approaches to reduce the overall disease burden and improve patient outcomes.
On top of the role of comorbidities and personal characteristics, weather, including meteorological as well as air quality conditions, has been shown to impact health, specifically respiratory diseases such as CAP (Hwang et al., 2023; Vicedo-Cabrera et al., 2023). Several studies have explored the direct and indirect influence of climatic conditions on health, focusing primarily on temperature’s potential role in CAP (Baker et al., 2019; Li et al., 2019). However, the nature of this relationship, whether j-shaped, u-shaped, or v-shaped, varies significantly based on geographical location, associated climate patterns, and specific temperature conditions (Adegboye et al., 2020; Wu et al., 2024; Braga et al., 2002; Falagas et al., 2008). Additionally, precipitation and relative humidity’s impact on CAP has been predominantly observed in regions with abundant rainfall, such as tropical areas (Nascimento-Carvalho et al., 2010).
Furthermore, most investigations into the effects of weather on respiratory diseases focus on air pollution, particularly in urban Asian contexts (Dong et al., 2021; Fan et al., 2024). Most of these studies tend to show positive correlations between ambient air pollutant levels and hospitalizations due to CAP (Ruchiraset and Tantrakarnapa, 2022; Zhang et al., 2021a). A systematic review and meta-analysis found a short-term correlation between ambient air pollutants (sulfur dioxide (SO2), ozone (O3), nitrogen dioxide (NO2), carbon monoxide (CO), and particulate matter PM10 and PM2.5) and hospitalizations of children due to CAP (Nhung et al., 2017). The study reported a positive correlation, with SO2, PM2.5, and PM10 showing the highest pollutant-specific excess risk percentages. Additionally, a long-term prospective observational study in a Chinese hospital indicated that meteorological and air pollution conditions contributed more to severe CAP in children than respiratory viruses (Hoffmann et al., 2022).
Consequently, while numerous studies have investigated the individual relationships between weather conditions, air quality, and CAP, there remains a notable gap in the literature regarding their interconnectedness. One study exists that looks at the twofold compound effect of weather conditions (meteorological and air quality) on pneumonia hospitalizations, indicating that it is the twofold compound rather than the individual effect of one specific weather condition that leads to a higher risk increase of acquiring pneumonia (Brenner et al., 2022). One other study assesses the modification effect of temperature on the relationship between air pollutants and the daily influence of influenza but not on pneumonia (Zhang et al., 2021b). Hence, no study has comprehensively assessed the connection between all three elements, namely the impact of weather conditions, comorbidities, and personal characteristics, on CAP. If CAP studies account for pre-existing characteristics, they tend only to cover basic personal characteristics such as age and sex and how they modulate the impact of air quality conditions on CAP (Jin et al., 2022). For other respiratory diseases, such as COVID-19, studies exist that investigate the role of comorbidities and air quality on hospitalizations (Sarmadi et al., 2021). There is one study dedicated solely to examining the heightened risk of mortality among individuals with pre-existing cardiovascular and respiratory conditions, as well as certain demographic groups, such as the elderly, infants, and those with low socioeconomic status, concerning ambient air temperature (Basu and Samet, 2002). However, this study does not explicitly focus on CAP.
We addressed these shortcomings by comprehensively assessing the impact of personal characteristics and comorbidities on the twofold compound effect of weather conditions (meteorological and air quality conditions) on CAP admissions. By doing this, we want to understand who is the most vulnerable to weather conditions in regard to CAP admissions, considering personal characteristics such as age, sex, and BMI alongside comorbidities such as respiratory (COPD and asthma), cardiovascular (chronic heart disease and heart insufficiency), behavioral (smoking), and other health and living conditions (tumor, diabetes, and living in care). To achieve this, we analyze the CAPNETZ cohort data from 10,660 patients treated for CAP in 22 German hospitals or outpatient clinics. In our methodology, we align medical data with daily high-resolution regional meteorological data, including temperature, relative humidity (RH), precipitation, and wind, as well as air quality data encompassing carbon monoxide (CO), dust aerosols (DA), nitrogen dioxide (NO2), ozone (O3), particulate matter smaller than 2.5 μm in diameter (PM2.5), sulphur dioxide (SO2), and total aerosols (TA). We employ logistic regressions to calculate what we term the “Pneumonia Risk Increase Factor” (PRIF), which quantifies the increased risk of CAP admissions induced by weather and/or air quality conditions. The objective of this study is to identify the personal characteristics or comorbidities that are most strongly associated with an increased risk of hospitalization for CAP in the context of specific weather conditions.
2 Materials and methods
2.1 Medical data description
The medical dataset includes 10,660 individuals (Supplementary Table 1) hospitalized for pneumonia across 22 German hospitals or medical practices from 2003 to 2017 (Supplementary Table 2). These data were collected from the prospective cohort CAPNETZ study registered under study NCT 02139,163. The data set includes detailed information for each pneumonia patient on personal characteristics, including sex, age, and BMI, as well as comorbidities, including asthma, Body Mass Index (BMI), chronic obstructive pulmonary disease (COPD), diabetes, chronic heart disease, heart insufficiency, smoking, tumor, and living conditions such as whether they are in care. A detailed description of the CAPNETZ methodology is given elsewhere (Welte et al., 2004). Inclusion criteria were age ≥ 18 years, pulmonary infiltrates diagnosed by chest imaging, and at least one of the following criteria: history of fever (temperature ≥ 38.3°C), cough, production of purulent sputum, or focal chest signs on auscultation. Exclusion criteria were acquired or therapeutically induced immune deficiency, active tuberculosis, or nosocomial acquisition of infection. Clinical data were stored electronically, including the exact day of hospitalization. All patients were followed up according to a standardized protocol for 180 days. To evaluate outcome parameters, all patients or their relatives are contacted either personally or by phone for structured interviews. Written informed consent is obtained from every patient before the study. The local ethical committees of each CAPNETZ participating center approved the study (Ethics approval Nr.301–2008, Hannover Medical School). We therefore confirm that all experiments were performed in accordance with relevant guidelines and regulations.
2.2 Meteorological and air quality data description
The analysis includes 12 meteorological and air quality (weather) variables. The meteorological conditions include five variables: air pressure, relative humidity, wind speed, maximum temperature, and precipitation exceeding the 95th percentile. The air quality conditions include seven variables: CO, NO2, O3, SO2, PM2.5, DA, and TA (Supplementary Table 3). The conditions used in the analysis are chosen from a larger list of conditions according to their statistical adequateness, as reported in Brenner et al. (2022).
The meteorological data (2003–2017) from the operational official station monitoring networks of the German Weather Service (DWD) covers daily mean values of air pressure, relative humidity, and maximum temperature (Behrendt et al., 2011; DWD Climate Data Center, 2021). Quality controls include temporal and content consistency checks to correct for random and gross measurement errors. The Shuttle Radar Topography Mission (SRTM) version 4 terrain model is an established spatial predictor of climatological effects due to terrain. The terrain elevation data with an original mean resolution of 60 × 60 meters in Germany are bilinearly resampled into 500 × 500 meter cells. To obtain regionalized climate values for each municipality in Germany, the standard interpolation method, Ordinary kriging with external drift (KED), is used to spatially interpolate the daily climate data. In the kriging-based approach, the study area of Germany is divided into a finite number of cells (2,226,005) to determine climate parameter fields. The individually optimized covariance model for each meteorological condition is used in KED to calculate daily meteorological maps using R-software. The SRTM terrain heights are integrated into the Kriging procedure to represent the external drift.
The precipitation and wind speed data comes from the COSMO-REA6 reanalysis, with a 6 × 6 km grid resolution (Bollmeyer et al., 2015). The extreme precipitation data is based on E-OBS observational data at the daily temporal scale and a spatial resolution of 0.02° x 0.02° (Copernicus Climate Change Service, 2020). The air pollutant data comes from Copernicus Atmosphere Monitoring Service global reanalysis (EAC4) with a spatial resolution of 0.75° x 0.75° (Inness et al., 2019). As a final step, current municipality geometries were homogenized to 2012 based on official Eurostat data (Europäische Kommission - Eurostat/GISCO, 2022) to calculate descriptive statistics of climate variables for each municipality. Proportional grid cells with appropriate precision weighting were also considered.
2.3 Data analysis
To assess the impact of weather conditions on CAP morbidity, we use real-cases (day person was hospitalized) and non-real cases (same location and pre-condition (personal characteristics and comorbidities) but a different hospitalization date). Five non-real cases are randomly generated for each person in the medical dataset. These non-real cases can be interpreted as alternative days on which a person could have been admitted but actually has not. The non-real cases are necessary to examine the impact of weather conditions on the risk of pneumonia-related hospitalization. We are not studying the probability of people being hospitalized for pneumonia. Instead, we examine how weather conditions modulate this probability.
With a logistic regression approach, we compare the conditions that were present on the days before real hospitalization cases with the conditions on other days without such a hospitalization, the non-real cases. We match the weather data to each case (real and non-real) in our medical dataset. The adequateness of the logistic regression is provided due to only a slightly significant deviation (p = 0.04) from the assumed logistic distribution, which is minimal given the number of observations (Hosmer et al., 2013). The weather conditions are assigned to each real and non-real case based on the location of the hospital and the hospitalization day. Since people tend to live near the hospitalized clinic, we assess the weather conditions in all municipalities that are less than 1-h driving time away from the clinic. A weighted average is built according to the municipality’s population and its distance to the hospital, using a distance decay function (Brenner et al., 2022).
Besides including the variables directly as independent variables, we also test the following: (1) linear and quadric terms of each independent variable, and (2) interaction term for each pair of conditions in the logistic regression. Each pair of two meteorological conditions, two air quality conditions, and a meteorological with an air quality condition is considered. The interaction terms are built by the multiplication of values of the two variables or their squared values. The variant with the best fit (lowest Akaike Information Criterion (AIC)) is chosen for the final regression for each pair of variables.
After identifying for each pair of variables the best variant and building a complete model, we successively reduce the number of terms (combinations of variables) in the regression equation by each time removing the term whose exclusion leads to the highest decrease of the AIC until no further reduction of the AIC is possible so that the best fitting (lowest AIC) model is found. This process leads to a final regression model with 36 independent variables. Since the literature has shown that weather conditions tend to influence hospitalization with a time delay, we ran the whole approach outlined above for a one-day lag, which led to the best fit of the model based on AIC (Pedder et al., 2021).
Our central aim is to study the impact of personal characteristics and comorbidities on the sensitivity to weather conditions in the context of CAP. Therefore, we repeat the above approach for each group of people with particular personal characteristics and comorbidities. For the personal characteristics of age and BMI, the median is used to categorize our complete sample into (i) young (<64) and old (≥64), (ii) low and high BMI (split at BMI = 24). For all other characteristics and comorbidities, we split our sample into the groups with and without the characteristic or comorbidity. The corresponding estimated coefficients and risks for obtaining CAP, which are estimated in the regression, are compared between the subgroups. With this approach, we examine whether people with particular personal characteristics or comorbidities react differently to each assessed weather condition by itself and each assessed twofold weather combination than the rest of the population. In total, we compare the risk for all 66 combinations of weather conditions and the eleven pairs of subgroups. We also evaluated the PRIFs for the entire population, which were either nearly identical to the cases classified as “without comorbidities” or fell between the two examined sub-groups (e.g., low/high). This prompted us to present the contrasting sub-groups (e.g., with/without, low/high, male/female) for more straightforward comparisons. A table of the regression results (lower confidence boundary (CB), estimate, and high confidence boundary) of all (whole population) and sub-populations based on sex, BMI, age, and with/without comorbidities are found in the supplementary material (Supplementary Table 4).
We study the increased probability of hospitalization due to weather conditions, meaning that we calculate for each independent variable (or combination of two variables) the factor with which the probability is multiplied for this variable’s values (similar to an odds ratio). We use the expression Pneumonia Risk Increase Factor (PRIF) for this factor. For example, the PRIF for specific values of a particular pair of conditions is calculated by inserting these values into the estimated regression equation to calculate the respective probability predicted by the regression model. All other variables are set to their median. The resulting predicted probability is then divided by the likelihood the regression model predicts for a situation where all variables take their median values. The result of this division is called PRIF in the paper, mathematically defined as in Equation 1.
where stands for the probability predicted by the model obtained by the logistic regression, and denote the values of the weather conditions that are looked at, denotes all other conditions, denotes all weather conditions, and ˜ stands for the median value. If the PRIF is 1, the risk under the considered conditions is the same as in the median situation. A value higher than one signals a higher risk of being hospitalized for CAP, and a lower value signals a lower risk compared to median conditions.
As usual in statistical approaches, the estimated PRIFs might strongly depend on the specific weather conditions in our real cases. Therefore, we use a bootstrap approach to calculate the reliability of the estimated PRIF values. We randomly redraw our real and comparison cases 200 times and use the above-described approach for each redrawing. This leads to different regression results for each bootstrap draw and allows us to calculate confidence intervals for all PRIF values. Using this bootstrap approach makes the results independent of single values in our data. Each individual data point enters each of the bootstrap draws with a likelihood of 63.25%. Hence, it is essential to focus not on the estimates themselves but on their confidence intervals. As usual, we use 95% confidence intervals, implying that the real values lay within them with a probability of 95%. Since we examine how much the likelihood of being hospitalized for CAP increases due to the weather conditions, the lower border of the confidence interval of the PRIF is of specific interest. We can state that the real PRIF takes this or a higher value with a likelihood of 97.5%. The lower boundary of the confidence interval is called the 2.5% percentile of the PRIF. In the following, we always present and discuss this 2.5% percentile of the PRIF as PRIF percentile or PRIF. This is a very conservative estimate of the risk increase for being hospitalized for CAP due to the respective weather conditions, for which we can be pretty sure (error probability: 2.5%) that the real value is at least as high.
3 Results
3.1 Weather vulnerable groups in the context of CAP
In the following, we assess the vulnerability to weather conditions in the context of CAP of certain groups based on their personal characteristics (sex, age, and BMI) as well as comorbidities (asthma, COPD, chronic heart disease, heart insufficiency, diabetes, smoking, and tumor) and living conditions (care). Overall, we find 53 combinations of weather conditions that lead to significant PRIF differences between the subgroups of personal characteristics and comorbidities. The results we display here are selected based on the following approach: coefficient plots are displayed for combinations where we see significant differences between the subgroups (confidence intervals do not overlap) and the lower 2.5% percentile of the PRIF being higher than 1.8. If more than seven cases are fulfilling this requirement, we display the seven cases with the highest values of the PRIF percentile. In the two-dimensional PRIF plots, we only display the combination with the highest 2.5% percentile for combinations with significant differences between the subgroups. We tend to see substantial differences between the subgroups for personal characteristics and comorbidities, which are discussed in more detail below.
3.1.1 Personal characteristics
The twofold combinations which display significant differences between the subgroups and lead to the highest lower confidence boundary coefficient for each personal characteristic group are displayed in Figure 1. Older people, males, and people with high BMI seem more vulnerable to weather conditions in the context of CAP than their respective counterparts. For both older people and males, we observe a stronger vulnerability to high CO conditions compared to young people and females. In the case of older people, only the CO concentration in the air matters (lower boundary of the PRIF values at 1.9). In the case of males, the PRIF is strongly increased if, in addition to high CO also, high values of SO2 are present (lower 2.5% percentile of the PRIF values at 3.7). Furthermore, we find that either combinations of meteorological conditions and air quality conditions or combinations of two air quality conditions cause the differences between the subgroups. Combinations of two meteorological conditions are not found to cause significant differences.
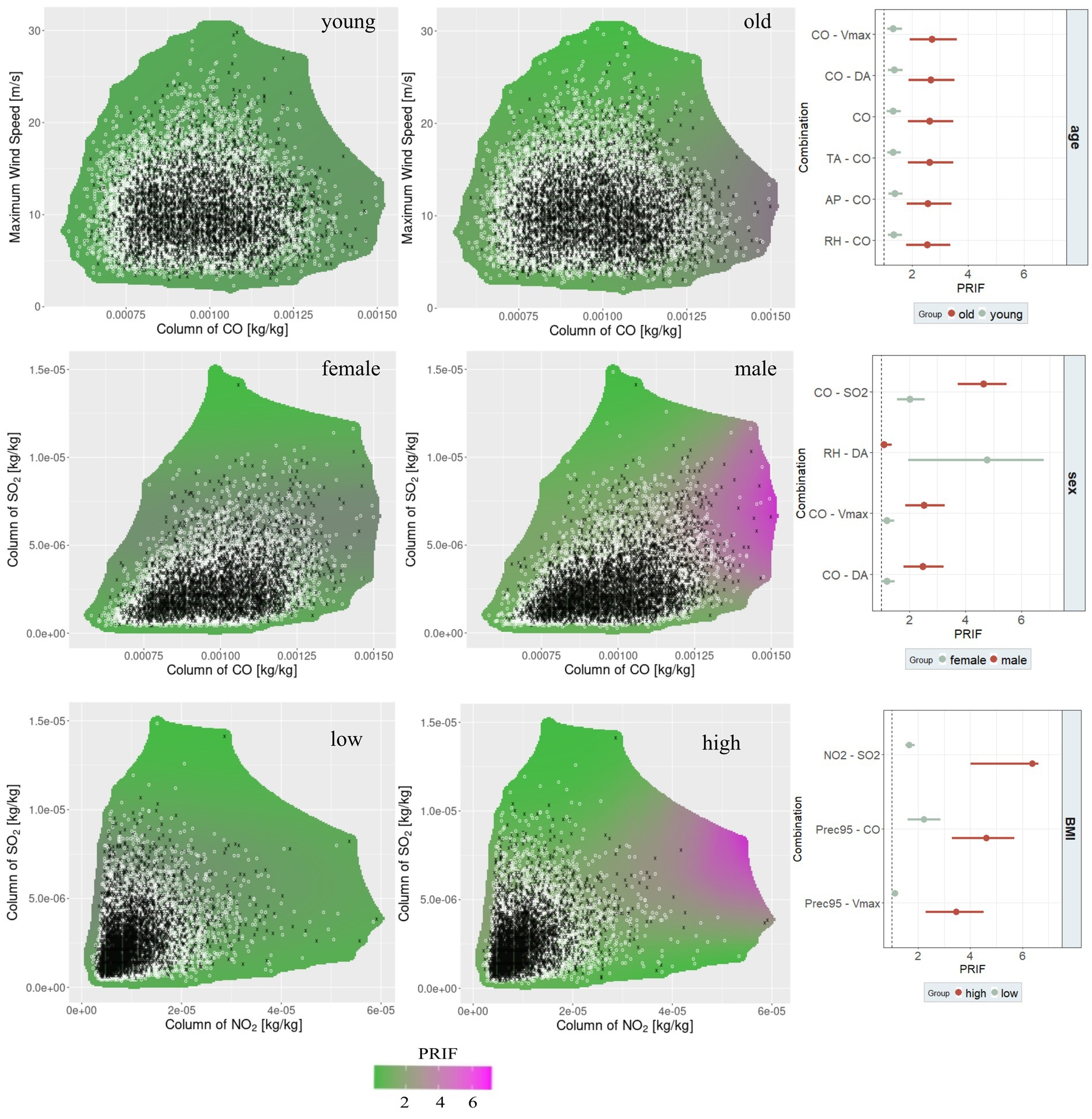
Figure 1. Highest two-dimensional PRIF figures for sub-groups with significant differences and highest lower confidence boundary and coefficient plot for significant differences between sub-groups for personal characteristics: age, sex, and BMI (black x = real cases, white x = non-real cases).
While females are less vulnerable to high CO and SO2 values, they are more susceptible to the combination of Relative Humidity (RH) and Dust Aerosols (DA). For people with high BMI, it is the combination of levels of NO2 and high levels of SO2 that leads to the highest PRIF values (3.30). Hence, for the higher BMI group and females, it is a mix of meteorological and air quality conditions that leads to significant differences between this subgroup and its counterpart.
3.1.2 Comorbidities
The twofold weather combinations, which lead to the highest 2.5% percentile of the PRIF for each comorbidity, are displayed in a two-dimensional PRIF plot together with the twofold weather combinations, which have significantly different subgroups displayed in coefficient plots (Figure 2). For most comorbidities, the PRIF for people with a comorbidity is higher than those without a comorbidity. There are a few deviations from this overall finding in the cases of smoking, COPD, and diabetes that we discuss in the respective sub-sections below. The highest 2.5% percentile of the PRIF in the comorbidities group is found for people with COPD (5.28), followed by people who are in care (5.23). For comorbidities, we also do not find combinations of two meteorological conditions that cause significant differences.
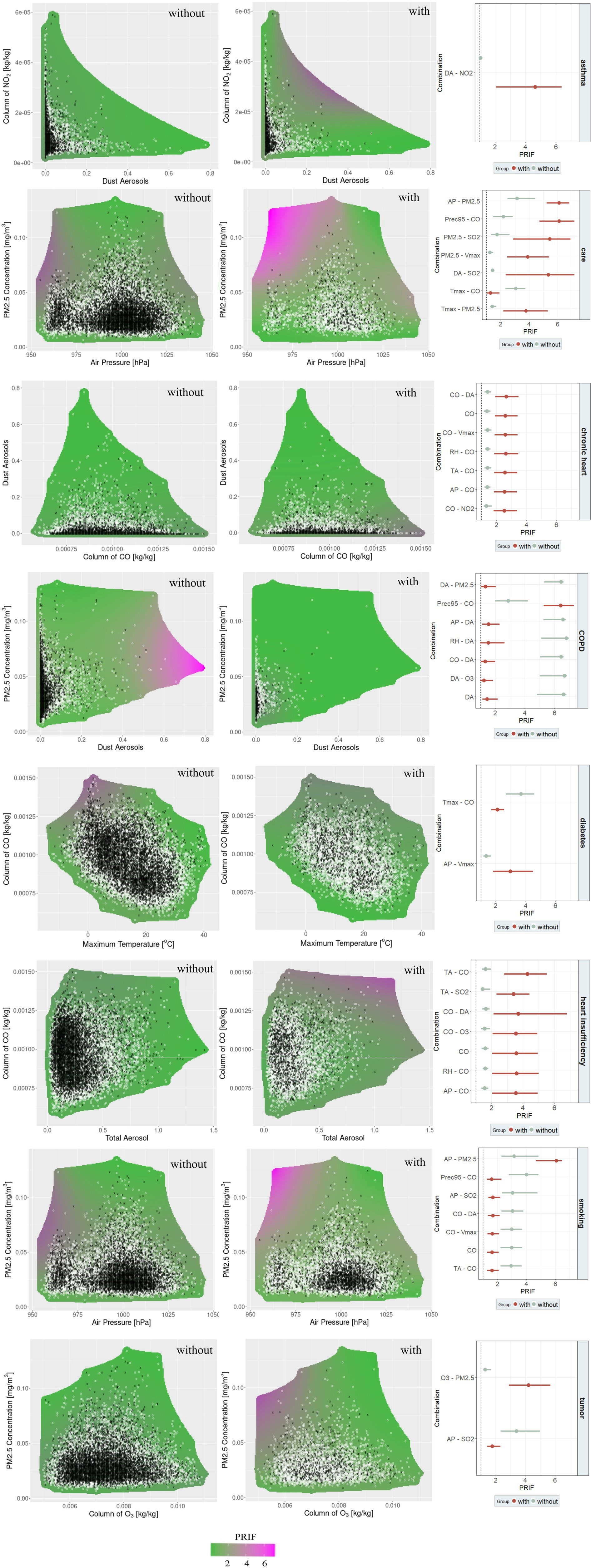
Figure 2. Highest two-dimensional PRIF figures for sub-groups with significant differences and highest lower confidence boundary and coefficient plot for significant differences between sub-groups selected comorbidities: asthma, care, chronic heart disease, COPD, diabetes, heart insufficiency, smoking, and tumor (black x = real cases, white x = non-real cases).
3.1.2.1 Asthma
For people with asthma, we only find one combination that shows a significant difference between the subgroups, namely a combination of high levels of DA and high levels of NO2, leading to a significantly higher PRIF for people with asthma (2.06).
3.1.2.2 People in care
We find 20 combinations that show significant differences between the subgroups, all of them, except one, displaying higher PRIFs for people in care. The highest PRIF (5.23) is reached for low air pressure and high levels of PM2.5. For six out of the seven displayed weather combinations, people in care have higher PRIFs compared to those not living in care (Figure 2: second row, right column). The results show that there are various weather combinations in which people in care are more vulnerable. Among those are conditions that combine two of the following factors: high levels of PM2.5, high levels of SO2, high levels of DA, and low air pressures.
3.1.2.3 Chronic heart disease
For chronic heart disease, we determine seven combinations with significant differences between the subgroups, all of them containing CO. The difference between the various combinations of CO with other factors is relatively small, implying that the level of CO is the main contributing factor. High levels of CO increase the vulnerability of people with chronic heart disease, leading to a PRIF value of around 1.9.
3.1.2.4 COPD
COPD is a special case. In total, we found 16 combinations that fulfill our requirement for further examination. In twelve of these cases, people without COPD have a higher PRIF. The combination which leads to the highest PRIF in this group is found for people without COPD and high levels of DA and medium to high levels of PM2.5 (5.30). At the same time, we find that the combination of high precipitation and high levels of CO leads to a comparably high PRIF of 5.28 for people with COPD. All other cases with high PRIF values are also combinations of DA with other factors. Hence, we can state that high levels of DA make people without COPD highly vulnerable.
3.1.2.5 Diabetes
For diabetes, we find two combinations with significant differences between the subgroups. The highest PRIF value is found for a combination of lower temperatures around 0°C and high levels of CO, which leads to a PRIF of 2.69. This holds for people without diabetes, meaning that people with diabetes are less vulnerable to low temperatures and high CO conditions. In contrast, people with diabetes are more susceptible to conditions with low air pressure and high wind speeds.
3.1.2.6 Heart insufficiency
For heart insufficiency, we find eleven combinations that display significant differences between the subgroups, all of them with higher PRIF values for people with heart insufficiency. All cases with PRIF values above 2 include CO, highlighting the importance of CO in the context of heart insufficiency. The combination which leads to the highest PRIF is high levels of TA and high levels of CO (2.80).
3.1.2.7 Smoking
For smoking, we found eleven combinations with significant differences between the subgroups, and only one of them has a higher PRIF for people who smoke compared to those who do not smoke. However, this combination leads to the highest values of the PRIF (4.68), implying a strong vulnerability of smoking people to one specific situation: low levels of air pressure and high levels of PM2.5. At the same time, people who smoke seem to be less vulnerable to high levels of CO.
3.1.2.8 Tumor
For tumor, we find two combinations with significant differences between the subgroups. The stronger difference is found for low levels of O3 and high levels of PM2.5, leading to higher PRIF values (2.90) for people with a tumor. The statistically not as strong combination is found for low air pressure and high levels of PM2.5, where people with a tumor seem to be less vulnerable.
4 Discussion
We found that people with particular personal characteristics (old, male, and high BMI), as well as people with comorbidities, tend to be more vulnerable to weather impacts in the context of CAP compared to people without comorbidities. The only comorbidities where we find mixed results are COPD, diabetes, and smoking. The highest 2.5% percentile of the PRIF for people with comorbidities is found for people with COPD (5.28), followed by people who are in care (5.23). The personal characteristics that lead to the highest PRIFs are a high BMI (4.02), old age (3.86), and male sex (3.74).
These findings are in accordance with the literature (Basu and Samet, 2002; Jin et al., 2022; Sarmadi et al., 2021). People with a high BMI might be particularly susceptible to weather impacts on CAP due to several factors. Firstly, obesity is often associated with compromised respiratory physiology, such as reduced lung capacity and impaired ventilation-perfusion matching (Littleton, 2012). Secondly, obesity is linked to chronic inflammation and altered immune function, which may weaken the body’s ability to fight off infections (Martí et al., 2001). Thirdly, obesity can lead to conditions such as obstructive sleep apnea, which further exacerbate respiratory issues and increase the risk of CAP (Gami et al., 2003). Older individuals are more susceptible to weather impacts on CAP due to a weaker immune system as well as reduced physiological resilience (Jin et al., 2022). Aging can reduce the body’s ability to regulate temperature and respond to environmental stressors, making older adults more vulnerable to high pollution levels (e.g., CO), which can increase the risk of pneumonia (Lin et al., 2009). Males might be more vulnerable in the CAP context due to occupational exposures such as outdoor labor as well as health-seeking behavior (Brucker et al., 2020). Studies have shown that males are often less likely to seek medical care promptly compared to females, resulting in delayed treatment and increased hospitalizations (Abdullah et al., 2022). However, we also find situations that females react more sensitively to. Hence, examining whether different lifestyles impact the vulnerability to weather and air conditions would be interesting. Finally, individuals with obesity and older age may have additional comorbidities such as diabetes and cardiovascular disease, which can further predispose them to respiratory infections and worsen the severity of CAP (Apovian, 2016).
However, our study goes beyond the basic assessment of sex, age, and BMI by also focusing on the role of multiple comorbidities in the context of CAP and weather. People in care, such as nursing homes or assisted living facilities, are often more vulnerable to weather impacts on pneumonia because they are typically older and have weaker immune systems, making them more susceptible to infections (Lin et al., 2009). Additionally, they may have limited mobility and be unable to avoid adverse weather conditions, which can exacerbate respiratory issues. Care facilities might also face challenges in maintaining optimal indoor air quality and temperature during extreme weather, further increasing the risk of pneumonia among residents. The highest 2.5% percentile of PRIFs is reached for people with COPD. This can be linked to factors such as weakened ventilatory strength, impaired mucociliary clearance, altered immune response and lung microbiome, increased inflammation, coexisting conditions, and medication (Almirall et al., 2017). COPD is characterized by airway inflammation and narrowing, creating an environment favorable to pathogens (Yende et al., 2008). This impairs lung function, making it harder to clear respiratory secretions and increasing infection risk. Additionally, weakened cilia reduce the body’s ability to defend against pathogens (Yaghi et al., 2012). Medications for COPD management, like corticosteroids, can further increase infection risk (Paul et al., 2015).
While people with COPD are highly vulnerable to conditions with high precipitation and high levels of CO, people without COPD have higher PRIFs for high levels of DA. This can be the case because people with COPD are usually more vigilant about monitoring their health and avoiding extreme weather conditions, thereby reducing their exposure to factors that could exacerbate pneumonia (Saeed et al., 2021). Regular medical check-ups and early interventions in COPD patients might lead to prompt treatment of pneumonia, mitigating severe outcomes (Newham et al., 2017). Simultaneously, they tend to be more likely to receive vaccinations for influenza and pneumococcal infections, offering additional protection against respiratory illnesses. For the smoking group, smokers are exposed to high CO levels anyhow, so that external high concentrations of CO in the air do not lead to a strong difference in total exposure for people who smoke (Strasser et al., 2007). Therefore, we also do not find a statistical difference.
The two air quality conditions that contribute the most to the increased risk of CAP hospitalizations are CO and PM2.5. These pollutants are generated predominantly by human activities like vehicle emissions, industrial processes, and combustion of fossil fuels, alongside natural phenomena such as volcanic eruptions and wildfires (Tucker, 2000; World Health Organization, 2021). Pollutants can impact respiratory health through respiratory irritation and potentially weaken the immune response, thereby increasing susceptibility to respiratory infections like pneumonia, particularly in vulnerable populations (Tasci et al., 2018). Due to the small size of PM2.5 aerosols, they can deeply penetrate into the lungs and induce inflammation in the respiratory tract by acting as carriers for harmful substances and pathogens, weakening the immune systems and sometimes even leading to immunosuppression (Horne et al., 2018). If respiratory function is already reduced, it is easier for air pollutants such as PM2.5 to penetrate the lungs and cause damage (Horne et al., 2018). CO is particularly harmful in driving negative impacts on pneumonia cases due to its ability to bind with hemoglobin, reducing the blood’s oxygen-carrying capacity and causing tissue hypoxia, which impairs immune function and respiratory response (Kavakli et al., 2011). CO exposure exacerbates pre-existing respiratory conditions like asthma and COPD. These mechanisms make CO’s impact on pneumonia more direct and severe compared to other pollutants.
At the same time, meteorological conditions can also impact the concentrations of these pollutants in the atmosphere by altering combustion processes, dispersion, and transport of pollutants. Furthermore, low atmospheric pressure can worsen respiratory symptoms by reducing oxygen availability. Higher air pressure may exacerbate air pollution levels by trapping pollutants closer to the ground, which can contribute to respiratory problems and increase the likelihood of hospital admissions for respiratory issues (Liu et al., 2020). During colder temperatures, individuals may spend more time indoors, which increases their exposure to indoor pollutants that can exacerbate respiratory issues (D'Amato et al., 2018). Simultaneously, cold temperatures can weaken the immune response of the respiratory system, making individuals more vulnerable to infections (Eccles and Wilkinson, 2015).
The twofold PRIF figures display that not many real cases tend to lie in the high PRIF areas (magenta). The relatively low frequency of pneumonia-contributing conditions in Germany can contribute to the low number of real cases in the high PRIF areas. However, it is plausible that climate change will lead to more frequent and intense pneumonia-contributing conditions in the near future. This includes the heightened risk of compound effects, such as arid and hot summers (Zscheischler and Fischer, 2020). The combination of rising temperatures and humidity may approach critical levels for survivability by the end of the twenty-first century (Marx et al., 2021). Despite improvements in air quality due to mitigation measures, high levels of PM2.5 and NO2 persist, especially in urban areas (Chau and Wang, 2020). More frequent climate extremes like droughts and heatwaves could increase the occurrence of wildfires in Germany, leading to a decline in air quality in affected areas due to smoke plumes (Dupuy et al., 2020; Kaskaoutis et al., 2024). Additionally, rising temperatures may elevate tropospheric ozone concentrations during droughts and heatwaves, further exacerbating respiratory risks (Dewan and Lakhani, 2022). The interplay between future climate extremes and air quality is anticipated to create conditions more conducive to pneumonia.
Hence, further research is necessary to ascertain whether individuals with multiple comorbidities exhibit greater susceptibility to weather-related impacts on CAP compared to those with only one comorbidity. It would also be beneficial to explore the combination of specific personal characteristics and comorbidities further to better identify individuals most susceptible to pneumonia-contributing conditions in greater detail. Additionally, to gain a deeper understanding of why individuals with specific comorbidities and personal characteristics are particularly vulnerable to weather-related impacts on CAP, several research approaches should be considered: (i) mechanistic studies (investigating the physiological mechanisms underlying the interaction between comorbidties and/or personal characteristics), (ii) epidemiological analyses (analyzing large-scale epidemiological datasets to assess the prevalence and incidence of CAP), and (iii) intervention studies (implementing intervention studies to evaluate the effectiveness of preventive measures, such as improved management of COPD and weight management strategies, in mitigating the impact of weather on CAP risk in this vulnerable population).
5 Conclusion
We show that people with particular personal characteristics (old, male, and high BMI), as well as people with comorbidities, are more vulnerable to weather impacts in the context of CAP compared to people without comorbidities. The personal characteristic leading to the highest pneumonia risk increase factor is a high BMI, with a PRIF of 4.02. The comorbidity that leads to the highest pneumonia risk increase is COPD, with a PRIF of 5.28, and people in care with a PRIF of 5.23. Air pollutants, specifically CO and PM2.5, play a major role in driving increased CAP hospitalizations. For meteorological conditions, air pressure and lower temperatures combined with air pollutants lead to high PRIFs. Our results underscore the heightened vulnerability to weather-related CAP among individuals with specific personal traits and comorbidities. Through this research, we have gained valuable insights into identifying at-risk groups and the specific weather conditions that significantly impact CAP admissions—information that has been largely absent in previous studies. These insights are crucial for implementing protective measures for at-risk populations and aiding healthcare providers and health policymakers in resource allocation during periods of pneumonia-contributing weather conditions. Hence, our results serve as a framework for guiding tailored and precise preventive measures during weather conditions conducive to pneumonia. These interventions target risk reduction, especially among the most susceptible individuals, thus enhancing pneumonia prevention and healthcare planning efficacy.
Data availability statement
The data utilized in this study pertain to sensitive patient health information and, therefore, cannot be made publicly available to ensure confidentiality and compliance with ethical standards. However, the R-script used for analysis and processing of the data is available upon request. Researchers interested in accessing the script for replication or further investigation may contact Thomas Brenner (dGhvbWFzLmJyZW5uZXJAdW5pLW1hcmJ1cmcuZGU=) to obtain a copy. We remain committed to transparency and reproducibility in research while upholding the privacy and confidentiality of the individuals involved.
Ethics statement
The studies involving humans were approved by the Hannover Medical School, Ethics Approval Number Nr.301–2008. The studies were conducted in accordance with the local legislation and institutional requirements. The participants provided their written informed consent to participate in this study. Written informed consent was obtained from the individual(s) for the publication of any potentially identifiable images or data included in this article.
Author contributions
TB: Writing – original draft, Writing – review & editing. A-CL: Writing – original draft, Writing – review & editing. SK: Writing – review & editing. CR: Data curation, Writing – review & editing. JB: Writing – review & editing. MK: Writing – review & editing. BW: Writing – review & editing. HP: Writing – review & editing. JR: Writing – review & editing. MW: Writing – review & editing. GR: Writing – review & editing. MP: Writing – review & editing. WB: Writing – review & editing. BS: Writing – review & editing.
Funding
The author(s) declare that financial support was received for the research, authorship, and/or publication of this article. The medical data set used in this study comes from the prospective cohort CAPNETZ study funded by the German Federal Ministry of Education and Research (registered under study NCT 02139,163). Open Access funding provided by the Open Access Publishing Fund of Philipps-Universität Marburg.
Conflict of interest
The authors declare that the research was conducted in the absence of any commercial or financial relationships that could be construed as a potential conflict of interest.
Publisher’s note
All claims expressed in this article are solely those of the authors and do not necessarily represent those of their affiliated organizations, or those of the publisher, the editors and the reviewers. Any product that may be evaluated in this article, or claim that may be made by its manufacturer, is not guaranteed or endorsed by the publisher.
Supplementary material
The Supplementary material for this article can be found online at: https://www.frontiersin.org/articles/10.3389/fclim.2024.1475075/full#supplementary-material
Abbreviations
AIC, Akaike Information Criterion; AP, Air pressure; CAP, Community-acquired pneumonia; CO, Carbon monoxide; COPD, Chronic Obstructive Pulmonary Disease; DA, Dust Aerosols; DWD, German Weather Service (Deutscher Wetterdienst); ECMWF, European Centre for Medium-Range Weather Forecasts; NO2, Nitrogen dioxide; SO2, Sulfur dioxide; O3, Ozone; PM, Particulate matter; SRTM, Shuttle Radar Topography Mission; TA, Total aerosols.
References
Abdullah, N. N., Mohd Arsat, M. H., Aziz, N. R. A., and Al-Kubaisy, W. (2022). Men health seeking behaviour: a literature review. Environ. Behav. Proceed. J. 7, 247–254. doi: 10.21834/ebpj.v7i20.3484
Adegboye, O. A., McBryde, E. S., and Eisen, D. P. (2020). Epidemiological analysis of association between lagged meteorological variables and pneumonia in wet-dry tropical North Australia, 2006-2016. J. Expo. Sci. Environ. Epidemiol. 30, 448–458. doi: 10.1038/s41370-019-0176-8
Almirall, J., Serra-Prat, M., Bolíbar, I., and Balasso, V. (2017). Risk factors for community-acquired pneumonia in adults: a systematic review of observational studies. Respiration 94, 299–311. doi: 10.1159/000479089
Apovian, C. M. (2016). Obesity: definition, comorbidities, causes, and burden. Available at: http://ajmc.s3.amazonaws.com/_media/_pdf/ace0042_05_2016_obesity_article01.pdf (Accessed August 07, 2024).
Baker, R. E., Mahmud, A. S., Wagner, C. E., Yang, W., Pitzer, V. E., Viboud, C., et al. (2019). Epidemic dynamics of respiratory syncytial virus in current and future climates. Nat. Commun. 10:5512. doi: 10.1038/s41467-019-13562-y
Basu, R., and Samet, J. M. (2002). Relation between elevated ambient temperature and mortality: a review of the epidemiologic evidence. Epidemiol. Rev. 24, 190–202. doi: 10.1093/epirev/mxf007
Behrendt, J., Penda, E., Finkler, A., Heil, U., and Polte-Rudolf, C. (2011) Beschreibung der Datenbasis des NKDZ. Volume no. 3.5.
Bollmeyer, C., Keller, J. D., Ohlwein, C., Wahl, S., Crewell, S., Friederichs, P., et al. (2015). Towards a high-resolution regional reanalysis for the European CORDEX domain. Q. J. R. Meteorol. Soc. 141, 1–15. doi: 10.1002/qj.2486
Braga, A. L. F., Zanobetti, A., and Schwartz, J. (2002). The effect of weather on respiratory and cardiovascular deaths in 12 U.S. cities. Environ. Health Perspect. 110, 859–863. doi: 10.1289/ehp.02110859
Brenner, T., Link, A. C., Reudenbach, C., Pott, H., Rupp, J., Witzenrath, M., et al. (2022). Effects of regional meteorological and air conditions on community-acquired pneumonia: Examining the interaction of individual, meteorological, and air characteristics (No. 01.22). Working Papers on Innovation and Space.
Brucker, N., do Nascimento, S. N., Bernardini, L., Charão, M. F., and Garcia, S. C. (2020). Biomarkers of exposure, effect, and susceptibility in occupational exposure to traffic-related air pollution: a review. J. Appl. Toxicol. 40, 722–736. doi: 10.1002/jat.3940
Chau, T.-T., and Wang, K.-Y. (2020). An association between air pollution and daily most frequently visits of eighteen outpatient diseases in an industrial city. Sci. Rep. 10:2321. doi: 10.1038/s41598-020-58721-0
Cillóniz, C., Polverino, E., Ewig, S., Aliberti, S., Gabarrús, A., Menéndez, R., et al. (2013). Impact of age and comorbidity on cause and outcome in community-acquired pneumonia. Chest 144, 999–1007. doi: 10.1378/chest.13-0062
Copernicus Climate Change Service (2020). Extreme precipitation risk indicators for Europe and European cities from 1950 to 2019. Available at: https://cds.climate.copernicus.eu/datasets/sis-european-risk-extreme-precipitationindicators?tab=download. doi: 10.24381/cds.3a9c4f89 (Accessed August 07, 2024).
D'Amato, M., Molino, A., Calabrese, G., Cecchi, L., Annesi-Maesano, I., and D'Amato, G. (2018). The impact of cold on the respiratory tract and its consequences to respiratory health. Clin. Transl. Allergy 8:20. doi: 10.1186/s13601-018-0208-9
Deb, A., Podmore, B., Barnett, R., Beier, D., Galetzka, W., Qizilbash, N., et al. (2022). Clinical and economic burden of pneumococcal disease among individuals aged 16 years and older in Germany. Epidemiol. Infect. 150:e204. doi: 10.1017/S0950268822001182
Dewan, S., and Lakhani, A. (2022). Tropospheric ozone and its natural precursors impacted by climatic changes in emission and dynamics. Front. Environ. Sci. 10:1007942. doi: 10.3389/fenvs.2022.1007942
Dong, J., Yang, R., Zhai, G., Wang, J., and Bao, H. (2021). Risks of hospital outpatient visits for overall and cause-specific respiratory disease associated with particulate matter pollution in Lanzhou, China. Air Qual. Atmos. Health 14, 1405–1415. doi: 10.1007/s11869-021-01030-w
Dupuy, J., Fargeon, H., Martin-StPaul, N., Pimont, F., Ruffault, J., Guijarro, M., et al. (2020). Climate change impact on future wildfire danger and activity in southern Europe: a review. Ann. For. Sci. 77, 1–24. doi: 10.1007/s13595-020-00933-5
DWD Climate Data Center (2021). Historische tägliche Stationsbeobachtungen (Temperatur, Druck, Niederschlag, Sonnenscheindauer, etc.) für Deutschland. Version v21.3, 2021.
Eccles, R., and Wilkinson, J. E. (2015). Exposure to cold and acute upper respiratory tract infection. Rhinology 53, 99–106. doi: 10.4193/Rhino14.239
Europäische Kommission - Eurostat/GISCO (2022). Administrative units, Statistical units (2016). Available at https://ec.europa.eu/eurostat/web/gisco/geodata/administrative-units/communes
Falagas, M. E., Theocharis, G., Spanos, A., Vlara, L. A., Issaris, E. A., Panos, G., et al. (2008). Effect of meteorological variables on the incidence of respiratory tract infections. Respir. Med. 102, 733–737. doi: 10.1016/j.rmed.2007.12.010
Fan, X., Jie, X., Zou, F., Wang, D., Da, H., Li, H., et al. (2024). Association between outdoor air pollutants and risk of acute exacerbation of chronic obstructive pulmonary disease in Xi’an, China. Air Qual. Atmos. Health 17, 1373–1390. doi: 10.1007/s11869-024-01513-6
Gami, A. S., Caples, S. M., and Somers, V. K. (2003). Obesity and obstructive sleep apnea. Endocrinol. Metab. Clin. N. Am. 32, 869–894. doi: 10.1016/S0889-8529(03)00069-0
Hespanhol, V., and Bárbara, C. (2020). Pneumonia mortality, comorbidities matter? Pulmonology 26, 123–129. doi: 10.1016/j.pulmoe.2019.10.003
Hoffmann, C., Maglakelidze, M., Schneidemesser, E.Von, Witt, C., Hoffmann, P., and Butler, T. (2022). Asthma and COPD exacerbation in relation to outdoor air pollution in the metropolitan area of Berlin, Germany, Respir. Res. 23:64. doi: 10.1186/s12931-022-01983-1
Horne, B. D., Joy, E. A., Hofmann, M. G., Gesteland, P. H., Cannon, J. B., Lefler, J. S., et al. (2018). Short-term elevation of Fine particulate matter air pollution and acute lower respiratory infection. Am. J. Respir. Crit. Care Med. 198, 759–766. doi: 10.1164/rccm.201709-1883OC
Hosmer, D. W., Lemeshow, S., and Sturdivant, R. X. (2013). Applied logistic regression third edition: David W. Hosmer, Jr., Stanley Lemeshow, Rodney X. Sturdivant. Hoboken, New Jersey: Wiley.
Hwang, H., Jang, J.-H., Lee, E., Park, H.-S., and Lee, J. Y. (2023). Prediction of the number of asthma patients using environmental factors based on deep learning algorithms. Respir. Res. 24:302. doi: 10.1186/s12931-023-02616-x
Inness, A., Ades, M., Agustí-Panareda, A., Barré, J., Benedictow, A., Blechschmidt, A.-M., et al. (2019). The CAMS reanalysis of atmospheric composition. Atmos. Chem. Phys. 19, 3515–3556. doi: 10.5194/acp-19-3515-2019
Institut für Qualitätssicherung (2020) Ambulant erworbene Pneumonie - Qualitätsindikatoren und Kennzahlen Stand. Available at: https://iqtig.org/downloads/auswertung/2019/pneu/QSKH_PNEU_2019_BUAW_V02_2020-07-14.pdf (Accessed 12 November 2023).
Jin, L., Zhou, T., Fang, S., Zhou, X., Han, B., and Bai, Y. (2022). The short-term effects of air pollutants on pneumonia hospital admissions in Lanzhou, China, 2014–2019: evidence of ecological time-series study. Air Qual. Atmos. Health 15, 2199–2213. doi: 10.1007/s11869-022-01244-6
Kaskaoutis, D. G., Petrinoli, K., Grivas, G., Kalkavouras, P., Tsagkaraki, M., Tavernaraki, K., et al. (2024). Impact of peri-urban forest fires on air quality and aerosol optical and chemical properties: the case of the august 2021 wildfires in Athens, Greece. Sci. Total Environ. 907:168028. doi: 10.1016/j.scitotenv.2023.168028
Kavakli, H. S., Erel, O., Delice, O., Gormez, G., Isikoglu, S., and Tanriverdi, F. (2011). Oxidative stress increases in carbon monoxide poisoning patients. Hum. Exp. Toxicol. 30, 160–164. doi: 10.1177/0960327110388539
Kyu, H. H. (2022). Age–sex differences in the global burden of lower respiratory infections and risk factors, 1990–2019: results from the global burden of disease study 2019. Lancet Infect. Dis. 22, 1626–1647. doi: 10.1016/S1473-3099(22)00510-2
Li, M., Zhou, M., Yang, J., Yin, P., Wang, B., and Liu, Q. (2019). Temperature, temperature extremes, and cause-specific respiratory mortality in China: a multi-city time series analysis. Air Qual. Atmos. Health 12, 539–548. doi: 10.1007/s11869-019-00670-3
Lin, H.-C., Lin, C.-C., Chen, C.-S., and Lin, H.-C. (2009). Seasonality of pneumonia admissions and its association with climate: an eight-year nationwide population-based study. Chronobiol. Int. 26, 1647–1659. doi: 10.3109/07420520903520673
Littleton, S. W. (2012). Impact of obesity on respiratory function. Respirology 17, 43–49. doi: 10.1111/j.1440-1843.2011.02096.x
Liu, Y., Zhou, Y., and Lu, J. (2020). Exploring the relationship between air pollution and meteorological conditions in China under environmental governance. Sci. Rep. 10:14518. doi: 10.1038/s41598-020-71338-7
Luna, C. M., Palma, I., Niederman, M. S., Membriani, E., Giovini, V., Wiemken, T. L., et al. (2016). The impact of age and comorbidities on the mortality of patients of different age groups admitted with community-acquired pneumonia. Ann. Am. Thorac. Soc. 13, 1519–1526. doi: 10.1513/AnnalsATS.201512-848OC
Martí, A., Marcos, A., and Martínez, J. A. (2001). Obesity and immune function relationships. Obes. Rev. 2, 131–140. doi: 10.1046/j.1467-789x.2001.00025.x
Marx, W., Haunschild, R., and Bornmann, L. (2021). Heat waves: a hot topic in climate change research. Theor. Appl. Climatol. 146, 781–800. doi: 10.1007/s00704-021-03758-y
Molinos, L., Clemente, M. G., Miranda, B., Alvarez, C., Del Busto, B., Cocina, B. R., et al. (2009). Community-acquired pneumonia in patients with and without chronic obstructive pulmonary disease. J. Infect. 58, 417–424. doi: 10.1016/j.jinf.2009.03.003
Nascimento-Carvalho, C. M., Cardoso, M.-R. A., Barral, A., Araújo-Neto, C. A., Oliveira, J. R., Sobral, L. S., et al. (2010). Seasonal patterns of viral and bacterial infections among children hospitalized with community-acquired pneumonia in a tropical region. Scand. J. Infect. Dis. 42, 839–844. doi: 10.3109/00365548.2010.498020
Newham, J., Presseau, J., Heslop-Marshall, K., Russell, S., Ogunbayo, O., Netts, P., et al. (2017). Features of self-management interventions for people with COPD associated with improved health-related quality of life and reduced emergency department visits: a systematic review and meta-analysis. Int. J. Chron. Obstruct. Pulmon. Dis. 12, 1705–1720. doi: 10.2147/COPD.S133317
Nhung, N. T. T., Amini, H., Schindler, C., Kutlar Joss, M., Dien, T. M., Probst-Hensch, N., et al. (2017). Short-term association between ambient air pollution and pneumonia in children: a systematic review and meta-analysis of time-series and case-crossover studies. Environ. Poll. 230, 1000–1008. doi: 10.1016/j.envpol.2017.07.063
Paul, K. J., Walker, R. L., and Dublin, S. (2015). Anticholinergic medications and risk of community-acquired pneumonia in elderly adults: a population-based case-control study. J. Am. Geriatr. Soc. 63, 476–485. doi: 10.1111/jgs.13327
Pedder, H., Kapwata, T., Howard, G., Naidoo, R. N., Kunene, Z., Morris, R. W., et al. (2021). Lagged association between climate variables and hospital admissions for pneumonia in South Africa. Int. J. Environ. Res. Public Health 18:6191. doi: 10.3390/ijerph18126191
Ruchiraset, A., and Tantrakarnapa, K. (2022). Association of climate factors and air pollutants with pneumonia incidence in Lampang province, Thailand: findings from a 12-year longitudinal study. Int. J. Environ. Health Res. 32, 691–700. doi: 10.1080/09603123.2020.1793919
Saeed, S., Awasthi, A. A., Nandi, D., Kaur, K., Hasan, S., and Janardhanan, R. (2021). Knowledge, attitude and practice towards COVID-19 among individuals with associated comorbidities. J. Med. Life 14, 225–237. doi: 10.25122/jml-2020-0184
Sarmadi, M., Moghanddam, V. K., Dickerson, A. S., and Martelletti, L. (2021). Association of COVID-19 distribution with air quality, sociodemographic factors, and comorbidities: an ecological study of US states. Air Qual. Atmos. Health 14, 455–465. doi: 10.1007/s11869-020-00949-w
Strasser, A. A., Lerman, C., Sanborn, P. M., Pickworth, W. B., and Feldman, E. A. (2007). New lower nicotine cigarettes can produce compensatory smoking and increased carbon monoxide exposure. Drug Alcohol Depend. 86, 294–300. doi: 10.1016/j.drugalcdep.2006.06.017
Tasci, S. S., Kavalci, C., and Kayipmaz, A. E. (2018). Relationship of meteorological and air pollution parameters with pneumonia in elderly patients. Emerg. Med. Int. 2018:4183203. doi: 10.1155/2018/4183203
Theilacker, C., Sprenger, R., Leverkus, F., Walker, J., Häckl, D., Eiff, C. von, et al. (2021). Population-based incidence and mortality of community-acquired pneumonia in Germany. PLoS One 16:e0253118. doi: 10.1371/journal.pone.0253118
Tucker, W. (2000). An overview of PM2.5 sources and control strategies. Fuel Process. Technol. 65-66, 379–392. doi: 10.1016/S0378-3820(99)00105-8
Vicedo-Cabrera, A. M., Melén, E., Forastiere, F., Gehring, U., Katsouyanni, K., Yorgancioglu, A., et al. (2023). Climate change and respiratory health: a European Respiratory Society position statement. Eur. Respir. J. 62:2201960. doi: 10.1183/13993003.01960-2022
Welte, T., Suttorp, N., and Marre, R. (2004). CAPNETZ-community-acquired pneumonia competence network. Infection 32, 234–238. doi: 10.1007/s15010-004-3107-z
World Health Organization (2021). WHO global air quality guidelines: Particulate matter (PM2.5 and PM10), ozone, nitrogen dioxide, sulfur dioxide and carbon monoxide. Bonn, Germany: WHO European Centre for Environment and Health.
Wu, J., Wu, Y., Wu, Y., Yang, R., Yu, H., Wen, B., et al. (2024). The impact of heat waves and cold spells on pneumonia risk: a nationwide study. Environ. Res. 245:117958. doi: 10.1016/j.envres.2023.117958
Yaghi, A., Zaman, A., Cox, G., and Dolovich, M. B. (2012). Ciliary beating is depressed in nasal cilia from chronic obstructive pulmonary disease subjects. Respir. Med. 106, 1139–1147. doi: 10.1016/j.rmed.2012.04.001
Yende, S., D'Angelo, G., Kellum, J. A., Weissfeld, L., Fine, J., Welch, R. D., et al. (2008). Inflammatory markers at hospital discharge predict subsequent mortality after pneumonia and sepsis. Am. J. Respir. Crit. Care Med. 177, 1242–1247. doi: 10.1164/rccm.200712-1777OC
Zhang, R., Meng, Y., Song, H., Niu, R., Wang, Y., Li, Y., et al. (2021b). The modification effect of temperature on the relationship between air pollutants and daily incidence of influenza in Ningbo, China. Respir. Res. 22:153. doi: 10.1186/s12931-021-01744-6
Zhang, J., Ren, D., Cao, X., Wang, T., Geng, X., Li, X., et al. (2021a). Ambient air pollutants and hospital visits for pneumonia: a case-crossover study in Qingdao, China. BMC Public Health 21:66. doi: 10.1186/s12889-020-10065-0
Keywords: pneumonia, weather, air quality, comorbidities, characteristics, Germany
Citation: Brenner T, Link A-C, Khan SA, Reudenbach C, Bendix J, Kutzinski M, Weckler BC, Pott H, Rupp J, Witzenrath M, Rohde G, Pletz MW, Bertrams W and Schmeck B (2024) Impact of comorbidities and personal characteristics on weather-related risk for community-acquired pneumonia. Front. Clim. 6:1475075. doi: 10.3389/fclim.2024.1475075
Edited by:
Happy Mathew Tirivangasi, University of Jyväskylä, FinlandReviewed by:
Harry César Kayembe, University of Kinshasa, Democratic Republic of CongoLeonard Chitongo, Marondera University of Agricultural Sciences and Technology (MUAST), Zimbabwe
Copyright © 2024 Brenner, Link, Khan, Reudenbach, Bendix, Kutzinski, Weckler, Pott, Rupp, Witzenrath, Rohde, Pletz, Bertrams and Schmeck. This is an open-access article distributed under the terms of the Creative Commons Attribution License (CC BY). The use, distribution or reproduction in other forums is permitted, provided the original author(s) and the copyright owner(s) are credited and that the original publication in this journal is cited, in accordance with accepted academic practice. No use, distribution or reproduction is permitted which does not comply with these terms.
*Correspondence: Ann-Christine Link, YW5uLWNocmlzdGluZS5saW5rQHVuaS1tYXJidXJnLmRl
†These authors share first authorship