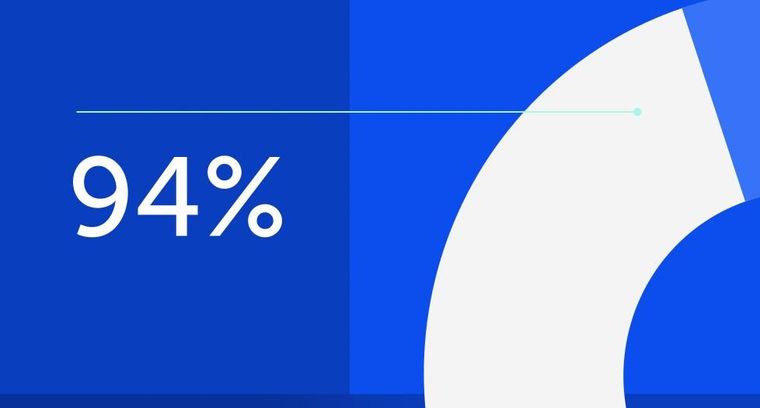
94% of researchers rate our articles as excellent or good
Learn more about the work of our research integrity team to safeguard the quality of each article we publish.
Find out more
ORIGINAL RESEARCH article
Front. Clim., 24 October 2024
Sec. Climate Adaptation
Volume 6 - 2024 | https://doi.org/10.3389/fclim.2024.1458999
This article is part of the Research TopicSustainable Cropping Systems for Small Grain Crops for Smallholder FarmersView all 5 articles
The study assesses how access to climate services influences cropping decisions and, subsequently, household food security. Employing a cross-sectional research design, the study surveyed a total of 217 smallholder crop farmers selected through a multistage sampling approach. Data were collected using a semi-structured questionnaire, and both descriptive statistics and a propensity score matching (PSM) model were employed for data analysis. Household food security was assessed using households' dietary diversity. The study's findings highlight that 69% of the surveyed farmers had access to weather forecasts, while only 24% had access to seasonal forecasts. Consequently, farmers with access to climate services exhibited changes in their farming decisions, including daily farming activities, planting timing, crop selection, and land preparation. Notably, approximately 35% of the respondents were identified as food insecure. The application of the propensity score matching model revealed that access to climate services had a statistically significant positive impact on farmers' decision-making processes and household food security. These results underscore the potential of climate services to enhance farmers' decision-making capabilities and, subsequently, improve household food security. Considering these findings, it is recommended that efforts to expand access to climate services among smallholder farmers in South Africa should be intensified. This can be achieved by developing and disseminating more accessible and tailored climate information, including seasonal forecasts. In addition, initiatives should ensure that climate services are integrated into agricultural extension services and support systems to equip farmers with the knowledge and tools they need to make informed decisions.
Extreme weather phenomena including droughts, floods, and windstorms are becoming more frequent and more intense due to the changes in climate (Sylla et al., 2018). Authors such as Toreti et al. (2020) noted that these occurrences lead to significant crop decreases in terms of both quantity and quality, often leading to crop failures. In smallholder crop farming, which is primarily rainfed, these crop losses and failures are already being felt (Calzadilla et al., 2014). According to Cudjoe et al. (2021), Asayehegn Gebreeyesus et al. (2017), and Idrisa et al. (2012), they are more felt in regions such as Africa where rainfed farmers with low adaptive capacity dominate. Kinda et al. (2019) assert that the effect of climate change, particularly due to rainfall variability, is more pronounced in developing nations. It is anticipated that these significant reductions in crop yields due to climate change will continue globally (FAO, 2016), particularly in Africa (Knox et al., 2012; Macauley and Ramadjita, 2015), and South Africa (Kogo et al., 2019), thus increasing food insecurity status. A study by FAO (2019) agrees that climatic variability and extremes are major contributors to the recent increase in serious food crises worldwide, through increased yield decline. A study by Kobusinge (2018) added that under the increasing climate change impacts, through extreme climate events, smallholder crop farmers from Africa are predicted to continue suffering from food insecurity. Sirba and Chimdessa (2021) noted that drought has caused Sub-Saharan Africa to be known as the world's most food-insecure region. According to FAO (2021), over a quarter of people (28.8%) in West Africa suffered from food insecurity in the past 3 years owing to the changing climate. Comparably, a recent study conducted in the Horn of Africa showed that there is a 0.36% increase in food insecurity status for every 1% rise in average temperature (Bedasa and Bedemo, 2023). This has also been experienced in Ghana, where the reduction in crop yields due to the 2015 El Nino drought event led to insufficient food for smallholder farmers (Owusu et al., 2019). In addition, because of persistent droughts that have reduced Lesotho's agricultural production, Bhaga et al. (2020) discovered that 30% of the population is anticipated to experience acute food insecurity. A report published by Statistics South Africa (2019) also reveals that food security has been and is still an issue among the population of South Africa. Similarly, Mdluli and Dunga (2022) added that in the past 2 years, an estimated number of 13.6 million people from South Africa were suffering from food insecurity. In addition, changes in climatic conditions have led to food insecurity among Eastern Cape smallholder farming families in South Africa (Amoah and Simatele, 2021). Amoah and Simatele (2021) further reported that toward the end and early months of the year, farmers tend to suffer from the impacts of climate change through increased droughts, heat waves, and floods that reduce the availability of food from their production. Thus, maintaining family food security under such disadvantaged conditions necessitates access to climate service information that will notify farmers about the start of seasonal rains and their absence throughout the production year (Murgor, 2015). It should be noted that in Africa, most smallholder farmers use indigenous knowledge to forecast weather conditions (Briggs, 2005; Zuma-Netshiukhwi, 2013; Antwi-Agyei et al., 2021). According to Nyamekye et al. (2019) and Gbangou et al. (2021), the indigenous knowledge system assists farmers in predicting weather conditions and seasons using various indicators such as the appearance of a certain plant or bird species. However, forecasting seasonal weather using solely indigenous knowledge is becoming unpredictable and unreliable (Elia et al., 2014; Myeni and Moeletsi, 2020). Hence, there is a need for access to climate service information to complement the use of indigenous knowledge systems.
Climate service is defined as a decision support tool developed from climatic information to enable people and organizations to make better pre-decisions (Tall, 2013). The services cover information on temperature, rainfall, wind speed, humidity, drought, and floods and are usually made available to users on a daily up to seasonal basis from various institutions using both media and human means such as radio, television, and extension services (Kadi et al., 2011). Moreover, the services may also include other useful information on farming practices such as those on pest and disease outbreaks and crop varieties (Nkiaka et al., 2019). In other words, climate services mean a tool that is created from climatic data aimed at notifying smallholder farmers on what to expect in the next few days, weeks, or months in terms of weather conditions so that they can make farming choices that are best suited for such conditions. Hansen et al. (2019) noted that seasonal climate forecasts and short-term weather forecasts are the two types of climate services that are often accessed and used by farmers. Seasonal climate forecasts are based on the probability that the rainfall, temperature, wind, and humidity are likely to be higher, lower, or normal for an upcoming season in a geographic region (Masesi, 2019; Ebhuoma, 2022). Seasonal forecasts generally show smallholder farmers what the next season may look like (Diouf et al., 2020). Weisheimer and Palmer (2014) argue that seasonal climate forecasts provide projections of how the weather will be on average during the season, 3 months in advance. On the other hand, short-term weather prediction is merely a scientific estimate of upcoming weather conditions for the next hours to days (Losloso et al., 2020). Short-term weather forecasts usually give detailed weather predictions such as the chance of rain and temperature for the next day (Murgor, 2015).
Authors such as Clarkson et al. (2019) attested that the usage of climate services positively influences smallholder crop farmers' confidence in making better farming choices to improve agricultural productivity, wellbeing, and coping with climate risks. In a study in Nigeria, farmers made daily management decisions regarding fertilizer application, land preparation, weed removal, rain and drought monitoring, and soil and water management, all informed by climate services (Abegunrin, 2021). Studies such as that of Muyiramye (2020) revealed that smallholder farmers in Rwanda used seasonal forecasts to determine which crop varieties to plant. Nonetheless, the literature is skewed on the evidence from the personal experience of smallholder farmers with access to and use of climate services and farming decision-making, with a lack of impact assessment studies. To the researcher's knowledge, at the time of research, there were no studies reviewed in the South African context that assessed the impact of climate services on cropping decisions using impact evaluation methods such as propensity score matching. Thus, it is unclear whether better agricultural choices made by smallholder farmers are informed by climate services or other confounding factors. The few available studies on climate services' effects on household food security status of smallholder farmers showed that in some African countries such as Rwanda, Namibia, Ghana, Uganda, and South Africa, climate service access and usage do improve their food security status (Birachi et al., 2020; Gitonga et al., 2020; Clarkson et al., 2019; Akwango et al., 2017; Zuma-Netshiukhwi et al., 2016). For example, propensity score matching (PSM) results indicated that Namibian smallholder farmers who accessed climate services had higher food security status than those who did not (Gitonga et al., 2020). Furthermore, increased food security status among households that used climate services has been reported also in South Africa (Zuma-Netshiukhwi et al., 2016).
Despite the literature indicating a positive contribution of obtaining and using climate services on smallholder farmers' household food security, not much has been done in the context of South Africa. In addition, from the reviewed articles, few studies focus on the impact of accessing climate services on food security (Gitonga et al., 2020; Mapanje et al., 2020), and most of them focus only on the impact of use (Zuma-Netshiukhwi et al., 2016; Akwango et al., 2017; Clarkson et al., 2019; Birachi et al., 2020). It is therefore upon this background that this study intends to understand the effect of receiving specific climate services on smallholder cropping decisions and household food security using impact evaluation methods.
The municipality that was studied was Elundini, which is a category B municipality of Joe Gqabi District in the northeastern part of the Eastern Cape, South Africa, as displayed in Figure 1. This municipality is the smallest within the district covering an area of 5,065 km2 and has 17 wards (Elundini Local Municipality, 2015). Maclear, Mount Fletcher, and Ugie are the main towns in this municipality. The area is home to the Xhosa population, and it is regarded to be among the vulnerable areas in terms of socio-economic status within the district (Joe Gqabi District Municipality, 2016). In terms of employment status, Elundini has a very small population that is formally employed especially in rural communities (ECSECC, 2017). It is recognized for its temperature variations ranging from 13°C to 33°C, with higher temperatures expected during summer and lower temperatures during winter (Maroyi, 2017). In a year, the area has 150 days of frost likely from March to November with Maclear and Mount Fletcher recording many winter snowfall incidents. The municipality is a high-rainfall region, receiving most of it during the summer months. The average rainfall is between 800 mm and 1,200 mm per annum (Maroyi, 2017). This high rainfall is largely visible in the eastern parts of the municipality. Furthermore, within the Joe Gqabi district, suitable soils for farming are mostly found in Elundini (Joe Gqabi District Municipality, 2016). Joe Gqabi District Municipality (2016) reports that Elundini is the only area with high agricultural potential within the Joe Gqabi district. It has a lot of natural resources and a good climate that makes it possible for households to engage in agriculture. Moreover, compared to other municipalities within the district, only in Elundini, there is a large population of smallholder crop growers (Joe Gqabi District Municipality, 2016; Elundini Local Municipality, 2020). According to Ngcaba and Maroyi (2021), most crop farmers in the municipality primarily cultivated maize, potatoes, spinach, carrots, cabbage, and beetroot. Nonetheless, climate change is affecting Elundini, especially in the eastern parts of the municipality through extreme events such as heavy rains, floods, storms, soil erosions, and land degradation (Elundini Local Municipality, 2015). This has affected crop production in terms of crop losses and increased pest incidences (Elundini Local Municipality, 2015). Morgan and Nearing (2010) state that climate change, particularly the increase in heavy rains and floods, often leads to soil erosion by separating soil particles from the surface. This results in poor soil quality and contributes to land degradation (Koch et al., 2013). Hermans and McLeman (2021) argued that extreme heat and drought events also influence soil erosion and land degradation. Furthermore, it has been observed that changes in climatic conditions in terms of heavy rains have major implications on soil erosion and land degradation (Nearing and Pruski, 2004). In addition, Ndlovu et al. (2020) agree that there is a positive correlation between climate variability and land degradation. Furthermore, crop production is vulnerable because the municipality of Elundini lacks a climate change response strategy (Elundini Local Municipality, 2020). According to the Elundini Local Municipality (2020) report, the municipality has acknowledged the necessity for developing a climate change response strategy. Therefore, farmers' access to climate services and their effect on their food security in Elundini are investigated.
Figure 1. Map of Elundini Local Municipality (2015). Source: Department of GIS, University of Fort Hare (2022).
The current study adopted a quantitative data method using a cross-sectional research design approach. To select respondents, a multistage sampling method was employed. The first step included purposive sampling, whereby Elundini municipality was purposively chosen as a study area. After selecting the municipality, two wards were purposively selected from each of the towns in Elundini. This is to ensure that every town within the municipality is represented, thereby maximizing the variation in the data as much as possible. Furthermore, a total of 12 villages were purposively selected to represent the municipality. This means two villages from each of the selected six wards. The final stage included random selection of 217 smallholder crop farmers from the villages. It should be noted that the targeted sample size calculated using Yamane's equation below was 370 smallholder crop farmers. However, due to farmers' accessibility and availability, the timing of the data collection, weather (thunderstorms, lightning, and hailstorms), and COVID-19 restrictions, only 217 smallholder crop farmers were successfully interviewed. The field survey was carried out using semi-structured questionnaires written in English but disseminated to farmers in Xhosa during the face-to-face interviews. The statements in the questionnaire were both in open-ended and close-ended formats. The study collected information directly from the smallholder crop farmers regarding their socio-economic and farm characteristics, awareness of, access to, and use of climate services, cropping decisions made, and food security status. Face-to-face interviews were conducted with smallholder crop farmers by 11 trained enumerators. The enumerators were from the University of Fort Hare and spoke Xhosa. In determining the appropriate size of the sample, Yamane (1967) equation was adopted. The equation is as follows:
where “n” represents the sample size, “N” represents the total number of crop farming households (sampling frame), and “e” is the error of margin (which is 0.05 with a confidence level of 95%). According to a Statistics South Africa (2016), the total number of crop farming households in Elundini was 4,730, which was used as a sampling frame.
The data analysis methods used in this study are explained below.
HDDS was used to measure smallholder crop farmers' food security. The choice of HDDs in this study is because the method is widely used in measuring the food consumption component, and there are no significant biases as smallholder crop farmers can recall what they ate the previous day (Kennedy et al., 2010). HDDS food items are grouped into 12 different categories, with each group contributing toward the household dietary score given that a food item within a food category was consumed in the last 24 h. These are “A—cereals; B—roots and tubers; C—vegetables; D—fruits; E—meat; F—eggs; G—fish and seafood; H—legumes and nuts; I—milk and milk products; J—oils and fats; K—sugar and honey; and L—miscellaneous” (Swindale and Bilinsky, 2006). A value of 1 was assigned to every single food group consumed and 0 if not, which means during data collection households, were either saying yes, they consumed food items within that food group or not. After that for each household, food items consumed were summed. Then based on their scores, the households were grouped into three categories where any household with a score that is 3 or less was regarded as a low dietary diversity household, a score between 4 and 6 was regarded as a medium, and a score that is 7 and above was regarded as a high dietary diversity household.
Following the study by Mapanje et al. (2020) and Gitonga et al. (2020), the current study employed PSM to control self-selection bias. PSM matches two groups of individuals who participated in an event with those who did not participate in an event but had similar propensity scores (Rubin, 2001). PSM also involves the removal of all the values of individuals who did not participate and have no similar propensity scores with any of the participating individuals (Lobut, 2017). According to Lobut (2017), one of the benefits of this method highlights which variable affects the likelihood of participation in a certain event. In addition, it guarantees that the matched groups are the same in all ways that can be seen and that the differences between the control and treated groups are not triggered by the things that can be seen (Adjin et al., 2020). The PSM method was adopted to assess the effect of weather and seasonal forecasts on cropping decisions informed by each type of forecast and household food security among smallholder crop farmers. The treatment variables used were access to weather or seasonal forecasts. The outcome variables were cropping decisions and household food security. In this analysis, farmers who made any cropping decision informed by weather or seasonal forecasts were assigned a value of 1, and those who did not were assigned a value of 0. The outcome variable in the assessment of the impact of climate services on food security was household dietary diversity scores, which is a continuous variable. Given that access to certain climate services is not random, but rather impacted by both observable and unobserved variables, or one of the variables, the binary probit models were employed in generating propensity scores. The model is represented as follows:
where f(Xi) indicates information that a farmer accessed climate services, (Di = 1) for farmers who accessed specific climate services, and ‘θ' represents the standard cumulative distribution function. Determinants (Xi) incorporate all observable factors as linear terms without higher-order components that influence farmers' household food security status and ability to access climate services. These observable factors include farmers' gender, education, and marital status. Every farmer who accessed specific climate services and those with no access in the sample have a propensity score computed, which is a continuous variable that may be written as follows:
For the matching of farmers who accessed to those with no access to specific climate services based on the propensity scores, the weight of every paired farmer with access to those with no access to specific climate services was estimated using nearest neighbor and Kernel-based matching methods. The choice of nearest neighbor matching (NNM) method among others such as radius and stratified matching methods was because NNM reduces the distance in propensity scores between the treatment unit and the closest units in the control group, thereby eliminating bias (Dehejia and Wahba, 2002). In addition, Caliendo and Kopeinig (2008) noted that NNM is the simplest matching method to use. On the other hand, the Kernel-based matching (KBM) method was chosen because it is a non-parametric matching estimator that creates the counterfactual outcome by averaging the weights of each member of the control group, leading to reduced variance as more data are used (Caliendo and Kopeinig, 2008). The mean difference in the results among farmers who accessed and those who did not for every stratum represents the influence of various climate services and is specified in the following way:
ADsq in Equation 4 represents the mean difference in block q, I(q) signify a group of components that form a bloc q; NTq and NCq represent the number of farmers that have access to climate services units in the block (q). According to the United Nations Development Program (2009), the estimator of the ATT is calculated by taking the mean of every AD, as shown in the equation below.
The total number of blocks is represented by ‘Q'. The counterfactual effect was created via the Kernel matching approach using weighted means of every member of farmers who did not access climate services. According to Caliendo and Kopeinig (2008), the gap between individuals in the do not have access and have access categories from which the counterfactual is derived was used to figure out the weights. Equation 6 below presents the Kernel matching ATT estimator.
where “G” is a parameter for bandwidth and Kernel function. The bandwidth parameter selection is more critical since it determines how well the estimated density function matches the actual underlying density function and how much it varies from the estimate. After matching and generating propensity scores, the average outcomes of smallholder crop farmers paired based on relevant characteristics such as their gender were evaluated. This is to see whether access to climate services statistically influenced the results.
Table 1 presents the descriptive statistics of the socio-economic characteristics of the respondents. The study results indicated that smallholder crop farmers in the study area are men (52%), married (51%), and unemployed (80%). Furthermore, the results in Table 1 indicated that most smallholder crop farmers in the study area were old, experienced in farming, with moderate household size, and farming on a very small piece of land. Moreover, the majority (94%) of the surveyed farmers were knowledgeable about the changes in climate and their impact on crop production. This could potentially make farmers seek adaptation strategies (Mandleni and Anim, 2011) such as weather and seasonal forecasts that can assist them in making better cropping decisions given the changes in climate conditions. Experienced old farmers are more aware of the negative impacts of climate change and, consequently, are more willing to access climate services in response to these challenges (Idoma and Mamman, 2016). Nonetheless, few crop smallholder farmers participated in farmers' organizations (29%); at the same time, they had limited access to government extension services (35%). Poor access to information hubs such as extension officers and farm organizations may hinder farmers' access to climate services (Buckland and Campbell, 2021). This is because, during agricultural meetings and workshops, extension agents in South Africa have the role of educating farmers about climate change and helping them adapt to it (Popoola et al., 2020), using various strategies, including climate services (Ncoyini et al., 2022). In addition, according to Thomas and Sanyaolu (2017), most smallholder farmers trust information from extension officers in rural areas; hence, they are seen as a dissemination tool for climatic information. Similarly, farm organizations are also treated as an information hub because being in a group gives farmers a chance to discuss how climate change affects agriculture and possible climate adaptation strategies (Tarhule and Lamb, 2003). However, in some parts of South Africa, extension agents are not very active and lack the ability or skills to help farmers understand climate information (Wilk et al., 2017).
Figure 2 presents descriptive results on farmers' food security statuses. The results showed that the majority of households (65%) had a high dietary diversity score, while 35% had a medium score, and no households fell into the low dietary diversity category. The findings indicate that farming households in the study area had more diverse diets. This might be because Elundini is a high-rainfall region, which allowed these households to grow vegetables and increase their farm income, thereby increasing their purchasing power for other food items they cannot grow. The findings from a study by Megbowon and Mushunje (2018) showed high dietary diversity among Eastern Cape farming households. Overall, the study findings indicate that smallholder crop farmers in Elundini municipality can be regarded to be food secure as shown in Figure 2. These findings align with other studies from different areas in Eastern Cape Province, South Africa (Ningi et al., 2021; Bese, 2020; Mayekiso, 2021), and are also contrary to another study from Nkonkobe renamed Raymond Mhlaba municipality of Eastern Cape province (Matebeni, 2008). The current results may be associated with smallholder crop farming households' ability to grow maize as their main crop, making them high consumers of cereals. Furthermore, according to reports from the Elundini Local Municipality (2020), maize is a critical crop contributing to smallholder farming households' food security in Elundini and across Eastern Cape province (Jezile et al., 2009). In addition, this might be associated with diversified livelihoods and income sources for food purchases among the interviewed sample. According to Adem et al. (2018), having diversified livelihood activities offers extra income, which would lessen the burden of household food expenses and encourage a wider variety of dietary options, thus better food security.
Figure 3 below presents the findings on farmers' access to specific climate services. The study's findings indicated that 69% of the surveyed farmers had access to weather forecasts, while only 24% had access to seasonal forecasts. The results imply that most of the smallholder crop farmers have access to the weather forecast, and very few have access to the seasonal forecast. This means that farmers in Elundini might be able to make short-term decisions based on the weather forecast. However, they are limited in making long-term seasonal cropping decisions due to the lack of information on what to expect in the next season. Therefore, it can be expected that smallholder crop farmers from the Elundini municipality might not be able to select the most appropriate crops to grow or change their farming methods properly, which can lead to lower yields and incomes. The findings align with evidence showing that in some African countries including Nigeria and South Africa, there is a gap in the accessibility of seasonal forecasts among smallholder farmers (Ofuoku and Obiazi, 2021; Ncoyini et al., 2022).
Table 2 presents farmers' cropping decisions influenced by access to climate services. Among the smallholder crop farmers who utilized these services, the majority (31%) made daily cropping decisions, including planning for irrigation, pest management, and weed removal. Some made informed decisions on what day and date to plant (18%), what crop to plant (11%), land preparations (11%), irrigation schedule (4%), fertilizer application (2%), and when to harvest (2%). The results imply that in the municipality, the fact that over a quarter of farmers who accessed climate services utilized them highlights the importance and usefulness of climate information in cropping decision-making, especially for immediate changes in farming choices. Therefore, there is a need to ensure that farmers in the study area are receiving climate information that is easy to integrate into their decision-making. The current study findings agree with the studies conducted in the KwaZulu-Natal province of South Africa, Nigeria, and Kenya, which showed that there are very few smallholder farmers making farming decisions based on climate services accessed in their study areas (Ncoyini et al., 2022; Ofuoku and Obiazi, 2021; Muema et al., 2018). Among the very few farmers who were able to make better farming choices informed using climate services, the findings indicated that in the study area, 31% of farmers used climate services for daily farming activities. The high proportion of farmers who used climate services for their daily farming activities in the current study can be associated with the high accessibility of daily forecasts. This confirms the findings by Antwi-Agyei et al. (2021) that to make farming choices, farmers depend on short-term weather forecasts such as daily and weekly forecasts. Furthermore, it was also discovered that smallholder crop farmers who accessed seasonal forecasts changed their planting dates. The results relate to Partey et al. (2020) who argue that in Ghana, most households that accessed seasonal climate services changed their planting dates based on the climate information.
While farmers made cropping decisions ranging from land preparation and harvest timing plans based on either weather or seasonal forecasts, only four common cropping decisions were analyzed in the study. This choice was made due to the poor variation in data for the other three cropping decisions (irrigation schedule, fertilizer application, and harvest timing), which could restrict inferential analysis. Table 3 presents the results from the propensity score matching on the impact of accessing weather and seasonal forecasts on farmers' cropping decisions. The NNM and KBM matching results indicate that the findings are statistically significant for several types of cropping decisions and are accurate as shown by a t-value above 2 and a low standard error. This means the relationship between access to weather or seasonal forecasts and cropping decisions is not driven by confounding effects and is robust to different conditions and expectations. The results from the NNM and KBM of the propensity score matching model show that access to short-term weather forecasts improves farmers' decision-making regarding daily farming activities by 18.8%−21.5%, improves farmers' decision-making on when to plant by 14.4%−16.1%, improves the decision on what type of crop to plant by 12.4%−19.2%, and when to prepare the land by 8%−8.7%. The results further show that access to seasonal forecasts improves farmers' decisions about their day-to-day farming activities by 25%−27% and about the suitable date to plant by 17.3%−20.2%, improves farmers' choices on what crop to plant by 19.2%−20.8%, and improves farmers decision on when to prepare the land for crop production by 15.3%−19.2% based on both algorithms. The results suggest that access to short-term weather and seasonal forecasts is essential for smallholder crop farmers in making decisions about daily farming activities, including the types of crops and crop varieties to plant, planting dates, and land preparation. This implies that smallholder farmers can also consider seasonal climate forecasts when carrying out their daily farming operations. In many cases, the decision to engage in daily farming activities is associated with access to short-term weather forecasts (FAO, 2019).
The current results relate to recent findings by Matere et al. (2023) who argue that constant dissemination of updated short-term weather information is essential for operational choices and risk mitigation throughout the planting season. Access to weather forecasts can have a crucial role in assisting farmers in deciding on the exact day to start sowing (Guido et al., 2020). Similarly, access to information that informs farmers about expected conditions for the next season, such as weather forecasts, is beneficial for determining the most suitable crops to plant (Tarhule and Lamb, 2003). A study by Chiputwa et al. (2020) reported similar results that the use of seasonal forecasts improved farmers' ability to choose the crop to sow by up to 13% in Senegal. Moreover, Deressa et al. (2009) found that in their study area, access to climate information improved the possibility of choosing crop varieties suited to dry conditions by 17.6%. Furthermore, the findings imply that when smallholder farmers have access to seasonal forecast information, they are better equipped to plan and decide on when to prepare the land. Similar results were discovered in Jamaica, where drought forecast information services had improved farmers' ability to decide on when to plant and what to plant among other farming decisions (Rahman et al., 2016). Furthermore, they relate to the findings from a qualitative study in Kenya, which associated better cropping decisions with accessing seasonal climate information among smallholder farmers (Rao et al., 2015). Furthermore, while decisions on what crop to plant and land preparations are typically informed by seasonal forecasts (FAO, 2019), the findings imply that weather forecasts also help farmers determine the type of crop to plant and when to prepare their land. It is evident that, compared to other cropping decisions influenced by both weather and seasonal forecasts, the chances of deciding when to prepare the land are lower based on the NNM and KBM results. Moreover, based on both the results of the impact of accessing weather and seasonal forecasts on cropping decisions, access to seasonal forecasts has a major impact on the different cropping decisions compared to access to weather forecasts. This implies that in the study area, seasonal climate forecasts can be considered the most important type of climate service that can assist farmers with their decision-making process. Therefore, for better cropping choices, smallholder farmers in the study area should integrate seasonal forecasts into their decision-making process. These findings confirm the argument that seasonal climate forecast is the most useful type of climate service (Vaughan et al., 2019).
Table 4 presents the results from the propensity score matching the impact of accessing weather and seasonal forecasts on household food security status. The NNM and KBM matching results indicate that the findings are statistically significant and are accurate as shown by a t-value above 2 and a low standard error. This implies that confounding factors do not cause the relationship between receiving weather or seasonal forecasts and household food security and are resistant to varying circumstances. The results from the NNM and KBM of the propensity score matching model indicate that having access to weather forecasts increases smallholder crop farmers' household dietary diversity scores by 0.559–0.903 units. Similarly, the improvements in the household dietary diversity scores of farmers who accessed seasonal climate information were even higher on both NNM and KBM by 0.701–0.971 units. The findings indicate that access to weather forecasts and seasonal climate information has positively impacted household dietary diversity scores. This means that smallholder farming households who are accessing both weather and seasonal climate forecasts consume more diverse and nutritious food than their counterparts who did not access them. Similar results were reported by Mesfin et al. (2022) that access to weather forecasts improved household food security of farming families. Furthermore, the findings relate to the observations made by Akwango et al. (2017) that farmers who used the information on early drought warning systems had household dietary diversity scores that were 36% more than those of farmers who did not use early drought warning systems. A recent study by Gitonga et al. (2020) also observed increased dietary diversity scores of up to 14% due to the climate information accessed by the Namibian farmers. The results further indicated that in both NNM and KBM, seasonal forecasts appear to be the most useful type in improving farmers' food security statuses too. This indicates that if smallholder crop farmers in Elundini can incorporate seasonal forecasts when making farming decisions, more food-secure households can be recorded. Therefore, stakeholders aiming to improve smallholder farming household food security should collaborate with forecast producers to ensure that they provide farmers with seasonal forecasts that are easy to access and incorporate into farmers' decision-making.
Table 4. Impact of accessing weather and seasonal forecasts on food security status (PSM estimates).
Figure 4 above shows the balance test results which indicate that before matching, there were differences in propensity scores between farmers who accessed and those who did not access weather forecasts (WFs) or seasonal forecasts (SFs). The figure further reveals that the matching process worked well, with propensity scores almost identical between farmers who accessed weather or seasonal forecasts (treated groups) and those who did not access them (control groups) after matching. This implies that based on propensity scores, smallholder crop farmers who were matched from control and treatment groups had similar characteristics. Furthermore, the variation in outcomes (cropping decisions and household dietary diversity scores) observed among the farmers with access to weather and seasonal forecasts and those with no access can therefore be attributed to accessing these two types of climate services.
Figure 4. Distribution of propensity scores before and after matching. (A) Distribution of propensity scores for weather forecasts (WFs). (B) Distribution of propensity scores for seasonal forecasts (SFs).
The study concluded that in the municipality of Elundini, a high proportion of smallholder crop farmers are accessing short-term weather forecasts with very few of them having access to seasonal forecasts. This led to smallholder crop farmers in the area generally making daily cropping decisions influenced by climate services. In addition, smallholder farmers in the study area were found to be food secure. Based on empirical results, the study concludes that access to both short-term weather and seasonal forecasts has a positive influence on different cropping decisions. This indicates that the cropping decisions (daily farming activities, planting day and date, what crop to plant, and land preparations) that smallholder farmers mentioned making based on either weather or seasonal forecasts are indeed attributed to their access to these two types of climate services. In addition, the study concludes that access to both short-term weather and seasonal forecasts had a positive impact on household dietary diversity scores. This indicates that access to climate services in Elundini municipality improves the food security status of smallholder farming households. Therefore, it is recommended that efforts to expand access to climate services among smallholder farmers in South Africa should be intensified. This can be achieved through the development and dissemination of more accessible and tailored climate information, including seasonal forecasts. In addition, climate services should be integrated into agricultural extension services and support systems to equip farmers with the knowledge and tools they need to make informed decisions. Such interventions have the potential to not only enhance farmers' resilience in the face of climate change but also contribute to greater food security and overall household welfare.
The raw data supporting the conclusions of this article will be made available by the authors, without undue reservation.
Y-IN: Formal analysis, Investigation, Methodology, Writing – original draft, Writing – review & editing. JM: Supervision, Writing – review & editing. LZ: Supervision, Writing – review & editing. SN: Supervision, Writing – review & editing.
The author(s) declare financial support was received for the research, authorship, and/or publication of this article. This study was conducted as part of a master's research project funded by an NRF grant titled: Global Change Social Sciences Research Programme, Project: Engaging South African farmers on climate variability and change through the use of climate services: a behavior change approach (2021–2023).
The authors would like to acknowledge farmers in the study area and enumerators who assisted with data collection.
The authors declare that the research was conducted in the absence of any commercial or financial relationships that could be construed as a potential conflict of interest.
The author(s) declared that they were an editorial board member of Frontiers, at the time of submission. This had no impact on the peer review process and the final decision.
All claims expressed in this article are solely those of the authors and do not necessarily represent those of their affiliated organizations, or those of the publisher, the editors and the reviewers. Any product that may be evaluated in this article, or claim that may be made by its manufacturer, is not guaranteed or endorsed by the publisher.
Abegunrin, O. O. (2021). Perceived benefits and use of weather forecast information among arable crop farmers in Ido, Oyo State Nigeria. Nigeria Agricultural J. 52, 83–88.
Adem, M., Tadele, E., Mossie, H., and Ayenalem, M. (2018). Income diversification and food security situation in Ethiopia: a review study. Cogent Food & Agriculture 4, 1513354. doi: 10.1080/23311932.2018.1513354
Adjin, K. C., Goundan, A., Henning, C. H., and Sarr, S. (2020). “Estimating the impact of agricultural cooperatives in Senegal: Propensity score matching and endogenous switching regression analysis,” in Working Papers of Agricultural Policy, No. WP2020-10 (Kiel: Kiel University, Department of Agricultural Economics, Chair of Agricultural Policy). Available at: https://nbn-resolving.de/urn:nbn:de:gbv:8:3-2021-00299-3
Akwango, D., Obaa, B. B., Turyahabwe, N., Baguma, Y., and Egeru, A. (2017). Effect of drought early warning system on household food security in Karamoja subregion, Uganda. Agric. Food Secur. 6:43. doi: 10.1186/s40066-017-0120-x
Amoah, L. N. A., and Simatele, M. D. (2021). Food security and coping strategies of rural household livelihoods to climate change in the Eastern Cape of South Africa. Front. Sustain. Food Syst. 5:692185. doi: 10.3389/fsufs.2021.692185
Antwi-Agyei, P., Dougill, A. J., and Abaidoo, R. C. (2021). Opportunities and barriers for using climate information for building resilient agricultural systems in Sudan savannah agro-ecological zone of north- eastern Ghana. Climate Serv. 22:100226. doi: 10.1016/j.cliser.2021.100226
Asayehegn Gebreeyesus, K., Temple, L., Sanchez, B., and Iglesias, A. (2017). Perception of climate change and farm level adaptation choices in central Kenya. Cahiers Agric. 26, 1–11. doi: 10.1051/cagri/2017007
Bedasa, Y., and Bedemo, A. (2023). The effect of climate change on food insecurity in the Horn of Africa. GeoJournal 88, 1829–1839. doi: 10.1007/s10708-022-10733-1
Bese, D. F. (2020). Sustainable Farmer Livelihoods and Enhancement of Food Security in Mbhashe Municipality, Eastern Cape Province, South Africa (Doctoral dissertation) University of the Free State, Bloemfontein, South Africa.
Bhaga, T. D., Dube, T., Shekede, M. D., and Shoko, C. (2020). Impacts of climate variability and drought on surface water resources in Sub-Saharan Africa using remote sensing: a review. Remote Sens. 12:4184. doi: 10.3390/rs12244184
Birachi, E., Hansen, J. W., Radeny, M., Mutua, M., Mbugua, M. W., Munyangeri, Y., et al. (2020). “Rwanda Climate Services for Agriculture: Evaluation of farmers' awareness, use and impacts,” in Working Paper. CGIAR Research Program on Climate Change, Agriculture and Food Security. Available at: https://cgspace.cgiar.org/handle/10568/108052 (accessed December 14, 2020).
Briggs, J. (2005). The use of indigenous knowledge in development: problems and challenges. Prog. Dev. Stud. 5, 99–114. doi: 10.1191/1464993405ps105oa
Buckland, S. F., and Campbell, D. (2021). An assessment of factors influencing awareness, access, and use of agro-climate services among farmers in Clarendon, Jamaica. Geoforum 126, 171–191. doi: 10.1016/j.geoforum.2021.07.032
Caliendo, M., and Kopeinig, S. (2008). Some practical guidance for the implementation of propensity score matching. J. Econ. Surv. 22, 31–72. doi: 10.1111/j.1467-6419.2007.00527.x
Calzadilla, A., Zhu, T., Rehdanz, K., Tol, R. S. J., and Ringler, C. (2014). Climate change and agriculture: Impacts and adaptation options in South Africa. Water Resour. Econ. 5, 24–48. doi: 10.1016/j.wre.2014.03.001
Chiputwa, B., Wainaina, Nakelse, T., Makui, Zougmoré, R. B., Ndiaye, O., and Minang, A. (2020). Transforming climate science into usable services: the effectiveness of co-production in promoting uptake of climate information by smallholder farmers in Senegal. Climate Serv. 20:100203. doi: 10.1016/j.cliser.2020.100203
Clarkson, G., Dorward, Osbahr, H., Torgbor, F., and Kankam-Boadu, I. (2019). An investigation of the effects of PICSA on smallholder farmers' decision-making and livelihoods when implemented at large scale–the case of northern Ghana. Clim. Serv. 14, 1–14. doi: 10.1016/j.cliser.2019.02.002
Cudjoe, G. P., Antwi-Agyei, B., and Gyampoh, B. A. (2021). The effect of climate variability on maize production in the ejura-sekyedumase municipality, Ghana. Climate 9:145. doi: 10.3390/cli9100145
Dehejia, R., and Wahba, S. (2002). Propensity score-matching methods for nonexperimental causal studies. Rev. Econ. Statist. 84, 151–161. doi: 10.1162/003465302317331982
Deressa, T. T., Hassan, R. M., Ringler, C., Alemu, T., and Yesuf, M. (2009). Determinants of farmers' choice of adaptation methods to climate change in the Nile Basin of Ethiopia. Global Environm. Change 19, 248–255. doi: 10.1016/j.gloenvcha.2009.01.002
Diouf, N. S., Ouedraogo, M., Ouedraogo, I., Ablouka, G., and Zougmor'e, R. (2020). Using seasonal forecast as an adaptation strategy: gender differential impact on yield and income in Senegal. Atmosphere 11:1127. doi: 10.3390/atmos11101127
Ebhuoma, E. E. (2022). Factors undermining the use of seasonal climate forecasts among farmers in South Africa and Zimbabwe: implications for the 1st and 2nd sustainable development goals. Front. Sustain. Food Syst. 6:761195. doi: 10.3389/fsufs.2022.761195
ECSECC (2017). Elundini Local Municipality, Socio-Economic Review, and Outlook. Available at: from https://www.ecsecc.org/documentrepository/informationcentre/elundini-local~municipality_74571.pdf (accessed April 17, 2017).
Elia, E. F., Mutula, S., and Stilwell, C. (2014). Indigenous knowledge use in seasonal weather forecasting in Tanzania: the case of semi-arid central Tanzania. South Afri. J. Librar. Inform. Sci. 80, 18–27. doi: 10.7553/80-1-1395
Elundini Local Municipality (2015). Final Integrated developmental plan, Maclear. Available at: http://www.elundini.org.za/images/IDP/IDP%202012_13.pdf (accessed May 16, 2016).
Elundini Local Municipality (2020). Tender: Identification of Interested Agro Processing Tenants for the Proposed Elundini Local Municipality Agro-Processing Hub. Maclear: Elundini Local Municipality. Available at: https://www.elundini.org.za/images/Tenders/2020-2021/agro-hub.pdf
FAO (2016). Save and Grow in Practice: Maize Rice Wheat. A Guide to Sustainable Cereal Production. Rome, Italy: Food and Agriculture Organization of the United Nations. Available at: http://www.fao.org/3/a-~i5318e.pdf
FAO (2019). Handbook On Climate Information for Farming Communities – What Farmers Need and What is Available. Rome: FAO.
FAO, IFAD, UNICEF, WFP, and WHO. (2021). “The State of food security and nutrition in the world 2021,” in Transforming Food Systems for Food Security, Improved Nutrition, and Affordable Healthy Diets for all (Rome, Italy: FAO).
Gbangou, T., Van Slobbe, E., Ludwig, F., Kranjac-Berisavljevic, G., and Paparrizos, S. (2021). Harnessing local forecasting knowledge on weather and climate in Ghana: documentation, skills, and integration with scientific forecasting knowledge. Weath. Clim. Soc. 13, 23–37. doi: 10.1175/WCAS-D-20-0012.1
Gitonga, Z. M., Visser, M., and Mulwa, C. (2020). Can climate information salvage livelihoods in arid and semiarid lands? An evaluation of access, use, and impact in Namibia. World Dev. Persp. 20:100239. doi: 10.1016/j.wdp.2020.100239
Guido, Z., Zimmer, A., Lopus, S., Hannah, C., Gower, D., Waldman, K., et al. (2020). Farmer forecasts: Impacts of seasonal rainfall expectations on agricultural decision-making in Sub-Saharan Africa. Clim. Risk Manage. 30:100247. doi: 10.1016/j.crm.2020.100247
Hansen, J. W., Furlow, J., Goddard, L., Nissan, H., Vaughan, C., Rose, A., et al. (2019). Scaling Climate Services to Enable Effective Adaptation Action (Report). Rotterdam; Washington, DC: Global Commission on Adaptation. Available at: https://hdl.handle.net/10568/105763
Hermans, K., and McLeman, R. (2021). Climate change, drought, land degradation and migration: exploring the linkages. Curr. Opin. Environm. Sustainab. 50, 236–244. doi: 10.1016/j.cosust.2021.04.013
Idoma, K., and Mamman, M. (2016). Access and utilization of climate change information and support services among vulnerable communities in Agatu LGA, Benue State, Nigeria. Int. J. Sci. Global Sustainab. 2, 18–18. Available at: https://fugus-ijsgs.com.ng/index.php/ijsgs/article/view/254 (accessed October 11, 2024).
Idrisa, Y. L., Ogunbameru, B. O., Ibrahim, A. A., and Bawa, D. B. (2012). Analysis of awareness and adaptation to climate change among farmers in the Sahel Savannah agro-ecological zone of Borno State, Nigeria. Int. J. Environ. Clim. Change 2, 216–226. doi: 10.9734/BJECC/2012/1475
Jezile, G. G., Westfall, D. G., Peterson, G., Child, D. R., Turner, D. P., and Averbeke, W. V. (2009). Effects of liming on microbial activity and N mineralization in broiler manure amended soils from Bizana, Eastern Cape, South Africa. South Afr. J. Plant Soil 26, 18–23. doi: 10.1080/02571862.2009.10639927
Joe Gqabi District Municipality (2016). Integrated Development Plan Review 2016/2021. Alwal North: JGDM.
Kadi, M., Njau, L. N., Mwikya, J., and Kamga, A. (2011). “The state of climate information services for agriculture and food security in East African Countries,” in CCAFS Working Paper No. 5 (Denmark: Copenhagen). Available at: www.ccafs.cgiar.orgb (accessed August 15, 2011).
Kennedy, G., Berardo, A., Papavero, C., Horjus, P., Ballard, T., Dop, M., et al. (2010). Proxy measures of household food consumption for food security assessment and surveillance: comparison of the household dietary diversity and food consumption scores. Public Health Nutr. 13:20102018. doi: 10.1017/S136898001000145X
Kinda, S. R., Badolo, F., and Tajani, F. (2019). Does rainfall variability matter for food security in developing countries? Cogent Econ. Finance 7:1640098. doi: 10.1080/23322039.2019.1640098
Knox, J., Hess, T., Daccache, A., and Wheeler, T. (2012). Climate change impacts on crop productivity in Africa and South Asia. Environm. Res. Lett. 7:034032 doi: 10.1088/1748-9326/7/3/034032
Kobusinge, R. (2018). Vulnerability to Climate Change for Food Security of Smallholder Farmers in Mahyoro Sub-County, Kamwenge District, Uganda (Doctoral dissertation) Makerere University, Kampala, Uganda, p. 46.
Koch, A., McBratney, A., Adams, M., Field, D., Hill, R., Crawford, J., et al. (2013). Soil security: solving the global soil crisis. Glob. Policy 4, 434–441. doi: 10.1111/1758-5899.12096
Kogo, B. K., Kumar, L., Koech, R., and Langat (2019). Modelling impacts of climate change on maize (Zea mays L.) growth and productivity: a Review of models, outputs, and limitations. J. Geosci. Environ. Prot. 7, 76–95. doi: 10.4236/gep.2019.78006
Lobut, C. (2017). Evaluation of the Impact of Computer-Aided Instruction on Student Performance. Sciences Po Department of Economics, Paris, France, p. 41.
Losloso, J. A. L., Predo, C. D., Sajise, A. J. U., Pulhin, J. M., and Espaldon, M. V. O. (2020). Determinants of the use of weather and seasonal climate information among smallholder maize farmers in Bulalacao, oriental Mindoro, Philippines. Philippine Agric. Sci. 103.
Macauley, H., and Ramadjita, T. (2015). Cereal Crops: Rice, Maize, Millet, Sorghum and Wheat, Cereal Crops. Available at: https://www.afdb.org/fileadmin/uploads/afdb/Documents/Events/DakAgri2015/Cerea~l_Crops-_Rice__Maize__Millet__Sorghum__Wheat.pdf (accessed October 21, 2015).
Mandleni, B., and Anim, F. D. K. (2011). Climate change awareness and decision on adaptation measures by livestock farmers. J. Agri. Sci. 3:3. doi: 10.5539/jas.v3n3p258
Mapanje, O. D., Siziba, S., Mtambanengwe, F., and Unganai, L. (2020). The impact of climate information services on smallholder farmers' livelihood outcomes. Afr. J. Rural Dev. 5, 29–47.
Maroyi, A. (2017). Assessment of useful plants in the catchment area of the proposed Ntabelanga dam in the Eastern Cape Province, South Africa. Sci. World J. 2017:3763607. doi: 10.1155/2017/3763607
Masesi, G. K. (2019). Access and utilization of seasonal climate forecast among smallholder farmers in Masinga Sub-County, Machakos County, Kenya (Doctoral dissertation). Chuka University.
Matebeni, F. (2008). Measuring rural household food security in the Nkonkobe Local Municipality, Eastern Cape Province of South Africa. (Master's Dissertation) Faculty of Agricultural Science, Stellenbosch University, Stellenbosch, South Africa.
Matere, S., Busienei, J. R., Irungu, P., Ernest Mbatia, O. L., Nandokha, T., and Kwena, K. (2023). Do farmers use climate information in adaptation decisions? case of smallholders in semi-arid Kenya. Inform. Dev. 2023:02666669231152568. doi: 10.1177/02666669231152568
Mayekiso, A. (2021). Economic Assessment of Indigenous Leafy Vegetables (ILVs) Production for Income Generation and Food Income Generation and Food Security in the Eastern Cape Province, South Africa (Doctoral dissertation) Faculty of Science and Agriculture, School of Agriculture, University of Limpopo, Polokwane, South Africa.
Mdluli, P., and Dunga, S. (2022). Determinants of poverty in South Africa using the 2018 general household survey data. J. Poverty 26, 197–213. doi: 10.1080/10875549.2021.1910100
Megbowon, E. T., and Mushunje, A. (2018). Assessment of food security among households in Eastern Cape province, South Africa: evidence from general household survey, 2014. Int. J. Soc. Econ. 45, 2–17. doi: 10.1108/IJSE-07-2016-0187
Mesfin, A. H., Gebremedhin, K. G., Birhan, Y. T., Yohannes, T., Weldeyesus, H., Tefera, T., et al. (2022). Weather information and shocks: policy implications to ensure food security in Tigray Region of Ethiopia. J. Arid Environ. 205:104769. doi: 10.1016/j.jaridenv.2022.104769
Morgan, R. P. C., and Nearing, M. A. (2010). Handbook of Erosion Modelling. Chichester: John Wiley & Sons, Ltd.
Muema, E., Mburu, J., Coulibaly, J., and Mutune, J. (2018). Determinants of access and utilization of seasonal climate information services among smallholder farmers in Makueni County, Kenya. Heliyon 4:e00889. doi: 10.1016/j.heliyon.2018.e00889
Murgor, D. K. (2015). Farmers Access to Climate and Weather Information and its Impact on Maize and Wheat Production in Uasin Gishu County, Kenya. (Doctoral dissertation) University of Eldoret, Eldoret, Kenya.
Muyiramye, D. (2020). “Agricultural vulnerability to changing rainfall patterns: Assessing the role of smallholder farmers' perceptions and access to weather forecast information in adaptation-decision making: A case study of the North-Western provinces, Rwanda,” in Second Cycle, A2E. Uppsala: SLU, Dept. of Urban and Rural Development. Available at: http://urn.kb.se/resolve?urn=urn:nbn:se:slu:epsilon-s-500079 (accessed June 30, 2020).
Myeni, L., and Moeletsi, M. E. (2020). Factors determining the adoption of strategies used by smallholder farmers to cope with climate variability in the Eastern Free State, South Africa. Agriculture 10:410. doi: 10.3390/agriculture10090410
Ncoyini, Z., Savage, M. J., and Strydom, S. (2022). Limited access and use of climate information by small-scale sugarcane farmers in South Africa: a case study. Clim. Serv. 26:100285. doi: 10.1016/j.cliser.2022.100285
Ndlovu, E., Prinsloo, B., and Le Roux, T. (2020). Impact of climate change and variability on traditional farming systems: Farmers' perceptions from south-west, semi-arid Zimbabwe. Jàmbá: J. Disast. Risk Stud. 12, 1–19. doi: 10.4102/jamba.v12i1.742
Nearing, M. A., Pruski, F. F., and O'Neal, M. R. (2004). Expected climate change impacts on soil erosion rates: a review. J. Soil Water Conserv. 59, 43–50.
Ngcaba, P., and Maroyi, A. (2021). Home gardens in the Eastern Cape Province, South Africa: a promising approach to enhance household food security and well-being. Biodiversitas J. Biol. Divers. 22:9. doi: 10.13057/biodiv/d220953
Ningi, T., Taruvinga, A., Zhou, L., and Ngarava, S. (2021). Determinants of water security for rural households: Empirical evidence from Melani and Hamburg communities, Eastern Cape, South Africa. Suid-Afrikaanse Tydskrif vir Natuurwetenskap en Tegnologie 40, 37–49. doi: 10.36303/SATNT.2021.40.1.802
Nkiaka, E., Taylor, A., Dougill, A. J., Antwi-Agyei, P., Fournier, N., Bosire, E. N., et al. (2019). Identifying user needs for weather and climate services to enhance 109 resilience to climate shocks in sub- Saharan Africa. Environ. Res. Lett. 14:123003. doi: 10.1088/1748-9326/ab4dfe
Nyamekye, A. B., Dewulf, A., Van Slobbe, E., and Termeer, K. (2019). Information systems and actionable knowledge creation in rice-farming systems in Northern Ghana. Afr.Geograph. Rev. 2019, 1–18 doi: 10.1080/19376812.2019.1659153
Ofuoku, A., and Obiazi, C. (2021). Constraints to access and utilization of meteorological services in Delta State, Nigeria. Yüzüncü Yil Üniversitesi Tarim Bilimleri Dergisi 31, 150–161. doi: 10.29133/yyutbd.738294
Owusu, K., Emmanuel, A. K., Musah-Surugu, I. J., and Yankson, P. W. K. (2019). The effects of 2015 El Nino on smallholder maize production in the transitional ecological zone of Ghana. Int. J. Clim. Change Strateg. Manage. 11, 609–621. doi: 10.1108/IJCCSM-02-2018-0014
Partey, S. T., Dakorah, A. D., Zougmore, R. B., Ouedraogo, M., Nyasimi, M., Nikoi, G. K., et al. (2020). Gender and climate risk management: evidence of climate information use in Ghana. Clim. Change 158, 61–75. doi: 10.1007/s10584-018-2239-6
Popoola, O. O., Yusuf, S. F. G., and Monde, N. (2020). Information sources and constraints to climate change adaptation amongst smallholder farmers in Amathole district municipality, Eastern Cape province, South Africa. Sustainability 12, 5846–5868. doi: 10.3390/su12145846
Rahman, T., Buizer, J., and Guido, Z. (2016). The Economic Impact of Seasonal Drought Forecast Information Service in Jamaica, 2014-15. Rep USAID Univ Ariz, Tucson, Arizona.
Rao, K. P. C., Hansen, J., Njiru, E., Githungo, W. N., and Oyoo, A. (2015). “Impacts of seasonal climate communication strategies on farm management and livelihoods in Wote, Kenya,” in CCAFS Working Paper, (No. 137) (Copenhagen: CGIAR Research Program on Climate Change, Agriculture and Food Security (CCAFS)), 42
Rubin, D. B. (2001). Using propensity scores to help design observational studies: Application to the tobacco litigation. Health Serv. Outcomes Res. Methodol. 2, 169–188. doi: 10.1023/A:1020363010465
Sirba, H. Y., and Chimdessa, T. B. (2021). Review of impact of climate change on food security in Africa. Int. J. Res. Innovat. Earth Sci. 8, 40–66.
Statistics South Africa (2016). “South African Community Survey 2016,” in Indicators Derived From the Full Population Community Survey. Available at: https://wazimap.co.za/profiles/municipality-EC141-elundini/ (accessed July 1, 2016).
Statistics South Africa (2019). Towards Measuring the Extent of Food Security in South Africa: An Examination of Hunger and FOOD ADEQUACY REPORT: 03-00-14. Available at: http://www.statssa.gov.za/publications/03-00-14/03-00-142017.pdf (accessed September 27, 2020).
Swindale, A., and Bilinsky, (2006). Household Dietary Diversity Score (HDDS) for Measurement of Household Food Access: Indicator Guide. Washington, DC: Food and Nutrition Technical Assistance Project, Academy for Educational Development.
Sylla, M. B., Pal, J. S., Faye, A., Dimobe, K., and Kunstmann, H. (2018). Climate change to severely impact West African Basin Scale Irrigation in 2?C and 1.5?C Global Warming Scenarios. Sci. Rep. 8:14395. doi: 10.1038/s41598-018-32736-0
Tall, A. (2013). “What do we mean by Climate Services?,” in Intergovernmental Board on Climate Services. Available at: https://library.wmo.int/opac/doc_num.php?explnum_id=3224 (accessed June 1, 2013).
Tarhule, A., and Lamb, J. (2003). Climate research and seasonal forecasting for West Africans: Perceptions, dissemination, and use? Perceptions, dissemination, and use? Bullet. Am. Meteorol. Soc. 84, 1741–1760. doi: 10.1175/BAMS-84-12-1741
Thomas, K. A., and Sanyaolu, A. S. (2017). Utilization of agro-meteorological Services among arable crop farmers in Oyo State, Nigeria. J. Agricult. Extens. 2,51–69. doi: 10.4314/jae.v21i1.5
Toreti, A., Deryng, D., Tubiello, F. N., Müller, C., Kimball, B. A., Moser, G., et al. (2020). Narrowing uncertainties in the effects of elevated CO2 on crops. Nature Food 1, 775–782. doi: 10.1038/s43016-020-00195-4
United Nations Development Program (2009). Handbook on Planning, Monitoring and Evaluating for Development Results. New York: UNDP.
Vaughan, C., Hansen, J., Roudier, P., Watkiss, P., and Carr, E. (2019). Evaluating agricultural weather and climate services in Africa: Evidence, methods, and a learning agenda. WIREs Clim Change. 10:e586. doi: 10.1002/wcc.586
Weisheimer, A., and Palmer, T. N. (2014). On the reliability of seasonal climate forecasts. J. R. Soc. Interface 11:20131162. doi: 10.1098/rsif.2013.1162
Wilk, J., Andersson, L., Graham, L. P., Wikner, J., Mokwatlo, S., and Petja, B. (2017). From forecasts to action - what is needed to make seasonal forecasts useful for South African smallholder farmers? Int. J. Disaster Risk Reduct. 25, 202–211. doi: 10.1016/j.ijdrr.2017.07.002
Zuma-Netshiukhwi, G. N. (2013). The Use of Operational Weather and Climate Information in Farmer Decision Making Exemplified for the South Western Free State, South Africa (Doctoral dissertation) University of the Free State, Bloemfontein, South Africa.
Keywords: access, climate services, impact, propensity score matching, smallholder farmers
Citation: Nocezo Y-I, Manyike JZ, Zhou L and Ngarava S (2024) The impact of access to climate services on smallholder farmers' cropping decisions and household food security in Elundini municipality, Eastern Cape province. Front. Clim. 6:1458999. doi: 10.3389/fclim.2024.1458999
Received: 03 July 2024; Accepted: 30 September 2024;
Published: 24 October 2024.
Edited by:
Cosmas Parwada, Midlands State University, ZimbabweReviewed by:
Friday Kubiku, Bindura University of Science Education, ZimbabweCopyright © 2024 Nocezo, Manyike, Zhou and Ngarava. This is an open-access article distributed under the terms of the Creative Commons Attribution License (CC BY). The use, distribution or reproduction in other forums is permitted, provided the original author(s) and the copyright owner(s) are credited and that the original publication in this journal is cited, in accordance with accepted academic practice. No use, distribution or reproduction is permitted which does not comply with these terms.
*Correspondence: Yanga-Inkosi Nocezo, yanganocezo12@gmail.com
Disclaimer: All claims expressed in this article are solely those of the authors and do not necessarily represent those of their affiliated organizations, or those of the publisher, the editors and the reviewers. Any product that may be evaluated in this article or claim that may be made by its manufacturer is not guaranteed or endorsed by the publisher.
Research integrity at Frontiers
Learn more about the work of our research integrity team to safeguard the quality of each article we publish.