- 1CICERO Center for International Climate Research, Oslo, Norway
- 2Met Office Hadley Centre, Exeter, United Kingdom
- 3Priestley Centre, University of Leeds, Leeds, United Kingdom
- 4Energy, Climate and Environment Program, International Institute for Applied Systems Analysis, Laxenburg, Austria
For effective radiative forcing (ERF) to be an ideal metric for comparing the strength of different climate drivers (such as CO2 and aerosols), the ratio of radiative forcing to global-mean temperature change must be the same for each driver. Typically, this ratio is divided by the same ratio for CO2 and termed efficacy. Previously it has been shown that efficacy is close to unity in abrupt perturbation experiments for a range of climate drivers, but efficacy with respect to CO2 has not been investigated in transient realistic simulations. Here, we analyse transient simulations from CMIP6 experiments and show comparable results between transient and abrupt perturbation experiments. We demonstrate that aerosol efficacy is not significantly different from unity, however inter-model differences in aerosol experiments are notably large.
1 Introduction
Effective radiative forcing (ERF) has become the preferred metric to quantify the top of atmosphere (TOA) perturbation to the Earth's energy budget by a given climate driver (Boucher et al., 2013; Myhre et al., 2013; Forster et al., 2021). This is because ERF, by definition, includes tropospheric rapid adjustments to the initial perturbation (Hansen et al., 2005; Sherwood et al., 2015) which leads to a more similar equilibrium temperature change for different climate drivers for a given forcing magnitude (Shine et al., 2003; Hansen et al., 2005; Richardson et al., 2019).
The utility of ERF as a metric to compare the relative strength of different climate drivers in inducing surface temperature change is also dependent on the behavior of the climate feedback parameter (α; W m.2 K−1), which represents the radiative response of the climate system to temperature change induced by a forcing (Forster et al., 2021). Namely, this dependency relies on whether α varies with forcing mechanism (Hansen et al., 2005) and time [e.g., Andrews et al. (2015), Rugenstein et al. (2016), Richardson et al. (2019)]. Quantifying the efficacy of different climate drivers (i.e., the global-mean surface temperature response per unit ERF relative to CO2) is therefore important for understanding the contribution of various climate drivers throughout the industrial era (Shindell, 2014; Richardson et al., 2019) and for the projection of future temperature trends and climate metrics (Myhre et al., 2013; Forster et al., 2021). The relationship between ERF and temperature change can be described as (Gregory and Andrews, 2016) in Equation (1):
where dT is the global-mean surface temperature change resulting from an ERF (dF) from a climate driver and dN is the TOA energy imbalance. Efficacy (Equation 2) is defined as Hansen et al. (2005) when the climate system is in equilibrium (dN = 0):
where dT is the global-mean surface temperature change resulting from an ERF (F) from climate driver i (such as non-CO2 greenhouse gases and aerosols) relative to that for CO2. An efficacy of one demonstrates that ERF is ideal for comparing surface temperature changes from different climate drivers, since the magnitude of warming in response to CO2 forcing is equal to that from i.
For a climate system not in equilibrium the efficacy becomes as in Equation (3):
Since the climate feedback parameter, , efficacy can also be described in terms of the radiative response induced by a forcing, whereby in Equation (4):
Here an efficacy close to unity illustrates that radiative feedback processes are similar for climate driver i relative to CO2.
Radiative feedbacks act to amplify or dampen climate sensitivity to a forcing and are demonstrated to play an important role in driving different temperature responses for different forcers (Shindell, 2014; Marvel et al., 2016; Smith and Forster, 2021; Salvi et al., 2022). Largely, a forcing occurring in regions with a weaker radiative response results in stronger warming per unit increase in ERF and an efficacy greater than unity. Zhou et al. (2023) calculate the efficacy of 10 different abrupt forcing agents to demonstrate its dependency on a combination of feedback state-dependence (e.g., Ceppi and Gregory (2019)) and “pattern-effect” (Haugstad et al., 2017), whereby different forcings initiate different radiative feedbacks via the pattern of change in sea-surface temperature (SST). They find enhanced warming over tropical regions leads to increased low clouds globally, a weaker lapse rate and more negative TOA radiative fluxes, resulting in a pattern-effect induced efficacy deviating from unity. Further, Zhou et al. (2023) find that a negative change in global surface temperature (e.g., from non-BC aerosol forcing) results in stronger radiative dampening and a reduced efficacy relative to abrupt 2xCO2 forcing. Comparing aerosols and greenhouse gases (GHGs), Salvi et al. (2022) also consider how historical forcings affect SST and stability and modulate radiative response, concluding that net radiative feedback is stronger for aerosols, consistent with their different latitudinal forcing distribution compared to GHGs; proposing that differences in α arise from different tropospheric stability responses and the subsequent impact on cloud and lapse-rate feedbacks. To further investigate how the spatial pattern of forcing affects climate feedback and sensitivity, Zhang et al. (2023) perform sensitivity simulations by applying solar forcing over the entire globe, Northern Hemisphere mid-latitudes, Southern Ocean, and tropics. They show that varied forcing patterns lead to large differences in feedback mechanisms. In particular, the lapse rate feedback and cloud feedback show large regional dependence which is mainly caused by differences in coupling between the surface and troposphere. Overall, their study found that the global mean surface temperature change is doubled when the forcing is imposed over the Southern Ocean compared to when the forcing is applied in the tropics.
Several studies report efficacies that deviate from unity (Hansen et al., 2005; Shindell, 2014; Shindell et al., 2015; Marvel et al., 2016; Modak et al., 2018), largely depending on the model and method used to derive forcing estimates. Richardson et al. (2019) conduct a multi-model analysis of 11 global climate models (GCMs) participating in the Precipitation Driver and Response Model Intercomparison Project [PDRMIP; Myhre et al. (2017)] to diagnose efficacy for abrupt forcing perturbations. With ERF calculated using fixed sea-surface temperatures and sea-ice (fsst) they report a solar forcing (2% increase in insolation) efficacy of less than one across all models (0.77-0-95) with multi-model mean efficacy of slightly less than one for 3x methane (CH4; 0.87), 5x sulfate (SO4; 0.94) and 10x black carbon (BC; 0.87), with large intermodel spread. They note that multi-model mean efficacy is marginally closer to unity when ERF is adjusted for land-surface temperature change and that efficacy varies substantially for SO4 and BC model ensembles in particular, causing uncertainty in whether it significantly deviates from unity.
Such abrupt forcing experiments more inherently embody the equilibrium temperature response however, and do not characterize transient changes in ERF, α and dT. The novelty in this study is that we analyse CMIP6 transient fully-coupled GCM simulations with ERF diagnosed from corresponding fsst simulations to compare efficacy calculated across the historical period against that diagnosed from abrupt 4xCO2.
2 Materials and methods
We use results from the CMIP6 DECK simulations (Eyring et al., 2016) and the two CMIP6 MIPs: DAMIP (Gillett et al., 2016) and RFMIP (Pincus et al., 2016). Specifically, we use the DECK fully coupled transient simulations forced by a 1% increase in CO2 until quadrupling (1pctCO2) and by all climate drivers over the industrial era from 1850 to 2014 (historical) along with the DAMIP fully coupled transient simulation of historical change in CO2 (hist-CO2), historical change in all well-mixed greenhouse gases (hist-GHG) and historical change in all aerosols (hist-aer). In addition, we include the fully coupled DECK simulation of abrupt quadrupling of CO2 (abrupt-4xCO2) which is distinct from the aforementioned transient simulations under investigation in this study. This abrupt-4xCO2 is similar to the setup of all experiments in the PDRMIP study (Richardson et al., 2019).
ERFs are derived from the RFMIP fsst simulations as detailed in Smith et al. (2020) and are calculated relative to the piClim-control reference case following the method denoted as ERF_trop in Smith et al. (2020), which corrects for land surface change in fsst experiments and the portion of tropospheric temperature and water vapor changes associated with it [also see further discussions in Andrews et al. (2021)]. Tables 1, 2 summarize the models and CMIP6 experiments included in this study, respectively. All CMIP6 data used are monthly means.
To calculate efficacy following Equation 3 we use the CMIP6 1pctCO2 experiment until doubling of CO2 (which occurs at year 70) as the reference CO2 ERF and surface temperature response for the six other experiments (Table 2), whereby the surface temperature response is calculated as the difference between last 5 years of each fully-coupled experiment and the pre-industrial simulation (piControl). Note, that the 5 years are centered around year 70 in the reference 1pctCO2. To derive an estimate of internal variability, the last 5 years of each experiment are also differenced from 10 different piControl 5-year periods taken from across the simulation length. As given in Equation 3, the TOA imbalance is subtracted from the ERF in each experiment to calculate efficacy (Hansen et al., 2005), including the reference 1ptCO2 experiment. Here, the TOA imbalance is calculated as the difference between the given transient CMIP6 experiment listed in Table 2 and the CMIP6 piControl experiment. Note that a line-by-line model (Myhre et al., 2006; Etminan et al., 2016) has been used to establish the relation of doubling of CO2 to quadrupling of CO2 since only quadrupling is available from the RFMIP simulations (Smith et al., 2020).
3 Results
Figure 1 shows efficacy values for the six CMIP6 experiments, using 1ptCO2 as the reference case. The uncertainties bars represent the outcomes relative to the ten 5-year periods in the piControl simulations. In most models, the hist-GHG experiment has efficacies closest to unity. The hist-GHG experiment includes all major GHGs over the industrial era, with CO2 being the dominant (Forster et al., 2021). The largest model range in efficacy is in the hist-aer experiment. However, the hist-aer experiment has the weakest ERF and thus the internal variability for the surface temperature response may cause part of the large range in efficacy and large uncertainty range for some of the models. The reasons for the large range in the efficacy in the hist-aer experiment could be manyfold, including that models have very different spatial distributions of aerosol, the type of aerosol i.e., absorbing vs. scattering, and their balance between aerosol-radiation interaction and aerosol-cloud interaction (Smith et al., 2020). On top of this the efficacy is dependent on the relation between Northern hemisphere vs. Southern Hemisphere forcing pattern (Zhang et al., 2023), possibly the land vs. ocean forcing (Shindell, 2014) and how the forcing pattern initiates the feedbacks (Haugstad et al., 2017). There is not a clear relation between the results for the historical and hist-aer experiments. All the models have an efficacy above one for the abrupt-4xCO2 experiment, except UKESM1. The efficacy for this experiment is weakly correlated with the effective climate sensitivity (EffCS; 0.35) with EffCS calculated in Zelinka et al. (2020).
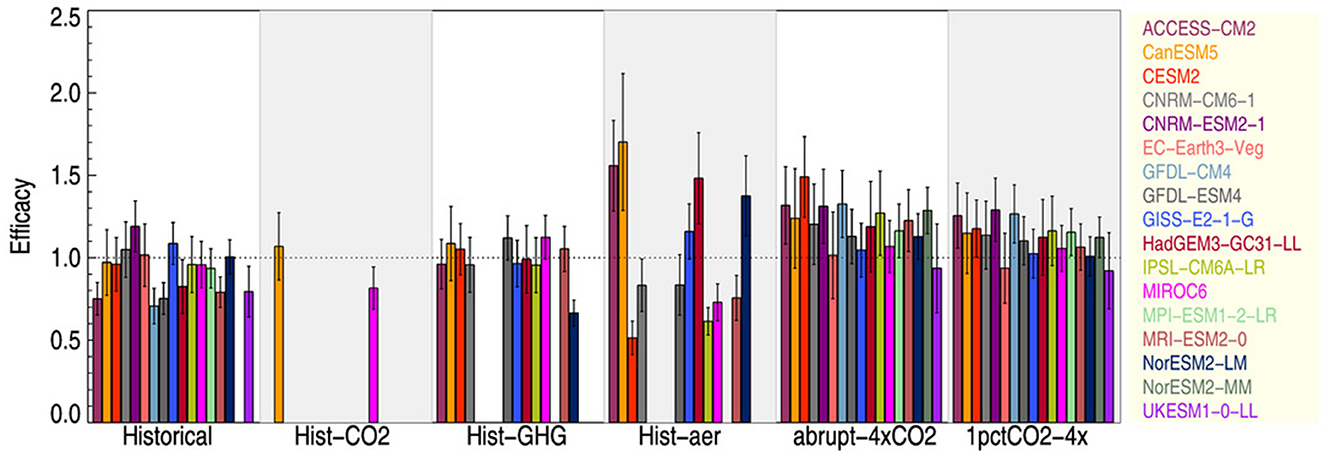
Figure 1. Efficacy for six CMIP6 experiments and seventeen CMIP6 models. The reference is the CMIP6 experiment 1ptCO2 at 2xCO2. Uncertainty bars shown as reference to ten PiControl time intervals.
Supplementary Figure S1 illustrates the same as Figure 1, except that the TOA imbalance has not been excluded in the calculations of efficacies. In Supplementary Figure S1 unlike in Figure 1, the efficacy is beyond unity for all models in the abrupt-4xCO2 experiment. Of the seven CMIP6 experiments analyzed in this study, the abrupt-4xCO2 and 1pctCO2-4x experiments have the largest TOA imbalance (Figure 2A). However, these experiments have at least twice as strong an ERF than in the other experiments. Figure 2B shows that the models have responded to about 80% of the initial ERF and thus 20% is remaining to full equilibrium (in abrupt-4xCO2). In millennial-length simulations of CO2 perturbation, the models are close to equilibrating and EffCS is 17% higher than the analysis of the 150 years simulations (Rugenstein et al., 2020). Figures 2B, C shows that the abrupt-4xCO2 experiment is closer to equilibrium than the other CMIP6 experiments. Among the six other CMIP6 experiments, which are transient simulations, there is a marked similarity in the remaining fraction of imbalance. However, it is apparent that the 1ptCO2 experiment at 4xCO2 (year 140) exhibits a relatively smaller imbalance compared to 1ptCO2 at 2xCO2, and likewise with hist-aer contrasted against the historical experiment. The latter effect may be because the rate of increase in negative aerosol forcing has been weaker or has even exhibited a reversal of sign over the last one to two decades of the CMIP6 historical experiments (Smith et al., 2021; Quaas et al., 2022) whilst there has been a continued increase in the GHG forcing (Forster et al., 2021).
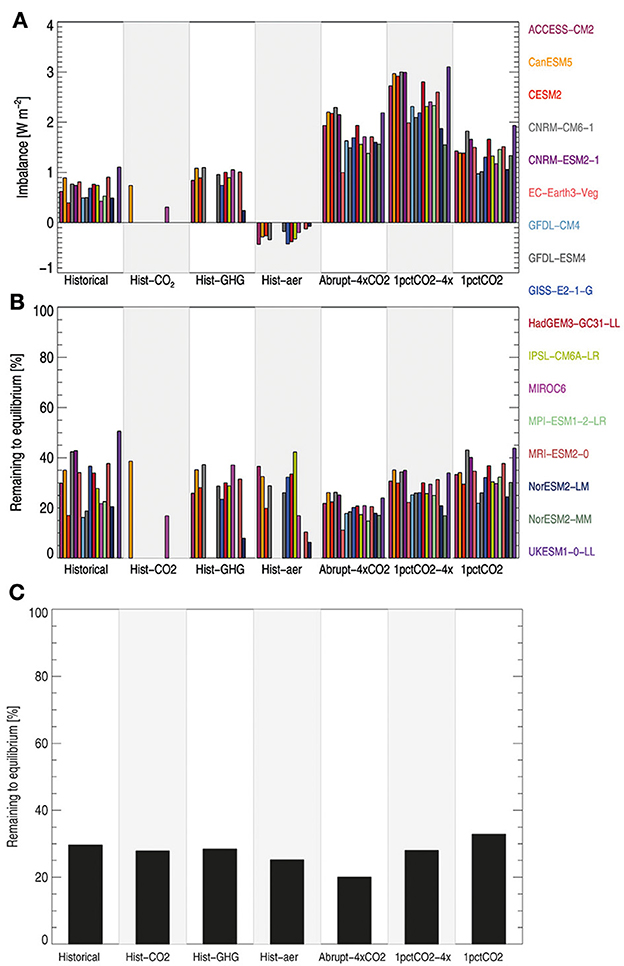
Figure 2. (A) TOA imbalance in seven CMIP6 experiments, (B) the remaining imbalance given in percent for individual CMIP6 models, (C) the remaining imbalance given in percent for CMIP6 multi-model mean.
Figure 3 shows the geographical distribution of efficacy calculated as the geographical distribution of dTi/dFi divided by the global mean αCO2. Note that the imbalance is not taken into account in the figure. Across all experiments, a clear Arctic amplification is evident (Previdi et al., 2021; Rantanen et al., 2022) and efficacies below 1 are common over ocean. The abrupt-4xCO2 has the highest efficacies (as shown in Figure 1, Supplementary Figure S1) with values around unity over ocean with much higher values over Antarctica than in the other CMIP6 experiments shown here. An interesting feature of the hist-aer experiment is the notably higher efficacies over land in the Northern hemisphere. Moreover, distinct strongly negative local efficacies over the southern hemisphere ocean are evident in the hist-aer experiment relative to the other experiments. The historical and hist-GHG experiments exhibit substantial similarity in most regions. However, deviations arise in areas with elevated anthropogenic aerosol abundance, such as China, India and eastern part of US, where the Historical experiment has efficacies below unity.
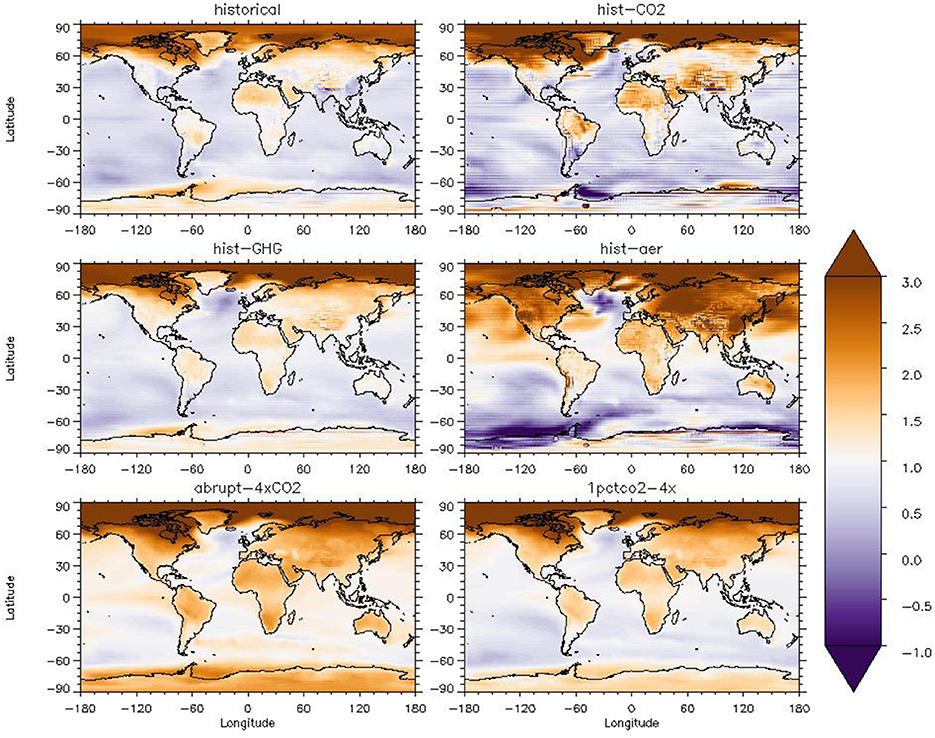
Figure 3. Geographical distribution of efficacy for six CMIP6 experiments. Multi-model mean results are shown.
Figure 4 shows the geometric mean efficacies from the CMIP6 experiments and compares them to the idealized experiments within PDRMIP. The PDRMIP results are same as in Richardson et al. (2019), but with the land surface temperature correction incorporated as in Andrews et al. (2021). Overall, the mean efficacies are close to unity, including for the experiments involving aerosols. The finding here is that efficacies in transient experiments are similar to abrupt experiments. Efficacies above unity occur in experiments with large forcings such as in the quadrupling of CO2 (transient and abrupt). The largest exception for the unity of efficacy is found for experiments with few models involved such as land use change (land use) and BC specified at lower altitudes in the atmosphere (BCSLT). In both of these experiments the surface temperature response is weak causing the internal variability to play a larger role than in many of the other experiments. In the land use experiment the direct change in the surface fluxes of sensible and latent heat can further cause difference in surface temperature response for a given ERF compared to the other experiments in this study.
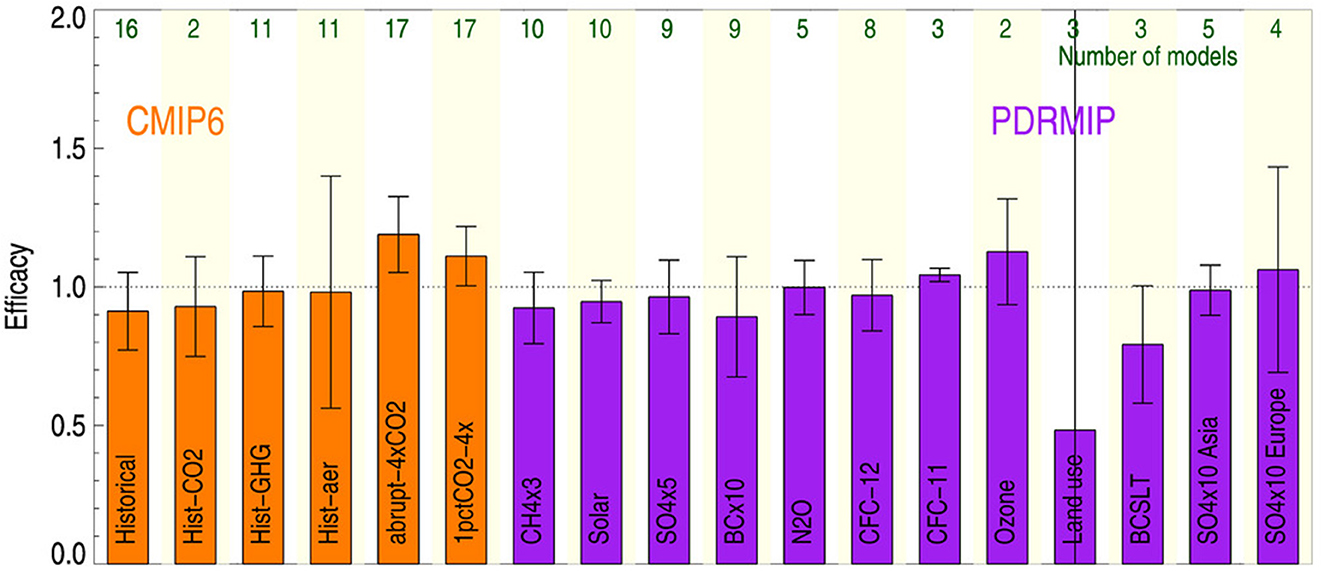
Figure 4. Efficacies in CMIP6 and PDRMIP experiments. The bars show the geometric means and uncertainties shown as one standard deviation. The number of model simulations available for each experiment is shown in green.
4 Discussions and conclusions
A main finding from this study is that there is no indication that efficacies for aerosols are very different from unity, as shown in both in the CMIP6 and PDRMIP simulations. However, model differences in the aerosol experiments are much larger than for the other experiments. The advantage of the PDRMIP SO4x5 experiment is that a large perturbation is performed, resulting in a robust surface temperature response and thus signal to noise is large. In the other aerosol experiments a low surface temperature response may have contributed to large model deviations in the efficacy.
The spatial pattern of the ERF of anthropogenic aerosol changes for 11 models are shown in Supplementary Figure S2 to investigate further the large range in the efficacy of hist-aer. The magnitude and spatial pattern vary between the models with all models having the strongest negative forcing over South-East Asia. The higher efficacy over land in the Northern hemisphere shown in Figure 3 is not a feature that is causing the hist-aer efficacy to have a large model diversity as shown in Figure 1. The land fraction aerosol ERF is actually slightly anticorrelated (-0.25 Pearson correlation coefficient) to the efficacy for the models in the hist-aer experiment. All models have a stronger aerosol ERF in the tropics relative to the global mean (ratio range from 1.07 to 1.30) and this ratio is moderately anticorrelated (−0.58) to the model range in hist-aer efficacy, meaning models with high tropical aerosol ERF generally have low efficacy. The correlation of the efficacy to the fraction of polar aerosol ERF to global mean and fraction of Northern hemisphere to global mean are both low (−0.30 and 0.24, respectively). To draw firm conclusions of temperature response from aerosol perturbations we show that a large model ensemble is required since the model variations are large. We find no systematic cause of this large model diversity in efficacy for aerosol perturbation in terms of aerosol type (sulfate or BC), transient or abrupt or regional aerosol forcing.
Our surface temperature responses have been taken for the 5 last years of transient simulations. Expanding this to 10 years generally changes the efficacy modestly, but somewhat more for the hist-aer experiment (from 0.98 to 1.09 for the geometric mean) than the other experiments. The standard deviation among the models for the hist-aer experiment changes slightly from 0.42 to 0.46 using 10 years instead of 5 years for the surface temperature response. Overall, the selection of number of years at the end of the simulations seem to weakly influence the efficacy results.
ERF was introduced to make it a good indicator of surface temperature change from various climate drivers (Boucher et al., 2013; Myhre et al., 2013; Sherwood et al., 2015; Forster et al., 2021), in practice that is to have the efficacy of unity for all climate drivers. In the past several studies have shown that efficacy is unity in abrupt multi-model studies (Richardson et al., 2019) and in a comprehensive single-model study (Hansen et al., 2005). Here, we demonstrate that ERF is a good climate metric as multi-model simulations have efficacy close to unity in several realistic transient simulations. Our results show large model spread for aerosols, but unlike other studies no systematic differences from GHG experiments (Shindell, 2014; Salvi et al., 2022).
Data availability statement
CMIP6 model data used in this study are freely available from the CMIP6 repository on the Earth System Grid Federation nodes (https://esgf-node.llnl.gov/search/cmip6/, World Climate Research Programme, 2020). The PDRMIP data are available through the World Data Center for Climate (WDCC) https://www.dkrz.de/up/systems/wdcc with https://doi.org/10.26050/WDCC/PDRMIP_2012-2021.
Author contributions
GM: Conceptualization, Formal analysis, Investigation, Methodology, Visualization, Writing—original draft, Writing—review & editing. RB: Methodology, Writing—original draft, Writing—review & editing. TA: Methodology, Validation, Writing—original draft, Writing—review & editing. PF: Methodology, Validation, Writing—original draft, Writing—review & editing. CS: Methodology, Validation, Writing—original draft, Writing—review & editing.
Funding
The author(s) declare that financial support was received for the research, authorship, and/or publication of this article. This study was supported by the European Union's Horizon 2020 research and innovation program under grant agreement no 820829 (CONSTRAIN project) and supported by the KeyCLIM project grant no. 295046 funded by the Norwegian Research Council. For PDRMIP parts of the computations were performed on and data has been interchanged shared and stored on resources provided by Sigma2 -the National Infrastructure for High-Performance Computing and Data Storage in Norway (project accounts NN9188K and NS9042K).
Conflict of interest
The authors declare that the research was conducted in the absence of any commercial or financial relationships that could be construed as a potential conflict of interest.
The author(s) declared that they were an editorial board member of Frontiers, at the time of submission. This had no impact on the peer review process and the final decision.
Publisher's note
All claims expressed in this article are solely those of the authors and do not necessarily represent those of their affiliated organizations, or those of the publisher, the editors and the reviewers. Any product that may be evaluated in this article, or claim that may be made by its manufacturer, is not guaranteed or endorsed by the publisher.
Supplementary material
The Supplementary Material for this article can be found online at: https://www.frontiersin.org/articles/10.3389/fclim.2024.1397358/full#supplementary-material
References
Andrews, T., Gregory, J. M., and Webb, M. J. (2015). The dependence of radiative forcing and feedback on evolving patterns of surface temperature change in climate models. J. Clim. 28, 1630–1648. doi: 10.1175/JCLI-D-14-00545.1
Andrews, T., Smith, C. J., Myhre, G., Forster, P. M., Chadwick, R., and Ackerley, D. (2021). Effective radiative forcing in a GCM with fixed surface temperatures. J. Geophys. Res.: Atmosph. 126:e2020JD033880. doi: 10.1029/2020JD033880
Boucher, O., Randall, D., Artaxo, P., Bretherton, C., Feingold, G., Forster, P., et al. (2013). “Clouds and Aerosols,” in Climate Change 2013: The Physical Science Basis. Contribution of Working Group I to the Fifth Assessment Report of the Intergovernmental Panel on Climate Change, eds. T. F. Stocker, D. Qin, G.-K. Plattner, M. Tignor, S. K. Allen, J. Boschung, et al. (Cambridge, United Kingdom and New York, NY: Cambridge University Press), 571–657. doi: 10.1017/CBO9781107415324.016
Ceppi, P., and Gregory, J. M. (2019). A refined model for the Earth's global energy balance. Climate Dynam. 53, 4781–4797. doi: 10.1007/s00382-019-04825-x
Etminan, M., Myhre, G., Highwood, E. J., and Shine, K. P. (2016). Radiative forcing of carbon dioxide, methane, and nitrous oxide: a significant revision of the methane radiative forcing. Geophys. Res. Lett. 43, 12614–12623. doi: 10.1002/2016GL071930
Eyring, V., Bony, S., Meehl, G. A., Senior, C. A., Stevens, B., Stouffer, R. J., et al. (2016). Overview of the Coupled Model Intercomparison Project Phase 6 (CMIP6) experimental design and organization. Geosci. Model Dev. 9, 1937–1958. doi: 10.5194/gmd-9-1937-2016
Forster, P., Storelvmo, T., Armour, K., Collins, W., Dufresne, J.-L., Frame, D., et al. (2021). “The earth's energy budget, climate feedbacks, and climate sensitivity,” in Climate Change 2021: The Physical Science Basis. Contribution of Working Group I to the Sixth Assessment Report of the Intergovernmental Panel on Climate Change, eds. V. Masson-Delmotte, P. Zhai, A. Pirani, S. L. Connors, C. Péan, S. Berger, et al. (Cambridge: Cambridge University Press).
Gillett, N. P., Shiogama, H., Funke, B., Hegerl, G., Knutti, R., Matthes, K., et al. (2016). The Detection and Attribution Model Intercomparison Project (DAMIP v1.0) contribution to CMIP6. Geosci. Model Dev. 9, 3685–3697. doi: 10.5194/gmd-9-3685-2016
Gregory, J. M., and Andrews, T. (2016). Variation in climate sensitivity and feedback parameters during the historical period. Geophys. Res. Lett. 43, 3911–3920. doi: 10.1002/2016GL068406
Hansen, J., Sato, M., Ruedy, R., Nazarenko, L., Lacis, A., Schmidt, G. A., et al. (2005). Efficacy of climate forcings. J. Geophys. Res.-Atmosph. 110:D18104. doi: 10.1029/2005JD005776
Haugstad, A. D., Armour, K. C., Battisti, D. S., and Rose, B. E. J. (2017). Relative roles of surface temperature and climate forcing patterns in the inconstancy of radiative feedbacks. Geophys. Res. Lett. 44, 7455–7463. doi: 10.1002/2017GL074372
Marvel, K., Schmidt, G. A., Miller, R. L., and Nazarenko, L. S. (2016). Implications for climate sensitivity from the response to individual forcings. Nat. Clim. Chang. 6, 386–389. doi: 10.1038/nclimate2888
Modak, A., Bala, G., Caldeira, K., and Cao, L. (2018). Does shortwave absorption by methane influence its effectiveness? Climate Dynam. 51, 3653–3672. doi: 10.1007/s00382-018-4102-x
Myhre, G., Forster, P. M., Samset, B. H., Hodnebrog, Ø., Sillmann, J., Aalbergsj,ø, S. G., et al. (2017). PDRMIP: a precipitation driver and response model intercomparison project—protocol and preliminary results. Bull. Am. Meteorol. Soc. 98, 1185–1198. doi: 10.1175/BAMS-D-16-0019.1
Myhre, G., Shindell, D., Bréon, F.-M., Collins, W., Fuglestvedt, J., Huang, J., et al. (2013). “Anthropogenic and natural radiative forcing,” in Climate Change 2013: The Physical Science Basis. Contribution of Working Group I to the Fifth Assessment Report of the Intergovernmental Panel on Climate Change, eds. T. F. Stocker, D. Qin, G.-K. Plattner, M. Tignor, S.K. Allen, J. Boschung, et al. (Cambridge, United Kingdom and New York, NY: Cambridge University Press), 659–740. doi: 10.1017/CBO9781107415324.018
Myhre, G., Stordal, F., Gausemel, I., Nielsen, C. J., and Mahieu, E. (2006). Line-by-line calculations of thermal infrared radiation representative for global condition: CFC-12 as an example. J. Quant. Spectrosc. Radiat. Transf. 97, 317–331. doi: 10.1016/j.jqsrt.2005.04.015
Pincus, R., Forster, P. M., and Stevens, B. (2016). The Radiative Forcing Model Intercomparison Project (RFMIP): experimental protocol for CMIP6. Geosci. Model Dev. 9, 3447–3460. doi: 10.5194/gmd-9-3447-2016
Previdi, M., Smith, K. L., and Polvani, L. M. (2021). Arctic amplification of climate change: a review of underlying mechanisms. Environm. Res. Lett. 16:093003. doi: 10.1088/1748-9326/ac1c29
Quaas, J., Jia, H., Smith, C., Albright, A. L., Aas, W., Bellouin, N., et al. (2022). Robust evidence for reversal of the trend in aerosol effective climate forcing. Atmos. Chem. Phys. 22, 12221–12239. doi: 10.5194/acp-22-12221-2022
Rantanen, M., Karpechko, A. Y., Lipponen, A., Nordling, K., Hyvärinen, O., Ruosteenoja, K., et al. (2022). The Arctic has warmed nearly four times faster than the globe since 1979. Commun. Earth Environm. 3:168. doi: 10.1038/s43247-022-00498-3
Richardson, T. B., Forster, P. M., Smith, C. J., Maycock, A. C., Wood, T., Andrews, T., et al. (2019). Efficacy of climate forcings in PDRMIP models. J. Geophys. Res.: Atmosph. 124, 12824–12844. doi: 10.1029/2019JD030581
Rugenstein, M., Bloch-Johnson, J., Gregory, J., Andrews, T., Mauritsen, T., Li, C., et al. (2020). Equilibrium climate sensitivity estimated by equilibrating climate models. Geophys. Res. Lett. 47:e2019GL083898. doi: 10.1029/2019GL083898
Rugenstein, M. A., Caldeira, K., and Knutti, R. (2016). Dependence of global radiative feedbacks on evolving patterns of surface heat fluxes. Geophys. Res. Lett. 43, 9877–9885. doi: 10.1002/2016GL070907
Salvi, P., Ceppi, P., and Gregory, J. M. (2022). Interpreting differences in radiative feedbacks from aerosols versus greenhouse gases. Geophys. Res. Lett. 49:e2022GL097766. doi: 10.1029/2022GL097766
Sherwood, S. C., Bony, S., Boucher, O., Bretherton, C., Forster, P. M., Gregory, J. M., et al. (2015). Adjustments in the forcing-feedback framework for understanding climate change. Bull. Am. Meteorol. Soc. 96, 217–228. doi: 10.1175/BAMS-D-13-00167.1
Shindell, D. T. (2014). Inhomogeneous forcing and transient climate sensitivity. Nat. Clim. Chang. 4, 274–277. doi: 10.1038/nclimate2136
Shindell, D. T., Faluvegi, G., Rotstayn, L., and Milly, G. (2015). Spatial patterns of radiative forcing and surface temperature response. J. Geophys. Res.-Atmosph. 120, 5385–5403. doi: 10.1002/2014JD022752
Shine, K. P., Cook, J., Highwood, E. J., and Joshi, M. M. (2003). An alternative to radiative forcing for estimating the relative importance of climate change mechanisms. Geophys. Res. Lett. 30, 2047. doi: 10.1029/2003GL018141
Smith, C. J., and Forster, P. M. (2021). Suppressed late-20th century warming in CMIP6 models explained by forcing and feedbacks. Geophys. Res. Lett. 48:e2021GL094948. doi: 10.1029/2021GL094948
Smith, C. J., Harris, G. R., Palmer, M. D., Bellouin, N., Collins, W., Myhre, G., et al. (2021). Energy budget constraints on the time history of aerosol forcing and climate sensitivity. J. Geophys. Res.: Atmosph. 126:e2020JD033622. doi: 10.1029/2020JD033622
Smith, C. J., Kramer, R. J., Myhre, G., Alterskjær, K., Collins, W., Sima, A., et al. (2020). Effective radiative forcing and adjustments in CMIP6 models. Atmos. Chem. Phys. 20, 9591–9618. doi: 10.5194/acp-20-9591-2020
Zelinka, M. D., Myers, T. A., Mccoy, D. T., Po-Chedley, S., Caldwell, P. M., Ceppi, P., et al. (2020). Causes of higher climate sensitivity in CMIP6 models. Geophys. Res. Lett. 47:e2019GL085782. doi: 10.1029/2019GL085782
Zhang, B., Zhao, M., He, H., Soden, B. J., Tan, Z., Xiang, B., et al. (2023). The dependence of climate sensitivity on the meridional distribution of radiative forcing. Geophys. Res. Lett. 50:e2023GL105492. doi: 10.1029/2023GL105492
Keywords: effective radiative forcing, imbalance, climate response, climate models, CMIP6
Citation: Myhre G, Byrom RE, Andrews T, Forster PM and Smith CJ (2024) Efficacy of climate forcings in transient CMIP6 simulations. Front. Clim. 6:1397358. doi: 10.3389/fclim.2024.1397358
Received: 07 March 2024; Accepted: 21 May 2024;
Published: 12 June 2024.
Edited by:
Yang Yang, Nanjing University of Information Science and Technology, ChinaReviewed by:
Lili Ren, Jiangsu Open University, ChinaJiyuan Gao, Nanjing University of Information Science and Technology, China
Copyright © 2024 Myhre, Byrom, Andrews, Forster and Smith. This is an open-access article distributed under the terms of the Creative Commons Attribution License (CC BY). The use, distribution or reproduction in other forums is permitted, provided the original author(s) and the copyright owner(s) are credited and that the original publication in this journal is cited, in accordance with accepted academic practice. No use, distribution or reproduction is permitted which does not comply with these terms.
*Correspondence: Gunnar Myhre, Z3VubmFyLm15aHJlQGNpY2Vyby5vc2xvLm5v