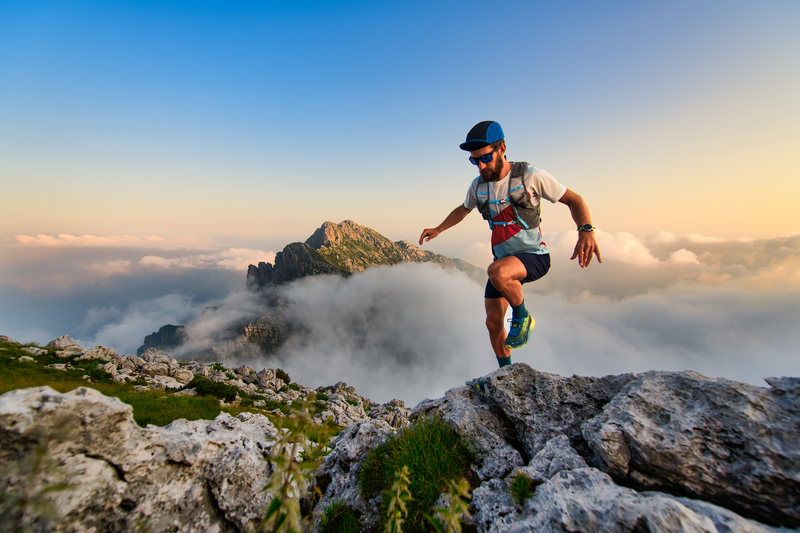
95% of researchers rate our articles as excellent or good
Learn more about the work of our research integrity team to safeguard the quality of each article we publish.
Find out more
ORIGINAL RESEARCH article
Front. Clim. , 05 April 2024
Sec. Climate Risk Management
Volume 6 - 2024 | https://doi.org/10.3389/fclim.2024.1330025
This article is part of the Research Topic Impacts of Climate change and Drylands: Challenges, Opportunities, and Innovations to Transform Global Drylands View all 4 articles
The resilience capacity of smallholder households is one of the main drivers of their ability to continue to farm and make investments in the fragile dryland regions. This paper aims to assess the resilience profile of smallholder farmers in the face of climate change and the factors influencing it in three dryland sub-regions of Senegal, namely, Louga, Kaffrine, and Thies. We developed a composite index of climate resilience (CICR) using data on farmers' perceptions of climate variability and their perceived ability to withstand, adapt, and bounce back in the event of climatic shocks. Drought, strong winds, and soil fertility decline because of climate change emerged as the main climate hazards impacting smallholder farming systems. The CICR value ranged from −2 for the most vulnerable households to +2 for the most resilient households. On average, all the households were found to be vulnerable, with an average CICR value of −0.2. The LOUGA region was the most vulnerable, with an average CICR value of −0.36, followed by THIES (-0.2). The KAFFRINE region was relatively less vulnerable, with a CICR value of −0.1. Ordered logit model estimates show that the chances of improving CICR decrease with the increase of the household head's age until 59 years. Access to training on climate-smart agricultural (CSA) practices and climate information appeared to have the potential to increase by 171% the chance of the household improving its resilience status. Analysis also shows that one more woman working off-farm or in-home gardening has the potential to multiply by four times the chances of households being more resilient. This highlights the importance of empowering women to enhance household resilience to climate change. The off-farm revenue increased the chance to improve the resilience status of the farm household by 62% and the receipt of transfer revenue by 50%. This study provides a robust method for quantifying resilience or wellbeing and its drivers and enriches our understanding of the resilience ability of farmers to climate change in a West African context. It can be useful in designing effective adaptation interventions and improving the overall wellbeing of smallholder farmers.
Drylands in developing countries account for 40% of the world's total land area and are home to 2.3 billion people, the majority of whom are dependent on agriculture UN, 2010-2020; Manap and Ismail, 2017. The growing frequency of droughts, floods, and various other forms of climatic variability is resulting in drastic adverse impacts on agricultural productivity, farm incomes, and food security in these regions (Battisti and Naylor, 2009; FAO, 2016). These phenomena destabilize the agro-ecosystems and livelihoods of millions of people, mainly in rural areas where survival depends essentially on rain-fed agriculture. Asset poverty, poor infrastructure, non-sustainable use of land and water resources, market risk, conflicts, inequities, and governance challenges particularly make the agricultural systems in Sub-Saharan Africa more vulnerable to climate change (Margaglio and Anna, 2019). The long-term adaptation strategy for agriculture depends on addressing similar issues in the short term too, recognizing the fundamental truth that adaptation is a location-specific and continuous learning process (FAO, 2008; Thornton et al., 2021). Adaptation strategies must, therefore, contribute to current development priorities, reduce vulnerability, and be in line with the shorter planning horizons of farmers. The resilience capacity of smallholder households is one of the main drivers of their ability to adapt to climate change, continue to farm, and make investments in fragile dryland regions (Oddsdóttir et al., 2013; Nébié et al., 2021).
Several development and research initiatives at local, national, and regional scales have been implemented in the sub-Saharan Africa region, aiming to contribute to building the resilience of communities and farmers and, consequently, reducing their vulnerability to climate change. Among these, a few to mention are CCAFS (Climate Change Agriculture and Food Security), USAID Feed the Future, CINSERE, CGIAR-ClimBer, and AICCRA (Accelerating the Impact of CGIAR Climate Research in Africa). During the one-decade implementation of CCAFS, climate-smart villages developed in the Kaffrine region to reach an in-depth integration between agricultural development and climate adaptation. This involved the use of climate information services and climate-smart agriculture technologies using an inclusive approach that put farmers at the heart of the process (Diaye et al., 2013; Sanogo et al., 2016; Sall et al., 2021). Currently, AICCRA is a major project supporting the scaling of climate-smart agriculture (CSA) solutions and climate information services (CIS) in six African countries, including Senegal, Mali, Ghana, Kenya, Ethiopia, and Zambia. In Senegal, the project covers the dryland regions of Kaffrine, Louga, and Thies which have been chosen because of their representativeness of the three major agro-ecological areas of Senegal (Peanut Bassin, Sylvo-pastoral area, and Niayes area) (Kumar et al., 2022). The major aim of CIS and CSA solutions and interventions promoted in Africa and other dryland regions has been to enhance resilience and adaptation of the farming systems and the farm households, along with mitigation co-benefits (Cowie et al., 2011).
Vulnerability and resilience are two complex and interrelated concepts in climate change research. Vulnerability focuses on exposure, sensitivity, and adaptive capacity before the natural shock takes place (pre-shock). On the other hand, resilience focuses on the dynamics of households and communities to respond to and recover from natural shocks (post-shock; Joakim et al., 2021). Developing an adequate measure of both vulnerability and resilience is therefore critical in supporting adaptation strategies that reduce the impact of climatic change and variability among smallholder farmers (Kumar et al., 2020). However, no systematic effort has been made to quantify the level of resilience of the targeted population to climate change and what the key determinants of resilience are at the household level. This information gap constrains the stakeholders and limits their ability to design context-specific and effective climate adaptation strategies as well as measure the changes they are achieving in terms of improving resilience while reducing vulnerability.
Therefore, the objective of this paper was to assess the level of farm households' resilience to climate change based on farmers' perceptions and to study the socio-demographic, technical, and economic factors that influence the level of households' vulnerability and resilience. Specifically, we aimed to:
- Estimate the resilience level of farm households by constructing a Composite Index of Climate Resilience (CICR);
- Analyze determinants of household vulnerability and resilience, considering various socio-demographic, technical, and economic characteristics of smallholders.
Literature reports different types of measures of resilience that differ from each other in terms of composition, geographical scope, and/or scale of analysis, for example, at the individual, community, or regional scale. A few examples of measuring resilience are individual resilience, community resilience, engineering resilience, ecological resilience, resilience in the face of a threat, resilience to disasters, psychological resilience, economic resilience, and resilience of livelihoods (Holling, 1973; Renschler et al., 2010; Winderl, 2014; Platt et al., 2016; Jones and Tanner, 2017; Giannini et al., 2021). However, the basic definition of this term is attributed to Holling (1973) in the ecological field. It is “the persistence of relationships within a system and is a measure of the ability of these systems to absorb changes of state variables, driving variables, and parameters, and persist.” He associates the term resilience with stability, which he defines as the ability of a system to return to an equilibrium state after a temporary disturbance. The more rapidly it returns and with the least fluctuation, the more stable it is. According to this definition, the ability is intrinsic to the system and comes before the disturbance. This ability will only be tested if a disturbance occurs. However, the system might not just return to its initial state following the disturbance but can change state, taking a better position for an upcoming disturbance. From this perspective, Renschler et al. (2010) conclude that resilience needs to be measured based on the initial state, and they propose a framework to define and measure resilience: the “PEOPLES” framework at the community level. This framework explicitly highlights the multidimensional nature of the resilience concept but also provides a detailed description of the process of resilience. When an extreme event occurs, there is a response from the elements of the system to recover and attenuate the effects of the shock until returning to the pre-shock situation or to a similar situation. Finally, resilience is often characterized as being composed of different processes and functions, such as iterative learning, access to knowledge, or the promotion of innovation (Jones and Boyd, 2011; Bene et al., 2015). On the other hand, the concept of vulnerability, just as abstract as resilience, tends to be understood in opposition to the latter. According to the Intergovernmental Panel on Climate Change (IPCC), it's the degree to which a system is susceptible to and unable to cope with the adverse effects of climate change, including climate variability and extremes. Vulnerability is a function of the character, magnitude, and rate of climate change and variation to which a system is exposed, its sensitivity, and its adaptive capacity (Malone, 2009). Lonescu et al. (2009) argue that defining vulnerability requires clearly stating three components: the entity that is vulnerable (the system), the stimulus to which it is vulnerable (the hazard), and the measure of the outcome of interactions between the entity and the stimulus (the valued attribute). To these three components, Fuessel (2005) added a temporal reference, which is the time period of reference for exposure.
Most of the approaches provided by the literature for measuring resilience and vulnerability are based on the construction of an index accounting in its design for many dimensions of vulnerability or resilience. The difference between the various indices lies in their purpose and the methodological approach used. Choices are made in terms of what indicator represents which dimension of the index. Then the indicators are combined using a weighted average. The weights provide the contribution of each dimension to the overall index value and are determined either by experts, as the result of a participatory exercise, a combination of both, or using the so-called objective approaches. The latter include deriving weights from a factor analysis or from econometric modeling.
The literature also tends to categorize indices as either built using objective approaches or subjective approaches. These approaches consider several socioeconomic variables, including income and social capital, to construct an index that requires the least possible judgment of the unit to which the measurement relates. Among the advantages of such approaches is the standardized and fixed nature of the measurement, which facilitates comparison. However, these require the collection of significant socio-economic data, which is costly in time and money. The choice of indicators to capture qualitative and intangible components of resilience is difficult, and it is also difficult to account for contextual elements (Ahern et al., 2006; Cutter et al., 2010; Clare et al., 2017). Subjective approaches to measuring resilience have emerged only recently. In its guide to the subjective measurement of wellbeing, the OECD mentions that there is a lot of recent evidence supporting the idea that subjective approaches to measuring wellbeing are valid, reliable, and can serve as a basis to help make policy decisions (OECD, 2013). Resilience falls well into this literature of wellbeing. Among the advantages of subjective approaches to resilience measurement is that they consider individuals' capacities to cope with the risks they face. It is a simple and robust way of measuring resilience, and the modules devoted to it in surveys are generally short and take little time to administer. Furthermore, subjective measures contribute to a better understanding of the drivers of wellbeing at the individual level. They put aside what one may believe about what wellbeing is supposed to be and concentrate only on the individual's self-assessment of his situation (Diener et al., 1985; Hoffman and Hancock, 2017). However, contrary to some objective measures like revenue, special care is needed to limit cognitive biases. This care should be oriented toward aspects like the question phrasing, the types of responses, the order of the questions, the survey mode, and context (OECD, 2013).
From an empirical point of view, Oriangi et al. (2019) studied for Uganda in the commune of Mbale the socio-economic-demographic determinants that contribute to the resilience of households to climatic hazards. They found that the ability of households to cover their daily expenses, the size of the household, and the social network of households largely determine their ability to prepare for shocks, cope with them, and adapt specifically to risks such as drought and erratic rainfall. From a methodological point of view, these authors used as indicators of resilience the answers (with the choice of answers: “probable” or “not probable”) to three different questions: “To what extent is the household able to prepare, recover, and adapt or change their source of income when faced with a long drought or erratic rainfall?” Each of these answers is then used in an individual linear model for the study of the determinants. This approach is quite like one of the approaches suggested by Jones and Tanner (2017), which includes several dimensions of resilience, in particular: the capacity to prepare for the shock, the capacity to cope with it when it occurs, and the ability to adapt and build a normal life around the effects of the shock. More specifically, Jones and Tanner (2017) define resilience as the ability of households to manage change by maintaining or transforming living standards in the face of shocks related to weather or climate events without compromising their long-term prospects. These authors opted for a subjective measurement of household resilience to climate change. Finally, subjective resilience is a self-assessment that the household makes of its own situation and of its ability to cope with and adapt to the risks.
The focus of this paper was resilience to climate risk in agriculture. First, we have done descriptive and inferential analysis to understand the characteristics of farm households as well as their vulnerability profile. Second, we formulated upstream questions that were asked to the respondents to capture various dimensions of the concept and construct resilience indicators. After reviewing the advantages and disadvantages of the different ways of asking questions to assess subjective resilience, it emerged that one of the least error-prone approaches was to ask a series of questions related to aspects known to affect disaster resilience. Resilience here covers the ability to cope with risk, to adapt, and to transform as defined by Folke et al. (2002), but also the ability to prepare before the risk occurs, while vulnerability refers to the inability to achieve the same. The basic resilience framework has been shown schematically in Figure 1 and builds on the works of Oriangi et al. (2020) in Uganda, who also proposed a set of binary questions (likely and unlikely as possible answers).
R0 to R3 steps represent the different components of the resilience-building mechanism. Before the occurrence of climate shocks, building household resilience consists of preparing (R0), which requires being proactive. Good preparation is supposed to mitigate the impacts of the shock. The shock comes and tests the ability of the household to cope (R1), which is a set of short-term measures and actions to be taken. After coping with the shock, the household should then be able to adapt (R2) to the new situation resulting from the shock. The final step is the transformative ability of the households, allowing them to innovate and find a new equilibrium state. Building on this framework, Table 1 lists five (05) questions (Q1–Q5), each of which lies in at least one component of the resilience measuring framework. The word “shock” designates one of the shocks: drought or scarcity of rain; the problem of declining soil fertility due to climate variability; high winds/dust storms. The household is the system being exposed to shocks. For each question, households were asked to express to what extent they agree with the statements according to the following response categories: “Totally agree” (2), “Partially agree” (1), “Don't know” (0), “Slightly disagree” (−1), and “Totally disagree” (−2). For example, the first question will allow for assessing the perception of the household with respect to dimension R1 (ability to cope).
The aggregation is done by a linear combination of the answers to each question for each of the shocks. The value of the indicator for the household “i” is then given by the formula:
With:
- CICR computes a Composite Index of Climate Resilience values, which are between −1 and 1;
- the value of the response given by the i-th household for the j-th question on the k-th climate shock of the indicator, verifying: −2 < Ik < 2;
- 0 < αk < 1 and , the relative importance of k-th shock.
In this study, K equals 3 and refers to the three climate risks that we considered: drought, declining soil fertility due to climate variability, and violent winds/dust storms. These three shocks turned out to be the most frequent shocks experienced by surveyed farm households. The weights αk are computed as , fk being the frequency of occurrences of the kth shock in the population and f the sum of fk over k. For the analysis of resilience and its determinants, the values of the CICR were used to build four classes of resilience separated by quartiles. Building on this as a dependent variable, we built a generalized ordered logistic regression, particularly the partial proportional odds (PPO) model for ordinal dependent variables developed by Williams (2006), to analyze the determinants of resilience. First, this model has been chosen within the available ordered logistic regressions used when the dependent variable is an ordered categorical variable. This choice is secondarily the result of a model selection process starting with the parallel lines model, whose assumptions did not hold (parallel lines). The PPO model offers the advantage of allowing for different sets of predictors to have varying effects on different thresholds of ordinal dependent variables, providing a better understanding of the relationship between variables.
This study was conducted in the intervention area of the AICCRA-SENEGAL project. The sample covered 18 villages in the regions of Kaffrine, Louga, and Thiès (see Figure 2). This AICCRA intervention area in Senegal was chosen for two reasons, namely, coverage of three agro-ecological zones and then in each zone, building on the achievements of previous projects. On this basis, three central villages were first chosen: Daga Birame in Kaffrine, Thiel in Louga, and Meouane in Thies. The other villages were then chosen around these three villages.
The data collected during the project's baseline survey conducted in June 2022 on 503 households was used for this resilience assessment study. Besides the data on resilience indicators, the exogenous variables included the Head of Household (HH)'s age and squared HH's age; diversification through cowpea, watermelon, and cattle ownership; the number of household members under 12 years old (<12 years old); the number of females engaged in agriculture; and the number of females engaged in home gardening, household's off-farm income including income transfers, ownership of assets such as television, smartphone, thresher, hoe, “charette” (horse cart), seeder, literacy and education of household members, access to training on climate smart agriculture and climate information services, the region of residence, whether the household practice livestock fattening.
The studied farm households were evenly distributed throughout the three dryland regions of Senegal covered by AICCRA, with more than 95% of households headed by men. Agriculture was the main activity for 84% of households, followed by livestock husbandry, which was practiced by at least 36% of households. On average, the age of the household head was 53 years, which indicates an aging trend among the farmers. In terms of climate risk perceptions, households were aware of the increased frequency of climatic extremes and climate change and its effects (90%). The climate risks and shocks perceived by the farm households are summarized below in Figure 3.
The drought, scarcity of rain, and declining soil fertility due to climate extremes were the two most frequent risks perceived by 96% of surveyed smallholder households. These two elements are indeed critical for households' livelihoods as their main crops, namely, millet, sorghum, groundnuts, and cowpea, are rain-fed. The effects of climate change (climate extremes) were observed mainly due to the longer dry spells during the rainy season, the shorter rainy season, and the fewer number of rainy days, all of which affected the plants' survival, growth, and yield. In certain years, even when there was enough rain, the land degradation and declining soil fertility were perceived to be a big challenge as the soils were getting poorer from year to year. Extreme rainfall events also lead to soil nitrogen leaching. The use of fertilizers becomes a necessity, and that results in additional costs. The increasing frequency of strong winds, dust, and storms was another related factor mentioned by 90% of households. Other shocks include occasional sudden flooding and increased occurrences of pest attacks, bovine disease outbreaks, and lighting.
Building on the three most important climate-related shocks and vulnerabilities, we designed the Composite Index of Climate Resilience (CICR) using the six (O6) questions mentioned in the methodology. Overall, the households studied were found to be vulnerable and achieved a low CICR score of −0.2 on a scale of −2 to +2. As indicated in Figure 4, the region of Louga was highly vulnerable and lowest on resilience, with a CICR value of −0.36, far less than the average value. Conversely, the two other regions have a lesser level of vulnerability to climate change.
The analysis of the CICR quartiles also shows that one household out of four has a level below −0.84; half of the households are below −0.23; and three households out of four are below 0.45 (see Table 1).
Using the quartile values (Q25, Q50, and Q75) as thresholds, we created four classes of resilience derived from the CICR, which are very vulnerable (25% of the population with the lowest level of resilience), vulnerable (added to the “very vulnerable” class, which gives 50% of the population with the lowest level of resilience), resilient (added to the “very resilient” class, which gives the 50% most resilient), and very resilient (25% of the population with the highest level of resilience). As a next step, smallholder households were distributed among different categories of resilience. Figure 5 presents the distribution of households across four classes of resilience and by region. The observation in the figure is consistent with the conclusions drawn from the previous figure.
The Kaffrine region was found to be relatively more resilient to climate change, with about 67% of its households being resilient or highly resilient.
Though the Thies region was the second best, with 43% of farm households as resilient, it had the relatively highest proportion of households among the three regions as highly resilient. That may present an opportunity for cross-learning among the farmers. Louga region, with 62% of households as vulnerable, remains the least resilient.
Based on focus group discussions (FGDs) with farmers and other stakeholders, a number of socio-economic-demographic farming system-level variables were identified to be included in the logistic regression as potential drivers of household resilience. The different variables included in the model are summarized in Table 2.
The model was found to be globally significant, with an overall R-squared of 16.5%. The McFadden R2 for the individual model components was 24, 20, and 18% for very vulnerable, vulnerable, and resilient household categories, respectively, which are signs of a good logistic model. The rate of good prediction (Count R2) ranged from 74 to 81%, showing that the right category is successfully predicted by the model 7–8 times out of 10.
We conducted a specification test results of which are summarized in Tables 3, 4. Without any surprise, “_hat” coefficients were all significant, which contains the information predicted by our model. As for “_hatsq” coefficients, their non-significance confirmed a good specification of our ordinal logistic regressions.
Furthermore, some additional diagnostic tests, such as the ROC curve, sensibility curve, and influentials check, were conducted to further confirm the good quality of the model. As shown in Figure 6 the area under the ROC curve is 82%, 80% and 77% respectively for models 1, 2 and 3, which support the idea of good quality of the model. The sensibility curves present the trade-off between sensibility and specificity. For the different models, the area under the ROC curve (AUC) ranged from 77 to 81%, which means a good predictive quality. Finally, we made sure to reduce, as much as possible, the effect of influential individuals in the early stages of the modeling process. The model is therefore free of the effects of atypical individuals.
The logistic regression results show a significant effect of several socio-demographic, economic, gender, and other farming system-related variables on the level of climate resilience of smallholder households (Table 5). Empirically understanding these context-specific drivers is the first step in designing appropriate resilience strategies.
The age of household head (HH) appears to have a significantly negative effect on the resilience of smallholders, with a threshold effect at the age of 59 years. This means that the older the HH, the less chance is there for the household to improve its level of resilience, so long as HH's age is <59 years. Beyond this age, it is rather the opposite effect that was observed. Our sample was made up of 30% of HH beyond the age of 59 years. The effect on a household's region of residence depends on its initial level of vulnerability or resilience. Belonging to the LOUGA region rather than KAFFRINE (taken as a reference category) makes it 3.3 times (OR = 0.32) less likely to transit from the extreme vulnerability class to any higher class and three times less likely to transit from any state of vulnerability to resilience. The resilient smallholder households belonging to THIES region compared to KAFFRINE are 1.21 times more likely to become very resilient, but 1.78 times less likely to leave the “very vulnerable” category. In sum, the region of LOUGA seems to possess inherent characteristics that result in increased vulnerability to climate change.
The number of household members (size) significantly and positively affects resilience, regardless of the level of the household on the scale of resilience. One additional member is associated with an increase in the household's chance of improving its resilience to climate change by 5% (OR = 1.05). However, the effect is not the same when the additional member has no activity while at work. Indeed, one such person reduces by 44% the chance to improve resilience (OR = 0.69).
Similarly, one additional person in the household who can read/write will improve resilience by 12%. This variable, being a proxy for the level of education of people in the household, implies that the level of education of the household helps getting out of a situation of vulnerability or improves the resilience of the household.
The household's income from off-farm activities and transfers and the possession of certain assets and equipment were key non-agriculture economic variables that influenced the level of resilience. Receiving income transfers from relatives or friends increases the household's chance of improving its resilience situation by 50% (OR = 1.50). Similarly, having an income outside agricultural activities increased this chance by 62% (OR = 1.62), particularly when households had a level of “resilience” or below. The practice of cattle fattening is a strategy most households in the region adopt to increase their income after selling the cows at festivals.
The ownership of various equipment and gadgets like threshers, and hoes positively impacted resilience, with an effect ranging from +1.48 to +4.04 times increase in the chance of improving the level of household resilience. Conversely, the possession of an Android phone or a horse cart negatively influences the chance of improving the resilience of households.
Variables aiming to capture the influence of gender were included for each household: the number of women practicing agriculture, the number of women engaged in livestock, and the number of women doing gardening. Thus, it appears that having an additional woman practicing agriculture (in strict terms and excluding gardening) reduces by 20% the chance of improving a household's resilience. At the same time, one additional woman practicing market gardening multiplied by 4.19 the chance of the household reaching the category “very resilient.” These two results tend to convey the idea that women should be encouraged to be active in activities other than rain-fed agriculture for an efficient contribution to household resilience to climate change. In our study area, women represent 56% of the individuals doing gardening.
Finally, having at least one member of the household trained in CSA and CIS has a positive and significant impact on resilience at the 1% level of significance. Training a member of the household on CSA/CIS multiplies by 2.71 the chance for the household to move to a better level of resilience, regardless of its initial status.
The findings of this study enhance our understanding of the process that strengthens or rather tends to weaken, the perceived level of resilience or vulnerability of smallholder farmers to climate change in the West African context. This study systematically measured and amply demonstrated that the resilience level of the smallholder households to climate change in the dryland regions of Senegal is considerably low. However, the proportion of smallholder households that were resilient substantially varied among the regions, from a low of 38% in Louga to 67% in Kaffrine in between was Thies. The Kaffrine region, during the past decade, has been the place for many interventions around CISs and CSA with the support of CCAFS and other programs. This might have allowed several smallholder farmers to enhance their ability to adapt to climate change through various capacity-building activities and sensitization on climate change to a relatively larger extent than in other regions (Sijmons et al., 2013; Bonilla-Findji et al., 2021).
The positive relationship found between the size of the household and the chance to have a better level of resilience when the additional member is engaged in some economic activity can be attributed to two factors, namely, economic and sociological. First, the household members in rural areas are a workforce for agriculture-related and off-farm activities. Therefore, the growing household's size was positive as long as each additional member contributed to productive activity. Second, three of 14 household members were not close relatives who established a direct social network in the home. The importance of social capital has been emphasized by Ledogar and Fleming (2008), Aldrich and Meyer (2015), Clare et al. (2017), and Carmen et al. (2022). This social capital is closely related and favors regular transfers of revenue from friends and relatives living in cities or abroad to support households in villages.
Training on CSA and CIS, education of household members, income transfer, and ownership of farm machinery (like threshers and hoes) were found to be the key global drivers influencing the climate resilience level of smallholder households. Nevertheless, a few determinants behaved differently for different categories of households; for example, households' women's engagement in home gardening was found to significantly increase the chances of moving the household from a resilient to a highly resilient category. Home gardening not only provided nutritious food to the farm family but also critical cash income for smoothing household consumption and buying farm inputs. However, under this resource-constrained dryland environment, home gardening has a positive effect only if the women of the household have the minimum required assets/inputs like access to water; otherwise, it may increase the vulnerability. Plausibly, home gardening by women in the vulnerable category did not increase the household's resilience. It may be because their poor resource status and inability to access the required infrastructure hindered them from harnessing their advantage.
The ownership of a horse-cart, which the majority of the farmers have, was found to have hurt the resilience of the smallholder household. This would need deeper analysis. Decreasing availability and increasing cost of feed and fodder for the horse, which has limited use for productive purposes, might have increased the transaction cost and made it disadvantageous for several households. Another key variable in the model, “the number of women of the household working in agriculture” had a significant and negative coefficient, suggesting its negative effect on resilience. The decision-making on major crops like millet, groundnuts, and cowpeas remains with the man, and the women just provide their labor. The greater magnitude of women's labor but the unavailability of men for timely decision-making might be affecting the outcomes of dryland crops negatively. This dimension thus needs a deeper gendered analysis.
This study assessed the resilience status of smallholder households in the dryland regions of Senegal, focusing on climate-related risks and factors influencing resilience. Key findings reveal a concerning trend of low resilience among smallholder farmers in the region, with significant variations across different farmers' groups and sub-regions. The smallholder households in the dryland region of Senegal predominantly rely on agriculture as their main source of income, and an aging trend among farmers is evident, with an average household head's age of 53 years. Climate risk perception was high, with 90% of households recognizing the increased frequency of climatic extremes and climate change impacts. Drought and declining soil fertility were the most significant risks, affecting 96% of households, particularly as their main crops are rainfed.
The Composite Index of Climate Resilience (CICR), developed as part of the present study, can be a very useful tool for quickly measuring the resilience status of farming systems. The index indicates that the farm households in the dryland region of Senegal achieved a low CICR score of −0.2 on a scale from −2 to +2 and need immediate context-specific strategies and actions to improve resilience for ensuring food and livelihood security. The study also clearly suggests differentiated climate adaptation strategies across different sub-regions, with the Louga region being the most vulnerable.
The analysis identified determinants that can significantly contribute toward household resilience, such as training farmers on CSA and CIS, education level, income transfer and ownership of farm machinery, and farm women's engagement in home gardening. This calls for enhancing targeted capacity-building efforts on CSA and CIS, along with improved access to scale-appropriate mechanization. Creating options for increased off-farm income and income transfer also needs to be part of the resilience-building strategy. The resilience outcome of certain interventions, such as women's engagement in home gardening, is not straightforward; hence, the introduction of this intervention for the vulnerable category of households might need more infrastructural and knowledge support. The negative effect of a greater number of women engaged in agriculture on resilience suggests a need to empower women, enabling them to actively play their role in farm decision-making and make greater efforts to enhance their capacity to contribute toward farm households' resilience. This suggests that one size does not fit all, and hence there is a need for careful designing of resilience-enhancing interventions.
In summary, this study underscores the urgent need for targeted interventions to enhance the resilience of smallholder farmers in Senegal's dryland regions. These interventions need to consider factors such as access to CSA and CIS, household size, education, income diversification, and gender inclusivity to improve the adaptive capacity of vulnerable communities facing climate change challenges.
The qualitative nature of this composite index of climate resilience constructed based on farmers' perceptions is its limitation to some extent. However, it would be a valuable tool for policy-makers to design resilience-enhancing interventions.
The raw data supporting the conclusions of this article will be made available by the authors, without undue reservation.
AY: Conceptualization, Data curation, Formal analysis, Writing—original draft. SK: Conceptualization, Funding acquisition, Supervision, Writing—review & editing. PH: Data curation, Writing—review & editing. OW: Project administration, Writing— review & editing. AWa: Writing—review & editing. AWh: Funding acquisition, Writing—review & editing.
The author(s) declare that financial support was received for the research, authorship, and/or publication of this article. This work was supported by the World Bank through the International Development Agency (IDA) in the AICCRA (Accelerating the Impacts of CGIAR Climate Research in Africa) project.
The authors are thankful to the Accelerating Impacts of CGIAR Climate Research in Africa (AICCRA)' project team in the Senegal cluster and to the field investigators and the community for their cooperation during the data collection.
The authors declare that the research was conducted in the absence of any commercial or financial relationships that could be construed as a potential conflict of interest.
All claims expressed in this article are solely those of the authors and do not necessarily represent those of their affiliated organizations, or those of the publisher, the editors and the reviewers. Any product that may be evaluated in this article, or claim that may be made by its manufacturer, is not guaranteed or endorsed by the publisher.
Ahern, N. R., Kiehl, E. M., Sole, M. L., and Byers, J. (2006). A review of instruments measuring resilience. Iss. Comprehens. Pediatr. Nurs. 29, 103–125. doi: 10.1080/01460860600677643
Aldrich, D. P., and Meyer, M. A. (2015). Social capital and community resilience. Am. Behav. Sci. 59, 254–269. doi: 10.1177/0002764214550299
Battisti, D. S., and Naylor, R. L. (2009). Historical warnings of future food insecurity with unprecedented seasonal heat. Science 323:2404244. doi: 10.1126/science.1164363
Bene, C., Frankenberger, T., and Nelson, S. (2015). “Design, monitoring, and evaluation of resilience interventions: conceptual and empirical considerations,” in Institute of Development Studies Working Paper, 2015, vol. 459 (Brighton: United Kingdom).
Bonilla-Findji, O., Eitzinger, A., Ouedraogo, M., Zougmoré, R. B., Läderach, P., and Sall, M. (2021). 2021- IFAD-UE/CCAFS CSA Monitoring: Kaffrine Climate-Smart Village (Senegal), 2021. Dakar: CGIAR-CCAFS. doi: 10.7910/DVN/SYIFEO
Carmen, E., Fazey, I., Ross, H., Bedinger, M., Smith, F. M., Prager, K., et al. (2022). Building community resilience in a context of climate change: the role of social capital. Ambio 51, 1371–1387. doi: 10.1007/s13280-021-01678-9
Clare, A., Graber, R., Jones, L., and Conway, D. (2017). Subjective measures of climate resilience: what is the added value for policy and programming? Glob. Environ. Change 46:17212. doi: 10.1016/j.gloenvcha.2017.07.001
Cowie, A. L., Penman, T. D., Gorissen, L., Winslow, M. D., Lehmann, J., Tyrrell, T. D., et al. (2011). Towards sustainable land management in the drylands: scientific connections in monitoring and assessing dryland degradation, climate change and biodiversity. Land Degrad. Dev. 22, 248–260. doi: 10.1002/ldr.1086
Cutter, S. L., Burton, C. G., and Emrich, C. T. (2010). Disaster resilience indicators for benchmarking baseline conditions. J. Homeland Secur. Emerg. Manag. 7:1732. doi: 10.2202/1547-7355.1732
Diaye, O., Moussa, A. S., Seck, M., Zougmoré, R. B., and Hansen, J. (2013). Communicating Seasonal Forecasts to Farmers in Kaffrine, Senegal for Better Agricultural Management. Available online at: https://www.mrfcj.org/pdf/case-studies/2013-04-16-Senegal.pdf (accessed February 15, 2023).
Diener, E., Emmons, R. A., Larsen, R. J., and Griffin, S. (1985). The satisfaction with life scale. J. Personal. Assess. 49, 71–75. doi: 10.1207/s15327752jpa4901_13
FAO (2008). The state of food insecurity in the world 2008. High food prices and food security threats and opportunities. Rome: FAO.
Folke, C., Carpenter, S., Elmqvist, T., Gunderson, L., Holling, C. S., and Walker, B. (2002). Resilience and sustainable development: building adaptive capacity in a world of transformations. J. Hum. Environ. 31, 437–440. doi: 10.1579/0044-7447-31.5.437
Fuessel, H. M. (2005). Vulnerability in Climate Change Research: A Comprehensive Conceptual Framework. Available online at: https://escholarship.org/uc/item/8993z6nm (accessed February 15, 2023).
Giannini, A., Nébié, E. K. I., Ba, D., and Ndiaye, O. (2021). Livelihood strategies shape vulnerability of households' food security to climate in Senegal. Front. Clim. 3:731036. doi: 10.3389/fclim.2021.731036
Hoffman, R. R., and Hancock, P. A. (2017). Measuring resilience. Hum. Fact. 59, 564–581. doi: 10.1177/0018720816686248
Holling, C. S. (1973). Resilience and stability of ecological systems. Ann. Rev. Ecol. Syst. 245, 1–23. doi: 10.1146/annurev.es.04.110173.000245
Joakim, E. P., Mortsch, L., and Oulahen, G. (2021). Using vulnerability and resilience concepts to advance climate change adaptation. Environ. Hazard. Resil. 13:31. doi: 10.4324/9781003171430-1
Jones, L., and Boyd, E. (2011). Exploring social barriers to adaptation: insights from Western Nepal. Glob. Environ. Change 21:126271264. doi: 10.1016/j.gloenvcha.2011.06.002
Jones, L., and Tanner, T. (2017). “Subjective resilience”: using perceptions to quantify household resilience to climate extremes and disasters. Region. Environ. Change 17, 229–243. doi: 10.1007/s10113-016-0995-2
Kumar, S., Mishra, A. K., Pramanik, S., Mamidanna, S., and Whitbread, A. (2020). Climate risk, vulnerability and resilience: supporting livelihood of smallholders in semiarid India. Land Use Pol. 97:104729. doi: 10.1016/j.landusepol.2020.104729
Kumar, S., Yessoufou, A. N. D., Houessionon, P., Worou, N., and Whitbread, A. M. (2022). Senegal Cluster Baseline Report. AICCRA report. Accelerating Impacts of CGIAR Climate Research for Africa (AICCRA). Available online at: https://cgspace.cgiar.org/bitstream/handle/10568/126562/AICCRA%20Baseline%20report%20final.pdf?sequence=4&isAllowed=y
Ledogar, R. J., and Fleming, J. (2008). Social capital and resilience: a review of concepts and selected literature relevant to aboriginal youth resilience research. Pimatisiwin 6, 25–46.
Lonescu, C., Klein, R. J. T., Hinkel, J., Kavi Kumar, K. S., and Klein, R. (2009). Towards a formal framework of vulnerability to climate change. Environ. Model. Assess. 14:116. doi: 10.1007/s10666-008-9179-x
Malone, E. L. (2009). Vulnerability and Resilience in the Face of Climate Change: Current Research and Needs for Population Information. Washington, DC: Population Action International, 1–31.
Manap, N. M. A., and Ismail, N. W. (2017). Land irrigation and food production in dryland developing countries. Int. J. Agric. For. Plant. 5, 1–14.
Margaglio, G., and Anna, C. (2019). Linking Climate Change, Migration Patterns and Vulnerability: The Case of Ndem, Senegal. Available online at: https://lup.lub.lu.se/student-papers/record/8995448/file/8995979.pdf (accessed February 15, 2023).
Nébié, E. K. I., Ba, D., and Giannini, A. (2021). Food security and climate shocks in Senegal: who and where are the most vulnerable households? Glob. Food Secur. 29:100513. doi: 10.1016/j.gfs.2021.100513
Oddsdóttir, F., Lucas, B., and Combaz, E. (2013). Measuring Disaster Resilience: GSDRC University of Birmingham.
OECD (2013). OECD Guidelines on Measuring Subjective Well-being. Paris: Organization for Economic Co-operation and Development. Available online at: https://www.oecd-ilibrary.org/economics/oecd-guidelines-on-measuring-subjective-well-being_9789264191655-en (accessed February 15, 2023).
Oriangi, G., Albrecht, F., Di Baldassarre, G., Bamutaze, Y., Mukwaya, P. I., Ardö, J., et al. (2020). Household resilience to climate change hazards in Uganda. Int. J. Clim. Change Strat. Manag. 12:59753. doi: 10.1108/IJCCSM-10-2018-0069
Oriangi, G., Bamutaze, Y., Mukwaya, P. I., Musali, P., Di Baldassarre, G., and Pilesjö, P. (2019). Testing the proposed municipality resilience index to climate change shocks and stresses in Mbale municipality in Eastern Uganda. Am. J. Clim. Change 8:5204523. doi: 10.4236/ajcc.2019.84028
Platt, S., Brown, D., and Hughes, M. (2016). Measuring resilience and recovery. Int. J. Disast. Risk Reduct. 19, 447–460. doi: 10.1016/j.ijdrr.2016.05.006
Renschler, C. S., Frazier, A. E., Arendt, L. A., Cimellaro, G. P., Reinhorn, A. M., and Bruneau, M. (2010). A Framework for Defining and Measuring Resilience at the Community Scale: The PEOPLES Resilience Framework. Buffalo, NY: MCEERBuffalo.
Sall, M., Diakhaté, P. B., and Ouédraogo, M. (2021). Mise en place de la plateforme d'innovation des chaînes de valeur agricoles climato-intelligentes dans la région de Kaffrine au Sénégal. Available online at: https://tapipedia.org/sites/default/files/mise_en_place_de_la_plateforme_dinnovation_des_chaines_de_valeur_agricoles_climato-intelligentes_dans_la_region_de_kaffrine_au_senegal.pdf (accessed February 15, 2023).
Sanogo, D., Camara, B. A., Diop, M., Ndiaye, O., Ky-Dembélé, C., Bayala, J., et al. (2016). Mise en place d'un Village Intelligent face au Climat pour la réduction des risques climatiques et de l'insécurité alimentaire à Daga-Birame, Sénégal. Guide de visite de terrain pour la Réunion du Comité Scientifique Indépendant du CCAFS. Available online at: https://core.ac.uk/download/pdf/132689819.pdf (accessed February 15, 2023).
Sijmons, K., Kiplimo, J., Förch, W., Thornton, P. K., Moussa, A. S., and Zougmoré, R. B. (2013). CCAFS site atlas - Kaffrine. CCAFS Site Atlas Series. Copenhagen: The CGIAR Research Program on Climate Change, Agriculture and Food Security (CCAFS). Available online at: https://ccafs.cgiar.org/atlas-ccafs-sites
Thornton, P., Nelson, G., Mayberry, D., and Herrero, M. (2021). Increases in extreme heat stress in domesticated livestock species during the twenty-first century. Glob. Change Biol. 27:576275762. doi: 10.1111/gcb.15825
UN (2010-2020). UN Decade for Deserts and the Fight against Desertification. Consulted on 10 April 2023. Available online at: https://www.un.org/en/events/desertification_decade/value.shtml
Williams, R. (2006). Generalized ordered logit/partial proportional odds models for ordinal dependent variables. Stata J. 6, 58–82. doi: 10.1177/1536867X0600600104
Winderl, T. (2014). Disaster Resilience Measurements: Stocktaking of ongoing efforts in developing systems for measuring resilience. United Nations Development Program-UNDP, 1–59. Available online at: http://www.preventionweb.net/files/37916_disasterresiliencemeasurementsundpt.pdf
Keywords: smallholders resilience, vulnerability, climate change, adaptation, Senegal
Citation: Yessoufou AN-D, Kumar S, Houessionon P, Worou ON, Wane A and Whitbread A (2024) Vulnerability and resilience in the face of climate changes in Senegal's drylands: measurement at the household level and determinant assessment. Front. Clim. 6:1330025. doi: 10.3389/fclim.2024.1330025
Received: 30 October 2023; Accepted: 05 March 2024;
Published: 05 April 2024.
Edited by:
Pia-Johanna Schweizer, Research Institute for Sustainability—Helmholtz Centre Potsdam, GermanyReviewed by:
Mariagrazia D'Angeli, Roma Tre University, ItalyCopyright © 2024 Yessoufou, Kumar, Houessionon, Worou, Wane and Whitbread. This is an open-access article distributed under the terms of the Creative Commons Attribution License (CC BY). The use, distribution or reproduction in other forums is permitted, provided the original author(s) and the copyright owner(s) are credited and that the original publication in this journal is cited, in accordance with accepted academic practice. No use, distribution or reproduction is permitted which does not comply with these terms.
*Correspondence: Shalander Kumar, c2hhbGFuZGVyLmt1bWFyQGljcmlzYXQub3Jn
Disclaimer: All claims expressed in this article are solely those of the authors and do not necessarily represent those of their affiliated organizations, or those of the publisher, the editors and the reviewers. Any product that may be evaluated in this article or claim that may be made by its manufacturer is not guaranteed or endorsed by the publisher.
Research integrity at Frontiers
Learn more about the work of our research integrity team to safeguard the quality of each article we publish.