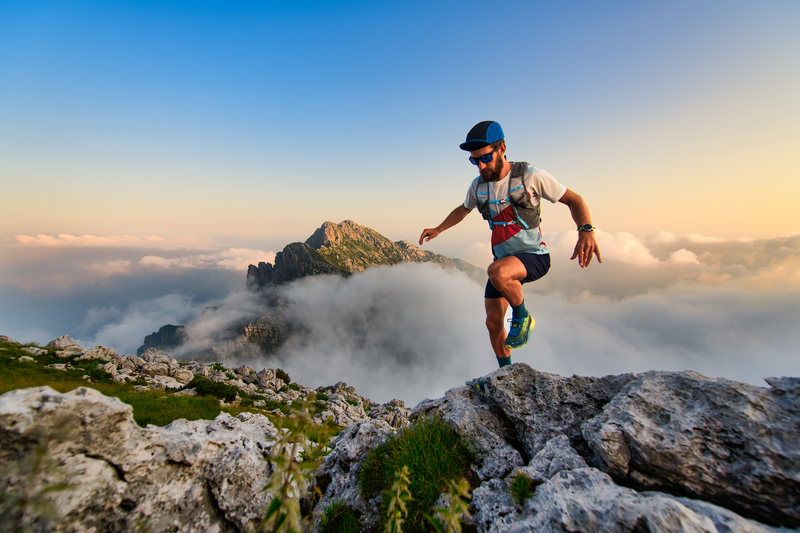
94% of researchers rate our articles as excellent or good
Learn more about the work of our research integrity team to safeguard the quality of each article we publish.
Find out more
ORIGINAL RESEARCH article
Front. Clim. , 13 November 2024
Sec. Climate Mobility
Volume 6 - 2024 | https://doi.org/10.3389/fclim.2024.1260028
This article is part of the Research Topic Climate Mobility Modeling: Methodological Advances and Future Prospects View all 5 articles
With an estimated 357.7 million internal displacements caused since 2008, weather-related disasters are a major driver of human mobility worldwide. As climate change is projected to increase the frequency and intensity of extreme weather events in many parts of the world, it is important to better understand how trends in weather patterns related to global warming have affected the intensity of disasters that have caused displacements. Here we combined observational and counterfactual climate data with global internal displacement records to estimate how climate change has affected precipitation and wind speeds at the time and location of floods and storms that led to internal displacements. We estimate that, on average, climate change increased precipitation and decreased wind speeds during such events by +3.7% and − 1.4%, respectively. However, the variability across events is considerable (±28.6 and ± 6.6%, respectively), highlighting the large signal of natural variability of the weather system as compared to the global warming signal. Our results caution against overstating the role of climate change in displacement-inducing disasters in the past, especially compared to socio-economic and development factors of vulnerability and adaptive capacity that determine whether weather-related hazards turn into disasters.’
Weather-related disasters represent a major cause of global population displacements. Between 2008 and 2023, floods, storms, wildfires, droughts, and extreme temperatures triggered around 357.7 million internal displacements worldwide (IDMC, 2024). Projected increases in the number of extreme precipitation events (Bador et al., 2018; Donat et al., 2016), heatwaves (Meehl and Tebaldi, 2004; Sillmann et al., 2013), and in the intensity of tropical cyclones (Knutson et al., 2010; Walsh et al., 2016) in coming decades due to global warming have amplified concerns over the potential consequences for involuntary migration. While an increasing direct and indirect role of global warming for human mobility is undisputed (IPCC, 2014; Piguet et al., 2011), the specifics of how many people are likely to move when and where as the result of climate change impacts remain subject to large uncertainties (Beyer et al., 2023). A series of estimates placing the number of future international migrants driven by sudden-or slow-onset climate-related hazards in the order of hundreds of millions to a billion (Barnett, 2001; Biermann and Boas, 2017; Christian, 2007; Myers, 2002) have been met with criticism, characterising them as inflated, alarmist, and lacking scientific rigour (Black, 2001; Castles, 2002; Gemenne, 2011; Kolmannskog, 2008). Biased estimates of the role of climate change in displacements triggered by disasters pose a serious issue as they can compromise effective decision making by national and international stakeholders, including humanitarian, legal, development, and security agencies (Ferris, 2020; Stott and Walton, 2013). In addition, over-estimation of climate change impacts in the political discourse entails the risk of diverting attention from important socio-economic issues underlying the local vulnerability to displacements, and hindering disaster risk reduction and preparedness measures (Hulme, 2011; Lahsen and Ribot, 2022; Raju et al., 2022; Ribot, 2014).
The past two decades have seen substantial progress on the attribution of observed extreme weather events and disasters to anthropogenic climate change (Clarke et al., 2023; Otto, 2017; Stott et al., 2016; Swain et al., 2020). Strong evidence has been presented in particular for human influence on temperature extremes and heatwaves (Christidis et al., 2011; Christidis et al., 2005; Fischer and Knutti, 2015; Perkins et al., 2012; Stott, 2003). For extreme precipitation events and storms, evidence tends to be more mixed (Stott et al., 2016). While at the global scale, a significant increase in the frequency of extreme precipitation events linked to global warming has been established (Lehmann et al., 2015; Papalexiou and Montanari, 2019), robust signals for intensifications of observed precipitation extremes emerge only for a minority of land areas, and statistical trends are often nonsignificant unless based on spatially aggregated data, due to a high degree of natural variability (Alexander et al., 2006; Christidis et al., 2005). Analyses of human influence on observed storm patterns, including the best-studied instance of tropical cyclones, has also produced mixed results (Knutson et al., 2010; Patricola and Wehner, 2018), though, there is evidence that the proportion of intense tropical cyclones has increased due to global warming (Seneviratne et al., 2021). As in the case of precipitation extremes, large internal variability and spatial heterogeneity complicate attribution exercises.
While the biophysical impacts of climate change are increasingly well understood (Pörtner et al., 2022), human responses to climate-related hazards, in particular with regard to mobility, continue to be subject to major uncertainties (Horton et al., 2021). Here, we contribute to closing this gap by investigating the effect that the changing climate has had on weather-related disasters that led to internal displacements. Classical event attribution approaches (Otto, 2017; Stott et al., 2016; Swain et al., 2020) are not suitable for the scale of this exercise, as that they involve tailored in-depth analyses of individual events. Climate model simulations based on factual and counterfactual atmospheric greenhouse gas concentration (Eyring et al., 2016) are not suitable either: although they may replicate the overall internal variability of the weather system, the timings of simulated and observed specific weather events generally do not coincide, so that such data cannot easily be linked to social events. Impact attribution framing provides a solution to this problem by rephrasing the attribution question to ‘What has been the influence of the long-term mean climatic trend observed in the past century on weather phenomena?’ (Mengel et al., 2021; O’Neill et al., 2022). Removing this long-term trend from observational data allows for a first-order approximation of the role of climate change in extreme events.
Here, we examined the impact of the changing climate on displacement-inducing disasters in the form of floods and storms, the two major causes of weather-related internal displacements worldwide – accounting for 97% of documented events (IDMC, 2024) as the result of their oftentimes sudden, immediate, widespread, and difficult-to-mitigate impacts compared to other hazards. By definition, internal displacements occur when “persons or groups of persons [are] forced or obliged to flee or to leave their homes or places of habitual residence, in particular as a result of or in order to avoid the effects of [drivers including] natural or human-made disasters, and who have not crossed an internationally recognised State border” (IOM, 2019). Here, we used geocoded global records of flood-and storm-induced internal displacements during 2017–2021, and compared precipitation and wind speed, respectively, observed during the events, against corresponding counterfactual data assuming no historic climate change trends since 1900. The distribution across events of the differences between the factual and counterfactual data provides a first estimate of the degree to which human influence on climate has globally affected the severity of displacement-inducing floods and storms until now.
Records of disaster-induced internal displacements worldwide, including information on the country, type of disaster, event start date, and number of displacements, have been compiled by the Internal Displacement Monitoring Centre since 2008 (IDMC, 2024), though, systematic sub-national geographical information has been incorporated by monitoring experts only since 2017. For these events, geographical coordinates were defined as the centroid of the specified administrative unit or units, using the OSMNames database (UN Statistics Division, n.d.). Details on the monitoring methodology—involving the collection of data from sources including national and subnational government authorities, UN agencies and other international organisations, related global databases, civil society organisations, news media outlets, and other entities—and caveats associated with the displacement data are available.1 Our final dataset includes 3,640 flood-induced and 2,284 storm-induced events across 154 and 136 countries, associated with a total of 39,477,764 and 49,993,210 recorded internal displacements, respectively, between 2017 and 2021. In terms of spatial resolution, 3.3% of these events were documented at national-level, 66.0% at the first sub-national administrative level, 0.1% at the second sub-national level, and 30.7% at the third sub-national level. Across events, the median size of the administrative units recorded as the locations of the disaster is 11,026 km2 (with a median absolute deviation across events of 10,943 km2); making the spatial resolutions of the displacement and of the climate data (see below) be of a similar order of magnitude.
Our analysis uses historical global gridded observational climate based on three alternative reanalysis datasets: 20crv3-ERA5 (Hersbach et al., 2020; Slivinski et al., 2019), GSWP3-W5E5 (Cucchi et al., 2020), and 20CRv3-W5E5 (Compo et al., 2011; Slivinski et al., 2019). These data are available on a 0.5° grid (~3,100 km2 at the equator), at daily resolution, and across 2017–2021 in the case of 20crv3-ERA5 and 2017–2019 for the other two datasets. For each of the three datasets, Mengel et al. (2021) generated counterfactual daily climate data, in which long-term trends related to global mean temperature change since 1900 were removed from climate variables. To achieve this, Mengel et al. (2021) estimated global-warming-related shifts in the statistical distribution of a given climate variable as follows. Assuming a smooth annual cycle, the parameters of the distributions were characterised by a set of Fourier modes, whose coefficients were represented by linear models that include global mean temperature change as a predictor. Counterfactual equivalents of these distributions then followed from setting global mean temperature change to its value in 1901. Finally, counterfactual daily climate time series were derived via quantile mapping, which ensured that the factual and counterfactual data for a given day maintain the same rank within their respective statistical distributions. In particular, and importantly, the timing of extremes is preserved in the counterfactual, which allows for a consistent like-for-like comparison of the observed and counterfactual data at the timing of the social impact. As such, our method of comparing observational and counterfactual climate circumvents the challenges associated with the scalability of individual event attribution and with the non-comparability of extreme events obtained from climate model simulations based on factual and counterfactual scenarios (see Introduction). The observational and counterfactual climate data are available.2
Here we did not attempt to further spatially or temporally downscale the observational and counterfactual data. While extreme precipitation and wind speed events can be very localised, our analysis is fundamentally constrained by the coarse resolution of the displacement data (see previous section); higher-resolution climate data would therefore not improve our ability to assess precipitation and wind speed at displacement sites. Independently of this issue, increasing the spatial or temporal resolution of the climate data would entail its own uncertainties (Lafferty and Sriver, 2023), likely even more so in the case of the counterfactual data. In this regard, we consider the 0.5°, daily resolution of the data a good compromise between robustness and granularity. Lastly, our analysis examines the relative change between observed and counterfactual conditions (see next section); as a result, some downscaling methods, such as the delta method that applies a constant high-resolution anomaly to the lower-resolution data (Beyer et al., 2020; Navarro-Racines et al., 2020), would not affect our results.
For each disaster causing internal displacements, we compared the observational against the counterfactual climate data at the relevant time and location to estimate to what extent the historical climate trend has increased or decreased the relevant variable. Denoting the observed and the counterfactual daily precipitation at location x and date by and , respectively, we computed for each flood-induced internal displacement event the relative change in precipitation at location and date that is related to climate change as
Thus, means that the observed precipitation at location and date was double the precipitation estimated for the no-climate-change scenario, while means that it was half.
An important caveat associated with our approach for floods is that we consider precipitation at the location of the disaster recorded by IDMC (2023) while in reality it could have been the result of heavy precipitation further upstream. Given the lack of information on the specifics of the displacement-inducing floods, including their exact location within administrative units, combined with the high complexity of geo-hydrological dynamics at the global scale, here, we take the simplifying assumption that the climate-change-induced change in precipitation at the disaster location is a reasonable proxy for the change in the precipitation that caused a flood. The relatively coarse spatial resolution of our climate data (~3,100 km2 at the equator) may lend support to this assumption. Philip et al. (2020) discuss alternative approaches.
We defined the average effect of climate change on precipitation during displacement-inducing floods as the weighted median of the estimated changes across all events, , where we chose the weight assigned to each estimate as the logarithm of the number of displacements, thus assigning greater weight to events that affected more people. The weighted median absolute deviation as well as summary statistics used to generate violin and boxplots representing the distribution of the across events were computed accordingly.
For storm-induced displacements, we proceeded analogously, computing the relative change in wind speed related to climate change as
where and denote the observed and counterfactual near-surface wind speed, respectively, at location and date . Displacement-weighted summary statistics of the set were computed as in the case of precipitation.
Given the extended temporal coverage of the 20crv3-ERA5 observational and corresponding counterfactual climate data, our main results of the estimated distributions of and focus on this dataset. In a supplementary analysis, we provide the corresponding distributions based on GSWP3-W5E5 and 20CRv3-W5E5 climate to assess the robustness of our estimates.
In addition to a global analysis across all flood and storm displacement locations , we determined the climate change effect across UN subregions (UN Statistics Division, n.d.) to examine patterns across smaller spatial scales.
Flood and storm displacements can be the result of extreme weather that takes place not only on the recorded dates of displacements but already in the days leading up to events. To assess the robustness of our analysis in this regard, we conducted a supplementary analysis estimating the climate change effect not for weather conditions on the displacement date but based on the observed and counterfactual total precipitation across the 3 days leading up to flood displacements (including the displacement date) and based on the observed and counterfactual mean wind speed across the 2 days leading up to storm displacements.
Because IDMC (2023) uses the same terminology as the original source when reporting displacement figures and the available data do not include multiple hazard types, it is possible for the cause of an event to be categorised as ‘storm’ when in reality the main driver of displacement was flooding associated with the storm. To examine potential consequences of this issue for our findings, in a supplementary analysis, we also estimated the effect of climate change on precipitation instead of wind speed at the location and date of storm displacements.
The estimated effects of climate change on daily precipitation and wind speeds during disaster-inducing flood and storm events are characterised by a high degree of spatial heterogeneity (Figure 1). Using 20crv3-ERA5 climate data, we estimate that climate change increased precipitation during displacement-inducing floods by an across-event average of +3.7% and decreased wind speed during displacement-inducing storms by −1.4% (Figures 2C,D). The variation across events (median absolute deviation of ±28.6% for precipitation, ±6.6% for wind speeds) is substantial in relation to the median effects.
Figure 1. Estimated effect of climate change on daily precipitation and wind speeds during floods and storms that triggered internal displacements in 2017–2021. Marker colours represent the increase or decrease in the relevant variable related to climate change, relative to the counterfactual scenario. Marker sizes represent the displacement magnitude.
Figure 2. Estimated changes in observed relative to counterfactual daily precipitation and wind speeds during floods and storms that caused internal displacements. (A,C) Scatter plots of the event-specific estimated relative differences and related to climate change, plotted against time and with displacement magnitudes represented by marker sizes. (B,D) Distributions of and across all events, represented by violin and boxplots. Blue lines, black boxes, and black whiskers represent weighted medians, upper and lower quartiles, and 10th and 90th percentiles, respectively.
The estimated changes in wind speeds related to climate change did not change significantly over time (Figure 2C; p = 0.53). Those for precipitation increased significantly over time (Figure 2A; p = 0.031); however, this significance disappears when removing the very few data points from the year 2017 (p = 0.059).
There is a moderate spatial variability of the estimated climate change effect across different world regions (Figure 3). Median effects on precipitation during flood displacements range from −13% for Eastern Asia to +40% for Northern Africa, while median effects on wind speed during storm displacements range from −13% for Melanesia to +3.8% for Western Asia. In most cases, region-specific interquartile ranges overlap with the previously reported median effects of +3.7% and − 1.4% for precipitation and wind speed estimated at the global level.
Figure 3. Estimated changes in observed relative to counterfactual precipitation and wind speeds during displacement-inducing disasters disaggregated by world region. Bar chats are analogous to those in Figures 2B,D. Only regions with more than three data points are shown.
The climate change effect distributions estimated based on 20crv3-ERA5 observational and counterfactual climate data (Figure 1) are very similar for the alternative GSWP3-W5E5 and 20CRv3-W5E5 data available only up to 2019 (see Methods; Supplementary Figure 1). Estimating climate change effects based on observational and counterfactual conditions during the days leading up to events rather than solely on the recorded date (see Methods) also led to very similar results as those in our main analysis (Supplementary Figure 2). Considering precipitation rather than wind speed during displacement events classified as storm-induced resulted in an estimated average effect of climate change on precipitation of +7.0% (±27.6%; Supplementary Figure 3), somewhat higher than the average effect estimated during flood displacements.
Our analysis suggests that mean climate trends since 1900 increased precipitation at the time and location of floods that caused internal displacements by an average of +3.7% and decreased wind speeds during displacement-inducing storms by an average of −1.4% (Figures 2B,D). However, in both cases, the variability in the effect of climate change across events—estimated at ±28.6 and ± 6.6%, respectively—is considerable in relation to the average, highlighting the large natural variability of the weather system and the fact that global warming is one of many determinants (Schwarzwald and Lenssen, 2022). The marked variability of the estimated climate change effects in some cases within small geographical regions (Figure 1) is the result of spatial heterogeneities even in the long-term average of the climate change effect, e.g., in Eastern Africa, Mengel et al. (2021) and of the daily time scale of our analysis, which can introduce divergent results between two events that are close in location but distant in time even when there is a broadly homogeneous long-term spatial pattern in the region. The relatively weak signal identified in our analysis for precipitation and wind speed in the special case of displacement-inducing disaster events is consistent with earlier attribution literature finding weakly or non-significant trends in the intensity of precipitation extremes in many areas as the result of high natural variability (Alexander et al., 2006; Christidis et al., 2005) and no strong signal in the historical effect of climate change on the intensity of storms (Knutson et al., 2010; Patricola and Wehner, 2018).
Our results challenge disaster displacement framings that place too strong an emphasis on climate change. Earlier work has pointed out—without in any way diminishing the role of global warming on extreme weather patterns or the importance of attribution science—a risk of climate-centric disaster framing downplaying the infrastructural, socio-economic, and political factors that determine local vulnerability and adaptive capacity, and the degree to which environmental hazards turn into disasters (IDMC, 2021; Lahsen and Ribot, 2022; Raju et al., 2022). Our quantitative analysis, suggesting highly spatio-temporally heterogeneous effects of historical climate trends on the intensity of precipitation and wind during flood-and storm-induced displacement events, at the global, regional, and subregional level (Figures 1–3), cautions against overstating the overall role of climate change in these events.
While our analysis did not suggest a robust increase in the examined climate change effect over time (Figures 2A,C), this may be a consequence of the relatively short period for which internal displacement data are available. Long-term future projections of increases in extreme precipitation and storms in many parts of the world (Pörtner et al., 2022) highlight that it remains important to closely monitor the role of climate change in displacement-inducing disasters events, and assess its contribution relative to other factors.
Given that the counterfactual climate data used in our analysis is based on the removal of the long-term mean climatic trend observed in the past century, it is important to reiterate that climate extremes may evolve differently from mean climate and that trends in extreme percentiles may not be fully captured in our approach. To examine this issue in depth, extreme wind and precipitation trends would need to be analysed individually. An additional natural next step to our analysis would be the incorporation of explicit flood models (Kumar et al., 2023; Trigg et al., 2021) instead of using local precipitation as a proxy. While these models come with their own uncertainties, comparing flood simulations based on observed and counterfactual climate would provide a more mechanistically rooted framework, taking into account interacting climate drivers (Zscheischler and Lehner, 2022), for assessing climate change impacts.
The relationship between climate-related disasters and human displacement is highly complex and context-specific (Beyer et al., 2023; Schewel et al., 2024). It is therefore important to re-emphasise that our results refer only to the effect of global warming on climate factors that led to internal disaster displacements, not on the magnitudes of displacement. The latter would require integrating observed and counterfactual climate into comprehensive quantitative models of climate-related displacements. While global-scale efforts in this direction have been made for slow-onset climate mobility (Benveniste et al., 2022; Clement et al., 2021)—though, not without limitations (Beyer et al., 2023)—fast-onset disaster displacement modelling is still at a very early stage. With this said, current trends in the increase of relevant data as well as advances in modelling techniques leave room for optimism with regard to a better quantitative understanding of the effect of climate change on disaster displacements.
The data analysed in this study is subject to the following licenses/restrictions: observational and counterfactual climate data used in this study are available from https://data.isimip.org/. Disaster displacement data are available from https://www.internal-displacement.org/database/displacement-data; additional geolocation data is available from the authors upon reasonable. Requests to access these datasets should be directed to Maria Teresa Miranda Espinosa, bWFyaWEuZXNwaW5vc2FAaWRtYy5jaA==.
RB: Conceptualization, Data curation, Formal analysis, Investigation, Methodology, Visualization, Writing – original draft. MTME: Data curation, Investigation, Writing – review & editing. SP: Data curation, Investigation, Writing – review & editing. MM: Data curation, Investigation, Writing – review & editing. AM: Investigation, Supervision, Writing – review & editing.
The author(s) declare that no financial support was received for the research, authorship, and/or publication of this article.
The authors declare that the research was conducted in the absence of any commercial or financial relationships that could be construed as a potential conflict of interest.
The author(s) declared that they were an editorial board member of Frontiers, at the time of submission. This had no impact on the peer review process and the final decision.
All claims expressed in this article are solely those of the authors and do not necessarily represent those of their affiliated organizations, or those of the publisher, the editors and the reviewers. Any product that may be evaluated in this article, or claim that may be made by its manufacturer, is not guaranteed or endorsed by the publisher.
The Supplementary material for this article can be found online at: https://www.frontiersin.org/articles/10.3389/fclim.2024.1260028/full#supplementary-material
Alexander, L. V., Zhang, X., Peterson, T. C., Caesar, J., Gleason, B., Klein Tank, A., et al. (2006). Global observed changes in daily climate extremes of temperature and precipitation. J. Geophys. Res.-Atmos. 111:6290. doi: 10.1029/2005JD006290
Bador, M., Donat, M. G., Geoffroy, O., and Alexander, L. V. (2018). Assessing the robustness of future extreme precipitation intensification in the CMIP5 ensemble. J. Clim. 31, 6505–6525. doi: 10.1175/JCLI-D-17-0683.1
Barnett, M. (2001). Humanitarianism with a sovereign face: UNHCR in the global undertow. Int. Migr. Rev. 35, 244–277. doi: 10.1111/j.1747-7379.2001.tb00013.x
Benveniste, H., Oppenheimer, M., and Fleurbaey, M. (2022). Climate change increases resource-constrained international immobility. Nat. Clim. Chang. 12, 634–641. doi: 10.1038/s41558-022-01401-w
Beyer, R., Krapp, M., and Manica, A. (2020). An empirical evaluation of bias correction methods for palaeoclimate simulations. Clim. Past 16, 1493–1508. doi: 10.5194/cp-16-1493-2020
Beyer, R. M., Schewe, J., and Abel, G. J. (2023). Modeling climate migration: Dead ends and new avenues. Front. Clim. 5:649. doi: 10.3389/fclim.2023.1212649
Biermann, F., and Boas, I. (2017). “Towards a global governance system to protect climate migrants: taking stock” in Research handbook on climate change, migration and the law eds. B. Mayer, F. Crépeau (Cheltenham, UK and Northampton MA, USA: Edward Elgar Publishing).
Black, R. (2001). Environmental refugees: myth or reality? UNHCR Working Paper No. 34. (Geneva, Switzerland: UNHCR), 1–19.
Castles, S. (2002). Environmental change and forced migration: Making sense of the debate. UNHCR Working Paper No. 70. (Geneva, Switzerland: UNHCR). 1–4.
Christidis, N., Stott, P. A., and Brown, S. J. (2011). The role of human activity in the recent warming of extremely warm daytime temperatures. J. Clim. 24, 1922–1930. doi: 10.1175/2011JCLI4150.1
Christidis, N., Stott, P. A., Brown, S., Hegerl, G. C., and Caesar, J. (2005). Detection of changes in temperature extremes during the second half of the 20th century. Geophys. Res. Lett. 32:32. doi: 10.1029/2005GL023885
Clarke, B., Otto, F., and Jones, R. (2023). When don’t we need a new extreme event attribution study? Clim. Chang. 176:60. doi: 10.1007/s10584-023-03521-4
Clement, V., Rigaud, K. K., de Sherbinin, A., Jones, B., Adamo, S., Schewe, J., et al. (2021). Groundswell part 2: Acting on internal climate migration. Washington DC, USA: World Bank.
Compo, G. P., Whitaker, J. S., Sardeshmukh, P. D., Matsui, N., Allan, R. J., Yin, X., et al. (2011). The twentieth century reanalysis project. Q. J. R. Meteorol. Soc. 137, 1–28. doi: 10.1002/qj.776
Cucchi, M., Weedon, G. P., Amici, A., Bellouin, N., Lange, S., Müller Schmied, H., et al. (2020). WFDE5: bias-adjusted ERA5 reanalysis data for impact studies. Earth Syst. Sci. Data 12, 2097–2120. doi: 10.5194/essd-12-2097-2020
Donat, M. G., Lowry, A. L., Alexander, L. V., O’Gorman, P. A., and Maher, N. (2016). More extreme precipitation in the world’s dry and wet regions. Nat. Clim. Chang. 6, 508–513. doi: 10.1038/nclimate2941
Eyring, V., Bony, S., Meehl, G. A., Senior, C. A., Stevens, B., Stouffer, R. J., et al. (2016). Overview of the coupled model Intercomparison project phase 6 (CMIP6) experimental design and organization. Geosci. Model Dev. 9, 1937–1958. doi: 10.5194/gmd-9-1937-2016
Ferris, E. (2020). Research on climate change and migration where are we and where are we going? Migr. Stud. 8, 612–625. doi: 10.1093/migration/mnaa028
Fischer, E. M., and Knutti, R. (2015). Anthropogenic contribution to global occurrence of heavy-precipitation and high-temperature extremes. Nat. Clim. Chang. 5, 560–564. doi: 10.1038/nclimate2617
Gemenne, F. (2011). Why the numbers don’t add up: a review of estimates and predictions of people displaced by environmental changes. Glob. Environ. Chang. 21, S41–S49. doi: 10.1016/j.gloenvcha.2011.09.005
Hersbach, H., Bell, B., Berrisford, P., Hirahara, S., Horányi, A., Muñoz-Sabater, J., et al. (2020). The ERA5 global reanalysis. Q. J. R. Meteorol. Soc. 146, 1999–2049. doi: 10.1002/qj.3803
Horton, R. M., de Sherbinin, A., Wrathall, D., and Oppenheimer, M. (2021). Assessing human habitability and migration. Science 372, 1279–1283. doi: 10.1126/science.abi8603
Hulme, M. (2011). Reducing the future to climate: a story of climate determinism and reductionism. Osiris 26, 245–266. doi: 10.1086/661274
IPCC, (2014). “Climate Change 2014: Synthesis Report”. In Contribution of Working Groups I, II and III to the Fifth Assessment Report of the Intergovernmental Panel on Climate Change. eds. R.K. Pachauri and L.A. Meyer. (Geneva, Switzerland: IPCC). 151.
Knutson, T. R., McBride, J. L., Chan, J., Emanuel, K., Holland, G., Landsea, C., et al. (2010). Tropical cyclones and climate change. Nat. Geosci. 3, 157–163. doi: 10.1038/ngeo779
Kolmannskog, V. (2008). Future floods of refugees: A comment on climate change, conflict and forced migration. Oslo, Norway: Norwegian Refugee Council.
Kumar, V., Sharma, K. V., Caloiero, T., Mehta, D. J., and Singh, K. (2023). Comprehensive overview of flood modeling approaches: a review of recent advances. Hydrology 10:141. doi: 10.3390/hydrology10070141
Lafferty, D. C., and Sriver, R. L. (2023). Downscaling and bias-correction contribute considerable uncertainty to local climate projections in CMIP6. Npj Clim. Atmospheric Sci. 6, 1–13. doi: 10.1038/s41612-023-00486-0
Lahsen, M., and Ribot, J. (2022). Politics of attributing extreme events and disasters to climate change. Wiley Interdiscip. Rev. Clim. Chang. 13:e750. doi: 10.1002/wcc.750
Lehmann, J., Coumou, D., and Frieler, K. (2015). Increased record-breaking precipitation events under global warming. Clim. Chang. 132, 501–515. doi: 10.1007/s10584-015-1434-y
Meehl, G. A., and Tebaldi, C. (2004). More intense, more frequent, and longer lasting heat waves in the 21st century. Science 305, 994–997. doi: 10.1126/science.1098704
Mengel, M., Treu, S., Lange, S., and Frieler, K. (2021). ATTRICI v1. 1–counterfactual climate for impact attribution. Geosci. Model Dev. 14, 5269–5284. doi: 10.5194/gmd-14-5269-2021
Myers, N. (2002). Environmental refugees: a growing phenomenon of the 21st century. Philos. Trans. R. Soc. Lond. Ser. B Biol. Sci. 357, 609–613. doi: 10.1098/rstb.2001.0953
Navarro-Racines, C., Tarapues, J., Thornton, P., Jarvis, A., and Ramirez-Villegas, J. (2020). High-resolution and bias-corrected CMIP5 projections for climate change impact assessments. Sci. Data 7:7. doi: 10.1038/s41597-019-0343-8
O’Neill, B., van Aalst, M., Zaiton Ibrahim, Z., Berrang-Ford, L., Bhadwal, S., Buhaug, H., et al. (2022). “Key risks across sectors and regions”. In Climate Change 2022: Impacts, Adaptation and Vulnerability. Contribution of Working Group II to the Sixth Assessment Report of the Intergovernmental Panel on Climate Change. eds. H.-O. Pörtner, D.C. Roberts, M. Tignor, E.S. Poloczanska, K. Mintenbeck, A. Alegría, et al. (Cambridge, UK and New York, NY, USA: Cambridge University Press). 2411–2538, doi: 10.1017/9781009325844.025
Otto, F. E. (2017). Attribution of weather and climate events. Annu. Rev. Environ. Resour. 42, 627–646. doi: 10.1146/annurev-environ-102016-060847
Papalexiou, S. M., and Montanari, A. (2019). Global and regional increase of precipitation extremes under global warming. Water Resour. Res. 55, 4901–4914. doi: 10.1029/2018WR024067
Patricola, C. M., and Wehner, M. F. (2018). Anthropogenic influences on major tropical cyclone events. Nature 563, 339–346. doi: 10.1038/s41586-018-0673-2
Perkins, S., Alexander, L., and Nairn, J. (2012). Increasing frequency, intensity and duration of observed global heatwaves and warm spells. Geophys. Res. Lett. 39:361. doi: 10.1029/2012GL053361
Philip, S., Kew, S., van Oldenborgh, G. J., Otto, F., Vautard, R., van der Wiel, K., et al. (2020). A protocol for probabilistic extreme event attribution analyses. Adv. Stat. Climatol. Meteorol. Oceanogr. 6, 177–203. doi: 10.5194/ascmo-6-177-2020
Piguet, E., Pécoud, A., and De Guchteneire, P. (2011). Migration and climate change: an overview. Refug. Surv. Q. 30, 1–23. doi: 10.1093/rsq/hdr006
Pörtner, H.-O., Roberts, D. C., Adams, H., Adler, C., Aldunce, P., Ali, E., et al. (2022). Climate change 2022: Impacts, adaptation and vulnerability. Switzerland: IPCC Geneva.
Raju, E., Boyd, E., and Otto, F. (2022). Stop blaming the climate for disasters. Commun. Earth Environ. 3:1. doi: 10.1038/s43247-021-00332-2
Ribot, J. (2014). Cause and response: vulnerability and climate in the Anthropocene. J. Peasant Stud. 41, 667–705. doi: 10.1080/03066150.2014.894911
Schewel, K., Dickerson, S., Madson, B., and Nagle Alverio, G. (2024). How well can we predict climate migration? A review of forecasting models. Front. Clim. 5:125. doi: 10.3389/fclim.2023.1189125
Schwarzwald, K., and Lenssen, N. (2022). The importance of internal climate variability in climate impact projections. Proc. Natl. Acad. Sci. 119:e2208095119. doi: 10.1073/pnas.2208095119
Seneviratne, S.I., Zhang, X., Adnan, M., Badi, W., Dereczynski, C., and Di Luca, A., (2021). “Weather and climate extreme events in a changing climate”. In Climate Change 2021: The Physical Science Basis. Contribution of Working Group I to the Sixth Assessment Report of the Intergovernmental Panel on Climate Change. eds. V. Masson-Delmotte, P. Zhai, A. Pirani, S.L. Connors, C. Péan, S. Berger, N. et al. (Cambridge, United Kingdom and New York, NY, USA: Cambridge University Press). 1513–1766, doi: 10.1017/9781009157896.013
Sillmann, J., Kharin, V., Zhang, X., Zwiers, F., and Bronaugh, D. (2013). Climate extremes indices in the CMIP5 multimodel ensemble: part 1. Model evaluation in the present climate. J. Geophys. Res.-Atmos. 118, 1716–1733. doi: 10.1002/jgrd.50203
Slivinski, L. C., Compo, G. P., Whitaker, J. S., Sardeshmukh, P. D., Giese, B. S., McColl, C., et al. (2019). Towards a more reliable historical reanalysis: improvements for version 3 of the twentieth century reanalysis system. Q. J. R. Meteorol. Soc. 145, 2876–2908. doi: 10.1002/qj.3598
Stott, P. A. (2003). Attribution of regional-scale temperature changes to anthropogenic and natural causes. Geophys. Res. Lett. 30:324. doi: 10.1029/2003GL017324
Stott, P. A., Christidis, N., Otto, F. E., Sun, Y., Vanderlinden, J., van Oldenborgh, G. J., et al. (2016). Attribution of extreme weather and climate-related events. Wiley Interdiscip. Rev. Clim. Chang. 7, 23–41. doi: 10.1002/wcc.380
Stott, P. A., and Walton, P. (2013). Attribution of climate-related events: understanding stakeholder needs. Weather 68, 274–279. doi: 10.1002/wea.2141
Swain, D. L., Singh, D., Touma, D., and Diffenbaugh, N. S. (2020). Attributing extreme events to climate change: a new frontier in a warming world. One Earth 2, 522–527. doi: 10.1016/j.oneear.2020.05.011
Trigg, M. A., Bernhofen, M., Marechal, D., Alfieri, L., Dottori, F., Hoch, J., et al. (2021). “Global flood models” in Global drought and flood: Observation Modeling, and Prediction. eds. H. Wu, D.P. Lettenmaier, Q. Tang and P.J. Ward, (Hoboken NJ, USA: John Wiley & Sons, Inc.) 181–200.
UN Statistics Division. (n.d.). Standard country or area codes for statistical use (M49) [WWW document]. Available at: https://unstats.un.org/unsd/methodology/m49/ (Accessed June 26, 2024).
Walsh, K. J., McBride, J. L., Klotzbach, P. J., Balachandran, S., Camargo, S. J., Holland, G., et al. (2016). Tropical cyclones and climate change. Wiley Interdiscip. Rev. Clim. Chang. 7, 65–89. doi: 10.1002/wcc.371
Keywords: climate change, attribution, internal displacement, migration, flood, storm
Citation: Beyer R, Miranda Espinosa MT, Ponserre S, Mengel M and Milan A (2024) Heterogeneous effects of climate change on displacement-inducing disasters. Front. Clim. 6:1260028. doi: 10.3389/fclim.2024.1260028
Received: 17 July 2023; Accepted: 07 October 2024;
Published: 13 November 2024.
Edited by:
Roman Hoffmann, International Institute for Applied Systems Analysis (IIASA), AustriaReviewed by:
Alex de Sherbinin, CIESIN, Columbia University, United StatesCopyright © 2024 Beyer, Miranda Espinosa, Ponserre, Mengel and Milan. This is an open-access article distributed under the terms of the Creative Commons Attribution License (CC BY). The use, distribution or reproduction in other forums is permitted, provided the original author(s) and the copyright owner(s) are credited and that the original publication in this journal is cited, in accordance with accepted academic practice. No use, distribution or reproduction is permitted which does not comply with these terms.
*Correspondence: Robert Beyer, cmJleWVyQGlvbS5pbnQ=
Disclaimer: All claims expressed in this article are solely those of the authors and do not necessarily represent those of their affiliated organizations, or those of the publisher, the editors and the reviewers. Any product that may be evaluated in this article or claim that may be made by its manufacturer is not guaranteed or endorsed by the publisher.
Research integrity at Frontiers
Learn more about the work of our research integrity team to safeguard the quality of each article we publish.