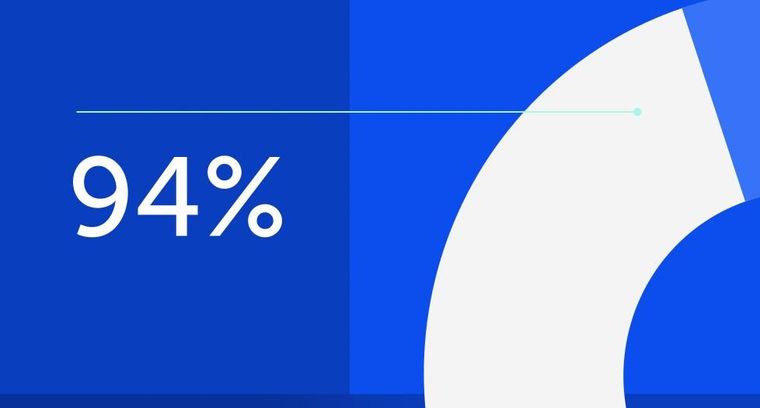
94% of researchers rate our articles as excellent or good
Learn more about the work of our research integrity team to safeguard the quality of each article we publish.
Find out more
ORIGINAL RESEARCH article
Front. Clim., 06 December 2023
Sec. Climate, Ecology and People
Volume 5 - 2023 | https://doi.org/10.3389/fclim.2023.1284829
This article is part of the Research TopicSeagrasses and Climate Change: Challenges and OpportunitiesView all 6 articles
Mangrove forests are degraded by extreme climatic events worldwide, often leaving behind dead standing stems called “ghost forests”. Ghost forests may provide opportunities for seagrass colonization but there is limited research into the conditions found within these ecosystems, or whether they provide a suitable habitat for seagrasses. This study aimed to characterize the environmental conditions within mangrove ghost forests, determine whether conditions are suitable for seagrass survival, and identify whether seagrass was present within the ghost forests of Moreton Bay, Queensland. Six study locations within mangrove ghost forests adjacent to live mangrove forests and seagrass meadows were selected and biophysical conditions within these habitats over the six sites were assessed. Two of the six study sites were found to have live seagrass present within the ghost forests (Godwin Beach and South Stradbroke). Suitable water temperature was linked to the presence and abundance of seagrass within mangrove ghost forests. Mangrove characteristics, including canopy cover, diameter at breast height, and stem densities, contributed to variation in the environment among the three habitats, suggesting that light is a key factor limiting seagrass colonization into live or ghost mangrove forests. Overall, these results suggest that ghost forests can provide suitable habitats for seagrasses, and degraded mangroves may transition to seagrass under future sea level rise scenarios.
There is widespread evidence of the growing impacts of extreme climate events and sea level rise on mangrove cover (Sippo et al., 2018; Goldberg et al., 2020). Some of the most significant dieback areas in Australia are in the Gulf of Carpentaria, where more than 7,400 hectares of mangroves perished in late 2015 and early 2016 (Duke et al., 2017). Mortality events are often due to periods of extreme conditions, including storms, droughts, and changes in hydrology with extreme El Niño, la Niña events (Lovelock et al., 2017; Sippo et al., 2018; Chung et al., 2023). Mangrove mortality often leads to the formation of “ghost forests” where recruitment of replacement mangroves can fail due to rolling deadwood (Duke et al., 2021) or other unsuitable conditions (e.g., subsidence that leads to increased inundation) (Cahoon et al., 2006). The dead trunks of ghost forests can remain standing for decades as evidenced in Moreton Bay (Quandamooka) where mangrove dieback is shown in satellite imagery from 2003 (Duke et al., 2010) and dead stems are still standing two decades later (e.g., in the ghost forest sites in this study).
Ghost forests may provide habitat for seagrasses, however little is known about how these dead trees affect coastal morphology, interact with hydrodynamics and their suitability for plants and animals. However, when mangroves die subsidence of the sediment occurs, resulting in loss of elevation as the root systems degrade (Cahoon et al., 2006; Friess et al., 2019). It can be inferred that given the bed level in mangrove ghost forests subsides due to below-ground biomass decomposition (Lang'at et al., 2014), the water depth will increase. An increase in water depth may potentially decrease mangrove recruitment as survival and growth of propagules may diminish with increasing inundation, however this increased inundation may improve suitability for seagrass growth (Boer, 2007). In some cases, the aboveground root systems within ghost forests remain (Krauss et al., 2018) and may attenuate waves, creating a low-wave energy environment that could facilitate seagrass recruitment and growth (Boer, 2007). Moreover, reduced wave energy may contribute to reducing turbidity and increased light penetration in the water column which can favor seagrass growth (Adams et al., 2016). Light is also expected to increase on the sediment surface when the mangrove canopy dies, which may increase suitability for seagrass growth. Therefore, mangrove ghost forests may potentially create high-light, shallow, low-wave energy environments suitable for seagrass growth (Figure 1).
Figure 1. Inferred conditions within mangrove ghost forests that may be suitable for seagrass growth based on known conditions within seagrasses and unsuitable conditions within live mangrove habitats. With vector art modified from Annie Carew (seagrass) and Tracey Saxby (mangroves), Integration and Application Network (https://ian.umces.edu/media-library/).
In addition to environmental suitability for seagrass growth in mangrove ghost forests, the availability of seagrass propagules may also limit seagrass recruitment in ghost forests. The availability of propagules is linked to the dispersal of seagrass propagules from neighboring sites. Dispersal is therefore linked to the distance to live meadows and meadow productivity (McMahon et al., 2014) and the size of the meadows (Rivers et al., 2011). Therefore we may expect that ghost forests in close proximity to productive (high cover and high density) seagrass meadows are more likely to be colonized by seagrass than those that are far from seagrass seed sources.
As climate change progresses and sea level rise accelerates, seagrasses are proposed to migrate landward, occupying newly inundated habitat, maintaining their position in the intertidal zone (Saunders et al., 2013). However, this is only likely if suitable habitat is available. Thus, mangrove mortality with extreme events or sea level rise, might provide opportunities for seagrass to migrate landward. However, the biophysical conditions within ghost forests and their suitability for seagrass growth have not been assessed. To assess the likelihood of seagrass' potential to colonize mangrove ghost forests we characterized the environmental conditions in mangrove ghost forests of Moreton Bay and investigated whether seagrass recruitment had occurred.
This study was conducted within Moreton Bay (Quandamooka), Queensland, Australia which is a semi-enclosed bay protected by Moreton Island (Mulgumpin) and North Stradbroke Island (Minjerribah) to the east (Figure 2). Moreton Bay has seven mangrove species covering more than 12,000 ha in 2012 (Lovelock et al., 2019) and seven seagrass species with 19,000 ha of habitat (Roelfsema et al., 2009) dominated by mixed-species meadows (Maxwell et al., 2019). Moreton Bay has a subtropical climate with a mean annual rainfall of 1,479 mm with wet summers and drier winters. Moreton Bay has a tidal range of approximately 2.8 m (measured at the Brisbane Bar), except for South Stradbroke Island, which has a tidal range of approximately 2 m (measured at the Gold Coast Seaway) (Department of Transport Main Roads, 2023). During the spring and summer seasons, Moreton Bay experiences dominant wind patterns that are characterized by strong winds from the south-east and north-east directions with the winter season dominated by weaker westerly winds.
Figure 2. Locality of ghost forest study sites, live mangroves (Bunting et al., 2022) and seagrass habitats (Short, 2021; Kovacs et al., 2022) within Moreton Bay.
Mangrove dieback has been observed in Moreton Bay (Figure 2). Between 2004 and 2007, 3,379 ha of mangrove loss was observed (19% of all tidal wetland areas in Moreton Bay) of which 2,627 ha was associated with subsiding soils that created ponds and 752 ha of the dieback that was not associated with subsidence (Duke et al., 2010). Six study sites with dead standing mangrove stems (ghost forests), as well as adjacent live mangroves and seagrass were selected for this study to investigate whether seagrass incursion into ghost forests may be linked to biophysical factors of their environment. “Adjacent” mangrove and seagrass habitats were within 100 m of the nearest edge of the ghost forest. The sites varied in exposure to the dominant SE wind direction. The northernmost sites (Godwin Beach and Beachmere) had a fetch of around 60 km, the central sites within the Bay (Boondall Wetlands, Wynnum Wetlands, Amity Point) had a fetch of around 45 km, and South Stradbroke with a fetch of 5 km. All sites were generally protected with shallow, low-energy conditions; however, Beachmere, Boondall Wetlands and Wynnum Wetlands are also influenced by riverine outflows.
This study investigated the characteristics of three habitats at each of the six sites, (1) mangrove ghost forests, (2) live mangrove forests, and (3) seagrass meadows. Mangrove ghost forests were identified through a combination of past studies (Duke et al., 2010; Baltais, 2014), and inspection of satellite images where areas of defoliated mangroves can clearly be identified (Google Earth Pro 7.3.6.9345, 2023) followed by ground-truthing at each site. Adjacent live mangroves and seagrass sites were selected using local maps [for mangroves using Accad et al. (2016)] and seagrass using maps of Kovacs et al. (2022). Maps of global seagrass (Short, 2021) were used for the area around South Stradbroke Island where there is no local mapping.
To characterize the three habitats within the six study locations we assessed eight variables (see Sections 2.4–2.5). Data were collected in three plots within the ghost forests and live mangrove forests and along three 50 m transects within the seagrass meadow (Figure 3A).
Figure 3. (A) Spatial representation of experimental setup showing the Amity Point site with three ecosystem types. Mangrove sites were either ghost forests or live and sampled using circular plots, while seagrass was sampled along transects [image from Google Earth (2017)], (B) seagrass growing in a ghost forest at Godwin Beach, and (C) ghost forest at low tide on South Stradbroke Island.
At each mangrove site, mangrove species and tree density were measured within three 7 m radial plots (153.94 m2), arranged parallel to the shore at approximately the same elevation within the intertidal zone following the methods of Howard et al. (2014). Individual trees (either live or dead) and the number of stems per plot were counted, as well as the diameter at breast height (DBH) of each tree within the plots.
Seagrass presence or absence at all sites was assessed following the established Seagrass Watch monitoring method (McKenzie et al., 2003) where quadrats are placed every 5 m along a shore-normal transect, and seagrass presence or absence, cover and species composition are observed and recorded. The presence or absence of seagrass wrack within each site was also recorded. At each seagrass site, three 50 m transect was laid out perpendicular to the shore. Transects were positioned parallel and approximately 25 m apart. Using a 0.5 m x 0.5 m quadrat, the seagrass was assessed every 5 m along the transect, assessing species composition and percentage cover (McKenzie et al., 2003).
Seagrass area associated with the broader site context (beyond the local transects described above) was assessed in a 250 m radius circular plot (expressed in hectares) to more broadly capture the relative cover and density of the seagrass (based on local observations of meadow sizes within Moreton Bay) in ArcGIS Pro (Esri Inc, 2021). A buffer of 250 m was chosen as this is the approximate distance for seed and ramet dispersal by species common in Moreton Bay (McMahon et al., 2014). We calculated two metrics, (1) the total seagrass area (in hectares) within the plot and (2) the proportion of the area within plot that had seagrass cover, expressed as percent cover.
In addition to assessing the plant communities we measured physical conditions (air temperature, sea temperature, light availability, sediment type and turbidity) within the three different habitat types (ghost forests, live mangroves, and seagrass meadows). Our survey occurred over six weeks, from mid-July to mid-August 2022.
Air temperature (°C) was measured using a EUTECH EcoScan digital thermometer and was recorded 2 m above the ground level and at least 1.5 m above the sea level at the center of the mangrove plot or seagrass transect. Water temperature was recorded using a YSI and Horiba 2000 water-quality probe at a depth of 15-30 cm. Light conditions at each mangrove location were estimated as percentage canopy cover (%) measured using a Model-A Statistical Forest Densiometer (Lemmon, 1956) that was used as a proxy for available light. In the seagrass meadows there was no overlying mangrove canopy and thus light availability was 100%. Across all sites, point-measurements of light and air and water temperatures within each habitat were taken in the morning during the same stage of the tidal cycle to ensure comparability. Three replicate measurements of light, air and water temperature were taken at random locations within each plot.
The sediment grain size composition at each site was determined using the Wentworth (1922) classification by collecting a 30 mL sediment sample within each plot or transect. These samples were transported to the laboratory and analyzed using a Malvern Particle Mastersizer 2000, which uses laser diffraction to measure the particle size (μm) as well as the particle size distribution of materials. Water quality was assessed by measuring turbidity (in Nephelometric Turbidity Units, NTU) using a YSI and Horiba 2000 water-quality probe.
Hydrodynamic parameters used to characterize each site included the average significant wave height under average wave conditions and the fetch length. The significant wave heights from multiple directions were obtained from a process-based Simulating WAves Nearshore (SWAN) model of Moreton Bay with a 100 m grid resolution for each of the sites. The maximum fetch length was determined from aerial imagery, measured across the largest unobstructed expanse of water for each of the sites.
The “time since mangrove death” was estimated by observing historical satellite imagery using Google Earth Pro Google Earth Pro 7.3.6.9345 (2023), progressively viewing older satellite images to identify when the ghost forests were intact (not defoliated). Accuracy of this method is limited by the frequency of satellite imagery captured in each area.
All analyses were conducted using R version 4.1.1 (R Core Team, 2021).
We used linear mixed-effects models to compare biophysical variables between the three habitat types. Each model was fit with a continuous biophysical variable as a response variable, and either a two-level or three-level categorical habitat type predictor. We modeled eight biophysical variables measured at the plot level that had intra-habitat variation. Air temperature, sea temperature, sediment grain size, turbidity and seagrass cover were compared among all three habitat types, while canopy cover, stem density and DBH were compared between live mangrove and ghost forest habitats. All models were fit with site-level random intercepts to adjust models for site-based environmental variation, fit using the lmer function of the lme4 package (Bates et al., 2015). We conducted pairwise comparisons between habitat types using the emmeans package (Lenth, 2023), evaluated model performance via the performance package (Lüdecke et al., 2021) and model diagnostics using DHARMa (Hartig, 2022).
Given the low sample size and potential non-linear responses, we evaluated potential predictors of seagrass colonization into ghost forests using a Random Forest classification model (Wright and Ziegler, 2017). The models were fit with 500 trees with a target node size of 10, measuring variable importance using the Gini index (Boulesteix et al., 2012). Model performance was evaluated using Brier's out-of-the-bag prediction error scores.
The response variable for random forest models was seagrass presence-absence in ghost forest plots. We modeled seagrass presence as a function of five ecological variables that potentially increased colonization probability: time since forest death (years), mean seagrass cover in the three adjacent seagrass transects (local) (%), seagrass cover area in 250 m radial plots (ha), canopy cover (%) and the presence or absence of the stilted mangrove Rhizophora stylosa.
We also included four environmental predictors in the model. Some environmental variables correlated strongly (Supplementary Figure 1), so we included only four that represented axes of environmental conditions: sea temperature, natural log of turbidity, sediment grain size and the highest wave height (across north-east, east and south-east directions). Finally, we included site identity as a categorical predictor of seagrass colonization.
Across the six study sites, three mangrove species were observed: Avicennia marina, R. stylosa, and Ceriops australis. All six sites had A. marina present, while R. stylosa was present at four of the sites (Godwin Beach, Wynnum Wetlands, Amity Point and South Stradbroke), and C. australis was found at two sites (Wynnum Wetlands and Boondall Wetlands). Seagrass was predominantly composed of Zostera muelleri, which was found in all seagrass plots and mangrove plots with seagrass present. Two other seagrass species were observed. Halophila ovalis was observed in seagrass sites at Amity Point, South Stradbroke, Wynnum and in one plot at Boondall, as well as two of the ghost forest plots in South Stradbroke. Cymodocea serrulata was rarer, observed only in one Amity Point and two South Stradbroke seagrass plots.
Seagrass plots had c. 1.75°C cooler sea temperatures than mangrove plots (Table 1, Figure 4A). Compared to live mangrove plots, seagrass plots had 1.4°C warmer air temperature and much sediment size; sediment size differed between ghost forests and seagrass plots, but air temperature was similar (Table 1, Figure 4). Seagrass plots, as expected, contained much higher seagrass cover than both mangrove habitats (Table 1, Figure 4B).
Table 1. Mean values with standard deviation for each of the biophysical conditions measured within ghost forests, live mangroves and seagrass meadows across the six study sites.
Figure 4. Raw biophysical observations (faded points), population-level means (bordered points) and 95% confidence intervals (error bars) across plots in three habitat types: living mangroves, ghost forests and seagrass for (A) sea surface temperature, (B) seagrass cover, (C) air temperature, (D) canopy cover, (E) sediment size, (F) stem density, (G) turbidity, and (H) diameter at breast height. Means were estimated using linear mixed effect models fit with random intercepts for each study site (Supplementary Figure 2). Model performance and pairwise comparisons between habitat types (including significance) are displayed in Table 1. Canopy cover, stem density and diameter at breast height were not measured in seagrass plots.
Live mangrove and ghost forests were similar in most biophysical parameters, including stem density and DBH (Table 1, Figure 4). Canopy cover was much higher in live mangrove plots (Table 1, Figure 4D); canopy cover within the ghost forests was made up of defoliated trunks and was low (mean = 14.5%) compared to live mangrove forests (74.5%) (Supplementary Table 1). This canopy cover difference was reflected in 1.3°C lower air temperature in live mangrove plots (Table 1). While seagrass cover was higher in ghost forests than live mangroves, it was not significantly higher (Table 1, Figure 4B).
Seagrass was found in five ghost forest plots in two sites (Godwin Beach and South Stradbroke) and in one live mangrove plot in Amity Point. Seagrass cover in the live mangrove plot was 0.05%. In ghost forests seagrass cover varied from 4.67–42.5%, averaging 29.0% (in plots with seagrass present). Seagrass wrack was observed in 12 (66.7%) ghost forest and 11 (61.1%) live mangrove plots.
The strongest predictor of seagrass colonization of ghost forests was the cover of seagrass in adjacent seagrass plots. The probability of seagrass presence in ghost forests increased from 18% to 45% where seagrass plots exceeded c. 40% of benthic cover (Figure 5B). We predicted slightly higher probability of seagrass colonization with greater seagrass coverage around the ghost forest plot and higher canopy cover. Both of these relationships were only apparent at > c. 5 ha of seagrass coverage and 45% cover (Figures 5C, D). Forests that died recently appeared more likely to exhibit colonization, but this may be due to Godwin Beach (with complete seagrass colonization of ghost forests), which was the most recent ghost forest to die (Supplementary Figure 3).
Figure 5. Predicted probability of seagrass presence in ghost mangrove plots across eight biophysical variables, estimated via Random Forest classification. Red lines in (A–D) and (F–I) are predicted probabilities across each predictor, holding all other variables at their mean. Red shading reflects standard errors in the predictions. (E, J) Probability for prediction based on Rhizophora stylosa presence and site identity is shown in red, with red representing standard errors.
Abiotic conditions were generally poor predictors of seagrass colonization in ghost forests, but predicted greater probability of colonization in plots with small sediment size, high wave energy and cool sea temperatures (Figures 5F, H, I).
Overall the random effect model correctly classified seagrass colonization patterns, but predicted a higher than observed probability of colonization in Wynnum and lower than observed in Godwin beach (Supplementary Figure 4).
Seagrass was found growing within mangroves at three sites, within ghost forests at Godwin Beach and South Stradbroke (Figure 3B), and live mangroves at Amity Point (Figure 3C), suggesting that it can migrate into ghost forests under specific conditions. Ghost forest plots with seagrass present had the highest seagrass cover in the adjacent area (Supplementary Figure 5). Although the total local area of seagrass present (seagrass outside of the seagrass plots) (Supplementary Figure 5) did not have a clear effect on the presence of seagrass within the ghost mangrove forests. This may indicate that seagrass colonization within ghost forests depends on the abundance of seagrass (seagrass cover) in the adjacent meadows than the dispersal distance (seagrass proximity to ghost forests). In other locations, colonization of sediment by seagrass has been linked to the presence of sexual propagules as well as through detached rhizome fragments (Marbà et al., 2004). Dispersal via seeds has been shown to be species-specific whereby Z. marina seeds disperse with negative buoyancy and have a limited dispersal range (Orth et al., 1994) compared with Posidonia australis which remains buoyant for hours and can drift tens of kilometers, driven by wind (Ruiz-Montoya et al., 2012). Our data therefore suggests that healthy populations of seagrass are important for seagrass colonization of mangrove ghost forests but colonization may be limited by the dispersal mechanisms of the local seagrass populations.
The presence of seagrass wrack within more than half of the ghost forest and live mangrove plots indicates that seagrass ramets and seeds are dispersed into these areas as has been observed in other locations (Balestri et al., 2011). However, the limited live seagrass present in many of the sites that had seagrass wrack and lack of colonization in sites with seagrass cover (e.g., Wynnum: Supplementary Figure 3), suggest factors other than dispersal limit seagrass growth in mangrove ghost forests.
Low wave energy conditions are required for seagrass survival to prevent the uprooting of the plant (Uhrin and Turner, 2018) and to give rise to low turbidity conditions that permit photosynthesis and growth of seagrass (Table 1). With the exception of Wynnum, our data suggest that there may be a threshold of turbidity for seagrass growth as seagrass was only found in ghost forests and live mangrove plots where the turbidity was less than 6 NTU, much lower than the average for all the ghost forests and live mangroves. While turbidity measured in NTU cannot be directly converted to Secchi depth measurements, empirical studies of the turbidity limits for seagrass growth in Moreton Bay varied between 2.3 and 5.2 m (Samper-Villarreal et al., 2016) which is relatively low and consistent with our results.
The only live mangrove site where seagrass was present was Amity Point, where the mangrove species within the plot included both A. marina and R. stylosa. Both ghost forest sites with seagrass present had A. marina and R. stylosa mangroves in the plot (Godwin Beach and South Stradbroke), while the three sites without any live seagrass (Beachmere, Boondall and Wynnum Wetlands) did not have R. stylosa present. R. stylosa has root architecture that may facilitate seagrass growth or its presence may be linked to environmental conditions suitable for seagrass, for example, low elevation and frequent tidal inundation. The link between tree species and seagrass presence requires further study.
Canopy cover, stem density, DBH and sea temperature were significant predictors of seagrass presence and seagrass cover (glm, p ≤ 0.05) confirming that light levels are a key factor limiting where seagrass can exist (Adams et al., 2016). With no foliage on the dead trees, sunlight can penetrate through to the sediment, whilst the remaining trunks provide some protection from wave energy. Given that mangrove dead wood can influence tidal flow patterns (Mugi et al., 2022) and their woody structure can passively trap mangrove propagules (McKee et al., 2007), the standing stems of ghost forests may in a similar way facilitate seagrass recruitment by moderating wave energy.
Of the three seagrass species that were found across the study sites, only two were present within the ghost forests: Z. muelleri and H. ovalis. Moreton Bay has five seagrass phanerogamic communities including one which is comprised of Z. muelleri and H. ovalis [Community 1 in Young and Kirkman (1975)]. This community was observed within locations with high variability in light (turbidity) and salinity conditions, and growing in coarse sand (Young and Kirkman, 1975). While Z. muelleri and H. ovalis are not typically described as stress-tolerant species, they are colonizing species, able to rapidly recover from disturbance (Kilminster et al., 2015) which may increase survival in the varied conditions of ghost forests. Thus, in other regions where these small, colonizing species are not present, for example in the Mediterranean where Posidonia oceanica dominate (Telesca et al., 2015) or tropical sites that are dominated by Enhalus acoroides [e.g. Xincun Bay, China (Twomey et al., 2021)], the capacity of seagrass to occupy mangrove ghost forests may be limited.
The identification of seagrass colonizing ghost forests offers new insight for managers of potential transitions among coastal ecosystems as sea level rise progresses. For example, areas that have low intertidal mangroves may anticipate a transition to ghost forests and seagrass with sea level rise. While the standing dead stems of the mangroves may decline over time as mangrove wood decomposes [as shown by historical change studies (Duke et al., 2010)], seagrass cover may protect soil carbon stocks (Ricart et al., 2020) and promote accretion and burial of remaining mangrove woody material (Krause-Jensen et al., 2019). Protection of carbon stocks and burial of wood would reduce CO2 emissions with mangrove degradation and thereby contribute to continued function of the habitat as a carbon sink (Rosentreter et al., 2023). These observations may reduce the perceived risks of sea level rise on blue carbon stocks (Lovelock and Reef, 2020), and instead indicate that soil carbon stocks may be secured if environmental conditions are suitable for seagrass growth. Thus, reduced nutrient and sediment pollution (and associated turbidity) are important for seagrass persistence, and also for protecting mangrove soil carbon. Further research could investigate the dynamics of carbon stocks in ghost forests, which would provide data to support the refinement of Australia's blue carbon method (Lovelock et al., 2023) and other market-based carbon methodologies for coastal wetlands that encompass transitions among coastal ecosystems over time with sea level rise.
While further research is needed, restoration practitioners may consider seagrass restoration in ghost forests a viable option. Ghost forest sites that may be suitable for seagrass restoration would have low canopy cover, low stem densities and water temperatures that are similar to that in adjacent meadows, and with a combination of mangrove species present. Additionally, these types of sites are most likely to need few restoration interventions if they are adjacent to dense seagrass meadows. By expanding the focus of seagrass restoration areas to include mangrove ghost forests, practitioners may increase the likelihood of successful restoration outcomes and contribute to the conservation and restoration of seagrass habitats under changing future sea level rise scenarios.
The original contributions presented in the study are publicly available. This data can be found here: Twomey, Alice J; Remmerswaal, Amelia; Lovelock, Catherine E: Seagrass presence and absence in mangrove ghost forests in Moreton Bay, Australia. PANGAEA, https://doi.org/10.1594/PANGAEA.963902.
R code to reproduce all results is available at Zenodo (Staples 2023).
Timothy Staples. (2023). TimothyStaples/ghost-forests: v1.1 (v1.1). Zenodo. https://doi.org/10.5281/zenodo.10202192.
AT: Conceptualization, Formal analysis, Investigation, Methodology, Visualization, Writing—original draft, Writing—review & editing. TS: Formal analysis, Visualization, Writing—review & editing. AR: Investigation, Writing—original draft. AW: Investigation, Writing—review & editing. CL: Funding acquisition, Resources, Supervision, Writing—review & editing.
The author(s) declare financial support was received for the research, authorship, and/or publication of this article. This research was supported by The University of Queensland's School of the Environment and the Australian Research Council award FL200100133.
The authors declare that the research was conducted in the absence of any commercial or financial relationships that could be construed as a potential conflict of interest.
All claims expressed in this article are solely those of the authors and do not necessarily represent those of their affiliated organizations, or those of the publisher, the editors and the reviewers. Any product that may be evaluated in this article, or claim that may be made by its manufacturer, is not guaranteed or endorsed by the publisher.
The Supplementary Material for this article can be found online at: https://www.frontiersin.org/articles/10.3389/fclim.2023.1284829/full#supplementary-material
Accad, A., Li, J., Dowling, R., and Guymer, G. (2016). “Mangrove and associated communities of Moreton Bay, Queensland, Australia: change in extent 1955-1997-2012” in Queensland Herbarium, Department of Science. Washington, D.C.: Information Technology and Innovation 129.
Adams, M. P., Hovey, R. K., Hipsey, M. R., Bruce, L. C., Ghisalberti, M., Lowe, R. J., et al. (2016). Feedback between sediment and light for seagrass: where is it important? Limnol. Oceanogr. 61, 1937–1955. doi: 10.1002/lno.10319
Balestri, E., Vallerini, F., and Lardicci, C. (2011). Storm-generated fragments of the seagrass Posidonia oceanica from beach wrack–a potential source of transplants for restoration. Biol. Conserv. 144, 1644–1654. doi: 10.1016/j.biocon.2011.02.020
Baltais, S. (2014). Using Objected Based Image Analysis to Improve our Understanding about the Factors Driving Change in a Coastal Landscape Amity Point, North Stradbroke Island. Brisbane: The University of Queensland.
Bates, D., Mächler, M., Bolker, B., and Walker, S. (2015). Fitting linear mixed-effects models using lme4. J. Stat. Softw. 67, 1–48. doi: 10.18637/jss.v067.i01
Boer, d. W. F. (2007). Seagrass-sediment interactions, positive feedbacks and critical thresholds for occurrence: a review. Hydrobiologia 591, 5–24. doi: 10.1007/s10750-007-0780-9
Boulesteix, A. L., Janitza, S., Kruppa, J., and König, I. R. (2012). Overview of random forest methodology and practical guidance with emphasis on computational biology and bioinformatics. Wiley Interdis. Rev. 2, 493–507. doi: 10.1002/widm.1072
Bunting, P., Rosenqvist, A., Hilarides, L., Lucas, R. M., Thomas, N., Tadono, T., et al. (2022). Global mangrove extent change 1996–2020: global mangrove watch version 3.0. Remote Sens. 14, 3657. doi: 10.3390/rs14153657
Cahoon, D. R., Hensel, P. F., Spencer, T., Reed, D. J., McKee, K. L., and Saintilan, N. (2006). Coastal wetland vulnerability to relative sea-level rise: wetland elevation trends and process controls. Wetlands Nat. Res. Manage. 12, 271–292. doi: 10.1007/978-3-540-33187-2_12
Chung, C. T., Hope, P., Hutley, L. B., Brown, J., and Duke, N. C. (2023). Future climate change will increase risk to mangrove health in Northern Australia. Commun. Earth & Environm. 4, 192. doi: 10.1038/s43247-023-00852-z
Department of Transport and Main Roads (2023). Maritime Safety Queensland Tide Tables Standard Port Tide Times. Queensland, Maritime Safety Queensland.
Duke, N., Haller, A., Brisbane, S., Wood, A., and Rogers, B. (2010). “‘Sinking Centres' in Moreton Bay mangroves. Maps showing areas of unusual anoxic ponds and mangrove dieback in tidal wetlands of the bay area in 2003-08,” in Report to H & A, QPIF, DEEDI, Queensland by Marine Botany Group. Brisbane: University of Queensland, 223.
Duke, N., Mackenzie, J., Hutley, L., Staben, G., and Brouke, A. (2021). “Assessing the Gulf of Carpentaria mangrove dieback 2017–2019,” in NESP Final Report 1 (Townsville, QLD: James Cook University).
Duke, N. C., Kovacs, J. M., Griffiths, A. D., Preece, L., Hill, D. J., Van Oosterzee, P., et al. (2017). Large-scale dieback of mangroves in Australia's Gulf of Carpentaria: a severe ecosystem response, coincidental with an unusually extreme weather event. Marine Freshwat. Res. 68, 1816–1829. doi: 10.1071/MF16322
Friess, D. A., Rogers, K., Lovelock, C. E., Krauss, K. W., Hamilton, S. E., Lee, S. Y., et al. (2019). The state of the world's mangrove forests: past, present, and future. Annu. Rev. Environ. Resour. 44, 89–115. doi: 10.1146/annurev-environ-101718-033302
Goldberg, L., Lagomasino, D., Thomas, N., and Fatoyinbo, T. (2020). Global declines in human-driven mangrove loss. Glob. Chang. Biol. 26, 5844–5855. doi: 10.1111/gcb.15275
Hartig, F. (2022). DHARMa: Residual Diagnostics for Hierarchical (Multi-Level/Mixed) Regression Models. R package version 0.4.6. Available online at: http://florianhartig.github.io/DHARMa/
Howard, J., Hoyt, S., Isensee, K., Pidgeon, E., and Telszewski, M. (2014). Coastal Blue Carbon: Methods for Assessing Carbon Stocks and Emissions Factors in Mangroves, Tidal Salt Marshes, and Seagrass Meadows. Arlington, VA: Conservation International, Intergovernmental Oceanographic Commission of UNESCO, International Union for Conservation of Nature.
Kilminster, K., McMahon, K., Waycott, M., Kendrick, G. A., Scanes, P., McKenzie, L., et al. (2015). Unravelling complexity in seagrass systems for management: Australia as a microcosm. Sci. Total Environ. 534, 97–109. doi: 10.1016/j.scitotenv.2015.04.061
Kovacs, E. M., Roelfsema, C., Udy, J., Baltais, S., Lyons, M., and Phinn, S. (2022). Cloud processing for simultaneous mapping of seagrass meadows in optically complex and varied water. Remote Sens. 14, 609. doi: 10.3390/rs14030609
Krause-Jensen, D., Serrano, O., Apostolaki, E., Gregory, D., and Duarte, C. (2019). Seagrass sedimentary deposits as security vaults and time capsules of the human past. Ambio 48, 325–335. doi: 10.1007/s13280-018-1083-2
Krauss, K. W., Demopoulos, A. W., Cormier, N., From, A., McClain-Counts, J. P., Lewis, I. I. I., et al. (2018). Ghost forests of Marco Island: Mangrove mortality driven by belowground soil structural shifts during tidal hydrologic alteration. Estuar. Coast. Shelf Sci. 212, 51–62. doi: 10.1016/j.ecss.2018.06.026
Lang'at, J. K. S., Kairo, J. G., Mencuccini, M., Bouillon, S., Skov, M. W., Waldron, S., et al. (2014). Rapid losses of surface elevation following tree girdling and cutting in tropical mangroves. PLoS ONE 9, e107868. doi: 10.1371/journal.pone.0107868
Lemmon, P. E. (1956). A spherical densiometer for estimating forest overstory density. Forest Sci. 2, 314–320. doi: 10.1093/forestscience/2.4.314
Lovelock, C. E., Accad, A., Dowling, R. M., Duke, N., and Yip, S. (2019). “Mangroves and saltmarshes of Moreton Bay,” in Moreton Bay Quandamooka & Catchment 299.
Lovelock, C. E., Adame, M. F., Bradley, J., Dittmann, S., Hagger, V., Hickey, S. M., et al. (2023). An Australian blue carbon method to estimate climate change mitigation benefits of coastal wetland restoration. Restor. Ecol. 31, e13739. doi: 10.1111/rec.13739
Lovelock, C. E., Feller, I. C., Reef, R., Hickey, S., and Ball, M. C. (2017). Mangrove dieback during fluctuating sea levels. Sci. Rep. 7, 1–8. doi: 10.1038/s41598-017-01927-6
Lovelock, C. E., and Reef, R. (2020). Variable impacts of climate change on blue carbon. One Earth 3, 195–211. doi: 10.1016/j.oneear.2020.07.010
Lüdecke, D., Ben-Shachar, M. S., Patil, I., Waggoner, P., and Makowski, D. (2021). performance: an R package for assessment, comparison and testing of statistical models. J. Open Source Softw. 6. doi: 10.21105/joss.03139
Marbà, N., Duarte, C. M., Alexandra, A., and Cabaço, S. (2004). “How do seagrasses grow and spread,” in European Seagrasses: An Introduction to Monitoring and Management, 11. The M&MS project, Monitoring and Managing of European Seagrasses EVK3-CT-2000-00044.
Maxwell, P., Connolly, R., Roelfsema, C., Burfeind, D., Udy, J., O'Brien, K., et al. (2019). “Seagrasses of Moreton Bay Quandamooka: Diversity, ecology and resilience,” in Moreton Bay Quandamooka & Catchment: Past, Present, and Future, 279–298.
McKee, K. L., Rooth, J. E., and Feller, I. C. (2007). Mangrove recruitment after forest disturbance is facilitated by herbaceous species in the Caribbean. Ecol. Appl. 17, 1678–1693. doi: 10.1890/06-1614.1
McKenzie, L., Campbell, S., and Roder, C. (2003). “Seagrass-watch: manual for mapping & monitoring seagrass resources by community (citizen) volunteers,” in Information Series QI01094 (Cairns, QLD: QFS, NFC).
McMahon, K., van Dijk, K.-J., Ruiz-Montoya, L., Kendrick, G. A., Krauss, S. L., Waycott, M., et al. (2014). The movement ecology of seagrasses. Proc. R. Soc. B Biol. Sci. 281, 20140878. doi: 10.1098/rspb.2014.0878
Mugi, L. M., Kiss, D., Kairo, J. G., and Huxham, M. R. (2022). Stocks and productivity of dead wood in mangrove forests: a systematic literature review. Front. For. Global Change 5, 767337. doi: 10.3389/ffgc.2022.767337
Orth, R. J., Luckenbach, M., and Moore, K. A. (1994). Seed dispersal in a marine macrophyte: implications for colonization and restoration. Ecology 75, 1927–1939. doi: 10.2307/1941597
R Core Team (2021). R: A Language and Environment for Statistical Computing. Vienna, Austria: R Foundation for Statistical Computing.
Ricart, A. M., York, P. H., Bryant, C. V., Rasheed, M. A., Ierodiaconou, D., and Macreadie, P. I. (2020). High variability of Blue Carbon storage in seagrass meadows at the estuary scale. Sci. Rep. 10, 5865. doi: 10.1038/s41598-020-62639-y
Rivers, D. O., Kendrick, G. A., and Walker, D. I. (2011). Microsites play an important role for seedling survival in the seagrass Amphibolis antarctica. J. Exp. Mar. Biol. Ecol. 401, 29–35. doi: 10.1016/j.jembe.2011.03.005
Roelfsema, C. M., Phinn, S. R., Udy, N., and Maxwell, P. (2009). An integrated field and remote sensing approach for mapping Seagrass Cover, Moreton Bay, Australia. J. Spatial Sci. 54, 45–62. doi: 10.1080/14498596.2009.9635166
Rosentreter, J. A., Laruelle, G. G., Bange, H. W., Bianchi, T. S., Busecke, J. J., Cai, W.-J., et al. (2023). Coastal vegetation and estuaries are collectively a greenhouse gas sink. Nat. Clim. Chang. 13, 579–587. doi: 10.1038/s41558-023-01682-9
Ruiz-Montoya, L., Lowe, R., Van Niel, K., and Kendrick, G. (2012). The role of hydrodynamics on seed dispersal in seagrasses. Limnol. Oceanogr. 57, 1257–1265. doi: 10.4319/lo.2012.57.5.1257
Samper-Villarreal, J., Lovelock, C. E., Saunders, M. I., Roelfsema, C., and Mumby, P. J. (2016). Organic carbon in seagrass sediments is influenced by seagrass canopy complexity, turbidity, wave height, and water depth. Limnol. Oceanogr. 61, 938–952. doi: 10.1002/lno.10262
Saunders, M. I., Leon, J., Phinn, S. R., Callaghan, D. P., O' Brien, K. R., Roelfsema, C. M., et al. (2013). Coastal retreat and improved water quality mitigate losses of seagrass from sea level rise. Glob. Chang. Biol. 19, 2569–2583. doi: 10.1111/gcb.12218
Sippo, J. Z., Lovelock, C. E., Santos, I. R., Sanders, C. J., and Maher, D. T. (2018). Mangrove mortality in a changing climate: an overview. Estuar. Coast. Shelf Sci. 215, 241–249. doi: 10.1016/j.ecss.2018.10.011
Telesca, L., Belluscio, A., Criscoli, A., Ardizzone, G., Apostolaki, E. T., Fraschetti, S., et al. (2015). Seagrass meadows (Posidonia oceanica) distribution and trajectories of change. Sci. Rep. 5, 12505. doi: 10.1038/srep12505
Twomey, A. J., Saunders, M. I., Callaghan, D. P., Bouma, T. J., Han, Q., and O'Brien, K. R. (2021). Lateral sediment erosion with and without the non-dense root-mat forming seagrass Enhalus acoroides. Estuar. Coast. Shelf Sci. 253, 107316. doi: 10.1016/j.ecss.2021.107316
Uhrin, A. V., and Turner, M. G. (2018). Physical drivers of seagrass spatial configuration: the role of thresholds. Landsc. Ecol. 33, 2253–2272. doi: 10.1007/s10980-018-0739-4
Wentworth, C. K. (1922). A scale of grade and class terms for clastic sediments. J. Geol. 30, 377–392. doi: 10.1086/622910
Wright, M. N., and Ziegler, A. (2017). ranger: a fast implementation of random forests for high dimensional data in C++ and R. J. Stat. Softw. 77, 1–17. doi: 10.18637/jss.v077.i01
Keywords: seagrass, mangroves, ecosystem transitions, sea level rise, Moreton Bay, ghost forest
Citation: Twomey AJ, Staples TL, Remmerswaal A, Wuppukondur A and Lovelock CE (2023) Mangrove ghost forests provide opportunities for seagrass. Front. Clim. 5:1284829. doi: 10.3389/fclim.2023.1284829
Received: 29 August 2023; Accepted: 30 October 2023;
Published: 06 December 2023.
Edited by:
Hung Manh Nguyen, Ben-Gurion University of the Negev, IsraelReviewed by:
Brett Bayles, Dominican University of California, United StatesCopyright © 2023 Twomey, Staples, Remmerswaal, Wuppukondur and Lovelock. This is an open-access article distributed under the terms of the Creative Commons Attribution License (CC BY). The use, distribution or reproduction in other forums is permitted, provided the original author(s) and the copyright owner(s) are credited and that the original publication in this journal is cited, in accordance with accepted academic practice. No use, distribution or reproduction is permitted which does not comply with these terms.
*Correspondence: Alice J. Twomey, YS50d29tZXlAdXEuZWR1LmF1
Disclaimer: All claims expressed in this article are solely those of the authors and do not necessarily represent those of their affiliated organizations, or those of the publisher, the editors and the reviewers. Any product that may be evaluated in this article or claim that may be made by its manufacturer is not guaranteed or endorsed by the publisher.
Research integrity at Frontiers
Learn more about the work of our research integrity team to safeguard the quality of each article we publish.