- School of Politics and International Studies, University of Leeds, Leeds, United Kingdom
Mobilizing broad support for climate action is paramount for solving the climate crisis. Research suggests that people can be persuaded to support climate action when presented with certain moral arguments, but which moral arguments are most convincing across the population? With this pilot study, we aim to understand which types of moral arguments based on an extended Moral Foundation Theory are most effective at convincing people to support climate action. Additionally, we explore to what extent Generative Pre-trained Transformer 3 (GPT-3) models can be employed to generate bespoke moral statements. We find statements appealing to compassion, fairness and good ancestors are the most convincing to participants across the population, including to participants, who identify as politically right-leaning and who otherwise respond least to moral arguments. Negative statements appear to be more convincing than positive ones. Statements appealing to other moral foundations can be convincing, but only to specific social groups. GPT-3-generated statements are generally more convincing than human-generated statements, but the large language model struggles with creating novel arguments.
Introduction
Mitigating climate change requires collective effort on an unprecedented scale and climate communication research has long been working on finding strategies to mobilize the public for effective climate action (Moser, 2016). With this pilot study we seek to contribute to our understanding of how different moral arguments can act as a catalyst for encouraging members of the wider public to embrace climate policies. Moral Foundations Theory (MFT) is an empirically tested theory to understand the morals that guide behavior (Graham et al., 2013). MFT establishes five moral foundations, (1) care/harm is the compassion foundation that is linked to our desire to avoid harming others and our ability to care and show kindness; (2) fairness/cheating is linked to our sense of justice, rights and autonomy; (3) loyalty/betrayal underlies virtues or patriotism and self-sacrifice for the ingroup; (4) authority/subversion builds on principles of leadership and followership and includes respect for traditions; (5) sanctity/degradation, also called the purity foundation is based in feelings of disgust and fear of contamination from impurity, immorality.
Previous research has found that political affiliation predicts which moral foundations resonate with respondents the most, specifically people with right-wing political leaning respond to purity, authority and loyalty moral foundations, while people with left-wing political leaning respond strongly to compassion and fairness moral foundations, which do also appeal to right-wing leaning individuals although not as strongly as to left-leaning individuals (Graham et al., 2009; Dickinson et al., 2016). Strimling et al. (2019) showed moreover that it is this resonance of compassion and fairness moral arguments across the political ideological spectrum that drives the overall progressive opinion change in societies. MFT has also been applied to climate change, investigating how to best encourage behavior change in this respect. Dickinson et al. (2016) found for instance that compassion and fairness were strong, positive predictors of willingness to act on climate change, particularly among younger, more liberal individuals. Milfont et al. (2019) confirmed this finding in the context of electricity conservation behavior, but they also pointed out that conservatives with high scores on compassion and fairness moral foundations were more receptive to pro-environmental behavioral change, even though conservatives typically do no exhibit strong pro-environmental attitudes. Among liberals, high scores on compassion and fairness morals intensified pro-environmental behavior. Outside the US, similar findings have been made for Europe, Welsch (2020) for instance confirms that fairness and compassion foundations are robust predictors for climate-friendly behaviors and endorsement of climate-friendly regulations. However, there is also some research that suggests that appealing to a conservative moral foundation such as purity (Feinberg and Willer, 2013) or all five moral foundations (conservative messaging) rather than just compassion and fairness (liberal messaging) might be a more successful communication strategies, particularly when delivered by a conservative communicator as it appeals more to conservatives without alienating the liberals (Hurst and Stern, 2020).
There have been various suggestions for expanding the MFT, including other moral foundations such as liberty/oppression, which builds on the idea that people exhibit resentment toward those, who dominate them and restrict their liberty (Iyer et al., 2012). We suggest here to expand the MFT in a yet another way by including good ancestors moral foundation that refers to a sense of moral responsibility to preserve or even enhance humanity's living conditions for future generations and to leave a positive legacy. While this foundation has some similarities to the existing foundations of compassion and loyalty, the key distinguishing characteristic is that the good ancestors foundation encompasses concern about future generations and earth stewardship, with the goal to protect the planet for humans and animal/plant life alike. The new foundation is based in research, which has shown that perceived responsibility toward future generations is a robust predictor for pro-environmental attitudes (Syropoulos and Markowitz, 2021). And Zaval et al. (2015) explicitly showed that individual's motivation to leave a positive legacy can be leveraged for positive climate action.
Research so far shows the role that moral foundations in general play in embracing climate action, but with the notable exception of Feinberg and Willer (2013) and Hurst and Stern (2020), no attempt has been made so far to directly and systematically use moral foundations in designing climate action arguments and test whether these arguments can convince the public and various socio-political groups to act on climate change. Moreover, Hurst and Stern (2020) tested only conservative (all five moral foundations combined) vs. liberal frames (compassion and fairness moral foundations combined) messaging, rather than messages based in each single moral foundation. While, Feinberg and Willer (2013) tested only the purity framing and found that it increased right-leaning individuals' pro-environmental attitudes. Our pilot study is the first attempt to address this gap. For that purpose, we pilot a range of statements conveying a specific moral foundation to identify, which are most convincing to encourage people to support climate change policies.
The second goal of our pilot is to understand whether an AI-based language model, GPT-3, can be used effectively to generate such moral arguments. The rationale for this is to envision climate change communication that is scalable, bespoke, and automated, for instance embedded within a carbon footprint tracking app, such as Cogo or Yayzy. This is based on increasing body of research that explores conversational AI and its effects on humans, including changing attitudes toward supporting the Black Lives Matter movement and climate change efforts (Chen et al., 2022).
Data and methods
To establish, which moral arguments for climate action are perceived as most convincing, and how this may differ between different socio-demographic and socio-political groups as well as to establish how convincing AI-generated moral statements are, we conducted an online survey with a sample (N = 371) of UK-based respondents recruited and compensated through Prolific. The sample was split randomly in two subsamples, to investigate whether negatively (N = 185) or positively (N = 186) framed arguments are perceived as more convincing, as there is a tendency in climate change communication to emphasize the need for positive climate change communication; a tendency that received some criticism (Moser, 2016).
We first devised a list of example statements, each conveying one of six moral categories (compassion, fairness, loyalty, purity, authority, good ancestor). We used these example statements as well as keywords describing the moral foundations as prompts to the text-davinci-003 text completion model of the pre-trained transformer GPT-3 (Brown et al., 2020), provided by OpenAI (https://platform.openai.com/playground). GPT-3 uses deep-learning to produce text which follows a given text prompt. We set the temperature parameter of this model to 0.7 to give the model some flexibility of how it returns a resulting statement. Setting the temperature parameter closer to 0 results in the model only choosing the word with the highest probability to follow the preceding word. Temperature values closer to 1 allows the model to select different probabilities for consecutive words, allowing for more varied results. We chose from a range of GPT-3-generated negative and positive statements to be included in the survey, typically including per moral foundation and framing (negative vs. positive) one human-generated statement and two GPT-3-generated statements for each moral category. We included more GPT-3-generated statements comparing to statement we generated, because one goal of this paper was to evaluate the effectiveness of AI in generating these statements. We did not aim for a systematic comparison between AI- and human-generated messages but would encourage such research in the future. Furthermore, we aimed at including mirroring positive and negative statements to allow for comparability (see complete survey with all statements in Supplementary material). For each statement respondents were asked to indicate on a scale from one to six how convinced they were by the statement and additionally on a binary scale (yes/no) whether the statement was perceived as applicable and novel (not encountered before) with respect to climate change policies.
The survey contained moreover a range of socio-demographic (age, gender, whether they had children, household income, educational background, ethnicity, religion) and socio-political (left-right political self-identification on a 10-point scale and Schwartz values) variables. We also included the 20-item Schwartz (2003) values scale (Sandy et al., 2017), because research suggests that values play an important role in predicting support for climate action (Nilsson et al., 2004; Dietz et al., 2007; Howell, 2013; Hornsey, 2021).
In our sample 60% were women, 75% were white British, 60% had an academic degree (BA/equivalent or higher, see Supplementary Figure S1), the age in the sample ranged from 18 to 81, with an average of 38 (sd = 12), the median annual household income was between £40,000 and £59,999 (see Supplementary Figure S2 for a distribution), 54% had children, 73% had no religious affiliation, 52% identified as politically left-leaning (see Supplementary Figure S3), and 62% held values indicating an openness to change, i.e., valuing self-direction and stimulation over conservative values (conformism, tradition and security). The vast majority (94%) embraced self-transcendent (universalism, benevolence) (see Supplementary Figure S4) over self-enhancement (achievement, power) values.
To analyze the results of the survey, we used a mixture of descriptive (capturing central tendencies), bivariate, and multivariate analyses (see R Markdown file link in Supplementary material). Bivariate analyses included correlations, two-sample t-test, paired t-tests and Wilcoxon tests to identify significant linear associations and group differences with respect to convincingness ratings and various socio-demographic and values indicators. The paired Wilcoxon tests were used where single convincingness scores for single statements had to be used instead of indices values, as the test does not require multivariate normality in the data. Correlations were also calculated between convincingness, novelty, and applicability for positive and negative statements. We used Confirmatory Factor Analysis (CFA) to test whether the moral statements used in the survey can be mapped onto underlying latent constructs representing the six extended MFT categories described above. We estimated the CFAs separately for negative and positive statements as the participants were randomly split into these two groups, resulting in effectively two samples. The goodness-of-fit of the CFA models was evaluated using four global fit indices: Comparative Fit Index (CFI), Tucker Lewis Index (TLI), Root Mean Square Error of Approximation (RMSEA) and Standard Root Mean Square Residual (SRMR). We also investigated whether the CFA models can be improved when removing certain statements form the model.
Results
Here we will report the most central results of our analysis, detailed results can be found in the Supplementary material. A summary of the most convincing statements can be found in Supplementary Table S1. It shows the most convincing moral arguments overall are rooted in good ancestors, compassion, and fairness moral foundations. Most respondents found also negatively framed statements to be more convincing than positively framed ones, with the exception for purity and good ancestors categories. While negative good ancestors were indeed generally very convincing to participants, this was less so for the statement that conveyed guilt (see GAN1 in Supplementary Table S1). An overview of all statements with their values for convincingness, applicability and novelty can be found in the Supplementary Table S1. Generally speaking, for both negative and positive statements, convincingness ratings are positively correlated with applicability (mean r = 0.47, but the range in correlations is larger for negative statements than positive statements), and negatively correlated with novelty (mean r = −0.27 and mean r = −0.25, respectively, but the range is larger for positive statements than for negative statements). Applicability of statements and novelty are mildly negatively correlated for negative and positive statements (mean r = −0.22 and mean r = −0.23, respectively, but as with convincingness and applicability, the range is larger with negative statements than positive). As Table 1 and Supplementary Table S1 show, respondents found GPT-3 generated statements often more convincing than statements we generated ourselves. All highest-ranked statements in terms of convincingness were GPT3 generated. While of course these were handpicked AI-generated statements, this shows nevertheless that AI-generated moral arguments can indeed be very convincing to people.
The CFAs conducted suggest that negative moral statements used in the survey can be successfully mapped onto the six extended MFT categories, and the model can be further improved when removing two statements (see Section 3 in Supplementary material). This means we can create indices from the single statements that represent the six extended MFT categories. Unfortunately, we were not able to do the same with the positive moral statements (see Section 3 in Supplementary material). It seems we were less able to generate consistent positive statements for each moral category and it should be noted that GPT-3 struggled with producing positive statements particularly for some moral categories such as fairness and authority. This also meant we were not able to create indices from the single statements which consistently represented the six extended MFT categories. In our bivariate analyses we hence use the most convincing statement from each category as a proxy measure for the respective positively framed moral category.
Overall, negatively framed statements conveying morals of compassion and fairness were the most convincing moral foundations for climate action, while statements conveying loyalty and authority were the least convincing (see Supplementary Figure S5; Supplementary Table S3). These findings are statistically confirmed using paired t-tests on the negative indices. For negative moral foundations indices, statistically significant (p-value < 0.05) differences in means were found, except between fairness (mean convincingness of 5.1) and compassion (mean convincingness of 5.1), the most convincing moral foundations and between loyalty (mean convincingness of 4.5) and authority (mean convincingness of 4.5), the least convincing moral foundations. Negatively framed purity-based arguments (mean convincingness of 4.9) are more convincing to participants comparing to statements based in authority, loyalty or good ancestors (mean convincingness of 4.7) moral foundation, but still less convincing comparing to statements based on compassion and fairness moral foundations.
When it comes to positively framed statements, now looking only at the most convincing statements not the indices, then we see those arguments building on good ancestors, but also purity, compassion, and fairness were the most convincing moral foundations for climate action, while again statements conveying loyalty and authority were the least convincing (see Supplementary Figure S6; Supplementary Table S4). This finding is also statistically supported using the Wilcoxon t-test on the highest ranked positive statements. Statistically significant (p-value < 0.05) differences in means were found, apart from between compassion (mean convincingness of 4.8) and fairness (mean convincingness of 4.7), compassion and purity, and between loyalty and authority. Statements based in authority (mean convincingness of 4.5) and loayalty (mean convincingness of 4.4) were again significantly the least convincing statements. The two most convincing statements were based in good ancestors (mean convincingness of 5.4) and purity (mean convincingness of 5.1). It should be noted that the highly ranked, positively framed statement representing the good ancestors moral foundation, can be interpreted more broadly as a moral foundation about our relationship with our planet, harboring ideas of earth stewardship (Chapin et al., 2022) and earth altruism (Österblom and Paasche, 2021). Similar can be said about the highly ranked, positively framed purity statement, and the two are significantly correlated (r = 0.31, p < 0.001).
Political self-identification is one of the main covariates for how individuals responded to the moral arguments for climate action (see Section 5 in Supplementary material). We asked participants to place themselves on a scale from 0 to 10 on how politically left or right leaning they were. We then split the respondents into two groups: those scoring <5 were in the “left-leaning” group and those scoring 5 or higher in the “right-leaning” group. On average, those in the left-leaning group found moral arguments more convincing than the right leaning group (see Figures 1, 2). Nevertheless, compassion (whether positively or negatively framed), fairness (negatively framed), and good ancestors (positively framed) were still the most convincing moral foundations overall, regardless of political leaning (see Supplementary Tables S6, S7).
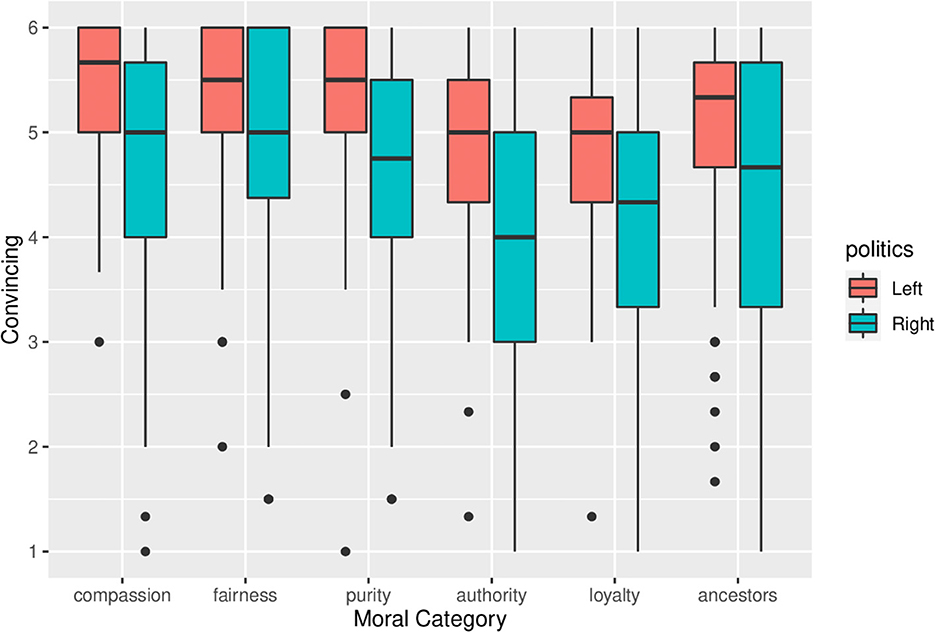
Figure 1. Boxplot of how convincing respondents found each moral category (indices based on negatively framed statements, grouped by political. Y-axis ranges from 1 = least convincing to 6 = most convincing. The midline in the boxplot represents the median, the ends represent the 1st and 3rd quartile respectively, and the dots represents outliers that fall outside of the interquartile range. See Section 5 Supplementary material for t-tests.
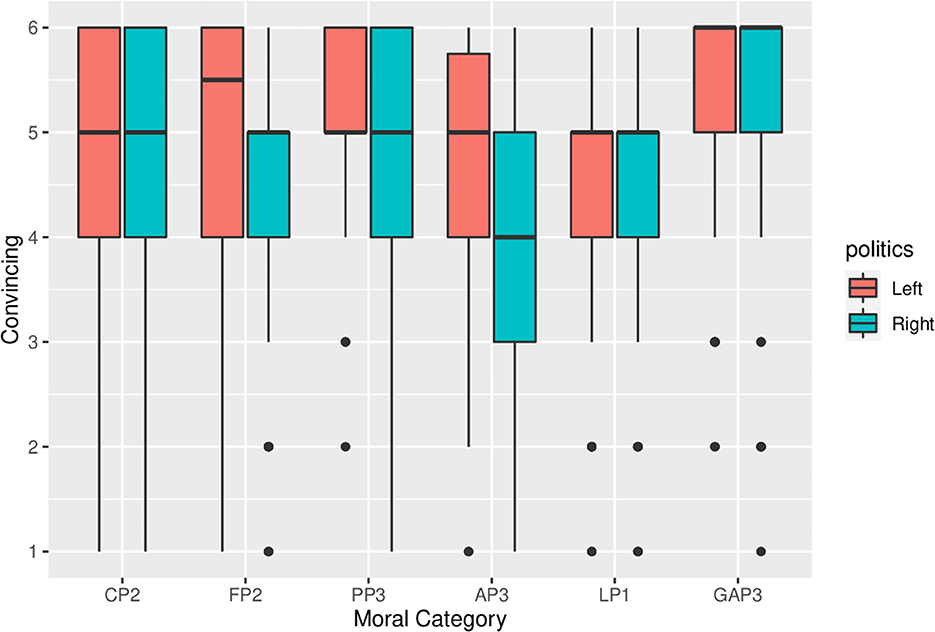
Figure 2. Boxplot of how convincing respondents found each positively framed moral arguments from each moral foundation category, grouped by political. Y-axis ranges from 1 = least convincing to 6 = most convincing. The midline in the boxplot represents the median, the ends represent the 1st and 3rd quartile respectively, and the dots represents outliers that fall outside of the interquartile range. See Section 5 Supplementary material for t-tests.
Socio-demographic variables did not strongly correlate with convincingness of moral arguments for climate action (see Section 5, Supplementary material). However, we found that respondents with a religious affiliation were more convinced by purity statements, and in particular by those referring to religious sentiments, than non-religious respondents (see Supplementary Table S5). Finally, values were also important covariates for how individuals responded to moral arguments for climate action. Specifically, participants scoring higher on the universalism (self-transcendence dimension) values, were more likely to find moral arguments for climate action convincing, particularly those conveying compassion (r = 0.33) (see Section 5, Supplementary material).
Discussion and conclusion
The first aim of this study was to identify which moral arguments for climate action are most convincing to people and whether various socio-political groups differ in which arguments they find most convincing. We found that political leaning of participants was the biggest indicator of how convincing respondents found statements in different moral categories. But we also found that overall statements conveying morals of compassion for others (“Climate change is already costing lives, with more people dying from floods, heatwaves and other extreme weather events”), leaving a good ancestral legacy (positive statement) (“The Earth is our only home, and we have a responsibility to protect it”), and fairness (“Climate change means that future generations, who have done nothing to contribute to climate change, will be most affected by its effects.”) were by far the most convincing arguments to survey respondents, regardless of any socio-demographic or political attributes.
The fairness statement, with its emphasis on future generations also has a good ancestors dimension. These results align with previous research findings, showing that those responsive to compassion moral foundations are more likely to embrace climate or pro-environmental action, irrespective of political affiliation (Dickinson et al., 2016; Milfont et al., 2019; Welsch, 2020). It also aligns with research that suggested that people can be motivated to take action on climate change by the desire to leave a positive legacy (Zaval et al., 2015; Syropoulos and Markowitz, 2021) and our study confirms that this is true irrespective of political affiliation. Our results also confirm earlier findings, that people, who hold self-transcendent values are more concerned about climate change (Nilsson et al., 2004; Dietz et al., 2007; Howell, 2013; Hornsey, 2021), and consequently are more responsive to moral arguments for climate action.
While we found that moral arguments appealing to religious feelings (purity) were perceived as the least convincing, this is because the vast majority in our sample was non-religious. However, these arguments do appeal to those who are religious. Hence, in more religious societies, these purity moral arguments may play an important role to mobilize climate action. There appears to be also an interesting positive connection between good ancestors morals and purity morals, maybe as the desire for leaving a positive legacy (a livable planet for future generations) acts as a religious substitute for some and complements religious feelings for others. No other socio-demographic indicators in our study yielded significant associations with convincingness ratings of the different moral categories. This result aligns with findings reported by Vartanova et al. (2021), who found that socio-demographic indicators did not make a significant difference to how applicable respondents found different moral arguments, however they did not test for political leaning or religious affiliation.
The second aim of this pilot project was to evaluate how useful GPT-3 is in generating moral arguments for climate action. When asked for general arguments for climate action (with no moral prompting), GPT-3 provides various reasons, ranging from avoiding heat waves, droughts, reducing GHGs, to pointing out that climate change poses a threat to public health and has negative economic impacts. These unprompted statements did not tend to explicitly convey a particular morality, however they did implicitly convey morals of harm and authority (e.g., “Debilitating environmental effects and a weakened global economy could result in mounting national debt, increased unemployment, and a decline in public health, undermining democratic processes and leaving governments unaccountable”). We also found the model excelled at producing statements about future generations even without explicit prompting and at generating negative statements across all moral categories. GPT-3 struggled with positive statements especially in the fairness and authority categories. These observations in themselves are noteworthy as they reveal the biases in existing human-generated climate communication, which presents in the data that the model was trained on. However, a more systematic analysis is required to better understand these biases.
Notably, all of the most convincing and most applicable moral arguments for climate change policies were generated by GPT-3 rather than human authors. On the other hand, the most novel moral arguments were human-generated, as measured through the binary yes/no survey question for each statement (see Supplementary Table S1). This suggests GPT-3 is successful at creating moral arguments, similar to prompts and existing data used for training and expressing them in a very convincing way, however, it struggles to come up with novel arguments. This large language model could therefore be used most effectively once research has established which moral arguments and framing are most convincing.
GPT-3 also struggled at times with generating statements that were sufficiently distinct for each of the moral foundation categories. There were occasionally overlaps across different foundations in the statements generated by GPT-3, even when being prompted with clear human-generated examples of the foundations. We have already noted above for instance the overlap between the GPT-3 generated good ancestor and purity statements. This reveals a potential pitfall to using AI-generated text based in moral foundations as the statements may not yet be differentiated enough, however with improving large language models this may well change.
This was merely a pilot study, exploring the effectiveness of moral arguments for climate actions and exploring whether AI can be used to generate such moral arguments. But our results are limited in many ways, first we have not conducted a systematic analysis of GPT-3 generated statements, exploring systematically the biases inherent in these large language models. The Prolific sample albeit sufficiently varied, is not representative and was drawn solely from the UK population. Furthermore, whether the moral arguments that respondents find convincing can indeed inspire actual climate action in terms of specific behavior or policy support, cannot be answered with this study. Finally, we were not able to conduct a dimension reduction into the six moral foundation categories on the positively framed statements. Nevertheless, our study suggests that Moral Foundations Theory can be used successfully to convince people to support climate change policies when framed negatively and that AI can be a useful tool for that purpose, albeit more systematic research is needed to expand on these initial findings. Finally, it is interesting to note that moral arguments advanced by climate protest movements such as Fridays for Future, appealing to justice, duty of care and responsibility for future generations (Spaiser et al., 2022) seem to resonate to a greater extent with people than arguments focusing on economic gains etc. as included for instance in the authority and loyalty based moral statements. But there are important subtle differences in the framing within even generally appealing moral foundations. Negative framing highlighting the harm from failed climate action for instance can be effective, but not if it invokes guilt (e.g., “future generations will not forgive us”).
Data availability statement
The original contributions presented in the study are included in the article/Supplementary material, further inquiries can be directed to the corresponding author.
Ethics statement
The studies involving human participants were reviewed and approved by Business, Earth and Environment, Social Sciences (AREA FREC) Committee, University of Leeds. The patients/participants provided their written informed consent to participate in this study.
Author contributions
NN and VS contributed to conception and design of the study. NN performed the preliminary statistical analysis and wrote the first draft of the manuscript. All authors contributed to manuscript and analysis revision, read, and approved the submitted version.
Funding
This research was funded by a UKRI Future Leaders Fellowship (Grant Number: MR/V021141/1), and an Alan Turing Institute Postdoctoral Enrichment Award (Reference 2022PEA).
Conflict of interest
The authors declare that the research was conducted in the absence of any commercial or financial relationships that could be construed as a potential conflict of interest.
Publisher's note
All claims expressed in this article are solely those of the authors and do not necessarily represent those of their affiliated organizations, or those of the publisher, the editors and the reviewers. Any product that may be evaluated in this article, or claim that may be made by its manufacturer, is not guaranteed or endorsed by the publisher.
Supplementary material
The Supplementary Material for this article can be found online at: https://www.frontiersin.org/articles/10.3389/fclim.2023.1193350/full#supplementary-material
References
Brown, T., Mann, B., Ryder, N., Subbiah, M., Kaplan, J. D., Dhariwal, P., et al. (2020). Language models are few-shot learners. Adv. Neural Inform. Proc. Syst. 33, 1877–1901. doi: 10.48550/arXiv.2005.14165
Chapin, F. S. III, Weber, E. U., Bennett, E. M., Biggs, R., Van Den Bergh, J., Adger, W. N., et al. (2022). Earth stewardship: shaping a sustainable future through interacting policy and norm shifts. Ambio 51, 1907–1920. doi: 10.1007/s13280-022-01721-3
Chen, K., Shao, A., Burapacheep, J., and Li, Y. (2022). A critical appraisal of equity in conversational AI: evidence from auditing GPT-3′s dialogues with different publics on climate change and Black Lives Matter. arXiv [Preprint].
Dickinson, J. L., McLeod, P., Bloomfield, R., and Allred, S. (2016). Which moral foundations predict willingness to make lifestyle changes to avert climate change in the USA? PLoS ONE 11, e0163852. doi: 10.1371/journal.pone.0163852
Dietz, T., Dan, A., and Shwom, R. (2007). Support for climate change policy: social psychological and social structural influences. Rural Sociol. 72, 185–214. doi: 10.1526/003601107781170026
Feinberg, M., and Willer, R. (2013). The moral roots of environmental attitudes. Psychol. Sci. 24, 56–62. doi: 10.1177/0956797612449177
Graham, J., Haidt, J., Koleva, S., Motyl, M., Iyer, R., Wojcik, S. P., et al. (2013). Moral foundations theory: the pragmatic validity of moral pluralism. Adv. Exp. Soc. Psychol. 47, 55–130. doi: 10.1016/B978-0-12-407236-7.00002-4
Graham, J., Haidt, J., and Nosek, B. A. (2009). Liberals and conservatives rely on different sets of moral foundations. J. Pers. Soc. Psychol. 96, 1029. doi: 10.1037/a0015141
Hornsey, M. J. (2021). The role of worldviews in shaping how people appraise climate change. Curr. Opin. Behav. Sci. 42, 36–41. doi: 10.1016/j.cobeha.2021.02.021
Howell, R. A. (2013). It's not (just)“the environment, stupid!” values, motivations, and routes to engagement of people adopting lower-carbon lifestyles. Global Environ. Change 23, 281–290. doi: 10.1016/j.gloenvcha.2012.10.015
Hurst, K., and Stern, M. J. (2020). Messaging for environmental action: the role of moral framing and message source. J. Environ. Psychol. 68, 101394. doi: 10.1016/j.jenvp.2020.101394
Iyer, R., Koleva, S., Graham, J., Ditto, P., and Haidt, J. (2012). Understanding libertarian morality: the psychological dispositions of self-identified libertarians. PLoS ONE 7, e42366. doi: 10.1371/journal.pone.0042366
Milfont, T. L., Davies, C. L., and Wilson, M. S. (2019). The moral foundations of environmentalism. Soc. Psychol. Bull. 14, 1–25. doi: 10.32872/spb.v14i2.32633
Moser, S. C. (2016). Reflections on climate change communication research and practice in the second decade of the 21st century: what more is there to say? WIRE's Climate Change 7, 345–369. doi: 10.1002/wcc.403
Nilsson, A., von Borgstede, C., and Biel, A. (2004). Willingness to accept climate change strategies: the effect of values and norms. J. Environ. Psychol. 24, 267–277. doi: 10.1016/j.jenvp.2004.06.002
Österblom, H., and Paasche, Ø. (2021). Earth altruism. One Earth 4, 1386–1397. doi: 10.1016/j.oneear.2021.09.003
Sandy, C. J., Gosling, S. D., Schwartz, S. H., and Koelkebeck, T. (2017). The development and validation of brief and ultrabrief measures of values. J. Pers. Assess. 99, 545–555. doi: 10.1080/00223891.2016.1231115
Schwartz, S. H. (2003). A proposal for measuring value orientations across nations. Question. Dev. Package Eur. Soc. Surv. 259, 261.
Spaiser, V., Nisbett, N., and Stefan, C. G. (2022). “How dare you?”—The normative challenge posed by Fridays for Future. PLoS Climate 1, e0000053. doi: 10.1371/journal.pclm.0000053
Strimling, P., Vartanova, I., Jansson, F., and Eriksson, E. (2019). The connection between moral positions and moral arguments drives opinion change. Nat. Hum. Behav. 3, 922–930. doi: 10.1038/s41562-019-0647-x
Syropoulos, S., and Markowitz, E. M. (2021). Perceived responsibility towards future generations and environmental concern: convergent evidence across multiple outcomes in a large, nationally representative sample. J. Environ. Psychol. 76, 101651. doi: 10.1016/j.jenvp.2021.101651
Vartanova, I., Eriksson, K., Hazin, I., and Strimling, P. (2021). Different populations agree on which moral arguments underlie which opinions. Front. Psychol. 12, 648405. doi: 10.3389/fpsyg.2021.648405
Welsch, H. (2020). Moral foundations and voluntary public good provision: the case of climate change. Ecol. Econ. 175, 106696. doi: 10.1016/j.ecolecon.2020.106696
Keywords: Moral Foundations Theory, climate change, climate action, climate communication, AI, GPT-3
Citation: Nisbett N and Spaiser V (2023) How convincing are AI-generated moral arguments for climate action? Front. Clim. 5:1193350. doi: 10.3389/fclim.2023.1193350
Received: 08 May 2023; Accepted: 21 June 2023;
Published: 06 July 2023.
Edited by:
Jose Antonio Rodriguez Martin, Instituto Nacional de Investigación y Tecnología Agroalimentaria (INIA), SpainReviewed by:
Charmaine Mullins Jaime, Indiana State University, United StatesKatherine Lacasse, Rhode Island College, United States
Copyright © 2023 Nisbett and Spaiser. This is an open-access article distributed under the terms of the Creative Commons Attribution License (CC BY). The use, distribution or reproduction in other forums is permitted, provided the original author(s) and the copyright owner(s) are credited and that the original publication in this journal is cited, in accordance with accepted academic practice. No use, distribution or reproduction is permitted which does not comply with these terms.
*Correspondence: Nicole Nisbett, bi5uaXNiZXR0QGxlZWRzLmFjLnVr