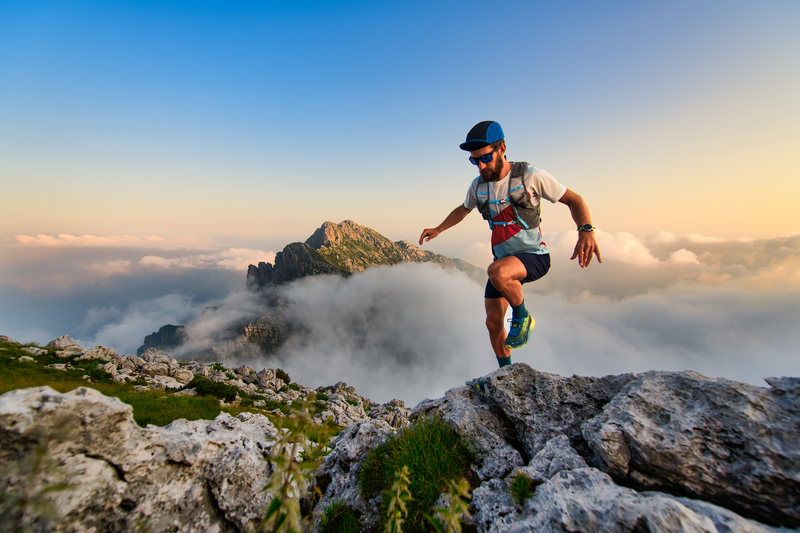
95% of researchers rate our articles as excellent or good
Learn more about the work of our research integrity team to safeguard the quality of each article we publish.
Find out more
REVIEW article
Front. Clim. , 04 January 2024
Sec. Climate Mobility
Volume 5 - 2023 | https://doi.org/10.3389/fclim.2023.1189125
This article is part of the Research Topic Climate Mobility Modeling: Methodological Advances and Future Prospects View all 6 articles
Climate change will have significant impacts on all aspects of human society, including population movements. In some cases, populations will be displaced by natural disasters and sudden-onset climate events, such as tropical storms. In other cases, climate change will gradually influence the economic, social, and political realities of a place, which will in turn influence how and where people migrate. Planning for the wide spectrum of future climate-related mobility is a key challenge facing development planners and policy makers. This article reviews the state of climate-related migration forecasting models, based on an analysis of thirty recent models. We present the key characteristics, strengths, and weaknesses of different modeling approaches, including gravity, radiation, agent-based, systems dynamics and statistical extrapolation models, and consider five illustrative models in depth. We show why, at this stage of development, forecasting models are not yet able to provide reliable numerical estimates of future climate-related migration. Rather, models are best used as tools to consider a range of possible futures, to explore systems dynamics, to test theories or potential policy effects. We consider the policy and research implications of our findings, including the need for improved migration data collection, enhanced interdisciplinary collaboration, and scenarios-based planning.
For more than 30 years, research published by scientists and reports in the news media have warned that climate change will cause mass migration and displacement on a global scale. The steady drumbeat of coverage and research, often based on simplistic assumptions about why and how people migrate, has fueled policies that focus on the security concerns associated with mass migration (McLeman, 2014; Boas et al., 2019). A predominant early assumption was that climate change and migration have a linear, cause-and-effect relationship, in which climate induced drought, rising sea levels, and natural disasters result in the movement of affected populations. Approaches to forecasting migration initially focused on hazard mapping, identifying areas threatened by climate change and assuming the vast majority of residents of affected areas would be forced to leave. This generated catastrophic projections of climate migration and “environmental refugees” (see Brown, 2008), leading one review article from 2011 to conclude that “estimates and projections regarding environmental displacement […] appear to have been put forward in order to generate media attention rather than to provide empirically grounded estimates and predictions” (Gemenne, 2011, S48).
Early projections failed to capture the complex drivers of migration and immobility, undermining their accuracy and relevance to real-world dynamics. They did not adequately account for how climate-related factors interact with non-climate related drivers of migration (see De Haas et al., 2020a), the potential for in-situ adaptation, place attachment (see Farbotko et al., 2020), and instances in which climate change impacts may suppress mobility, particularly among economically disadvantaged populations (see Zickgraf, 2021). These shortcomings spurred more nuanced investigations into how climate change impacts intersect with existing mobility systems and development conditions to affect the nature, volume, direction, and composition of migration flows. Attempts to forecast climate-related migration have grown in number and sophistication in recent years, particularly since the early 2010s.
This article reviews the current state of climate-related migration forecasting models. We consider some of the most prevailing and promising approaches for predicting the volume, composition, and direction of future climate-related migration. Our findings are based on a systematic literature review of thirty forecasting models. Importantly, we do not review models of historical climate-migration interactions, instead focusing on models that address potential future climate-related migration.
Our analysis finds that the field of climate-related migration forecasting remains in its infancy, and numerical estimates of future climate-related migration are best taken as speculative. Although significant advancements in modeling have been made, we find several key limitations, both empirical and conceptual, that constrain the real-world applicability of their projections. These limitations include the lack of reliable data on migration flows in many countries most vulnerable to climate change; a theoretical and empirical overemphasis of climate-related drivers of migration that neglects other political, economic, demographic, cultural, and technological determinants of migration and immobility; and a related dearth of reliable data on these non-climate related variables. Recognizing these limitations, we suggest that at this stage, climate-related migration forecasting models are best used as tools to explore potential migration scenarios under various climate, development, and policy futures, and to explore how climate impacts may affect broader social systems within which migration is embedded.
The article proceeds as follows. Section 1 considers ways of conceptualizing climate-related migration, and the challenges entailed in defining and quantifying “climate migration.” Section 2 presents the most common types of forecasting models. Section 3 describes our review process, and Section 4 describes the state of the field based on our review of thirty models. Section 5 presents five forecasting models in-depth, to illustrate the strengths and weaknesses of prominent modeling approaches. Sections 6 and 7 discuss key challenges to forecasting and directions for future research.
The International Organization for Migration, a United Nations agency, defines climate migration as “the movement of a person or groups of persons who, predominantly for reasons of sudden or progressive change in the environment due to climate change, are obliged to leave their habitual place of residence, or choose to do so, either temporarily or permanently, within a State or across an international border” (International Organization for Migration, 2019). This definition is broad. It encompasses many different kinds of climate-related migration, spanning the spectrum of forced to voluntary, internal and international, temporary and permanent. This breadth poses a challenge to research, forecasting, and policy-making related to migration and climate change, because the kinds of migration being studied can vary considerably and require significantly different policy responses.
Brown and McLeman (2013) suggest climate-related migration may be categorized according to the nature of the climatic stimulus (sudden-onset climate events vs. gradual changes in prevailing conditions) and the nature of the migration response (distress migration vs. adaptive or amenity-seeking migration). Sudden-onset climatic events, such as floods and storms, are associated with distress migration or displacement, in which large numbers of households abandon their place of residence—often with relatively short notice. Alternatively, households can become trapped in place by sudden-onset events (e.g., floods that shut down roads). Climate-induced displacement often takes place over relatively short distances, most often within countries, and return migration tends to be common (McLeman, 2018; De Haas et al., 2020b). The link between a sudden-onset climate event and distress migration is more direct, yet where, how, and whether people move in response to that event is shaped by pre-existing migration systems, the resources and networks of affected households, government or humanitarian interventions, and the broader development context. This helps explain why in the United States, for example, Hurricane Katrina led to long-distance inter-state displacement for those with stronger networks and resources, in-state displacements for others, while the populations without access to transportation became trapped in New Orleans and disproportionately perished (Fussell et al., 2014).
Slow-onset changes include increasing temperature, irregular rainfall patterns, sea-level rise, ocean acidification, soil salinization, loss of biodiversity, and desertification (De Sherbinin, 2020). These slow-onset changes interact with migration outcomes indirectly and often in a non-linear fashion (McLeman, 2018). Other political, economic, cultural, or conflict-related drivers of migration or immobility may be stressed by climate change, and these social phenomena mediate climate impacts and the responses of both individuals and households. For this reason, slow-onset climate changes contribute to migration but are often not the proximate cause of migration. Moreover, the same climate stress can lead to countervailing migration outcomes. For example, in rural Ethiopia, men's labor migration has been found to increase during periods of drought, while women's marriage migration decreases (Gray and Mueller, 2012).
Motivations for migration in climate-stressed contexts are rarely for climate or environmental reasons alone. People continue to move for better education, economic opportunities, family, or security-related reasons. The Amakrane et al. (2023) finds that in the face of slow-onset events, livelihood opportunities elsewhere and loss of income due to changes in personal circumstances were more salient drivers than climate-related impacts. Some describe slow-onset climate impacts as threat multipliers—for example, drought can reduce crop yields and thus household incomes, or it can exacerbate conflict in water-scarce regions (Goodman, 2007; McLeman, 2014; Sofuoglu and Ay, 2020). In the context of slow-onset climate change, the idea of “thresholds” or “tipping points” becomes important. Many affected households will attempt to adapt in place despite significant livelihood stress, until in-situ adaptation fails and/or a tipping point is reached, after which a significantly higher share of households choose migration as their primary adaptation strategy (see Gray and Mueller, 2012; McLeman, 2018).
Figure 1 presents a conceptual framework illustrating the macro-, meso- and micro-level factors1 that mediate the relationship between environmental change (including climate change) and migration and immobility outcomes. It adapts the conceptual framework developed for the influential Foresight: Migration and Global Environmental Change study conducted by the government of the United Kingdom (Black et al., 2011). Additionally, it integrates the aspiration/ability model (Carling, 2002; Carling and Schewel, 2018) to highlight different immobility outcomes alongside migration. In this model, a migration “aspiration” is defined simply as a conviction that migration is preferable to non-migration; it can vary in degree and in the balance between choice and coercion. When people develop a wish to leave, the outcome depends on their capacity to convert this wish into reality, given context-specific obstacles and opportunities, captured by the term “ability” (Carling and Schewel, 2018, p. 955).2 This framework can help explain why fewer people tend to migrate than migration theories would predict (Schewel, 2020)—a reality that also appears to be the case in many environmentally stressed regions (see Farbotko and McMichael, 2019; Wiegel et al., 2021). Some may still feel a clear commitment and desire to stay in place and may be called “voluntarily immobile.” Others may never meaningfully consider migrating, some of whom may be called “acquiescently immobile” (see Schewel, 2020). Still others may desire to leave but lack the ability to do so, becoming “trapped” or “involuntarily immobile” (Carling, 2002; Black et al., 2011; Zickgraf, 2021).
Figure 1. Contextual factors that mediate the effects of environmental change on migration and immobility outcomes. Figure inspired by Black et al. (2011, p. 33), the aspiration/ability model (Carling and Schewel, 2018), and the social transformation framework (De Haas et al., 2020b).
Because climate change often acts indirectly on pre-existing migration systems, it is difficult to disentangle the relative impact of climate-related variables from other migration drivers. The question of causality is especially challenging for forecasting models (see Cottier et al., 2022). To date, forecasting has tended to operate under the assumption that populations are relatively fixed unless uprooted by a climate event. In reality, populations are constantly moving both internally and internationally. They move in seasonal, temporary, or permanent ways for work, education, security, family, or even adventure across stages of life (Bredeloup, 2013; Olwig, 2018; Cundill et al., 2021; Van Praag, 2021). Population movements within and from a country even have a patterned relationship with levels of socioeconomic development. For example, more people move to towns and cities as economies industrialize, and in many countries, a growing percentage of the population migrates internationally as countries move from low- to middle-income status–a phenomenon referred to as a country's “mobility transition” (Zelinsky, 1971; De Haas, 2010; Clemens, 2020; Schewel and Asmamaw, 2021). This suggests that modeling should not focus exclusively on estimating future “climate migrants,” pushed out of their homes by environmental stress, but rather on clarifying how climate change will reshape or constrain existing mobility systems.
Current approaches to forecasting climate-related migration have their roots in a history of forecasting population growth and distribution. Population forecasting is meant to predict future population distributions across rural and urban places based on factors like fertility, mortality, and migration. Population forecasting has been utilized by statistical agencies and development planners for decades (Shryock et al., 1975). Over time, population forecasting tools advanced, allowing researchers to incorporate a greater array of assumptions and to project more specific forecasts (e.g., by age) (Wiśniowski et al., 2015).
Migration modelers build on the sub-component of migration within population forecasting through a variety of methods. Often modelers utilize past migration data to predict future migration trends, incorporating additional variables, such as economic or demographic factors, into their models (Disney et al., 2015). Uncertainty is inherent within all forecasting models, as no model can predict future shocks or unforeseen events like wars, pandemics, or major technological breakthroughs. Yet, this uncertainty can be compounded by the lack of high quality longitudinal historic migration data in most places that makes it difficult to know precisely how people have moved in the past, and thus how they might move in the future. Further, migration models tend to focus on economic or demographic variables, but social, political, and cultural factors also play a role in determining who migrates, where they go, and the degree of choice in the migration process. Migration modelers still struggle to capture the nuanced relationship between these interacting drivers of migration in different socioeconomic contexts due to difficulties with gathering or accessing the relevant data as well as challenges isolating the relevant variables from one another theoretically and empirically (Lutz and Goldstein, 2004).
Climate-related migration modeling emerges in this context, as researchers incorporate climate-related indicators into population and migration models of all kinds. Climate-related variables are not yet commonly included in more general migration modeling, but a sub-field of climate-related migration modeling has developed to address this gap. Researchers utilize a variety of models from different disciplines to forecast climate-related migration.
Climate-related forecasting model types include: exposure models that overlay climate-related hazards on a population distribution map to identify at-risk populations; agent-based models that simulate the actions and interactions of individual agents; gravity models that use population size, distance, and other variables to project future population distributions; radiation models that use population size and distance to model the flows of people between places; statistical extrapolation models or discrete history event models that clarify historical climate-migration interactions to project future trends; systems dynamics models that simulate the non-linear behavior of complex systems using complex econometric models and utility functions; computable general equilibrium models that use large, economic models to assess potential policy effects on real-world economic problems; integrated assessment models that integrate human systems and natural systems into one modeling framework to support informed policy making; and machine learning models that have the ability to process large amounts of data and draw inferences from patterns within the data, potentially identifying thresholds or tipping points in migration systems.
Model types differ significantly in assumptions made, data inputs required, and the nature of results. Each model type has advantages, disadvantages, and a preferred scope of application. For example, agent-based models (ABMs) are data-intensive models that are well suited to explore nuanced questions and mechanisms in circumscribed geographic settings such as villages, cities, and other sub-national settings (Rigaud et al., 2018). ABMs model the behavior of autonomous agents to explore how individual actors interact with their environment, influencing their migration decisions, and leading to changes at the population level (Thober et al., 2018).
ABMs tend to require rich data inputs to calibrate the model. When this data exists, they are able to explore causal and feedback mechanisms, migration motivations, and complex decision-making. By exploring variations in migration or staying behavior based on individual and household characteristics, they also have the potential to explore differences in aspiration/ability that might lead to different migration and immobility outcomes (Figure 1). Typically, ABMs use data obtained through household survey (ideally longitudinal) research. ABMs tend to estimate volumes, or relative increases or decreases in migration flows from a particular area, rather than estimating how many people will move to destination A vs. destination B. Like other models, ABMs are more accurate at predicting short-term changes in the volume and composition of migration flows.
Gravity models are another popular but fundamentally different alternative. Gravity models tend to forecast spatial patterns over large geographic areas, such as entire nations or regions. The name comes from Newton's law of gravity, which states that any two bodies attract one another with a force proportional to the product of their masses and inversely proportional to the square of the distance between them. In gravity models, population size is indicative of relative “attractiveness,” but the force of attraction decays with distance. From this basic interplay of population size and distance, additional inputs can be added to explore their effects on future population distributions, including climate variables.
Gravity models do not directly model migration. Instead, climate-related migration is assumed to be the primary driver of deviations between population distributions in model runs that include climate impacts and the development-only (the “no climate”) models that include non-climate related drivers (Rigaud et al., 2018). This relatively straightforward approach makes them an attractive choice for migration modelers. However, using population as a proxy for migration can also be problematic, because population is also a function of changing administrative boundaries, fertility, and mortality. Fertility and mortality rates change over time and are also affected by climate change (Casey et al., 2019; Vollset et al., 2020; Gerlagh et al., 2023), yet in many gravity models, fertility and mortality rates are held constant.
Strengths of the gravity model include the ability to reproduce past shifts in population distribution, providing some assurance that if population trends continue to behave as in the past, these models can be trusted to forecast future trends. Gravity models are also relatively flexible in terms of data inputs and can generate results over broad geographic scales. However, these models have limitations. First, global or regional models often rely on census data collected over 10-year increments, which means the models may not accurately represent mobility patterns over short periods of time and from places where census data is rarely or poorly collected. Census data typically focuses on interprovincial moves, though research suggests that in many places climate-related migration occurs mostly over short distances (Hoffmann et al., 2020). Thus, gravity models can over represent long-distance rather than short-distance movers, which may reflect different segments of society. Further, these models cannot tell us anything about migration motivations or the degree to which migration is voluntary or forced. Finally, they do not forecast migration well at a micro level.3 For this reason, they are not typically utilized for forecasting migration from small island states or other small geographic regions.
Another approach to forecasting climate-related migration draws on discrete history event modeling via various kinds of econometric models. These approaches use historical data to evaluate how sudden- and/or slow-onset climate impacts affected internal or international migration patterns in the past. Those historical relationships are then used to project the probability of future migration under different climate scenarios. Discrete history event modeling has the advantage of basing model assumptions in real-world experiences, rather than extrapolating migration trends from population projections as gravity models do. However, these studies need to carefully control for other determinants of migration to avoid overestimating the influence of environmental factors on migration outcomes. Discrete history event modeling has been fundamental to enhancing our theoretical understanding of the climate-migration-development nexus (see, for example, Henry et al., 2004; Feng et al., 2010; Gray and Mueller, 2012; Nawrotzki et al., 2015; Gray and Wise, 2016). Not all studies that use this approach attempt to forecast future migration; nevertheless, discrete history event modeling contributes to the evidence base and theoretical assumptions that inform the development of forecasting models. In this article, we refer to discrete history event models that use their findings to forecast migration as “statistical extrapolation models.”
Although ABMs, gravity models, and statistical extrapolation approaches are some of the better-known model types, modelers continue to experiment with many others. Radiation models, for example, have been used to forecast migration flows between places with very few data inputs. They rely on basic inputs related to population size, distance, and climate, much like gravity models. The same limitations associated with using population as a proxy for migration discussed above for gravity models also apply to radiation models. Systems dynamics models offer the opportunity to explore complex systems dynamics and the potential impact of different policy scenarios, but they tend to lack spatial specificity. Computable general equilibrium and integrated assessment models share a similar focus on exploring the effects of policies on societal outcomes but have only recently been applied to forecast climate-related migration. There is significant interest in machine learning models, because in theory they could process large amounts of data to identify thresholds in climate-migration relationships, but the large data-inputs required to use machine-learning significantly limits their application in most contexts.
To understand the state of the field in climate migration forecasting, we conducted a systematic literature review. Our initial search in SCOPUS, Web of Science, and Proquest identified 269 unique papers that included the terms “climate,” “migration,” and “model” in the title or abstract. An additional search for relevant gray literature in PAIS, IMF eLibrary, OECD iLibrary, CIAO, and Google yielded 64 unique results.4 We also conducted a search for migration models that included climate change as a variable which added one additional paper. We did not limit the search based on date of publication. Once duplicates and papers focused on animal migration were removed, 115 articles remained. We then removed papers that only analyzed past migration (not addressing future migration), as well as all theoretical and review papers. Finally, we incorporated several articles that were found through country-specific searches (e.g., small island states) or recommended in expert interviews (e.g., discrete history event models that had a small or secondary section focused on forecasting). In total, we identified 30 articles that specifically address the topic of climate-related migration forecasting.
The 30 articles were then coded using the program SysRev along a variety of dimensions, including model type, geographic scope, migration type, model inputs and outputs, and climate factors. Each model was coded twice by two researchers, and discrepancies were double-checked and rectified by the research team. An overview of the thirty models is provided in the Supplementary material, and a summary of our literature review process in Appendix A.1.
Table 1 presents an overview of the key characteristics of climate migration forecasting models reviewed. Forecasting models covered multiple scales of migration (e.g., sub-national, national, regional, and global). Some forecast migration at one scale, while others combine scales and are counted twice in the summary statistics. For example, 70% (21) of the models forecasted trends at the national level, 20% (6) at the global level, 20% (6) at the regional level, and 27% (8) at the subnational level. Of those models that focused on specific countries, seven focused on the United States, six on Bangladesh, two on Brazil, two on Mexico, and two on Thailand (Figure 2). One model focused on Burkina Faso, Central America, Kiribati, Maldives, Mali, the United Kingdom, New Zealand, and Nigeria, respectively. The Groundswell Reports include country-level analyses for Ethiopia, Bangladesh, Mexico, Vietnam, Morocco, and the Kyrgyz Republic (see Sec. 5.1).
Of the models reviewed, 33% (10) employed the agent-based model, 27% (8) used an econometric model (e.g., 2SLS, probit, Bayesian, ANOVA, statistical extrapolation), and 17 and 7% (5 and 2) employed the gravity model and the radiation model, respectively. Integrated multi-regional, applied general equilibrium, spatial equilibrium, residential sorting, and system dynamics models were each utilized once. The temporal range of predictions spanned from 2030 to 2100. Notably, models do not typically provide sequential predictions but rather a discrete prediction for migration in 2030 or in 2100, for instance. One paper estimated future migration based on degree of future climate-related stress (e.g., one standard deviation increase in soil salinization), as opposed to a specific timescale. Overall, most papers provided projections for 2100 (41%) or 2050 (31%).
The most common climate-related data inputs included precipitation (e.g., average rainfall, monthly and yearly) (53%), temperature (e.g., average temperature) (47%), sea level rise (43%), droughts (13%), and storms (7%). The pairing of precipitation and temperature was most common across all models, with 37% of the papers analyzing these together. Some models also included climate impacts like crop yields and water availability, or sunshine hours, elevation, days of extreme heat, erosion, and flooding. Climate inputs originated from a variety of sources ranging from national weather station data to independent survey data. However, the most common source was the representative concentration pathways (RCPs). Forecasts that take a scenarios-based approach most often use RCPs to project future climate scenarios. RCPs describe the possible trajectories for greenhouse gas emissions and can be used for climate modeling and research.
As Figure 1 highlights, many political, economic, demographic, socio-cultural, technological and other environmental factors mediate the effects of climate change on migration or immobility outcomes. However, no model can incorporate all relevant mediating factors. The most common development-related data inputs in the forecasting models we assessed are the shared socioeconomic pathways (SSPs). SSPs are scenarios for how the world might evolve in the absence of the implementation of additional climate policies. They include indicators of population, economic growth, education, urbanization, and the rate of technological development. SSPs and RCPs are the most common aggregate indicators used to forecast potential future scenarios of development and climate change trajectories.
The majority of models include additional or independent inputs related to population distribution and the economic, political, or social context (see Supplementary material). Additional demographic inputs include education level, age, gender or sex, rural or urban location, marital status, and number of children. Many of these variables are more easily incorporated into models that focus on micro-level household dynamics, such as ABMs. Two models also include population, fertility, or mortality projections. Economic inputs—including GDP, household income, and occupation—are included in 17 of the models analyzed. Fewer models consider other economic factors, such as local wage rates of wage differences between regions, percent of the population working in agriculture, employment data, household assets, and local amenities.
Models include political and social factors less frequently than economic inputs. Only 17% of the identified models included political inputs and 30% included social inputs. Political inputs can include government stability, and the freedoms, rights, and liberties enjoyed by citizens. In some cases, political inputs measure the political feasibility of climate adaptation through, for example, policies or global cooperation. Notably, only one model considered conflict. It does so by assuming that conflicts will occur in countries where agricultural goods” prices increase by more than 10%, as compared to a non-climate change scenario (Burzyński et al., 2021). Social inputs tend to capture social networks, or the connections an individual or household has to others at origin or at a potential destination. Models utilized survey data related to social networks, remittances, and diaspora size or pre-existing datasets from the World Bank or United Nations.
Models vary in the type of migration projected. Of the models we reviewed, the majority (24 or 80%) forecast internal migration trends, and just over 40% assess international migration (13 or 43%). Eight models explore both internal and international migration. Most models do not state explicitly whether they are forecasting temporary or permanent migration. Short-term or temporary migration generally refers to migration lasting between 3 and 12 months, and long-term or permanent migration generally refers to a change of residence for 1 year or more (though definitions vary by country). Only 20% of the models explicitly focus on permanent migration, while 10% investigate temporary, seasonal, or circular migration. Only one model considers cascading migration, which in this case refers to the impacts that in-migration may have on out-migration from the same location (De Lellis et al., 2021). One model directly forecasts immobility (Smirnov et al., 2023). Gravity models have indirectly estimated trapped populations, suggesting many people will become trapped in a closed border scenario, but the models are not designed to directly forecast involuntary immobility (Rigaud et al., 2018; Jones, 2020; Clement et al., 2021).
Depending on the model type, future climate-related migration is forecast directly or indirectly. Gravity models forecast climate-related migration indirectly. In gravity models, climate-related migration is assumed to constitute the difference in projected population distributions between scenarios with and without varying degrees of climate change. Other models, such as the agent-based models reviewed here, estimate relative changes in total out-migration, in-migration, or return migration. However, their results tend to be aspatial, meaning they do not indicate the trajectories migrants follow. Some models present numerical estimates for future climate-related migrants, while others present their findings in terms of percentage increases or decreases in migration.
Scenarios-based outputs are increasingly common. Scenarios forecasting presents a range of possible migration outcomes based on different climate futures—for example, status quo, low emissions, or high emissions futures. Various development scenarios can also be paired with climate scenarios. Finally, some models may pair estimated increases in migration to urban areas with data on food, housing, and employment to anticipate the material demands future in-migration would generate (Davis et al., 2018).
Section 4 presents a general picture of the field of climate-related migration forecasting. To illustrate more clearly the variety of approaches, and the strengths and weaknesses of different climate-related migration forecasting models, this section presents an in-depth analysis of five models purposely selected for variation across model type, world regions, and geographic scope. These five models are summarized in Table 2, and the Supplementary material provide further details about data inputs and outputs. The main approaches, contributions, key findings, and limitations of each model are reviewed below. The first Groundswell report involved six European and American institutions and took nearly 2 years to complete. Findings were oriented toward a broader audience and published as a World Bank report (Model 1). Models 2 to 5 are published in peer-reviewed journals and produced by smaller teams of academic experts.
The Groundswell reports provide the first global picture of the potential scale of internal climate-related migration across six world regions: Sub-Saharan Africa, South Asia, and Latin America (Part I) and East Asia and the Pacific, North Africa, and Eastern Europe and Central Asia (Part II). Within each region, a country-level case study is included: Ethiopia, Bangladesh, Mexico, Vietnam, Morocco, and the Kyrgyz Republic. The Groundswell reports apply a scenarios-based gravity model to forecast future population distributions and areas that are likely to see greater in- and out-migration under different climate and development scenarios. The model applies demographic, socioeconomic, and climate impact data at a 14-square kilometer grid cell level using the Gridded World Population dataset to model likely shifts in population within countries. To address the uncertainties of analyzing migration over the next 30 years, the report considers three potential climate and development scenarios (using RCP and SSP scenarios as inputs): a pessimistic reference scenario, a more inclusive development scenario, and a more climate-friendly scenario. Climate impacts considered by the model include water scarcity, declining crop yields, and sea level rise. The Groundswell model does not directly model migration. Instead, climate-related migration is assumed to be the primary driver of deviations between population distributions in model runs that include climate impacts and the development-only (the “no climate”) models that include non-climate related drivers (Rigaud et al., 2018).
The Groundswell reports provide a global estimate of up to 216 million internal climate migrants by 2050 across all six regions, but projections vary significantly depending on the climate and development scenario. Projections tend to be higher for pessimistic scenarios vs. more optimistic climate and development scenarios. However, there are important regional differences. In East Africa, for example, there is a larger share of climate-related migrants relative to the general population under more inclusive development scenarios as compared to the pessimistic scenario. This may be explained by development-driven migration; more people tend to migrate as they gain access to higher education, incomes, and infrastructure (see also De Haas, 2010). Estimates of climate migrants as a percentage of a region's total population generally fluctuates between 0 and 3% of a region's total population, with North Africa being an exception where climate-related internal migration could reach as much as 6% (13 million, or half of all internal migrants) in 2050 under the pessimistic scenario.
The Groundswell reports reveal important insights into how slow-onset climate change impacts, population dynamics, and development contexts could shape future mobility trends. The model is notable for its flexible data inputs, scalability, and application across world regions. Importantly, Groundswell projections include relatively granular maps depicting which locations are likely to see more or less in- or out-migration, while many other modeling approaches lack this spatial information.
There are also limitations to the Groundswell model. Because it uses population as a proxy for migration, the model is subject to the same limitations described in Section 2.2 for gravity models generally. Population is also a function of changing administrative boundaries, fertility, and mortality. Fertility and mortality rates can also change in response to development or climate changes (Casey et al., 2019; Vollset et al., 2020; Gerlagh et al., 2023), but these are held constant in the Groundswell models. Further, there is also no way to distinguish between “distress” or “opportunity” migration under these different scenarios, or at a more fundamental level, whether “climate migration” is an accurate characterization of the many different kinds of movements that may occur under different climate and development scenarios (including economic, educational, marriage, or conflict-related movements).
Further, the Groundswell model does not attempt to forecast international migration, planned relocation, involuntary immobility, or cascading effects. It omits many political and economic factors (like access to land, resources, jobs, conflict, or shocks) that will certainly affect future migration trends. It cannot capture migration over distances of < 14 kilometers, and thus cannot be applied to smaller geographic areas such as small island states. The model does not include short-term climate variations or sudden-onset events. Like other forecasting models, it does not incorporate the impact of future adaptation efforts (e.g., improved crop varieties, irrigation, water conservation agriculture, or coastal defenses) into its projections.
These limitations recognized, the Groundswell models represent an important step in climate-related migration modeling, that inspired future gravity modeling used in the Great Climate Migration project in Latin America (Lustgarten, 2020) and the Amakrane et al. (2023).
Model 2 by Davis et al. (2018) applies a diffusion-based model of human mobility in combination with population, geographic, and climatic data to estimate the sources, destinations, and flux of potential migrants as driven by sea level rise in Bangladesh in the years 2050 and 2100. By linking the sources of migrants displaced by sea level rise with their likely destinations, the model purports to offer an effective approach for predicting climate-driven migration flows, especially in data-limited settings. The authors describe the model as universal because it uses few data inputs and is parameter-free.
According to the paper's authors, the baseline model results showed good agreement with available division-level internal migration from the 2011 Bangladesh census, meaning the model predictions accurately aligned with actual internal migration patterns in Bangladesh using information on population distribution and distance. However, this constitutes only a one-year projection (using 2010 data to forecast 2011 trends). By mid-century, the model estimates that nearly 900,000 people are likely to migrate as a result of direct inundation from mean sea level rise alone, and Dhaka will be the top destination for migration. In large part because of the generally high population density across Bangladesh, however, the authors find most migrants will choose destinations close to their homes. The authors also analyze the additional jobs, housing, and food that would be required to support these migrants at their expected destinations.
This model is distinct for how few data inputs are required. It builds on a radiation model published by Simini et al. (2012), which estimated internal and commuting mobility trends in the United States based only on population distribution and distance estimates. By adding data inputs on elevation and projected sea level rise, Davis et al. (2018) present a streamlined and simple approach to forecasting displacement from sea level rise. The model was expanded by De Lellis et al. (2021), who added a single parameter to the model on baseline migration rates, as well as a “resilience index.” These additions led to different predictions, however, namely a wider spatial distribution of migrants and a predicted outflow from Dhaka. Other models focused on sea level rise and migration in Bangladesh come to still different conclusions. Chen and Mueller (2018), for example, use a statistical extrapolation approach and find that inundation has negligible effects on internal migration in Bangladesh; gradual increases in soil salinity have more direct and significant effects on internal and international migration trends. These discrepant findings raise concerns about the real-world applicability of the model to forecast climate-related migration over the longer term. Model projections remain heavily dependent on the baseline assumptions, inputs, and parameters modelers choose to include or exclude.
Model 3, the Systems Dynamics Model (Naugle et al., 2022), couples migration decision making and behavior with the interacting dynamics of economy, labor, population, violence, governance, water, food, and disease. The model is applied to a test case of migration within and beyond Mali. This model is notable for the wide range of factors incorporated beyond climate and population variables, particularly political, economic, health, and conflict-related factors, and its experimentation with how several different policy interventions might affect migration outcomes. The systems dynamics model is designed to allow for interactive effects, causal loops, and flows. Each component within the model can impact other factors, not just migration. This allows the model to move beyond linear, input/output modeling approaches to incorporate non-linear, “systems” dynamics.
Model outputs are given in terms of the fraction of the Malian population choosing to live in each simulated region. Potential locations include rural Mali, urban Mali, neighboring countries (Burkina Faso, Côte d'Ivoire, Gabon, Gambia, Ghana, Guinea, Mauritania, Niger, Senegal), the United States, and “the rest of the world” (as one category). The model generally finds that as temperatures increase, economic factors make migration from Mali to other locations more attractive, and the population tends to move out of both urban and rural areas of Mali toward neighboring countries, the United States, and the rest of the world. The model forecasts the impact of various policy options on migration outcomes and finds that providing contraception (reducing birth rates) reduces migration by limiting pressures on the economy, resources, food availability, and water availability. Increasing the effectiveness of governance in the model improves the economic situation by increasing the internal gross regional product, and ultimately reduces migration. Increasing infrastructure and services provided by the Malian government was relatively ineffective at reducing migration.
The focus of this model is on exploring feedback effects and policy interventions. To do so, some baseline assumptions are unrealistic. For example, in the base case simulation, temperatures remain stable throughout the time horizon and gross regional product tracks (World Bank, 2017) projections. The authors do not take a position on the validity of the base case projection. Rather, they emphasize the opportunity to explore the differences between this and climate change scenarios to understand the causes of variations in the results. This highlights that such a model is best used to explore systems dynamics and potential policy effects, rather than generate numerical projections of future climate-related migration.
Model 4 by Entwisle et al. (2020) uses an ABM focused on land use, social networks, and household dynamics to examine how extreme floods and droughts might affect migration from 41 rural villages in Northeast Thailand, where rice cultivation is common. The ABM models the dynamic and interactive pathways through which climate-migration relationships operate, including out and return migration, for each village. This model pays attention to variables that existing migration research confirms are fundamental to migration systems but are often left out of forecasting models: namely social networks, life-course dynamics, and return migration. Importantly, it starts from the assumption that climate change does not “uproot” otherwise immobile populations but will have impacts on already established migration systems.
The ABM is grounded in longitudinal survey, qualitative, spatial, social, and environmental data. Like Model 1, this approach focuses on how changes in precipitation affect crop yields and thus livelihoods and household assets and thus does not assume a direct “climate effect” on migration. It incorporates additional feedback into the model through, for example, social networks and remittances. Interestingly, the results find minimal to no climate-related effects on rural out-migration. One potential reason for this is that out-migration is already a normal part of social systems in this area, and these continue for other non-climate related reasons. However, the model finds that increased floods and droughts lead to a notable decline in return migration.
Like systems dynamics models, the ABM approach is able to run repeated and nuanced experimental scenarios. Running the model with and without social networks yields important differences in outcomes. In every scenario, without the facilitating effects of social networks, climate impacts decrease out-migration. This may be because social networks significantly lower the costs of moving, by sending money and helping prospective migrants (usually relatives) find housing and work. Without social networks, migration is a more individual decision and becomes costlier. The model also finds return migration stays constant without social networks. A likely explanation is that social networks are the main mechanism through which prospective return migrants learn about the hardships of floods or drought. Without social ties, decisions to return home are less affected by these climate impacts.
This ABM model has several strengths. First, it is grounded in decades of panel survey data. Further, it looks at the full spectrum of migration, both out- and return-migration, and incorporates different propensities for migration across the life-course. Although the authors do not explore this much in their paper, the model is better suited than others to assess heterogeneity (due to its micro-level, localized inputs) within and between villages and provide micro-level evaluations of how potential climate-related stresses might disproportionately impact marginalized or vulnerable populations.
There are several limitations to this approach for forecasting future climate-related migration. First, the model predicts increases and decreases in rates of out- or return-migration but yields no information on the spatial trajectories of potential migration flows. Further, the data demands of this model make it challenging to scale or apply to other areas that lack reliable, longitudinal data. Experts also note that this ABM model, like many other ABMs and computational models, relies on assumptions about what the underlying utility function looks like—that is, what people prioritize when they consider the costs and benefits of migration and make migration decisions. In reality, utility functions differ significantly across populations, based on education, socioeconomic status, gender, culture, or personal disposition. For these and other reasons, the authors expressly state that their interest is at the level of model development and theoretical development. “Our intent…is to use the model to explore the implications of the theories embedded within it” (Entwisle et al., 2020, p. 1472). The model does not seek to provide real-world projections of future climate-related migration for planning purposes. Nevertheless, it makes an important contribution to how modelers understand and can incorporate important but often overlooked factors (like social networks, life course or return dynamics) into climate-related migration forecasting models.
Model 5 by Chen and Mueller (2019) approaches climate-migration interactions using historical data; the relationships uncovered are then used to forecast future trends. This econometric model forecasts climate-related international migration from Bangladesh. It is notable for modeling several climate-related factors, including remote-sensing measures of flooding and rainfall and in-situ measures of monsoon onset, temperature, radiation, and soil salinity. It uses nationally representative migration data to evaluate which locations and population groups are more likely to migrate across the border to other South-East Asian countries, with a particular focus on international migration to India.
Chen and Mueller (2019) find that different climate variables vary in their relationship to cross-border migration. Short-term, adverse weather events are associated with decreased international migration, while increases in soil salinity are associated with increased cross-border migration. Soil salinity has a stronger effect on migration from poorer households. The model outputs do not provide an estimate of climate-related migrants by a particular date; rather, projections are based on the degree of future climate change. For example, the model predicts a total of 17,874 more migrants moving to India in response to a one standard deviation increase in soil salinity, with out-migration concentrating in the southwestern coastal regions of the country.5 The model predicts a total of 5,754 fewer migrants moving to India in response to an increase in flooding of one standard deviation. The authors do not attempt to forecast when those changes in soil salinity or inundation will occur.
Chen and Mueller (2019) examine household vulnerability to flooding and soil salinization to explore whether households with greater human, social, or physical capital are more inclined to migrate. They also consider the impact of age, gender, and religion on migration outcomes. They find that wealthier households are less likely to migrate in response to gradual changes in soil salinity, perhaps because they are better equipped to diversify livelihoods locally, while poorer households are more likely to use international migration as an adaptation strategy as soil salinity increases. These and other related findings provide important evidence for how climate-migration interactions might vary for different social groups and more vulnerable households. Chen and Mueller (2019) do not use these more nuanced analyses, however, to forecast future trends based on household characteristics.
The authors are transparent about the limitations of their model. They lack information on the duration of each event and are unable to validate whether moves are temporary or permanent. The measure for soil salinity is relatively coarse and focuses on changes over 5 years. This misses the potential mobility implications of seasonal or annual variations. They also note that soil salinity can result from changes in landscape and deforestation along the coast, sea level rise and storm surges and ground management. It is not clear what factors are contributing most to increase soil salinization and thus where policymakers should prioritize funding for adaptive investments. Finally, they note one drawback of using a large administrative dataset is the absence of detailed survey questions that would be required to more carefully explore the reasons for migration and obstacles potential migrants face.
As Section 5 shows, modeling approaches vary considerably, each bringing distinct strengths and weaknesses. Yet, there are several limitations that characterize the field of climate-related migration forecasting more generally. First, non-climate related drivers of migration, including political, economic, cultural, and social factors, remain underrepresented in the models reviewed. For example, only one model incorporates conflict as a potential driver (Burzyński et al., 2022), and just five of the thirty models reviewed incorporate covariates for social networks. Social networks are a fundamental migration facilitator, impacting who is most likely to move and where they will migrate (Haug, 2008). Also known as the “friends and family effect,” social networks significantly reduce the informational, capital, and social costs of moving (Hatton and Williamson, 1994; Nawrotzki et al., 2015). Often, social, cultural or political factors are not included as inputs because there is not sufficient or reliable data.
A second limitation is the predominant focus on slow-onset climate changes, excluding sudden-onset events or other forms of environmental change not directly caused by climate change. Many models forecast migration in relation to one or two climate hazards only (e.g., sea level rise, or temperature and precipitation), when in reality, several interrelated slow- and sudden-onset climate changes (alongside other forms of environmental change) may affect future population mobility or immobility (McLeman, 2018; De Sherbinin, 2020; Hauer et al., 2020). In the broader climate-migration literature, discrete history event modeling has been used to model historical migration responses to both sudden-onset events and multi-hazard environments (Gray and Mueller, 2012; Nawrotzki and Bakhtsiyarava, 2017). These findings can “ground truth” future projections, even if the exact timing or severity of future natural disasters remains unknown.6
A third limitation is the neglect of potential climate-related immobility. Only one of the thirty models (Smirnov et al., 2023) directly forecasts immobility, and none are able to distinguish between voluntary or involuntary forms. Alongside the push and pull factors that may motivate migration, migration trends are also shaped by retain and repel factors,7 as well as political, economic, or other constraints that deprive individuals and households of the capability to move (see Schewel, 2020 for a review). These place-based ties and migration constraints are not yet incorporated into the theoretical frameworks that underlie forecasting models, which means models risk overpredicting future migration.8 Climate-related immobility is as important a development and humanitarian concern as climate-related mobility. As climate change advances, the most vulnerable populations are those already disadvantaged groups who risk becoming “trapped” in ever-more precarious living conditions (Black et al., 2011). Not all populations living in environmentally stressed areas, however, see themselves as “trapped” or “involuntarily immobile.” A growing body of research finds a surprisingly significant portion of vulnerable populations do not want to migrate,9 raising important questions about the underlying utility functions we use in migration modeling (see Czaika and Reinprecht, 2022) and highlighting the need to invest in in-situ climate adaptation.
Finally, and perhaps most obviously, there are future unknowns that forecasting models cannot readily integrate. Scenarios based modeling, although it captures a wide range of possible futures, does not incorporate potential climate tipping points or irreversible environmental changes, such as the loss of the Amazon rainforest or the West Antarctic ice sheet, that could have devastating consequences for populations and ecosystems across the globe (Lenton et al., 2019). When climate-related events intensify and become increasingly widespread, this can have cascading effects on economic and social systems (e.g., global trade and supply networks) (Organisation for Economic Co-operation Development., 2008). Migration responses to climate change under these conditions could reach thresholds where the volumes, nature, direction, and composition of migration flows begin to diverge fundamentally from historical patterns (McLeman, 2018). And other major unknowns like pandemics, wars, or technological revolutions will exert their own effects on migration trends (de Valk et al., 2022). Though inherently challenging to model, the exclusion of these future uncertainties undermines the accuracy of long-term projections, especially to time horizons like 2100.
The forecasting models reviewed above provide insight into the wide range of approaches to estimating future climate-related migration. Uncertainty is inherent to any future-oriented exercise. As the statistician George Box famously said, “all models are wrong, but some are useful.” Forecasting models will always fall short of the complexities of reality, but models can still reduce uncertainty, even if they do not eliminate it completely. The models reviewed above are some of the best attempts to forecast climate-related migration, and modelers are making consistent efforts to push the field forward. Here, we outline several strategies for supporting these advancements, including new and refined approaches to modeling, greater collaboration between stakeholders and scholars from different disciplines, and improved data inputs.
There are several promising approaches to modeling that could improve climate-related migration forecasting. The first is advancing multi-level modeling, which combines model types to capitalize on their independent strengths. For example, gravity models could be used to identify areas that might see significantly greater rates of out- or in-migration, and agent-based models could be used in those targeted areas to model migration or immobility outcomes for different socioeconomic groups.
Further model refinement will also require inputs from a broad community of experts. Greater trans-disciplinary collaboration between natural and social scientists could help modelers capture climate-related and environmental conditions that are unaccounted for in current models. Hydrologists, for example, can provide relatively granular insight into household exposure to flooding and sea level rise, but their expertise is seldom incorporated into existing migration models. Prevailing models tend to assume the same level of exposure and vulnerability to climate events like flooding or sea level rise across a village or region, when in reality, the intensity of exposure can vary significantly (Davis et al., 2018; Rigaud et al., 2018).
Finally, and perhaps most importantly, improvements in data collection are fundamental to enhance climate-related migration forecasting. Data on past and present migration is extremely limited and unreliable in many world regions, particularly coveted migration flow data. Improved data collection requires investments in the statistical bureaus of climate-vulnerable countries to improve the quality and frequency of census data collection. More high-quality individual and household survey data are also needed to better capture existing migration systems, migration aspirations and capabilities, and perceptions of climate change. There have been some innovative advancements in collecting novel forms of data for climate-related migration forecasting (Isaacman et al., 2017; Ash and Obradovich, 2020; Luca et al., 2022; e.g., cell-phone data or experiments with remote sensing data), however these efforts are incipient and not yet widespread. There is growing enthusiasm to incorporate machine learning into forecasting to identify thresholds or tipping points in climate-migration interactions (Jones, 2017). However, machine learning requires very large data inputs, and is difficult to apply to many regions of the world where reliable data remains scarce.
In summary, the field of climate-related migration forecasting is still in its infancy, and at this nascent stage, forecasting models are not yet able to provide concrete numerical estimates of future climate-related migration. Modeling human migration is an exercise fraught with uncertainty and adding the dimension of climate change only compounds that uncertainty. Debates about causality in research on environmental change and migration remain unsettled (see Cottier et al., 2022), and given the political sensitivities that surround discussions of migration, it is important not to give the air of statistical precision to a social phenomenon that resists easy quantification (Lustgarten, 2020). For these reasons, scenarios-based forecasting approaches, which consider the full range of possible futures, are arguably more useful for real-world planning than singular narratives of future climate-migration. Scenarios-based forecasting can assist policymakers to consider a range of possible “what if” scenarios (Jones, 2020).
Given the high levels of uncertainty inherent within climate-related migration forecasting, policy makers and development planners should consider triangulating the predictions of forecasting models with insights from qualitative foresight exercises. Foresight exercises bring migration, climate, and regional experts together with local stakeholders to anticipate potential climate-related migration trends for particular countries and regions (cf. Vezzoli et al., 2017). Local stakeholders and scholars who specialize in a region may be able to identify gaps and inaccuracies in the inputs or assumptions of a given forecasting model, offer cultural and historical insights that might improve the interpretation of their findings, and offer caveats about the applicability of the models” findings for particular populations or sub-regions.
All authors listed have made a substantial, direct, and intellectual contribution to the work and approved it for publication.
This review was made possible by the generous support of the United States Agency for International Development (USAID) under the terms of contract no. 7200AA18C00057, which supports the Research Technical Assistance Center (RTAC).
The authors declare that the research was conducted in the absence of any commercial or financial relationships that could be construed as a potential conflict of interest.
All claims expressed in this article are solely those of the authors and do not necessarily represent those of their affiliated organizations, or those of the publisher, the editors and the reviewers. Any product that may be evaluated in this article, or claim that may be made by its manufacturer, is not guaranteed or endorsed by the publisher.
The Supplementary Material for this article can be found online at: https://www.frontiersin.org/articles/10.3389/fclim.2023.1189125/full#supplementary-material
1. ^The macro level refers to societal and structural level factors; the meso level refers to social and community-level factors; the micro refers to individual and household level factors.
2. ^de Haas (2021) integrated Amartya Sen's concept of ‘capability' into the model, giving rise to the complementary aspiration-capability framework, which focuses more explicitly on the relationship between migration and development (see also Carling and Schewel, 2018; Schewel, 2020).
3. ^The micro level refers to the individual and household level; the meso level refers to social and community-level; and macro level refers to the societal level.
4. ^Search terms in databases included: climate OR migration OR model OR drought OR water OR storm OR flood OR displacement OR mobility OR population movement AND country name. Search terms in Google included: migration model [country name].
5. ^To give a sense of relative scale, there were 2.5 million Bangladeshi migrants in India in 2020 (Singh, 2022).
6. ^The authors thank Valerie Mueller for this point.
7. ^Retain factors are the attractive conditions at home that encourage individuals to stay in their current location, while repel factors describe conditions in alternative locations that discourage migration (see Schewel, 2020).
8. ^The recent forecasting model developed by Benveniste et al. (2022) is a notable exception. It explores how climate change may affect what they call ‘resource constrained international immobility.'
9. ^For example, a recent survey by the Africa Climate Mobility Initiative (Amakrane et al., 2023) finds that majority of people (55%) who suffer from climate disruptions do not consider migrating. A smaller share (23%) have considered moving but lack the resources.
Abel, G., Bijak, J., Findlay, A., McCollum, D., and Wiśniowski, A. (2013). Forecasting environmental migration to the United Kingdom: an exploration using Bayesian models. Pop. Environ. 35, 183–203. doi: 10.1007/s11111-013-0186-8
Adams, H., and Kay, S. (2019). Migration as a human affair: integrating individual stress thresholds into quantitative models of climate migration. Environ. Sci. Policy 93, 129–138. doi: 10.1016/j.envsci.2018.10.015
Amakrane, K., Rosengaertner, S., Simpson, N. P., de Sherbinin, A., Linekar, J., Horwood, C., et al. (2023). African Shifts: The Africa Climate Mobility Report, Addressing Climate-Forced Migration & Displacement; Africa Climate Mobility Initiative and Global Centre for Climate Mobility. New York, NY.
Ash, K., and Obradovich, N. (2020). Climatic stress, internal migration, and syrian civil war onset. J. Conf. Resol. 64, 3–31. doi: 10.1177/0022002719864140
Barbieri, A. F., Domingues, E., Queiroz, B. L., Ruiz, R. M., Rigotti, J. I., Carvalho, J. A., et al. (2010). Climate change and population migration in Brazil's Northeast: scenarios for 2025–2050. Pop. Environ. 31, 344–370. doi: 10.1007/s11111-010-0105-1
Beckwith, J. (2019). Modeling Climate Driven Urban Migration in the United States REU Final Reports. Report 14. Portland State University. Available online at: https://pdxscholar.library.pdx.edu/reu_reports/14
Bell, A. R., Wrathall, D. J., Mueller, V., Chen, J., Oppenheimer, M., Hauer, M., et al. (2021). Migration towards Bangladesh coastlines projected to increase with sea-level rise through 2100. Environ. Res. Lett. 16, 024045. doi: 10.1088/1748-9326/abdc5b
Benveniste, H., Oppenheimer, M., and Fleurbaey, M. (2022). Climate change increases resource-constrained international immobility. Nat. Clim. Change 12, 634–641. doi: 10.1038/s41558-022-01401-w
Black, R., Adger, N., Arnel, N., Dercon, S., Geddes, A., Thomas, D., et al. (2011). Foresight: Migration and Global Environmental Change, Final Project Report. London: The Government Office for Science.
Boas, I., Farbotko, C., Adams, H., Sterly, H., Bush, S., Van der Geest, K., et al. (2019). Climate migration myths. Nat. Clim. Change 9, 901–903. doi: 10.1038/s41558-019-0633-3
Bredeloup, S. (2013). The figure of the adventurer as an African migrant. J. Afr. Cult. Stu. 25, 170–182. doi: 10.1080/13696815.2012.751870
Brown, O. (2008). Migration and climate change. United Nations. Int. Org. Migration' Res. Series 31, 1–14. doi: 10.18356/26de4416-en
Brown, O., and McLeman, R. (2013). Climate Change and Migration: An Overview. The Encyclopedia of Global Human Migration. London: Blackwell Publishing Ltd.
Burzyński, M., Deuster, C., Docquier, F., and Melo, D. e. J. (2022). Climate change, inequality, and human migration. J. Eur. Econ. Assoc. 20, 1145–1197. doi: 10.1093/jeea/jvab054
Burzyński, M., Docquier, F., and Scheewel, H. (2021). The geography of climate migration. J. Demograph. Econ. 87, 345–381. doi: 10.1017/dem.2021.6
Cai, R., and Oppenheimer, M. (2013). “An agent-based model of climate-induced agricultural labor migration,” in 2013 Annual Meeting (Washington, DC: Agricultural and Applied Economics Association), doi: 10.22004/ag.econ.150972
Cameron, M. P. (2018). Climate change, internal migration, and the future spatial distribution of population: a case study of New Zealand. Pop. Environ. 39, 239–260. doi: 10.1007/s11111-017-0289-8
Carling, J. (2002). Migration in the age of involuntary immobility: theoretical reflections and cape verdean experiences. J. Ethn. Migr. Stu. 28, 5–42. doi: 10.1080/13691830120103912
Carling, J., and Schewel, K. (2018). Revisiting aspiration and ability in international migration. J. Ethnic Migr. Stu. 44, 945–963. doi: 10.1080/1369183X.2017.1384146
Casey, G., Shayegh, S., Moreno-Cruz, J., Bunzl, M., Galor, O., Caldeira, K., et al. (2019). The impact of climate change on fertility. Environ. Res. Lett. 14, 054007. doi: 10.1088/1748-9326/ab0843
Cattaneo, C., and Massetti, E. (2019). Does harmful climate increase or decrease migration? Evidence from rural households in Nigeria. Clim. Change Econ. 10, 1950013. doi: 10.1142/S2010007819500131
Chen, J., and Mueller, V. (2018). Coastal climate change, soil salinity and human migration in Bangladesh. Nat. Clim. Change 8, 981–985. doi: 10.1038/s41558-018-0313-8
Chen, J., and Mueller, V. (2019). Climate-induced cross-border migration and change in demographic structure. Pop. Environ. 41, 98–125. doi: 10.1007/s11111-019-00328-3
Clemens, M. (2020). “The emigration life cycle: how development shapes emigration from poor countries,” in Center for Global Development Working Paper Series (Washington, DC: Center for Global Development). doi: 10.2139/ssrn.3679020
Clement, V., Rigaud, K. K., De Sherbinin, J., Jones, A., Adamo, B., Schewe, S., et al. (2021). Groundswell Part 2. Washington, DC: World Bank.
Cottier, F., Flahaux, M. L., Ribot, J., Seager, R., and Ssekajja, G. (2022). Framing the frame: Cause and effect in climate-related migration. World Dev. 158, 106016. doi: 10.1016/j.worlddev.2022.106016
Cundill, G., Singh, C., Adger, W. N., De Campos, D., Vincent, R. S., Tebboth, K., et al. (2021). Toward a climate mobilities research agenda: intersectionality, immobility, and policy responses. Global Environ. Change 69, 102315. doi: 10.1016/j.gloenvcha.2021.102315
Czaika, M., and Reinprecht, C. (2022). Why do people stay put in environmentally stressful regions? Cognitive bias and heuristics in migration decision-making. Reg. Environ. Change 22, 84. doi: 10.1007/s10113-022-01934-y
Davis, K. F., Bhattachan, A., D'Odorico, P., and Suweis, S. (2018). A universal model for predicting human migration under climate change: examining future sea level rise in Bangladesh. Environ. Res. Lett. 13, 064030. doi: 10.1088/1748-9326/aac4d4
De Haas, D., Castles, H. S., and Miller, M. J. (2020a). The Age of Migration: International Population Movements in the Modern World, 6th Edn. London: Red Globe Press.
De Haas, D., Fransen, H., Natter, S., Schewel, K. K., and Vezzoli, S. (2020b). Social Transformation. Oxford: International Migration Institute Network (IMI).
De Haas, H. (2010). Migration Transitions: A Theoretical and Empirical Inquiry into the Developmental Drivers of International Migration. IMI Working Paper Series, WP24. Oxford: International Migration Institute.
de Haas, H. (2021). A theory of migration: the aspirations-capabilities framework. Comp. Migrat. Stud. 9:8. doi: 10.1186/s40878-020-00210-4
De Lellis, D., Ruiz Marín, P. M., and Porfiri, M. (2021). Modeling human migration under environmental change: a case study of the effect of sea level rise in Bangladesh. Earth's Future 9, e2020EF. doi: 10.1029/2020EF001931
De Sherbinin, A. (2020). Climate Impacts as Drivers of Migration. Washington, DC: Migration Policy Institute.
de Valk, H. A. G., Acostamadiedo, E., Guan, Q., Melde, S., Mooyaart, J., Sohst, R. R., et al. (2022). “How to predict future migration: different methods explained and compared,” in Introduction to Migration Studies. IMISCOE Research Series, ed P. Scholten (Cham: Springer).
Disney, G., Wiśniowski, A., Forster, J. J., Smith, P. W., and Bijak, J. (2015). Evaluation of Existing Migration Forecasting Methods and Models. Report for the Migration Advisory Committee: Commissioned research. Southampton: ESRC Centre for Population Change, University of Southampton.
Entwisle, B., Verdery, A., and Williams, N. (2020). Climate change and migration: new insights from a dynamic model of out-migration and return migration. Am. J. Sociol. 125, 1469–1512. doi: 10.1086/709463
Entwisle, B., Williams, N. E., Verdery, A. M., Rindfuss, R. R., Walsh, S. J., Malanson, G. P., et al. (2016). Climate shocks and migration: an agent-based modeling approach. Pop. Environ. 38, 47–71. doi: 10.1007/s11111-016-0254-y
Fan, Q., Fisher-Vanden, K., and Klaiber, H. A. (2018). Climate change, migration, and regional economic impacts in the United States. J. Assoc. Environ. Res. Econ. 5, 643–671. doi: 10.1086/697168
Fan, Q., Klaiber, H. A., and Fisher-Vanden, K. (2012). “Climate change impacts on U.S. migration and household location choice,” in 2012 Annual Meeting (Seattle, WA: Agricultural and Applied Economics Association). doi: 10.22004/ag.econ.124588
Farbotko, C., Dun, O., Thornton, F., McNamara, K. E., and McMichael, C. (2020). Relocation planning must address voluntary immobility. Nat. Clim. Change 10, 702–704. doi: 10.1038/s41558-020-0829-6
Farbotko, C., and McMichael, C. (2019). Voluntary immobility and existential security in a changing climate in the Pacific. Asia Pacific Viewpoint 60, 148–162. doi: 10.1111/apv.12231
Feng, S., Krueger, A. B., and Oppenheimer, M. (2010). Linkages among climate change, crop yields and Mexico–US cross-border migration. Proc. Nat. Acad. Sci. 107, 14257–14262. doi: 10.1073/pnas.1002632107
Feng, S., Oppenheimer, M., and Schlenker, W. (2012). Climate Change, Crop Yields, and Internal Migration in the United States (No. w17734). New York, NY: National Bureau of Economic Research.
Fussell, E., Hunter, L. M., and Gray, C. L. (2014). Measuring the environmental dimensions of human migration: the demographer's toolkit. Global Environ. Chang. 28, 182–191. doi: 10.1016/j.gloenvcha.2014.07.001
Gemenne, F. (2011). Why the numbers don't add up: a review of estimates and predictions of people displaced by environmental changes. Global Environ. Change 21, 41–49.
Gerlagh, R., Lupi, V., and Galeotti, M. (2023). Fertility and climate change. The Scand. J. Econ. 125, 208–252. doi: 10.1111/sjoe.12520
Goodman, S. (2007). National Security and the Threat of Climate Change. England: The CNA Corporation.
Gray, C., and Mueller, V. (2012). Drought and population mobility in rural Ethiopia. World Dev. 40, 134–145. doi: 10.1016/j.worlddev.2011.05.023
Gray, C., and Wise, E. (2016). Country-specific effects of climate variability on human migration. Clim. Change 135, 555–568. doi: 10.1007/s10584-015-1592-y
Hassani-Mahmooei, B., and Parris, B. W. (2012). Climate change and internal migration patterns in Bangladesh: an agent-based model. Environ. Dev. Econ. 17, 763–780. doi: 10.1017/S1355770X12000290
Hatton, T. J., and Williamson, J. G. (1994). What drove the mass migrations from Europe in the late nineteenth century? Popul. Dev. Rev. 20, 533–559. doi: 10.2307/2137600
Hauer, M. E., Fussell, E., Mueller, V., Burkett, M., Call, M., Abel, K., et al. (2020). Sea-level rise and human migration. Nat. Rev. Earth Environ. 1, 28–39. doi: 10.1038/s43017-019-0002-9
Haug, S. (2008). Migration networks and migration decision-making. J. Ethn. Migrat. Stud. 34, 585–605.
Henry, S., Schoumaker, B., and Beauchemin, C. (2004). The impact of rainfall on the first out-migration: a multi-level event-history analysis in Burkina Faso. Pop. Environ. 25, 423–460. doi: 10.1023/B:POEN.0000036928.17696.e8
Hoffmann, R., Dimitrova, A., Muttarak, R., Crespo Cuaresma, J., and Peisker, J. (2020). A meta-analysis of country-level studies on environmental change and migration. Nat. Clim. Change 10, 904–912. doi: 10.1038/s41558-020-0898-6
Isaacman, S., Frias-Martinez, V., Hong, L., and Frias-Martinez, E. (2017). “Climate change induced migrations from a cell phone perspective,” in NetMob Conference (Milan).
Jones, B. (2020). Modeling Climate Change-Induced Migration in Central America and Mexico. Methodological Report. New York, NY: Marxe School of Public and International Affairs, Baruch College and the CUNY Institute for Demographic Research (CIDR).
Jones, N. (2017). How machine learning could help to improve climate forecasts. Nature 548, 7668. doi: 10.1038/548379a
Kniveton, D. R., Smith, C. D., and Black, R. (2012). Emerging migration flows in a changing climate in dryland Africa. Nat. Clim. Change 2, 444–447. doi: 10.1038/nclimate1447
Lenton, T. M., Rockström, J., Gaffney, O., Rahmstorf, S., Richardson, K., Steffen, W., et al. (2019). Climate tipping points - too risky to bet against. Nature 575, 592–595. doi: 10.1038/d41586-019-03595-0
Luca, M., Barlacchi, G., Oliver, N., and Lepri, B. (2022). “Leveraging mobile phone data for migration flows,” in Data Science for Migration and Mobility, eds A. Salah, E. E. Korkmaz, and T. Bircan (London: British Academy Scholarship Online).
Lutz, W., and Goldstein, J. R. (2004). Introduction: how to deal with uncertainty in population forecasting? Int. Stat. Rev. 72, 1–4. doi: 10.1111/j.1751-5823.2004.tb00219.x
McLeman, R. (2018). Thresholds in climate migration. Pop. Environ. 39, 319–338. doi: 10.1007/s11111-017-0290-2
McLeman, R. A. (2014). Climate and Human Migration: Past Experiences, Future Challenges. Cambridge: Cambridge University Press.
Naugle, A. B., Backus, G. A., Tidwell, V. C., Kistin-Keller, E., and Villa, D. L. (2022). “A regional model of climate change and human migration,” in Research Anthology on Environmental and Societal Impacts of Climate Change, eds M. Ait-El-Mokhtar, A. Boutasknit, R. Ben-Laouane (New York, NY: IGI Global), 449–71.
Nawrotzki, R. J., and Bakhtsiyarava, M. (2017). International climate migration: evidence for the climate inhibitor mechanism and the agricultural pathway. Pop. Space Place 23, e2033. doi: 10.1002/psp.2033
Nawrotzki, R. J., Riosmena, F., Hunter, L. M., and Runfola, D. M. (2015). Amplification or suppression: Social networks and the climate change—migration association in rural Mexico. Global Environ. Change 35, 463–474. doi: 10.1016/j.gloenvcha.2015.09.002
Oakes, R., Milan, A., and Campbell, J. (2016). Kiribati: Climate Change and Migration - Relationships Between Household Vulnerability, Human Mobility and Climate Change. United Nations University Institute for Environment and Human Security. Available online at: http://collections.unu.edu/view/UNU:5903 (accessed May 20, 2023).
Oliveira, J., and Pereda, P. (2020). The impact of climate change on internal migration in Brazil. J. Environ. Econ. Manage. 103, 102340. doi: 10.1016/j.jeem.2020.102340
Olwig, K. F. (2018). Migration as adventure: narrative self-representation among Caribbean migrants in Denmark. Ethnos 83, 156–171. doi: 10.1080/00141844.2016.1209538
Organisation for Economic Co-operation Development. (2008). International Migration Outlook 2008—OECD. Available online at: https://www.oecd.org/els/mig/internationalmigrationoutlook2008.htm (accessed November 27, 2023).
Petracou, E. V., Xepapadeas, A., and Yannacopoulos, A. N. (2017). Climate change and environmentally induced migration across regions: cooperative and non-cooperative solutions. Homo Oeconomicus 34, 137–164. doi: 10.1007/s41412-017-0038-3
Rigaud, K. K., De Sherbinin, D., Jones, A., Bergmann, B., Clement, J., Ober, V., et al. (2018). Groundswell: Preparing for Internal Climate Migration. Washington, DC: World Bank.
Robinson, C., Dilkina, B., and Moreno-Cruz, J. (2020). Modeling migration patterns in the USA under sea level rise. Plos ONE 15, e0227436. doi: 10.1371/journal.pone.0227436
Schewel, K. (2020). Understanding immobility: Moving beyond the mobility bias in migration studies. Int. Migr. Rev. 54, 328–355. doi: 10.1177/0197918319831952
Schewel, K., and Asmamaw, L. B. (2021). Migration and development in Ethiopia: exploring the mechanisms behind an emerging mobility transition. Migration Stu. 9, 1673–1707. doi: 10.1093/migration/mnab036
Shryock, H. S., Siegel, J. S., and Larmon, E. A. (1975). The Methods and Materials of Demography, Vol. 2. Silver Hill, MD: US Bureau of the Census.
Simini, F., González, M. C., Maritan, A., and Barabási, A. L. (2012). A universal model for mobility and migration patterns. Nature 484, 96–100. doi: 10.1038/nature10856
Singh, R. (2022). Origin of World's Largest Migrant Population, India Seeks to Leverage Immigration. Washington, DC: Migration Policy Institute. Available online at: https://www.migrationpolicy.org/article/india-migration-country-profile
Smirnov, O., Lahav, G., Orbell, J., Zhang, M., and Xiao, T. (2023). Climate change, drought, and potential environmental migration flows under different policy scenarios. Int. Migr. Rev. 57, 36–67. doi: 10.1177/01979183221079850
Sofuoglu, E., and Ay, A. (2020). The relationship between climate change and political instability: the case of MENA countries (1985, 01–2016. 12). Environ. Sci. Pollut. Res. 27, 14033–14043. doi: 10.1007/s11356-020-07937-8
Speelman, L. H., Nicholls, R. J., and De Safra Campos, R. (2021). The role of migration and demographic change in small island futures. Asian Pacific Migr. J. 30, 282–311. doi: 10.1177/01171968211044082
Thober, J., Schwarz, N., and Hermans, K. (2018). Agent-based modeling of environment-migration linkages. Ecol. Soc. 23, 22–68. doi: 10.5751/ES-10200-230241
Van Praag, L. (2021). Can I move or can I stay? Applying a life course perspective on immobility when facing gradual environmental changes in Morocco. Climate Risk Manage. 31, 100274. doi: 10.1016/j.crm.2021.100274
Vezzoli, S., Bonfiglio, A., and De Haas, H. (2017). Global Migration Futures: Exploring the Future of International Migration With a Scenario Methodology. Oxford: International Migration Institute.
Vollset, S. E., Goren, E., Yuan, C. W., Cao, J., Smith, A. E., Hsiao, T., et al. (2020). Fertility, mortality, migration, and population scenarios for 195 countries and territories from 2017 to 2100: a forecasting analysis for the global burden of disease study. The Lancet 396, 1285–1306. doi: 10.1016/S0140-6736(20)30677-2
Wiegel, H., Warner, J., Boas, I., and Lamers, M. (2021). Safe from what? Understanding environmental non-migration in Chilean Patagonia through ontological security and risk perceptions. Reg. Environ. Change 21, 43. doi: 10.1007/s10113-021-01765-3
Wiśniowski, A., Smith, P. W., Bijak, J., Raymer, J., and Forster, J. J. (2015). Bayesian population forecasting: extending the Lee-Carter method. Demography 52, 1035–1059. doi: 10.1007/s13524-015-0389-y
World Bank (2017). World Development Indicators. World Bank. Available online at: http://data.worldbank.org/datacatalog/world-development-indicators.
Zelinsky, W. (1971). The hypothesis of the mobility transition. Geographical Review 61, 219–249. doi: 10.2307/213996
Zickgraf, C. (2021). Theorizing (Im)mobility in the face of environmental change. Reg. Environ. Change 21, 126. doi: 10.1007/s10113-021-01839-2
Keywords: climate, migration, forecasting, climate mobility, models, scenarios
Citation: Schewel K, Dickerson S, Madson B and Nagle Alverio G (2024) How well can we predict climate migration? A review of forecasting models. Front. Clim. 5:1189125. doi: 10.3389/fclim.2023.1189125
Received: 18 March 2023; Accepted: 17 November 2023;
Published: 04 January 2024.
Edited by:
Andrea Milan, International Organization for Migration, SwitzerlandReviewed by:
Kelsea Best, The Ohio State University, United StatesCopyright © 2024 Schewel, Dickerson, Madson and Nagle Alverio. This is an open-access article distributed under the terms of the Creative Commons Attribution License (CC BY). The use, distribution or reproduction in other forums is permitted, provided the original author(s) and the copyright owner(s) are credited and that the original publication in this journal is cited, in accordance with accepted academic practice. No use, distribution or reproduction is permitted which does not comply with these terms.
*Correspondence: Kerilyn Schewel, a2VyaWx5bi5zY2hld2VsQGR1a2UuZWR1
Disclaimer: All claims expressed in this article are solely those of the authors and do not necessarily represent those of their affiliated organizations, or those of the publisher, the editors and the reviewers. Any product that may be evaluated in this article or claim that may be made by its manufacturer is not guaranteed or endorsed by the publisher.
Research integrity at Frontiers
Learn more about the work of our research integrity team to safeguard the quality of each article we publish.