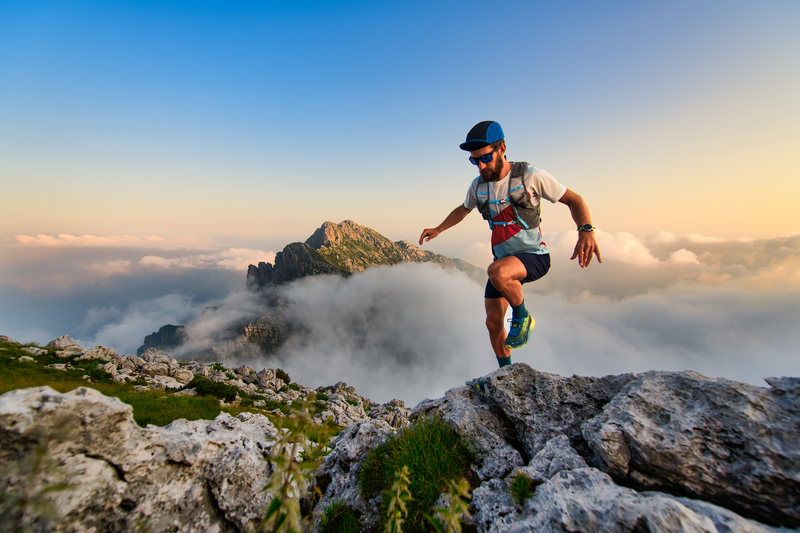
94% of researchers rate our articles as excellent or good
Learn more about the work of our research integrity team to safeguard the quality of each article we publish.
Find out more
ORIGINAL RESEARCH article
Front. Clim. , 20 October 2022
Sec. Climate Adaptation
Volume 4 - 2022 | https://doi.org/10.3389/fclim.2022.947308
This article is part of the Research Topic The Effects of Deforestation: its Role on Climate Change and Impact on Ecosystems View all 4 articles
Adaptation reduces the harmful effects of climate change. Nonetheless, the process of adaptation is associated with costs. If these costs are not managed accordingly, they have the potential to deter adaptation thereby impacting on its sustainability. Estimating adaptation costs to climate change provides vital information for decision-making among resource constrained smallholder maize farmers and enhances sustainable adaptation. However, there is no method for estimating adaptation costs that caters for the needs of smallholder maize farmers. Therefore, it was imperative to develop a context-specific tool for estimating adaptation costs for smallholder maize farmers using a bottom-up approach. Principles of quantification of theoretical constructs including conceptualization, operationalisation and attribute development were adopted in developing the tool for estimating adaptation costs to climate change. The tool is composed of three evaluation tools and three adaptation costs equations for the pre-, during- and post-adaptation phases, a total adaptation costs equation and a Summated Rating Scale for sustainability of adaptation activities. The tool is user-friendly, relatively easy to use, can be interpreted easily and modified to suit different smallholder farmers. It is hoped that the tool will assist smallholder maize farmers to consider all factors of critical importance before implementing adaptation plans and ensure reduced adaptation costs while enhancing sustainability.
It is widely acknowledged that adaptation reduces the harmful effects of climate change. Although this may be the case, the process of adaptation is dynamic, complex and multifaceted in nature. This is because it occurs in technical, biophysical, psychological and social dimensions. Some scholars (Batie, 2008; Levin et al., 2012) have branded processes such as adaptation to climate change “wicked problems” because they are difficult to comprehend. It has also been established that despite the many positive outcomes brought forward through adaptation, it has a “dark side”. Kates (2000) noted that adaptation creates winners and losers thereby worsening inequalities between different groups in societies for example, the rich and the poor. Barnett and O'neill (2010) observed the social injustices among vulnerable groups in society and the diminished environmental integrity brought forward by adaptation. Kori (2021) concurred and noted that adaptation to climate change is associated with costs that may be financial or non-financial, direct or indirect, tangible or intangible. In any of these cases, if these costs are not managed accordingly, they may be detrimental to the sustainability of adaptation especially in communities that survive on climate dependent livelihoods such as farming. The costs may also deter efforts to implement adaptation measures among communities willing to adapt. Such situations have a bearing on sustainable adaptation. Therefore, it is important for smallholder farmers to estimate the costs of adaptation before implementing adaptation plans to strategize accordingly and enhance sustainable adaptation.
Lim et al. (2005) reported that sustainable climate change adaptation supports sustainable development. This could be one of the reasons why the Sustainable Development Goals (SDGs) of the United Nations gave high priority to climate change issues with SDG 13 specifically targeting to combat climate change and its impacts. One of the ways in which this is supposed to be achieved is through strengthening resilience and adaptive capacity in all countries. In the same vein, Lim et al. (2005) emphasized that to promote sustainable adaptation, focus should be on practice rather than theory where strategies for climate change adaptation should be integrated into sustainable development plans. As postulated, climate change and sustainability should not be treated in isolation.
Several calls have been made to mainstream adaptation in the context of sustainable development. However, no subsequent actions are yet to be implemented as Bhatasara and Nyamwanza (2018) noted that climate change research in general, still lacks the sustainability dimension. The same authors specifically noted the narrow conceptualization of sustainability in adaptation research in Africa, yet the region is highly susceptible to climate change. Pachauri et al. (2014) reported that climate change will slow down economic growth, erode food security and exacerbate poverty in Africa. This calls for African communities especially those that rely on climate sensitive livelihoods like farming to adapt to climate change in a sustainable manner.
Estimating the costs associated with adaptation could be one of the ways that can ensure sustainable adaptation. It is envisaged that estimating the costs associated with adaptation will ensure sustainable adaptation through providing vital information for resource constrained smallholder farmers that will enhance informed decision-making. Apart from that, smallholder farmers will be equipped with information that will enable them to consider all factors of critical importance before implementing adaptation plans. However, there is no context-specific method for estimating adaptation cost to climate change that also enhance sustainability particularly for smallholder maize farmers. Traditional approaches that have been used in estimating adaptation costs such as the Cost Benefit Analysis (CBA), Cost Effective Analysis (CEA), and Multi-Criteria Analysis (MCA) among others do not give a deeper understanding of the cost involved prior to, during and post adaptation phases especially for individual smallholder farmers. Furthermore, the methods do not consider potential interrelationships between various adaptation cost variables. These valuation methods only provide support to help select among numerous possible adaptation options but do not go further to establish what happens thereafter which may have a strong bearing on sustainable adaptation.
The valuation methods also do not fully consider the non-economic and non-market costs such as the social, psychological and environmental effects of adaptation as they are difficult to quantify and attach reliable monetary values (Fankhauser and Dietz, 2014). For example, CBA Allows for comparison between costs and benefits of adaptation strategies using a common metric normally valued in monetary terms. However, not all costs and benefits of adaptation can be valued in monetary terms. This leads to exclusion of relevant and important aspects that need to be considered to ensure sustainable adaptation. The United Nations Framework for Climate Change Convention (UNFCCC, 2011) observed that valuation of adaptation costs using monetary terms is problematic and has led to considerable disagreements among economists about the discount rates at which future costs should be discounted. This leads to discrepancies and inconsistences in results.
The traditional methods used in valuing adaptation strategies assume a stable climate for example, CEA. As such, they ignore small but frequent events that can affect agricultural production (Meyer et al., 2013). Therefore, the approaches do not explicitly deal with realities of climatic variations and changes that smallholder farmers are normally confronted with. It is also important to note that the bulk of research on adaptation cost to climate variability and change have been extensively at national and international levels. For example, the CBA is commonly used for government planning by experienced and professional decision makers at a macro level for big projects (Birol et al., 2010).
The top-down approach therefore, often yields results that do not offer a direct operational relevance when applied to individual farm levels. Apart from that, existing valuation approaches involve the use of powerful, complicated tools and techniques. This raises the question of expertise in terms of usage among smallholder farmers who are the main recipients and end-users. Individual smallholder maize farmers may not be able to apply complex valuation approaches in their own capacities and to their own circumstances. For example, the use of CBA at individual farm level requires downscaling impact assessments to local levels (Sain et al., 2017). This requires certain levels of skill and training which most of the smallholder farmers do not have hence only experienced practitioners are able to use it.
The limitations of the traditional valuation approaches have been recognized by decision makers, policy makers and governments. This has seen the development of alternative adaptation decision making approaches such as the Robust Decision-Making Approaches. However, the methods are still relatively new in the academic and policy platforms and only a few applications exist (Hallegatte et al., 2012). It has also been noted that decision makers are still unfamiliar with the alternative approaches as it takes time to familiarize new methods hence the use of traditional approaches is still prevalent (Dittrich et al., 2016). Despite all the efforts put forward in coming up with improved valuation methods a gap still remains that they are top-down approaches.
The above narrative indicate that existing valuation approaches have a fair share of weaknesses. None of them provides a single best method for adaptation cost appraisals that enhances sustainable adaptation for smallholder farmers. It is within this context that this study was conceived. The aim was to develop a tool to estimate the costs associated with specific adaptation measures for smallholder maize farmers. This was achieved through conducting a case study of an African country, Zimbabwe, with high levels of poverty, low levels of development and a high dependency on climate sensitive livelihoods and low adaptive capacity. In addition to that, the study also aimed to respond to the observations noted in the preceding paragraphs and sought to develop a context-specific and simplified tool for estimating adaptation cost to climate change that offers a direct operational relevance to smallholder maize farmers. This was done through a series of steps. First, adaptation cost elements were established. Secondly, cost categories and variables were conceptualized and operationalized according to smallholder maize farmers' narratives. As a final step, a context-specific tool for estimating adaptation cost to climate change for smallholder maize farmers was developed. This study contributes to the sustainable adaptation debate through providing a sustainability indicator as a tool for adaptation assessment and decision-making for smallholder maize farmers.
An integrated approach was adopted for the research. In this section, an outline of the steps taken in developing the tool for estimating adaptation cost to climate change is given. A conceptual framework in which this study is embedded is given first. This is followed by a description of the study area and the reasons why it was considered suitable for the study are explained. An explanation of the research design and sampling procedure that came up with the sample of the study follows thereafter. This is followed by the data collection and analysis methods, tools and techniques. Details of how trustworthiness and quality of research were built close this section.
This study is based on the argument that, while existing climate change adaptation perspectives situate sustainability within the confines of development, they have been less forthcoming in providing the necessary tools that can enhance sustainability. To address this gap, this study aimed to develop a tool for estimating adaptation costs for smallholder maize farmers. While acknowledging that the terms sustainability and adaptation are interwoven in many ways, in this study these terms have been merged and contextualized to suit the smallholder maize farmers scenarios and borrowed insights from Chikozho (2010) and the Pachauri et al. (2014) to define the term. As such, sustainable adaptation refers to processes executed by smallholder maize farmers to adjust, moderate, better cope, or manage the impact of climate change while ensuring that the actions do not jeopardize such efforts in the future. While maintaining this definition, it is noted that the processes often used to manage the impact of climate change are associated with challenges, burdens, conflicts and contradictions that unfold prior to, during and after implementation of adaptation plans. At this point, the traditional adaptation process is invoked. It should be noted that it is divided into 3 main stages. These are the pre-, during- and post-adaptation stages.
The pre-adaptation stage corresponds to the assessment and planning stages of the traditional adaptation process. The during-adaptation stage correspond to the implementation stage and the post-adaptation stage to the monitoring and evaluation stage. It is deduced that during the pre-adaptation phase, costs that affect the sustainable adaptation arise from the negative impact of climate change on maize farming. During-adaptation stage, costs that affect sustainable adaptation arise from implementing adaptation measures. Post-adaptation, costs arise from the effects of adaptation measures on implementing and/or external actors as well as the environment. It is envisaged that adaptation costs encountered during each stage deter adaptation efforts and hence affect sustainable adaptation.
The idea posited by Eriksen and O'brien (2007) that strengthening adaptive capacity enhances sustainable climate adaptation was borrowed to strengthen the conceptualization. In this case, adaptive capacity can be strengthened through providing appropriate tools that facilitate informed decision-making for smallholder farmers. Tools that enable them to consider all factors of critical importance taking into account their own circumstances in terms of availability of resources, when to adapt and how to adapt are necessary.
The study was conducted in Chirumanzu District located in the Midlands Province of Zimbabwe. The area lies in Natural Region III with some parts falling under Natural Region IV (Kapungu, 2013). Natural Region III and IV are semi-intensive and semi-extensive farming regions, respectively. Although both regions are suitable for livestock production, smallholder agriculture is more inclined toward crop production with maize being one of the most produced crops. The two Natural Regions III and IV in which Chirumanzu lies, are prone to climatic variations and changes. The regions receive rainfall ranging from 500–750 to 400–510 mm per annum, respectively (Musara et al., 2011). The area is subject to extreme temperature, severe mid-season dry spells and frequent seasonal droughts (Simba and Chayangira, 2017), yet rain fed agriculture is the major source of livelihood.
Chirumanzu District has 25 wards. Wards are administrative divisions for election purposes normally represented by a councilor. Eight wards (11, 12, 15, 16, 18, 19 and 20) benefited from both the old resettlement programme of the 1980s and the Fast Track Land Reform Programme of 2000. This research focussed on smallholder maize farmers who benefited from the resettlement programme who are making efforts to adapt to climate change. The farmers have adapted through several ways including conservation farming, diversification, changing planting dates, irrigation and preserving soil biodiversity (Kori et al., 2020). Further research has proven that these farmers encounter several problems, dangers and challenges (considered costs) while adapting to climate variability and change (Kori, 2021). Such a community subject to climate variations, changes and extreme events, with farmers making efforts to adapt and attesting to experiencing adaptation costs provided a suitable area for the study. Figure 1 shows the map of Chirumanzu and the location of the wards selected for the study.
Figure 1. Map of Chirumanzu, Zimbabwe showing the location of the study area. Source: Author's graphical representation of the study area.
A sequential exploratory mixed method design (Creswell et al., 2003; Cresswell and Plano Clark, 2011) was adopted for this research. Data collection and analysis was conducted in stages. The initial phase constituted qualitative data collection and analysis forming the core component (Schoonenboom and Johnson, 2017). Results of the qualitative phase then informed the second phase which constituted the supplementary component (Schoonenboom and Johnson, 2017). In Morse (2016) notation system the design is denoted:
signifying that more weight is attached to the core qualitative component (written in capital letters) while the quantitative component (written in small letters) only supplemented it. Figure 2 shows a diagram that further details the research design.
The population of the study was all the smallholder maize farmers in Chirumanzu resettlement areas. Sampling was done in two stages to come up with a representative sample. The first stage aimed at identifying wards to work in. Heterogeneous or maximum variation purposive sampling (Etikan et al., 2016) was conducted. The selection criterion was dominant soil types in Chirumanzu. Dominant soil types were considered specifically for three reasons. Firstly, soil type is one of the major factors that drive maize farming. Secondly, different soil types behave differently to variations and changes in climate. Thirdly, soil types determine how the farmer will respond to the impacts of climate variations and changes. It was acknowledged that soil types may overlap across wards however, consideration was given to the dominant soil type covering at least 80 % of the ward. Verification and confirmation of soil types was also done at individual farm level during the interviews. Four dominant soil types were discovered from the nine wards that benefited from the resettlement programme. These are sandy, clay, shallow-sodic and sandy loam. This led to a selection of four representative wards. Ward 11 was selected to represent sandy loam soils. Ward 12 was selected to represent shallow-sodic soils. Ward 15 was selected to represent clay soils. Ward 20 was selected to represent deep sandy soils.
The second stage involved identification and selection of maize farmers to interview. Homogenous purposive sampling was used (Etikan et al., 2016). The selection criterion was farmers who had experienced variations and changes in climate and adopted adaptation measures. Apart from that, farmers were supposed to be producing high yields consistently, always had enough maize grain to feed their families and had extra to sell. While acknowledging that there are other factors that influence adaptation, in this study, it was assumed that performance was a result of adoption of adaptation measures while all other factors were held constant. This was informed by the need to establish the sustainability of successful adaptation in developing the framework for estimating adaptation cost. The total sample size was informed by data saturation. Data saturation was reached mostly between the 8th and 9th farmer throughout the four wards. After the 8th and 9th farmers no new information was gathered however, interviews were continued up to the 15th farmer in wards 11, 12 and 20 in case more insightful data arises (Peterson, 2019). However, in ward 15, interviews were stopped at the 9th farmer due to commotion that erupted during data collection. Therefore, the total sample size was 54 farmers. Figure 3 shows the sampling procedure.
The main data collection tool was a semi-structured interview guide. The interview guide contained open-ended questions regarding the problems, dangers and challenges encountered by smallholder maize farmers (adaptation cost elements) during the three phases of adaptation (pre-, during-, post-). The data enabled the researcher to gather perspectives and understanding of the costs associated with adaptation to climate change among smallholder maize farmers. This data was then used in developing the tool for estimating adaptation cost through a series of steps as explained below.
Step 1: Clustering and categorizing adaptation cost elements
The cost elements were first clustered and categorized into homogenous groups. This was done by identifying common themes in qualitative data collected on problems, dangers and challenges encountered by smallholder maize farmers to establish relationships between cost elements. This yielded cost variables and categories of adaptation.
Step 2: Conceptualization
The adaptation cost variables and categories were defined first (conceptualization) in the context of the study considering the primary data collected on the adaptation cost elements and variables. Conceptualization provided an opportunity to understand what would be included and excluded in the definitions. In defining the cost variables one major question was asked: How does the cost variable manifest as specified by maize farmers? Answering this question was key to determining a contexualised definition of the cost variables. Reference was given to the qualitative data collected, particularly direct quotations on adaptation cost elements by farmers. The definitions provided by the conceptualization process clarifies the operational meanings of cost variables that underpin the concept of adaptation cost to climate change in this paper. Definitions of the cost variables are therefore context-specific. Although the definitions of adaptation cost variables established in this research may differ from regular definitions given in literature, they offer a direct operational relevance to smallholder maize farmers in Chirumanzu and may be extended to other smallholder farmers elsewhere.
Step 3: Operationalisation
Means of measuring the cost variables were formulated (operationalisation). Unobservable cost variables were linked with observable indicators (Leggett, 2011) mentioned by smallholder maize farmers. In other words, indicators were developed making reference to qualitative data on the adaptation cost elements in order to ensure conformity to maize farmers' experiences. The process helped answer two basic questions: What is being measured?, How is it being measured?
Step 4: Attribute development
Attributes were developed for the established indicators. Attributes were treated as changeable characteristics which define a cost variable (Markus and Borsboom, 2013). The approach involved continuously reviewing qualitative data on the cost elements with the aim of identifying major themes until a basic structure emerged for the cost variables. The basic structure laid the foundations for generic attribute development. Since attributes had changeable characteristics, values were often attached to represent the variations. Therefore, qualitative (non-numeric) values of attributes were established however, they were represented quantitatively (numerically). While the underlying cost variables remained qualitative, the numerical numbers represented the respondents' personal evaluation regarding the cost variables. Evaluation and frequency attributes (Spector, 1992) were developed for the cost variables. Evaluation attributes established how maize farmers would rate adaptation cost experiences. Frequency attributes established how often maize farmers experienced a specific adaptation cost. Simple worded statements were used while developing attributes. The number of attribute choices was determined by the nature of the data collected and substantiated with literature. Frequency attribute choices were ordered along a measurement continuum of low occurrence to high occurrence and numbers were assigned to each choice. Evaluation attribute choices differed from one indicator to another since the levels of measurement varied from one indicator to the next. Attribute choices indicated the extent to which adaptation cost variables are encountered or experienced.
Step 5: Assigning levels of measurement
Features of the traditional Likert scale (Likert, 1932) were borrowed to assign levels of measurement for each attribute choice. Levels of measurement were assigned for each attribute choice and these were ranked on a scale of 0 to 5 resulting in a 6-point unipolar scale (Spector, 1992). It was acknowledged that farmers may not encounter all the adaptation costs thus an even number of attribute choices with five scale points in the response set, with an additional scale point of zero signifying never having experienced an indicator was adopted.
Both positive and negative verbal attribute choices were used with closed range (Schwarz et al., 1985) to avoid vague quantifier verbal labels which can be interpreted in different ways. Positive attribute choices assume normal scaling while negative attribute choices assume reversed scaling. Hence in a reversed scale, 5 = 0, 4 = 1, 3 = 2, 2 = 3, 1 = 4 and 0 = 5. The numbers reflect that each level is proportionally greater or smaller than the level before it. This would help answer the question; What score can be assigned by different maize farmers to the levels of attribute choices determining adaptation cost. The total score will give the overall weight of individual farmer's cost of adaptation.
To estimate the adaptation cost for an individual farmer, a mean score will be calculated by summing up the pre-, during- and post-adaptation scores. The total score will be divided by the total number of indicators to come up with a mean adaptation cost that falls within the 0–5 range.
Where;
The number of indicators = No. of cost variables
Pre-adaptation costs = the mean score of cost incurred before implementation of adaptation plans
During-adaptation costs = the mean score of costs incurred while implementing adaptation plans
Post-adaptation costs = the mean score of costs incurred as a result of implementing adaptation plans
A mean sum of one signifies very low adaptation cost. A mean sum of two signifies low adaptation cost. A mean sum of three signifies average adaptation cost. A mean sum of four signifies high adaptation cost. A mean sum of five signifies very high adaptation cost.
This research relied much on the initial phase constituting the qualitative component. Therefore, it was important to establish trustworthiness to enhance credibility and reduce bias. Trustworthiness was enhanced through building credibility (Lincoln and Guba, 1985). Credibility was established through identifying irregular and/ or contrary cases that were unique to one respondent which is in line with Burnard et al. (2008) approach. This also ensured that response bias was reduced through inclusion of these outliers ensuring that less common responses were not left out. Furthermore, this ensured that there was no researcher interference or alteration of the perception of data and insights offered.
Table 1 shows the adaptation cost elements for the three stages of the adaptation process and a brief description of each to further explain the adaptation cost elements. In total 20 cost elements were established. Seven cost categories and 29 cost variables were deduced from the list of adaptation cost elements. Two cost categories and ten cost variables were deduced from the pre-adaptation phase. Three cost categories and 13 cost variables were deduced from during-adaptation phase. Two cost categories and six cost variables were deduced from post-adaptation phase.
Definitions of cost variables based in the context of this study are presented in Tables 2–4. The cost variables are defined as specified by maize farmers during the interviews. Established indicators for cost variables are also presented. These specify what is being measured by the cost variables and how it is measured. Established indicators are reflective and unidimensional in nature.
Attribute choices for the pre-adaptation phase are all evaluative in nature. They evaluate how maize farmers rate adaptation costs. Table 5 shows an evaluation tool for pre-adaptation phase. Attribute choices for four of the pre-adaptation indicators (marked with two stars in Table 5) assume reversed scaling while the rest take up normal scaling. Table 6 presents an evaluation tool for during-adaptation phase. Out of the 13 indicators, nine attribute choices were frequency response choices with close-range quantifiers for each of the six fixed reference points. Ratio quantifier labels specifying the number of times an adaptation cost was encountered were used. Only four indicators, the extent of unintended damage to arable land, the amount of risk posed to the maize enterprise, amount of extra money spent on additional inputs required and amount of decline in yield related to timing are evaluative response choices. Out of the 13 indicators for during-adaptation phase, attribute choices for only one indicator assume reversed scaling while the rest assumes normal scaling. Table 7 shows an evaluation tool for post-adaptation phase. Attribute choices for the post-adaptation phase are all frequency response choices. Likewise, for each indicator, close-ranged ratio-quantifier labels were assigned to the attribute choices. All the attribute choices for the post-adaptation phase take up normal scaling.
The procedure for calculating total adaptation cost is shown below:
Where;
Total adaptation cost = The overall cost for the three phases of adaptation
Pre-adaptation cost = The mean score of cost incurred before implementation of adaptation plans as calculated using Formula 1 below.
During-adaptation cost = The mean score of costs incurred while implementing adaptation plans as calculated using Formula 2 below.
Post-adaptation = The mean score of costs incurred as a result of implementing adaptation plans as calculated using Formula 3 below.
Since ten variables were identified for pre-adaptation phase and defined in Table 2. Therefore, pre-adaptation cost will be calculated as:
Since 13 variables were identified for the during-adaptation phase and defined in Table 3. Therefore, during-adaptation, cost will be calculated as:
Since 6 variables were identified for post-adaptation phase and defined in Table 4. Therefore, the post-adaptation cost will be calculated as:
The summated rating scale shown in Figure 4 is in the form of a color ramp. It depicts adaptation cost level for individual farmers as well as sustainability of adaptation activities on a six-point Likert scale.
Green represents zero, no adaptation cost hence extremely sustainable adaptation activities. Light green represents 1 signifying very low adaptation cost hence sustainable adaptation activities. Light brown represents 2, signifying low adaptation cost hence moderately sustainable adaptation activities. Orange represents 3, which signify high adaptation cost hence moderately unsustainable adaptation activities. Red represents 4 indicating very high adaptation cost hence unsustainable adaptation activities. Dark red represents 5 indicating extremely high adaptation cost hence extremely unsustainable adaptation activities. Sustainability of adaptation activities decreases from zero to five hence a mean score of five represents extremely unsustainable adaptation activities while zero represents extremely sustainable adaptation activities.
Many factors and/or issues affect the ability of individual smallholder farmers to achieve sustainable adaptation (Mcneeley, 2017). In this study, focus was on the costs associated with adaptation that often deter adaptation efforts thereby affecting its sustainability. The two most important factors that establish sustainable adaptation according to Eriksen and Brown (2011) are the authority to make adaptation decisions and flexibility to adapt. This study aimed to establish a tool that would offer smallholder maize farmers that authority and flexibility to make decisions about when and how to adapt taking into consideration their own circumstances in ensuring that adaptation costs are kept at a minimum as much as possible to achieve sustainable adaptation. This section starts by discussing the adaptation cost elements, categories and variables. This is followed by a discussion on the definitions and indicators of adaptation costs. A discussion on the attribute choices, level of measurement and evaluation tools come thereafter. The section closes by discussing the adaptation cost calculations and the summated rating scale.
The adaptation cost elements established in this study both advances past empirical research and offers new and novel insights on the process of adaptation among smallholder farmers. In general findings illustrate that pre-adaptation cost elements triggers adaptation among smallholder maize farmers. It is during this stage that farmers realize that there is need to adapt. Pre-adaptation cost elements drives the cognitive and affective components of risk perception (Terpstra, 2011). The cognitive component focuses on farmers' perceived risk of climate variability and change as well as its impacts. The affective component focuses on farmers' feelings, thoughts and emotions toward climate variability and change events. According to the Protection Motivation Theory (Feng et al., 2017), the cognitive and affective components drive farmers toward performing threat appraisals. Threat appraisals are important in adaptation decision making because it is during this stage where farmers realize the need to adapt or not.
Some cost elements for the pre-adaptation phase established in this study suggest that climate change has a psychosocial impact (Grothmann and Patt, 2005) on smallholder maize farmers. The psychosocial impact has not been comprehensively researched possibly due to difficulties in attaching a measurement. For example, negative feelings generated by the impact of climate change on the maize enterprises. Such costs are equally important just like the financial costs and need to be included in adaptation cost appraisals to reflect the full cost of adaptation.
Some cost elements in the pre-adaptation phase demonstrate maladaptive behavior. According to the IPCC Fifth Assessment Report, maladaptation refers to actions or inactions that increases the risk of adverse climate-related outcomes, increases vulnerability to climate change, or diminishes welfare, now or in the future. This study established a form of maladaptation among smallholder maize farmers where they would live in aguish due to the impact of climate change and not do anything (inaction) about it, for example, passive feelings. However, this does not solve the problem of climate change. In the same vein, this study also established another form of maladaptation where smallholder farmers would rather contemplate leaving farming and go to find other means of survival (forceful feelings). In both cases explained, the actions and/or inactions of smallholder maize farmers increases risk and vulnerability of climate change both at the present and in the future thereby affecting sustainability of adaptation.
Some of the during-adaptation cost elements established in this study fall under barriers to climate change adaptation (Biesbroek et al., 2013; Eisenack et al., 2014). According to the IPCC Fifth Assessment Report, barriers to adaptation are described as factors that make it difficult to implement adaptation measures. Some scholars use the term limits to adaptation to refer to barriers of adaptation. In this study, some of the costs established suggest limits and/or barriers to adaptation. For example, high cost and scarcity of inputs required for adaptation a cost element established for during-adaptation stage can limit implementation of adaptation measures. Furthermore, high costs incurred and scarcity of inputs required for adaptation as a cost suggest a key form of maladaptation. According to Barnett and O'neill (2010) adaptation approaches may be maladaptive if their economic, social and environmental costs are higher than alternative adaptation approaches. In this case, there are some adaptation measures with relatively lower costs than others. For example, moisture conservation through mulching could be relatively cheaper because the method uses natural resources than other moisture conservation techniques like construction of retaining walls to reduce excess runoff and increase infiltration in the field (see Shah et al., 2012; Baig et al., 2013).
Post-adaptation cost elements established in the study advances literature on post-adaptation behavior among smallholder farmers. Post-adaptation cost elements established largely relate to socially related issues impacting vulnerable groups in society mainly children and women. Children were deprived of their right to education while women were excessively burdened with extra work. This suggest that adaptation may promote socio-economic inequalities (Barnett and O'neill, 2010) among children and women if not managed accordingly. This may result in disproportionately burdening the vulnerable thereby reinforcing social differentiation, which constitute unsustainable adaptation (Eriksen and O'brien, 2007).
The definition of adaptation cost is semantically vague in existing literature. As a result, conceptualisations vary making it difficult to make significant conclusions especially for individual smallholder farmers. This study defined adaptation cost in the context of smallholder maize farmers in Chirumanzu as the financial and non-financial challenges, problems, danger, burdens, conflicts and contradictions that unfold prior to, during and after implementation of adaptation plans. This paved way for the development of a local and context-specific adaptation cost framework for smallholder maize farmers.
The definitions provided by the conceptualization process clarifies the operational meanings of cost variables that underpin the concept of adaptation cost to climate variability and change in this paper. Although the definitions of adaptation cost variables established in this research may differ from regular definitions given in literature, they offer a direct operational relevance to smallholder maize farmers in Chirumanzu and may be extended to other smallholder farmers elsewhere. The definitions clearly state what should be included and excluded in measuring the adaptation cost variables associated with adaptation to climate variability and change among smallholder maize farmers. Thus, these definitions move adaptation cost research forward by submitting a possible solution to the dilemma of measuring non-financial, indirect and intangible costs of adaptation.
Indicators hypothesized as cost variables in the adaptation cost tool are reflective in nature (Bollen and Bauldry, 2011). This is because they fulfill the three main characteristics of reflective indicators. Firstly, the underlying latent constructs are existing (Borsboom et al., 2004) among smallholder maize farmers and were not formed. Secondly, the direction of causality is from the latent constructs to the items. Thirdly, dimensions have a common shared theme with interchangeable items. On this basis, the tool for estimating adaptation cost to climate variability and change is a reflective measurement tool (Abbasi et al., 2017). Treating indicators as reflective accorded the opportunity to avoid construct misidentification and misspecification while enhancing construct validity (Freeze and Raschke, 2007).
Some of the indicators for cost categories and variables established in the study are latent constructs. This means that they cannot be measured or observed directly. This corresponds to Fankhauser and Dietz (2014) who noted imprecise representations of non-economic costs of adaptation. However, the same authors noticed that there are measurable impacts associated with non-economic costs of adaptation that are observable in the market economy. As such, inferences were made from explanations given by smallholder maize farmers during the interviews to establish measurable and/ or observable impacts that led to the development of the definitions of the latent constructs.
The three evaluation tools are used to assess adaptation cost scores for the three main stages of adaptation. The levels of measurement assumes both normal and reverse scoring to enhance reliability of the adaptation cost tool by reducing response bias (Navarro-González et al., 2016) whereby personal factors affect score and validity of interpretations. Response bias can be either response set or response style (Sonderen et al., 2013). With the former, participants tend to choose desirable answers rather than the actual truth while with the later respondents have a tendency to respond without paying attention to the content (Suárez Álvarez et al., 2018). Hence, both normal and reverse keyed attribute choices were deliberately included to reduce response bias. It is hoped that this will stimulate farmers to think about their adaptation cost experiences before assigning scores thereby reducing response bias.
The three evaluation tools also have evaluative and frequency response choice responses (Spector, 1992). Both the evaluative and frequency response choices come from the theoretical concept that is intended to be measured (Decastellarnau, 2018). In this case, the evaluative and frequency response choices came from the underlying theoretical adaptation cost variables as specified by smallholder maize farmers during the interviews. This emphasizes how crucial it is to utilize the bottom-up approach as it enhances the suitability of the tool to the smallholder farmers who are the main recipients and end-users.
The adaptation cost calculations provide a basis for calculating the cost associated with adaptation for each of the three phases of adaptation. It was imperative that the formulae for the three phases of adaptation be normalized. Hence, the score for each adaptation phase should be divided by the total number of indicators for that phase so that the mean adaptation cost falls within the zero to five range. To get the total cost of adaptation, farmers need to sum up the mean adaptation cost scores for the three phases. Another normalization procedure is also required to maintain the total cost of adaptation within the zero to five range. As such, the sum of the adaptation cost scores for pre-, during- and post-adaptation phases will be divided by three.
The Summated Rating Scale for estimating adaptation cost is expressed on a continuum with verbal labels at different scale points. Although it has been proved that verbal labels increase cognitive effort through reading and trying to process all the available options (Kunz, 2015), it has been shown that acquiescence is higher with fully labeled scales (Eutsler and Lang, 2015). Labeling all the points on a scale has been found to have a positive impact on reliability of responses given (Menold et al., 2014).
A score of zero on the Summated Rating Scale for estimating adaptation cost implies that a farmer did not experience any of the 29 adaptation costs listed in the pre-, during- and post-adaptation evaluation tools. A mean score of zero is a theoretical representation as well as a justified consideration that a farmer may not experience any of the adaptation costs listed. A score of one implies that a farmer experienced very few adaptation costs. In quantitative terms, only 0.2 of the adaptation costs would be experienced. A score of two implies that a farmer experienced few adaptation costs, which is 0.4 in quantitative terms. A score of three implies that a farmer experienced 0.6 adaptation costs. A score of four implies that a farmer experienced 0.8 adaptation costs. A score of five implies a farmer experienced all the 29 adaptation costs listed in the evaluation tools.
The tool developed in this study offers a simplified way for adaptation cost appraisal at local levels. It forms the basis of positively evaluating adaptation costs among smallholder farmers leading to the establishment of perceived adaptation cost (Mitter et al., 2018). Since perceived adaptation cost is a sub-component of adaptation appraisal (Grothmann and Patt, 2005), it will positively influence adaptation intention and enhance adaptation decision-making among smallholder maize farmers.
The tool developed in this study evades the need for skills and training as it offers a simplified, user-friendly framework for estimating adaptation cost. The advantage of the approach is that it does not require quantitative and complex measurements of adaptation outcomes. Instead, it relies on maize farmers' adaptation cost experiences. Since different types of farmers adopt different adaptation plans and have different adaptive capacities, individual farmers would be able to estimate specific adaptation costs for their respective adaptation plans taking it to account available resources and adaptive capacities.
The tool may provide a solution to the challenge that most smallholder farmers have of keeping records. Many studies have proved that smallholder farmers do not keep records. For example, Gichohi (2020) found out that smallholder farmers maintain sketchy farm records that can barely inform important decisions neither do they have the ability to interpret farm records while making decisions. Therefore, this study provides an approach that relies on farmers' memory of events and experiences to enable farmers to make important adaptation decisions.
The approach used in this study emphasizes the need to desist from always attempting to quantify adaptation costs in financial terms which has been found challenging in past research. For example, this study proposed measuring labor related issues in non-financial terms. Labor related issues such as labor productivity and labor opportunity cost are difficult to calculate and has not been estimated with much accuracy using financial terms (Doss, 2018). As such, this study recommends that labor opportunity cost be measured in terms of the number of important activities children or members of the household forego to pave way for adaptation activities. This kind of measurement tries to speak to the cultural views of labor that do not consider other children's and women's tasks, responsibilities and rights as important.
This study stresses the need to consider non-economic, indirect and intangible costs of adaptation to comprehensively capture the cost associated with adaptation. Fankhauser and Dietz (2014) stresses that non-economic costs are more significant in developing countries than economic costs and the absence of a market price is one of the main reasons why assessing non-economic costs is challenging. Thomas et al. (2019) advised that the intangible cost of adaptation should be addressed. This study filled in the gap in existing knowledge by infusing non-economic costs of adaptation such as social, psychological and environmental costs that have not been fully considered in past research and provided a non-financial measurement criterion for the costs.
The costs associated with adaptation have the potential to limit its sustainability especially among resource constrained smallholder farmers. Smallholder farmers need to be equipped with the necessary tools to enhance adaptation decision making. This would ensure that they make informed decisions that improves the sustainability of adaptation plans. This study aimed to develop a tool for estimating adaptation cost to climate change that smallholder maize farmers can use while making adaptation decisions with a strong focus on sustainability of adaptation plans. The study outlines the activities that were conducted in coming up with a context-specific tool for enhancing sustainable adaptation among smallholder maize farmers. It is hoped that the tool will assist smallholder farmers to estimate their respective adaptation costs before implementing adaptation plans. In doing so, they will be able to make informed adaptation decisions that would enhance sustainable adaptation. The tool for estimating adaptation cost offer a simplified way of estimating adaptation cost using non-financial terms. It eases the challenging task of comprehending adaptation costs in quantitative terms. The evaluation tools, equations and the summated rated scale are structured in a simplified way devoid of complicated tools and techniques to enhance usability by the end-users, smallholder maize farmers. Overall, the study submits a possible solution to the dilemma of measuring adaptation costs. It is important to note that the framework developed in this study used data solicited from smallholder maize farmers in Chirumanzu District only. This means that the tool is context-specific. This raises issues of transferability. The tool should therefore be applied to other smallholder maize farmers with caution bearing in mind that there could be other costs that may have been omitted. In addition, some costs may not apply to areas other than Chirumanzu. Further research is therefore recommended to establish whether there are differences in adaptation cost experiences between smallholder maize farmers in Chirumanzu and smallholder farmers elsewhere. This would enable the establishment of similarities and differences across different farmers in different areas. This conforms with the need to test and validate the tool for estimating adaptation cost to climate variability and change developed in this study.
The original contributions presented in the study are included in the article/supplementary material, further inquiries can be directed to the corresponding author.
The studies involving human participants were reviewed and approved by University of Venda Ethics Committee. The patients/participants provided their written informed consent to participate in this study.
Data analysis and first draft: DS. Inputs, comments, scientific validity, writing of final manuscript, conceptualization, and data collection: DS and EK. Graphics: EK. Both authors have read and agreed to the published version of the manuscript.
The research was funded by the National Research Foundation (NRF), South Africa (Grant number 105215) and the University of Venda Research and Publication Committee.
The authors declare that the research was conducted in the absence of any commercial or financial relationships that could be construed as a potential conflict of interest.
All claims expressed in this article are solely those of the authors and do not necessarily represent those of their affiliated organizations, or those of the publisher, the editors and the reviewers. Any product that may be evaluated in this article, or claim that may be made by its manufacturer, is not guaranteed or endorsed by the publisher.
Abbasi, A. Z., Ting, D. H., and Hlavacs, H. (2017). Engagement in games: developing an instrument to measure consumer videogame engagement and its validation. Int. J. Comput. Games Technol. 2017, 7363925. doi: 10.1155/2017/7363925
Baig, M. B., Shahid, S. A., and Straquadine, G. S. (2013). Making rainfed agriculture sustainable through environmental friendly technologies in Pakistan: A review. Int. Soil Water Conserv. Res. 1, 36–52. doi: 10.1016/S2095-6339(15)30038-1
Barnett, J., and O'neill, S. (2010). Maladaptation. Global Environ. Change 20, 211–213. doi: 10.1016/j.gloenvcha.2009.11.004
Batie, S. S. (2008). Fellows address: wicked problems and applied economics. Am. J. Agric. Econ. 90, 1176–1191. doi: 10.1111/j.1467-8276.2008.01202.x
Bhatasara, S., and Nyamwanza, A. (2018). Sustainability: a missing dimension in climate change adaptation discourse in Africa? J. Integ. Environ. Sci. 15, 83–97. doi: 10.1080/1943815X.2018.1450766
Biesbroek, G. R., Klostermann, J. E., Termeer, C. J., and Kabat, P. (2013). On the nature of barriers to climate change adaptation. Reg. Environ. Change 13, 1119–1129. doi: 10.1007/s10113-013-0421-y
Birol, E., Koundouri, P., and Kountouris, Y. (2010). Assessing the economic viability of alternative water resources in water-scarce regions: combining economic valuation, cost-benefit analysis and discounting. Ecol. Econ. 69, 839–847. doi: 10.1016/j.ecolecon.2009.10.008
Bollen, K. A., and Bauldry, S. (2011). Three Cs in measurement models: causal indicators, composite indicators, and covariates. Psychol. Methods 16, 265. doi: 10.1037/a0024448
Borsboom, D., Mellenbergh, G. J., and Van Heerden, J. (2004). The concept of validity. Psychol. Rev. 111, 1061. doi: 10.1037/0033-295X.111.4.1061
Burnard, P., Gill, P., Stewart, K., Treasure, E., and Chadwick, B. (2008). Analysing and presenting qualitative data. Br. Dental J. 204, 429–432. doi: 10.1038/sj.bdj.2008.292
Chikozho, C. (2010). Applied social research and action priorities for adaptation to climate change and rainfall variability in the rainfed agricultural sector of Zimbabwe. Phys. Chem. Earth. 35, 780–790. doi: 10.1016/j.pce.2010.07.006
Cresswell, J. W., and Plano Clark, V. L. (2011). Designing and Conducting Mixed Method Research, 2nd Edn. Thousand Oaks, CA: Sage.
Creswell, J. W., Clark, V. P., Gutmann, M. L., and Hanson, W. E. (2003). “An expanded typology for classifying mixed methods research into designs,” in Handbook of Mixed Methods in Social and Behavioral Research, eds A. Tashakkori and C. Teddlie (Sage Publications).
Decastellarnau, A. (2018). A classification of response scale characteristics that affect data quality: a literature review. Qual. Quant. 52, 1523–1559. doi: 10.1007/s11135-017-0533-4
Dittrich, R., Wreford, A., and Moran, D. (2016). A survey of decision-making approaches for climate change adaptation: are robust methods the way forward? Ecol. Econ. 122, 79–89. doi: 10.1016/j.ecolecon.2015.12.006
Doss, C. R. (2018). Women and agricultural productivity: reframing the issues. Develop. Policy Rev. 36, 35–50. doi: 10.1111/dpr.12243
Eisenack, K., Moser, S. C., Hoffmann, E., Klein, R. J., Oberlack, C., Pechan, A., et al. (2014). Explaining and overcoming barriers to climate change adaptation. Nat. Clim. Change 4, 867–872. doi: 10.1038/nclimate2350
Eriksen, S., and Brown, K. (2011). Sustainable adaptation to climate change. Milton Park: Taylor and Francis. doi: 10.3763/cdev.2010.0064
Eriksen, S. H., and O'brien, K. (2007). Vulnerability, poverty and the need for sustainable adaptation measures. Clim. Policy 7, 337–352. doi: 10.1080/14693062.2007.9685660
Etikan, I., Musa, S. A., and Alkassim, R. S. (2016). Comparison of convenience sampling and purposive sampling. Am. J. Theor. Appl. Stat. 5, 1–4. doi: 10.11648/j.ajtas.20160501.11
Eutsler, J., and Lang, B. (2015). Rating scales in accounting research: the impact of scale points and labels. Behav. Res. Account. 27, 35–51. doi: 10.2308/bria-51219
Fankhauser, S., and Dietz, S. (2014). Noneconomic Losses in the Context of the UNFCCC Work Programme on Loss and Damage. Centre for Climate Change Economics and Policy and Grantham Research Institute on Climate Change and the Environment.
Feng, X., Liu, M., Huo, X., and Ma, W. (2017). What motivates farmers' adaptation to climate change? The case of apple farmers of Shaanxi in China. Sustainability. 9, 519. doi: 10.3390/su9040519
Freeze, R., and Raschke, R. L. (2007). “An assessment of formative and reflective constructs in IS research,” in Proceedings of the European Conference on Information Systems (St. Gallen: University of St Gallen).
Gichohi, P. M. (2020). The role of record keeping and maintenance in enhancing decision making among smallholder dairy farmers in gitugi ward In Murang'a County, Kenya. Inform. Develop. 36, 535–545. doi: 10.1177/0266666919879728
Grothmann, T., and Patt, A. (2005). Adaptive capacity and human cognition: the process of individual adaptation to climate change. Global Environ. Change 15, 199–213. doi: 10.1016/j.gloenvcha.2005.01.002
Hallegatte, S., Shah, A., Brown, C., Lempert, R., and Gill, S. (2012). Investment decision making under deep uncertainty: application to climate change. World Bank Policy Research Working Paper. doi: 10.1596/1813-9450-6193
Kapungu, S. T. (2013). A Study of Rural Women Farmers' Access to Markets in Chirumanzu. Stellenbosch: Stellenbosch University.
Kates, R. W. (2000). Cautionary tales: adaptation and the global poor. Clim. Change 45, 5–17. doi: 10.1023/A:1005672413880
Kori, D. (2021). Developing a framework for estimating adaptation cost to climate variability and change for maize farmers in resettlement areas of Chirumanzu District, Zimbabwe. Doctor of Philosophy In Rural Development, University Of Venda.
Kori, D. S., Francis, J., and Zuwarimwe, J. (2020). Adapting to the threat and impact of climate variability: realities of maize farmers in resettlement areas of Chirumanzu District Zimbabwe. J. Rural Stud. 79, 145–156. doi: 10.1016/j.jrurstud.2020.08.012
Kunz, T. (2015). Rating Scales in Web Surveys. A Test of New Drag-and-Drop Rating Procedures. Technische Universitat.
Leggett, A. (2011). Constructs, Variables, and Operationalization. Available online at: https://www.academia.edu
Levin, K., Cashore, B., Bernstein, S., and Auld, G. (2012). Overcoming the tragedy of super wicked problems: constraining our future selves to ameliorate global climate change. Policy Sci. 45, 123–152. doi: 10.1007/s11077-012-9151-0
Lim, B., Spanger-Siegfried, E., Burton, I., Malone, E., and Huq, S. (2005). Adaptation Policy Frameworks for Climate Change: Developing Strategies, Policies and Measures. Princeton, NJ: Citeseer.
Lincoln, Y., and Guba, E. (1985). Naturalistic Inquiry Sage: Newbury Park. New York, NY: Ca Google Scholar.
Markus, K. A., and Borsboom, D. (2013). Frontiers of Test Validity Theory: Measurement, Causation, and Meaning. New York, NY: Routledge. doi: 10.4324/9780203501207
Mcneeley, S. M. (2017). Sustainable climate change adaptation in Indian Country. Weather Clim. Soc. 9, 393–404. doi: 10.1175/WCAS-D-16-0121.1
Menold, N., Kaczmirek, L., Lenzner, T., and Neusar, A. (2014). How do respondents attend to verbal labels in rating scales? Field Methods 26, 21–39. doi: 10.1177/1525822X13508270
Meyer, V., Becker, N., Markantonis, V., Schwarze, R., Van Den Bergh, J. C., Bouwer, L. M., et al. (2013). Assessing the costs of natural hazards–state of the art and knowledge gaps. Nat. Hazards Earth Syst. Sci. 13, 1351–1373. doi: 10.5194/nhess-13-1351-2013
Mitter, H., Schönhart, M., Larcher, M., and Schmid, E. (2018). The stimuli-actions-effects-responses (saer)-framework for exploring perceived relationships between private and public climate change adaptation in agriculture. J. Environ. Manag. 209, 286–300. doi: 10.1016/j.jenvman.2017.12.063
Musara, J. P., Zivenge, E., Chagwiza, G., Chimvuramahwe, J., and Dube, P. (2011). Determinants of smallholder cotton contract farming participation in a recovering economy: empirical results from Patchway District, Zimbabwe. J. Sustain. Develop. Afr. 13, 1–12.
Navarro-González, D., Lorenzo-Seva, U., and Vigil-Colet, A. (2016). How response bias affects the factorial structure of personality self-reports. Psicothema 28, 465–470. doi: 10.7334/psicothema2016.113
Pachauri, R. K., Allen, M. R., Barros, V. R., Broome, J., Cramer, W., Christ, R., et al. (2014). Climate Change 2014: Synthesis Report. Contribution of Working Groups I, Ii and Iii to the Fifth Assessment Report of the Intergovernmental Panel On Climate Change. Geneva: IPCC.
Peterson, J. S. (2019). Presenting a qualitative study: a reviewer's perspective. Gift. Child Quart. 63, 147–158. doi: 10.1177/0016986219844789
Sain, G., Loboguerrero, A. M., Corner-Dolloff, C., Lizarazo, M., Nowak, A., Martínez-Barón, D., et al. (2017). Costs and benefits of climate-smart agriculture: the case of the dry corridor in guatemala. Agric. Syst. 151, 163–173. doi: 10.1016/j.agsy.2016.05.004
Schoonenboom, J., and Johnson, R. B. (2017). How to construct a mixed methods research design. KZfSS Kölner Zeitschrift für Soziologie und Sozialpsychologie. 69, 107–131.
Schwarz, N., Hippler, H.-J., Deutsch, B., and Strack, F. (1985). Response scales: effects of category range on reported behavior and comparative judgments. Public Opin. Quart. 49, 388–395.
Shah, H., Khan, M. A., Farooq, U., Akmal, N., Munir, S., and Hussain, B. (2012). Potential for investment in indigenous technologies: a case of low cost soil and water conservation structures in rainfed Pothwar, Pakistan. Pakis. J. Agri. Res. 25, 1–13.
Simba, F., and Chayangira, J. (2017). Rainfall seasons analysis as a guiding tool to smallholder farmers in the face of climate change in midlands in Zimbabwe. J. Earth Sci. Clim. Chang. 8, 392. doi: 10.4172/2157-7617.1000392
Sonderen, E. V., Sanderman, R., and Coyne, J. C. (2013). Ineffectiveness of reverse wording of questionnaire items: let's learn from cows in the rain. PLoS ONE 8, E68967. doi: 10.1371/annotation/af78b324-7b44-4f89-b932-e851fe04a8e5
Suárez Álvarez, J., Pedrosa, I., Lozano, L. M., García Cueto, E., Cuesta Izquierdo, M., and Muñiz Fernández, J. (2018). Using reversed items in likert scales: a questionable practice. Psicothema 30, 149–158. doi: 10.7334/psicothema2018.33
Terpstra, T. (2011). Emotions, trust, and perceived risk: Affective and cognitive routes to flood preparedness behavior. Risk. Anal. 31, 1658–1675. doi: 10.1111/j.1539-6924.2011.01616.x
Thomas, K., Hardy, R. D., Lazrus, H., Mendez, M., Orlove, B., Rivera-Collazo, I., et al. (2019). Explaining differential vulnerability to climate change: a social science review. Wiley Interdiscip. Rev. Clim. Change 10, E565. doi: 10.1002/wcc.565
Keywords: adaptation costs, adaptation process, maize, smallholder farmers, sustainable adaptation
Citation: Shoko Kori D and Kori E (2022) Towards sustainable adaptation: A tool for estimating adaptation costs to climate change for smallholder farmers. Front. Clim. 4:947308. doi: 10.3389/fclim.2022.947308
Received: 18 May 2022; Accepted: 26 September 2022;
Published: 20 October 2022.
Edited by:
Bao-Jie He, Chongqing University, ChinaReviewed by:
Hailong Wang, Sun Yat-sen University, ChinaCopyright © 2022 Shoko Kori and Kori. This is an open-access article distributed under the terms of the Creative Commons Attribution License (CC BY). The use, distribution or reproduction in other forums is permitted, provided the original author(s) and the copyright owner(s) are credited and that the original publication in this journal is cited, in accordance with accepted academic practice. No use, distribution or reproduction is permitted which does not comply with these terms.
*Correspondence: Dumisani Shoko Kori, ZF9zaG9rb0B5YWhvby5jb20=
Disclaimer: All claims expressed in this article are solely those of the authors and do not necessarily represent those of their affiliated organizations, or those of the publisher, the editors and the reviewers. Any product that may be evaluated in this article or claim that may be made by its manufacturer is not guaranteed or endorsed by the publisher.
Research integrity at Frontiers
Learn more about the work of our research integrity team to safeguard the quality of each article we publish.