- Department of Mechanical, Aerospace and Civil Engineering, Tyndall Centre, University of Manchester, Manchester, United Kingdom
This paper explores the implications of siting a bioenergy with carbon capture and storage (BECCS) facility to carbon emission performances for three case-study supply chains using the Carbon Navigation System (CNS) model. The three case-study supply chains are a wheat straw derived BECCS-power, a municipal solid waste derived BECCS-waste-to-energy and a sawmill residue derived BECCS-hydrogen. A BECCS facility needs to be carefully sited, taking into consideration its local low carbon infrastructure, available biomass and geography for successful deployment and achieving a favorable net-negative carbon balance. On average, across the three supply chains a 10 km shift in the siting of the BECCS facility results in an 8.6–13.1% increase in spatially explicit supply chain emissions. BECCS facilities producing low purity CO2 at high yields have lower spatial emissions when located within the industrial clusters, while those producing high purity CO2 at low yields perform better outside the clusters. A map is also generated identifying which of the three modeled supply chains delivers the lowest spatially explicit supply chain emission options for any given area of the UK at a 1 MtCO2/yr capture scale.
Introduction
Bioenergy with Carbon Capture and Storage (BECCS) will most likely play an important and critical role in limiting global temperature rise to 1.5 and 2.0°C and reach global net-zero targets by offsetting hard-to-abate residual emissions (IPCC, 2018; CCC, 2019). BECCS is the installation of carbon capture and storage (CCS) technology onto a bioenergy facility where the captured biogenic CO2 is transported for secure and “permanent” geological storage (Gough et al., 2018; Freer et al., 2021). BECCS as a form of carbon dioxide removal (CDR) has been projected to supply between 43.5 and 96.5 MtCO2/yr within the UK by 2050, depending on the assumed scenario within the committee on climate change sixth carbon budget (CCC, 2020a). BECCS is often projected to contribute the majority of negative emissions, with direct air capture and storage (DACS) and other CDR approaches providing the remainder (CCC, 2020a). The majority of nationally determined contributions do not specify what kind of BECCS will be used to create their negative emissions and often claim a generic form of BECCS will achieve their targets. BECCS is not a monolithic technology, and no two facilities are the same.
A realistic scale and footprint of an envisaged medium-sized BECCS supply chain situated in the Humber region of England, which is storing CO2 offshore in the Endurance saline aquifer is presented in Figure 1. The green lines depict the biomass routings, the blue lines depict the CO2 transportation to the Endurance field and the red line depicts hydrogen routing for national grid injection from the chosen facility site. The deployment of BECCS and other CDR approaches will play a critical role in meeting national Net-Zero commitments through the generation of negative emissions, but the roll out of the technologies has been slow, although has been accelerating in recent years with multiple large scale BECCS projects soon to come online (Norwegian Ministry of Petroleum Energy, 2016a,b; Brevik, 2017; Greenberg et al., 2017; Kemper, 2017; Occidental Petroleum Organisation, 2017; Global CCS Institute, 2018).
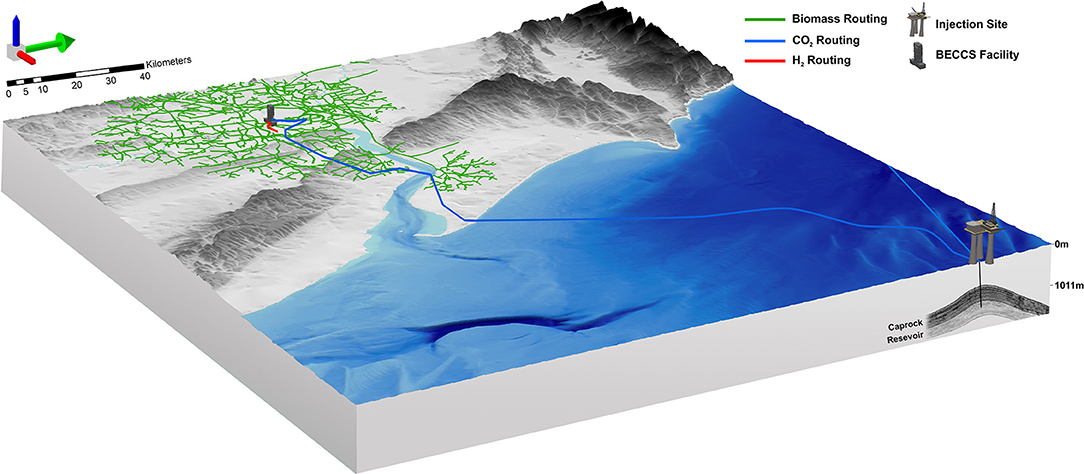
Figure 1. BECCS supply chain block diagram, depicting an envisaged 0.5 MtCO2/yr BECCS facility operating in the Humber region of England with geological storage of CO2 in the endurance reservoir via pipeline. The diagram is to scale in the horizontal dimension, a vertical exaggeration factor of 10 was applied to the vertical dimension.
The Committee on Climate Change Sixth Carbon Budget has developed six classifications for BECCS being BECCS-Power, BECCS-Waste-to-Energy, BECCS-Manufacturing/Construction, BECCS-Hydrogen, BECCS-Biofuels and BECCS-Biomethane, which is beneficial to predict the future deployment of the different types of BECCS (CCC, 2020b). However, these classifications are restrictive and do not reflect the full spectrum of the types of BECCS. These BECCS classifications fail to capture supply chains that straddle multiple classifications such as municipal solid waste (MSW) to hydrogen BECCS supply chains and does not reflect future BECCS types such as algae/aquatic biomass derived BECCS or new forms of BECCS like alkaline thermal treatment BECCS (Pour et al., 2018; Melara et al., 2020; Zhou and Park, 2020).
BECCS is an incredibly diverse and unique technology that needs to be carefully tailored to its local infrastructure, available biomass, economic environment, energy systems, land management strategies, socio-political context and geography for successful deployment and integration (Forster et al., 2020; Clery et al., 2021). A BECCS facility and supply chain that performs optimally in one location will not operate at the same level in another location (Freer et al., 2021).
This analysis presented here uses the Carbon Navigation System (CNS) model to simulate and evaluate the carbon performances of BECCS supply chains across the UK (Freer et al., 2021). The CNS was developed as a heuristic to aid policymakers and decision making with the sustainable and carbon-efficient deployment of BECCS across the UK. This paper describes the analysis of three BECCS supply chains across the UK, each calibrated to store 1 MtCO2/yr as this reflects the scale of upcoming large-scale BECCS facilities, generating carbon performance heatmaps depicting the variation in supply chain performance across different geographies, biomass distributions, access to geological storage and access to low carbon infrastructure. The heatmaps were generated for Scotland, England and Wales, while also focusing within five industrial carbon capture (ICCS) clusters across the UK. The paper will also determine the level of impact on the spatially explicit supply chain emissions by shifting the location of the BECCS facility across the UK. Spatially explicit supply chain emissions are the emissions that vary depending on the location of the energy facility; these include transportation and resource gathering. This paper also provides a comparative decision map depicting which of the three modeled BECCS supply chains should be chosen to store 1 MtCO2/yr for the least amount of spatially explicit supply chain emissions at any location in the UK.
The Carbon Navigation System Model
The CNS model, developed in Freer et al. (2021), is a carbon-efficient digital twin of the UK's industrial freight transport network for cargo and tanker transportation, which has been used to model BECCS supply chains in a macro-energy system analysis. A digital twin is a virtual representation of assets or networks that allows for the simulation of processes or interactions within a virtual version of the assets or networks to allow for system optimization or learning (Zhang et al., 2021). The CNS model simulates and calculates the spatial explicit supply chain emissions of specific BECCS supply chains to generate high spatial resolution carbon performance heatmaps for the UK. The CNS can search and route for any specific quantity of biomass to any location in the UK, route the produced CO2 to its most suitable geological storage site and route its energy output to its end-user, all while automatically switching between truck, rail, shipping and pipeline transportation to minimize CO2 emissions and calculate carbon optimal routings.
The CNS model's structure was designed so that it could be tailored and customized to analyze any form of BECCS. The model is split into multiple segments, called limbs, which focus on a specific aspect of a BECCS supply chain (Freer et al., 2021). The four limbs of the CNS model are the biomass limb which focuses on biomass transportation, the CO2 limb which focuses on the transportation and storage of CO2 via an ICCS cluster, the energy output limb which focuses on the transportation and distribution of the energy produced by the facility (including heat, power and biofuels), and the newly developed hydrogen limb to cover the transportation and distribution of hydrogen fuel across the UK. The hydrogen limb was added to the model due to the increasing potential role hydrogen will play in future energy systems, as the UK hydrogen strategy aims to generate 5 GW of low-carbon hydrogen by 2030 (HM Government, 2021). The four limbs all converge around the BECCS facility, and the structure of the CNS model is presented in Figure 2. The data used to build the model networks were extracted and georeferenced from National Infrastructure Planning documents and opensource transportation polylines published by the UK Government (BGS, 2020; HM Government, 2020a; HyNet, 2021b; Net Zero Teesside, 2021). The truck shapefiles were taken from the 2018 OS Open Roads dataset, the rail shapefiles were taken from the 2017 Freight Usage dataset and the shipping routes were taken from the 2016 MMO Anonymised AIS Derived Track Lines dataset (HM Government, 2020a). Within Freer et al., the methods in which the shapefiles were processed, refined, filtered and combined into multiple networks is explained in more detail (Freer et al., 2021).
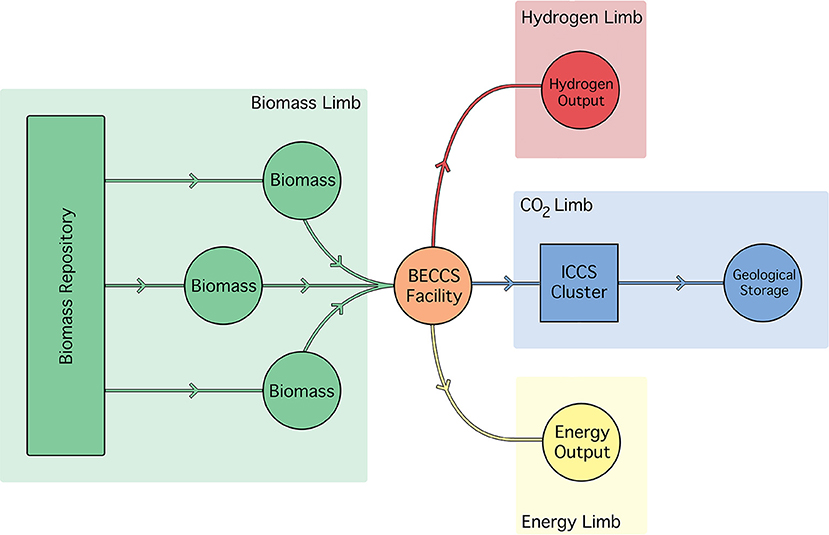
Figure 2. Carbon navigation system (CNS) model network key, biomass limb highlighted in green, hydrogen limb highlighted in red, CO2 limb highlighted in blue and energy output limb highlighted in yellow.
The scope of the CNS model focuses on the spatially explicit supply chain emissions produced by a BECCS supply chain, being the emissions that vary depending on where the facility is located. The spatially explicit emissions include the gathering of biomass, transportation of biomass, transportation of CO2, transportation of hydrogen fuel and the transportation of other energy outputs. The CNS model does not calculate the spatially static supply chain emissions such as biomass drying, biomass grinding, fuel combustion, CO2 compression, CO2 injection or CO2 remediation approaches as these are better calculated via a life-cycle analysis (LCA) (Laude et al., 2011; Pour et al., 2018; Yi et al., 2018; Bello et al., 2020; García-Freites et al., 2021; Lask et al., 2021). The scope of the CNS model has been specially designed to plug into any BECCS LCA to improve the spatial context of the analysis.
High Spatial Resolution Biomass Mapping
A high-resolution biomass repository was developed alongside the CNS model detailing the spatial distribution and quantities of various biomass types across the UK down to the plot of land resolution. Using the biomass repository, the CNS can accurately search and route specific quantities of biomass to any location in the UK. The biomass repository focuses on agricultural residues and industrial wastes but also includes many of the primary feedstocks used to generate the residues and wastes. A range of biomass remote sensing and mapping techniques were used to create the repository, including the main four mapping techniques below:
l. The visual assessment of land performed by farmers and experts (Newnham et al., 2010)
ll. Accounting of biomass as it is collected and transported (Ali et al., 2017)
lll. In-situ mechanical or electronic sensors collecting readings of biomass as it arrived at a transfer station of facility (Hakl et al., 2012)
lV. Spectral image analysis taken by satellite and drones (Jones et al., 2020)
Geospatial BECCS Analysis
The geospatial analysis of BECCS is a growing discipline within the literature as the deployment of the technology accelerates, and the spatial implications on BECCS supply chains need to be identified, evaluated and monitored. Many of the nations worldwide are banking on CDR approaches, predominantly afforestation and BECCS, to generate their negative emissions to offset their hard-to-abate sectors. However, many of these commitments have yet to declare where these CDR approaches will be sited and what are the land-use and operational footprints of these projects.
In particular, many LCAs and Techno-Economic Assessments (TEAs) for BECCS, bioenergy and bio-economy projects do not use realistic spatial data within their studies, where instead, they use simplified distances traveled and distributions of biomass in their study parameters (Laude et al., 2011; Pour et al., 2018; Yi et al., 2018; Bello et al., 2020; García-Freites et al., 2021; Lask et al., 2021). The poor siting of a BECCS facility relative to its biomass, CO2 infrastructure and energy end-users have severe emissions implications for the project (Freer et al., 2021) and will result in far-reaching fiscal, temporal, environmental and social knock-on effects. The spatial context needs to be better considered and incorporated into studies to avoid these emissions implications and knock-on effects.
The literature is now starting to consider the spatial implications of BECCS as the large-scale deployment of the technology nears. Figure 3 depicts which of the BECCS studies ranging from LCAs to TEAs and environmental assessments have considered the spatial implications of their projects, graded on the percentage of their methodologies, results, discussions and conclusions explore the spatial implications. The spatial resolution of the studies has also been evaluated and represented in the figure using a symbol hierarchy to demonstrate which studies had the highest resolution. The other focuses of the studies have also been graded in the same manner to highlight the primary objectives of the studies, including the fiscal, emissions, temporal, environmental and social context of the studies.
BECCS Geospatial Analysis Context and Resolution
The focus and resolution of BECCS geospatial analysis studies vary drastically from study to study, ranging from studies with almost zero geographic perspective to those with high spatial resolutions. The spatial context of BECCS studies is not typically the primary focus of the analysis, resulting in many using very little spatial data. Many BECCS studies have opted to map the locations of key infrastructure for BECCS, such as CO2 storage sites and point emission sources, but have not carried out extensive spatial analysis (Baik et al., 2018; da Silva et al., 2018; Albanito et al., 2019; Melara et al., 2020; Sagues et al., 2020).
The spatial resolution of the majority of transnational BECCS studies are limited by the data availability and data quantity, resulting in most transnational BECCS studies opting for a country block spatial resolution with values assigned to each country with no differentiation of geospatial data within the country blocks (Fajardy and Mac Dowell, 2018; Negri et al., 2021). The Rosa et al. (2021) trans-national study currently has the highest spatial resolution of all trans-national BECCS studies by using 1 km blocks rather than country blocks.
Some BECCS studies have opted for large spatial blocks, ~40–50 km, to differentiate the distribution of resources and infrastructure (Zhang et al., 2019; Gabrielli et al., 2020), but the most common form of spatial resolution used in BECCS studies are the local authority blocks. The use of local authority blocks offers an acceptable level of resource and infrastructure differentiation within a country while not producing extremely large quantities of data. Local authority blocks are most commonly used for countries with large land areas, such as the US and China (Baik et al., 2018; Dolan et al., 2020; Xing et al., 2021).
The spatial resolution of BECCS studies has increased over time with the development and popularization of high spatial resolution mapping of the technology. Most high spatial resolution studies opt to use small spatial blocks, ranging from 1 to 2 km, to differentiate resources within the countries (Donnison et al., 2020; Krause et al., 2020; Rosa et al., 2021). The Freer et al. (2021) study is currently the only geospatial analysis BECCS study that has achieved a higher spatial resolution down to the plot of land. This study does have a higher spatial resolution than the Rosa et al. study, but the Rosa et al. study covers a much larger study area for Europe compared to only the UK in the Freer et al. study.
BECCS Geospatial Analysis Study Areas and Scale
The study areas of geospatial BECCS studies have been primarily focused within the northern hemisphere. The majority of the studies focus on the UK and the US due to a high level of data availability, political will and abundance of pre-existing infrastructure compatible with BECCS (Sanchez and Callaway, 2016; Baik et al., 2018; Fajardy and Mac Dowell, 2018; Albanito et al., 2019; Zhang et al., 2019; Dolan et al., 2020; Donnison et al., 2020; Melara et al., 2020; Sagues et al., 2020; Freer et al., 2021). Studies focusing on Europe and the countries within are becoming more frequent as the potential for trans-national collaborations develop and mature (Gabrielli et al., 2020; Krause et al., 2020; Negri et al., 2021; Rosa et al., 2021). Geospatial BECCS studies focusing within Asia are not well represented in the literature, with only one study focusing on China (Xing et al., 2021). This lack of representation is also apparent for countries within the Middle East, South America, Indo-Australasia and Africa, showing a major research gap.
There has been a clear progression of the scale of analysis within geospatial BECCS studies. The first studies focused on smaller regional evaluations, which then developed into national evaluations. The next stage of evaluation will be the analysis of multiple collaborating countries, which can be seen in the publication of trans-national BECCS studies in recent years.
BECCS Biomass Types
The most researched type of biomass feedstocks in BECCS geospatial analysis studies are energy crops including miscanthus, switchgrass, willow short rotation coppicing (SRC) and poplar SRC (Fajardy and Mac Dowell, 2018; Albanito et al., 2019; Dolan et al., 2020; Donnison et al., 2020; Negri et al., 2021; Xing et al., 2021). However, these studies do not specify where exactly the biomass is or will be grown, as they use generalized blocks. There are fewer studies involving other biomass types such as agricultural residues including wheat straw, corn stover and woody residues (Sanchez and Callaway, 2016; Fajardy and Mac Dowell, 2018; Krause et al., 2020; Negri et al., 2021; Xing et al., 2021) or industrial wastes including MSW, forestry residues, wastewater treatment, pulp, paper and manure (da Silva et al., 2018; Zhang et al., 2019; Gabrielli et al., 2020; Melara et al., 2020; Sagues et al., 2020; Freer et al., 2021; Rosa et al., 2021). This paper addresses some of these gaps by specifically considering opportunities for utilizing waste and residues in BECCS facilities.
Other BECCS Context
Early BECCS analyses rarely focused on spatial context. However, more recent studies have recognized the integral and critical role of spatial context in the sustainable development and deployment of the technology, but a paradigm shift toward the incorporation of the spatial context of BECCS starting to play an integral and critical role in the sustainable development and deployment of the technology.
The majority of geospatial BECCS studies tend to focus more on the fiscal and emissions contexts of BECCS due to nations exploring the logistics and operations of deploying BECCS (Sanchez and Callaway, 2016; Baik et al., 2018; Fajardy and Mac Dowell, 2018). Only a few studies focus on the environmental and temporal contexts and very few focusing on the social context (Zhang et al., 2019; Gabrielli et al., 2020; Melara et al., 2020; Sagues et al., 2020; Freer et al., 2021; Negri et al., 2021; Rosa et al., 2021; Xing et al., 2021). There is a clear gap in the research focusing on the spatial aspects of BECCS with a social framing, exploring the spatial variations in public perception, ethics and governance of BECCS.
BECCS Supply Chain Tethering
Supply chain tethering, a concept developed in Freer et al. (2021), is a quantifiable and comparable metric of understanding which limb of a supply chain produces the most CO2 emissions and is used to inform decision making on how to best target carbon emissions saving measures. For example, a BECCS supply chain can be 6.04 times more tethered to its CO2 infrastructure compared to its biomass infrastructure, indicating that the CO2 emissions produced in CO2 transportation are 6.04 times larger than the CO2 emissions produced in biomass transportation. Knowing the scale of tethering for a BECCS facility will allow prioritization of carbon saving measures, as in the example case, measures targeting CO2 transportation emissions would have greater overall savings compared to measures targeting emissions from biomass transportation.
Some styles of BECCS may also be more tethered to certain aspects of the supply chain, as the sugar beet derived bioethanol BECCS supply chain in the Freer et al. study was more tethered to its biomass infrastructure (Freer et al., 2021). However, due to no two BECCS supply chains operating the same way, not all BECCS supply chains are more tethered to their biomass than their CO2 or energy output and not all supply chains are more tethered to its CO2. This difference in tethering is also apparent when a BECCS supply chain is shifted location, as in one location the supply chain may be more tethered to its CO2 infrastructure, while in another location it may be more equally tethered to its CO2 and biomass infrastructure. Each supply chain must be evaluated on a case-by-case basis and, depending on the locations of the supply chain, exists on a spectrum of tethering between the limbs of the supply chains.
Methodology
BECCS Supply Chain Descriptions
Three BECCS supply chains were selected and applied through strict criteria to maximize the impact and real-world applications of the results. To be included, supply chains must: utilize a UK domestic feedstock; the biomass types must cover a range of spatial distributions; similar supply chains should be mentioned in industrial and governmental reports; must use a mixture of carbon capture technologies; must cover a range of different types of BECCS; and one must generate hydrogen fuel. Based on a literature search, the following three supply chains met all of the criteria and have been used in this analysis (Muresan et al., 2013; Moreira et al., 2016; Mendiara et al., 2018; Pour et al., 2018; Tagomori et al., 2019; Agrawal and Rao, 2020; Emenike et al., 2020; Ghiat et al., 2020; Patzschke et al., 2020; García-Freites et al., 2021):
1. The “MSW supply chain,” evaluates the direct combustion of the organic fraction of municipal solid waste (MSW) for electricity generation with fitted post-combustion monoethanolamine carbon capture technology. This supply chain best represents the BECCS-Waste-to-Energy classification of BECCS.
2. The “Wheat Straw supply chain,” evaluates the combustion of wheat straw in an oxygen-rich environment fitted with oxy-fuel carbon capture technology for electricity generation. This supply chain best represents the BECCS-Power classification of BECCS.
3. The “Sawmill Residue supply chain,” evaluates the co-generation of electricity and hydrogen derived from the gasification of sawmill residues converted into pellets through the process of integrated gasification combined cycle (IGCC) fitted with pre-combustion carbon capture technology. This supply chain best represents the BECCS-Hydrogen classification of BECCS.
Block flow diagrams for each of the three supply chains are presented in Figure 4, depicting the types of biomass used, energy output generated and CO2 transportation via an ICCS cluster for offshore geological storage. Each block flow diagram illustrates potential geological storage in either the Goldeneye, Endurance, or Hamilton reservoirs.
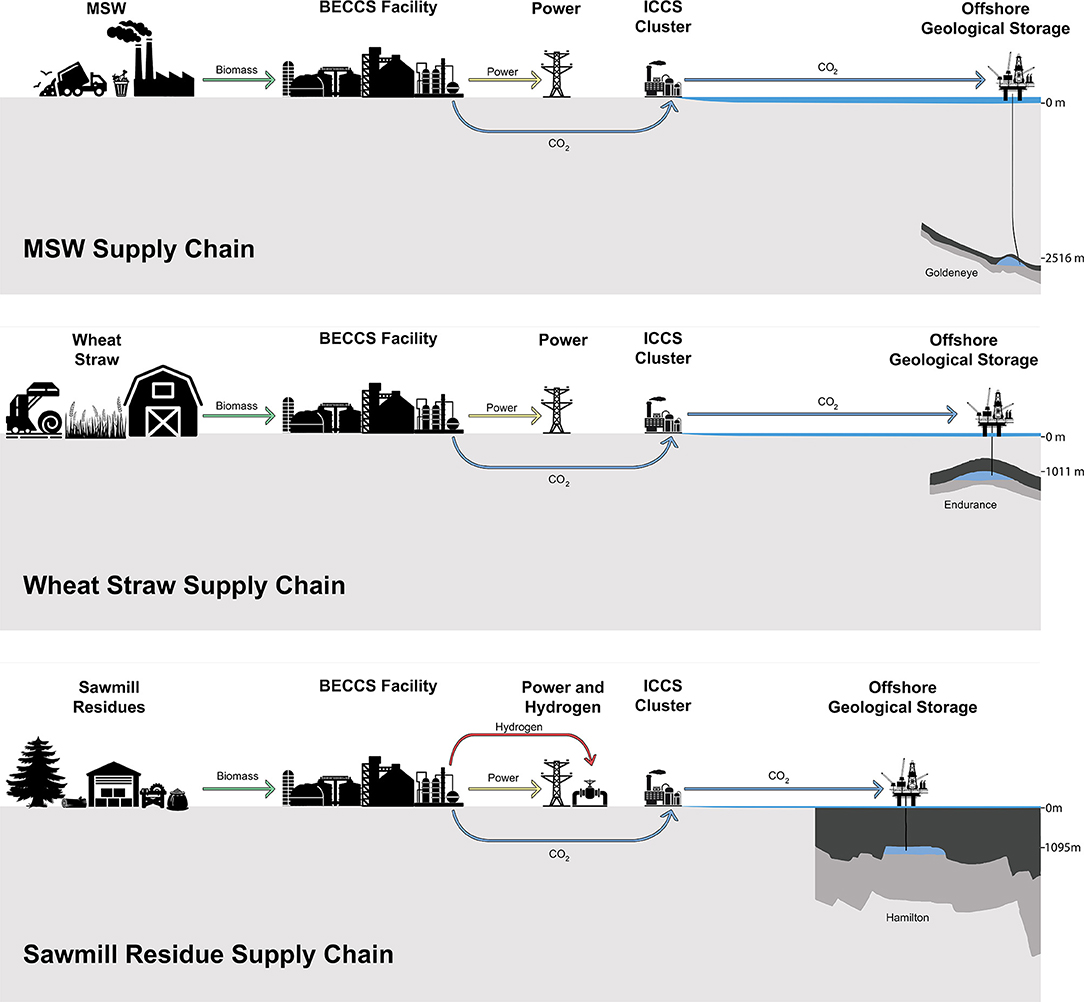
Figure 4. Block flow diagrams for the MSW supply chain, wheat straw supply chain and sawmill residue supply chain with geological storage in either the goldeneye, endurance or Hamilton reservoirs, with relative geological and water depths.
BECCS Supply Chain Biomass Mapping
Different methods could be used to map the supply chains, each with different relative strengths and weaknesses in relation to the quality of data recording, scale and resolution; consequently a variety of mapping methods has been used to map the organic fraction of MSW, wheat straw, and sawmill residue.
The spatial distributions for the organic fraction of MSW, wheat straw and sawmill residue are presented in Figure 5.
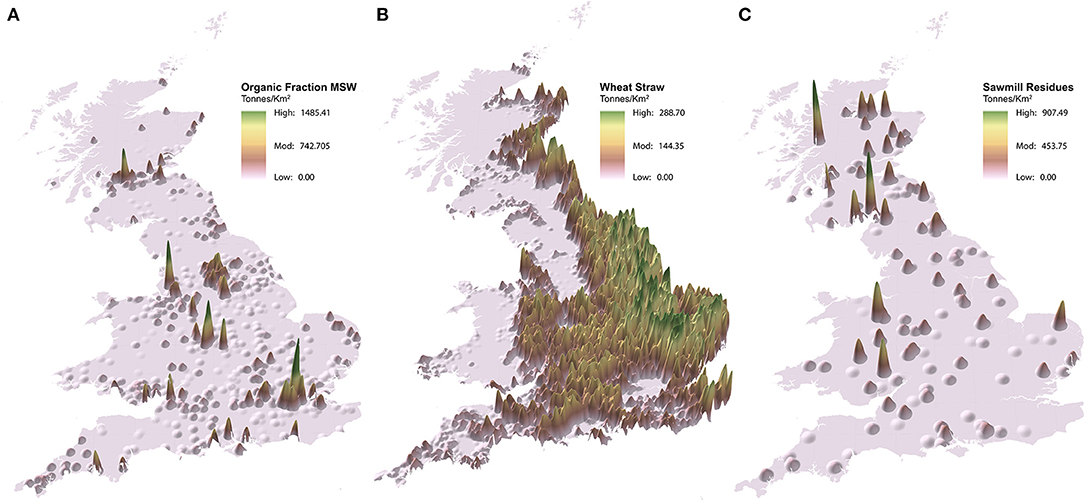
Figure 5. (A) Spatial distribution of organic fraction of MSW, (B) spatial distribution of wheat straw, (C) spatial distribution of sawmill residue.
The mapping of the organic fraction of MSW used waste generation data recorded by local authorities. The amount of biomass generated at each waste facility/site was allocated and integrated into the biomass repository. The supply chain taps into the organic fraction of MSW sent for incineration and landfill waste stream, as this waste redirection offers better utilization of the resource and targets the base resources of the waste management hierarchy for greater biomass availability.
The mapping of the wheat straw used a spectral image analysis approach. The base data for the current distribution of wheat straw locations in the UK was taken from the UKCEH Land Cover Plus Crops dataset for 2020 (UKCEH, 2019). From this dataset, the wheat straw plots of land were extracted and had their areas measured. A wheat straw yield was applied to each polygon, which is presented in Table 1. The total wheat straw was then verified against reported DEFRA census data to verify the results (DEFRA, 2020), of which they did match.
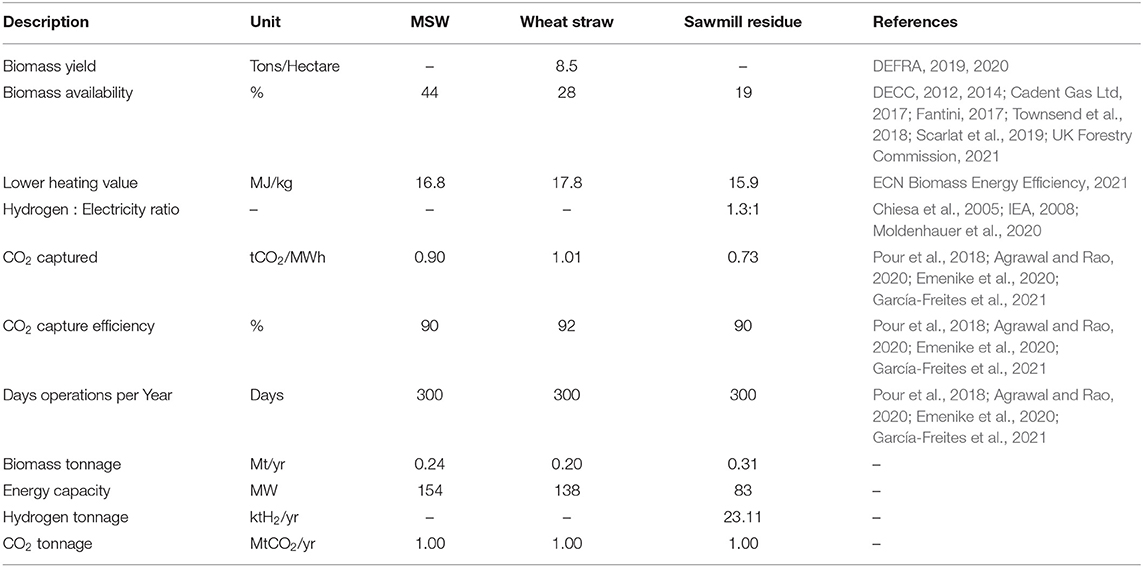
Table 1. MSW supply chain, wheat straw supply chain and sawmill residue supply chain inputs and output parameters.
The mapping of the sawmill residue was performed using a combination of biomass accounting and satellite image analysis. The data availability for the UK forestry industry is very high. However, most of the data has been anonymised, making it difficult to allocate amounts of biomass to specific locations. This anonymising issue was bypassed by using satellite imagery to measure the area of the lumber yards at each of the sawmills in the UK. The amount of timber processed at eight sawmills was found and assigned to their respective sawmill lumber yard (UK Forestry Commission, 2021). These eight data points were then used to determine the amount of roundwood produced per m2 of lumber yard and used to determine the amount of roundwood produced at all the other sawmills. The total amount of roundwood was then verified against the total anonymised data from the forestry commission to verify the results, of which they did match. Sawmill residue yields for sawdust and chipwood were then applied to each site.
Carbon Navigation System Model Parameters
The three supply chains have all been calibrated and standardized to store 1 MtCO2/yr and produce an energy output in the form of power or power and hydrogen. A 1 MtCO2/yr storage scale was selected as this reflects the scale of upcoming large-scale BECCS facilities, although future analysis will focus on the performance of smaller-scale facilities. The parameters of the supply chains are presented in Table 1.
Carbon Navigation System Infrastructure
CO2 Transportation Infrastructure
The UK has one of the fastest developing CCS infrastructure networks in the world, with plans rapidly evolving as the first geological injection, scheduled for 2024 (Acorn, 2017a; CCC, 2019; OGC, 2019; Pale Blue Dot, 2019; ZeroCarbonHumber, 2019; HyNet, 2020; BEIS, 2021).
The UK has adopted an ICCS cluster approach with five ICCS clusters under development and chosen due to their proximity to gas terminals for offshore geological storage, pre-existing infrastructure and in the catchment of multiple CO2 emitters. The five ICCS clusters are in Scotland, Teesside, North-West England, Humberside, and South Wales. The current UK CCS strategy is to establish four low carbon clusters by 2030 and one net-zero cluster by 2040 (BEIS, 2018; HM Government, 2018). The locations of the ICCS clusters are presented in Figure 6.
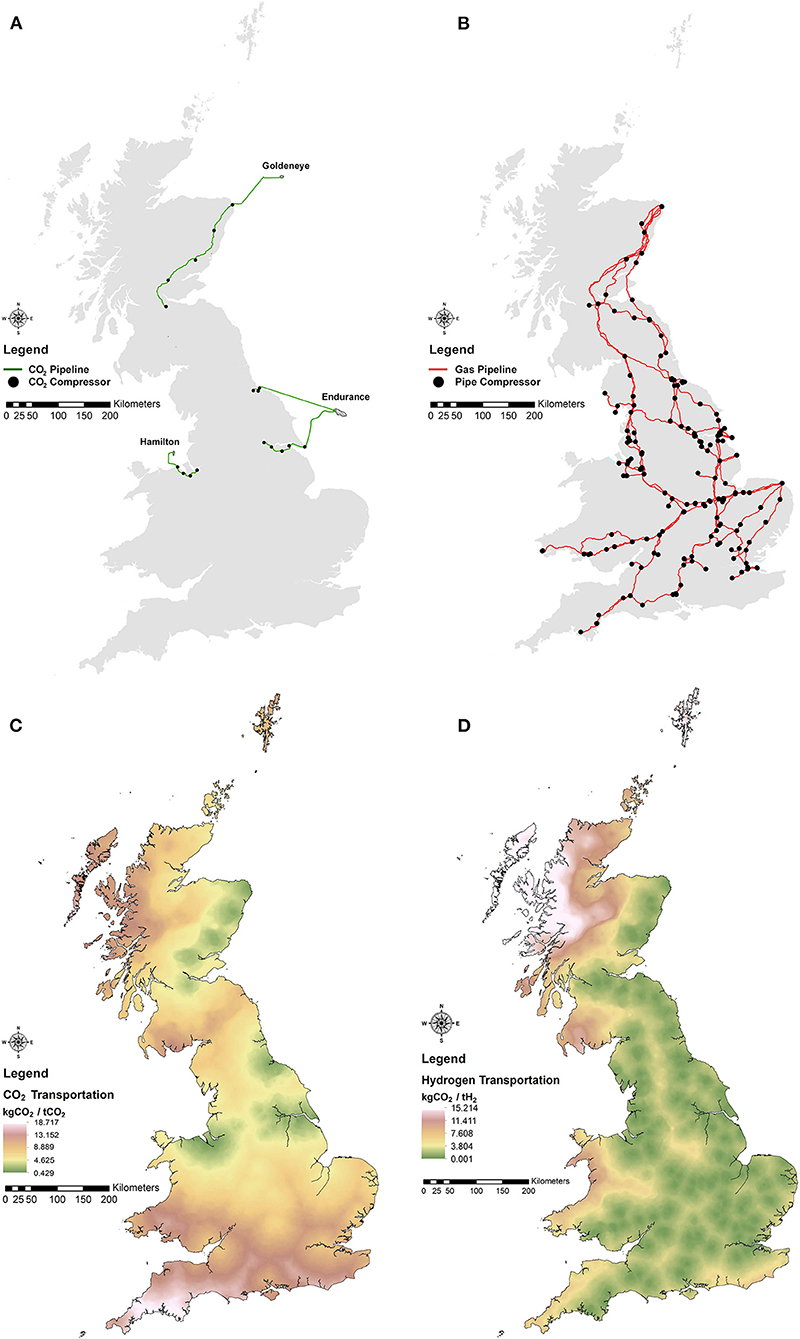
Figure 6. Updated CO2 and H2 limb networks for the CNS model, (A) CO2 compatible pipeline network for the UK, (B) National grid pipeline network for the UK, (C) CO2 emissions associated with the transportation of CO2 from anywhere in the UK into either the Hamilton/Endurance/Goldeneye storage site via an ICCS cluster, (D) CO2 emissions associated with the transportation of H2 from anywhere in the UK into the national grid via injection.
The Teesside, North West and Humberside ICCS clusters have opted to construct new CO2 compatible pipelines for offshore geological storage of CO2 (OGC, 2019; ZeroCarbonHumber, 2019; HyNet, 2020), while the Scottish cluster will repurpose the feeder 10 and offshore pipelines for CO2 transportation (Acorn, 2017a,b; Pale Blue Dot, 2019). Due to the lack of proximal geological storage to the South Wales cluster, the cluster has opted to transport CO2 via ship to be integrated into the North West cluster.
The UK has access to a large potential of secure offshore geological storage sites in the North Sea and the east Irish Sea, with theoretical storage capacities estimated to be between 61.7 and 75.6 GtCO2 (Pale Blue Dot, 2020). The most suitable storage sites in the North Sea in terms of reservoir thickness, permeability, storage capacity, level of containment risk, CAPEX + OPEX costs, level of seismic surveys, CO2 injection rates, caprock viability and level of pre-existing infrastructure are Captain X, Goldeneye, Forties 5, Bunter Enclosure 36, Endurance, Viking A, Hewett, Hamilton and Morecambe formations (Jin et al., 2012; Pale Blue Dot, 2016, 2020; Williams et al., 2016; Akhurst et al., 2017; HM Government, 2018). This analysis assumes an early level of development and deployment of geological storage, resulting in the use of the Goldeneye, Endurance and Hamilton storage sites in the analysis, with Monte Carlo theoretical storage capacities not exceeding 50% of estimates (P50) of 37, 248, and 122 MtCO2, respectively (Pale Blue Dot, 2020). The Goldeneye and Hamilton reservoirs are depleted gas fields, while the Endurance reservoir is a saline aquifer.
This study reflects the current UK CCS strategy by focusing on England, Scotland and Wales. This research can be rolled out to cover Northern Ireland and the potential Londonderry shipping ICCS cluster (BEIS, 2020), but has not been included due to limitations and barriers such as grid reference projection, the integration of island infrastructure, potential tariffs and politics within Ireland and implications of CO2 storage with the IMO London Protocol (Dixon, 2019).
The UK CO2 pipeline networks and the carbon transportation costs of CO2 storage in the Goldeneye, Endurance and Hamilton reservoirs from anywhere in the UK are presented in Figure 6.
H2 Transportation Infrastructure
Hydrogen has many advantages as a clean energy vector due to its high energy density, potential for combustion products without pollutants, renewability, and its possible use in transport, industrial and domestic applications (Rashid et al., 2015; Felgenhauer et al., 2016; Zhou and Park, 2020). Hydrogen can be transported by ship or pipeline, with straightforward modifications to existing infrastructure, with onshore and offshore storage potential in the UK and could substitute fossil fuels in various applications including industrial processes, heat and transport (Kuo and Wu, 2015; Gerboni, 2016; Gondal, 2016; Dawood et al., 2020; ETIP Bioenergy, 2021; HM Government, 2021; Yu et al., 2021). It has been estimated that the deployment of hydrogen to phase out fossil fuels in the UK could result in a 0.6–2.3% emissions reduction by 2030 (FCH, 2020). The UK Hydrogen strategy has also set ambitions to generate 5 GW of low carbon hydrogen by 2030 (HM Government, 2021). Although, uncertainties remain in the magnitude and extent of the UK hydrogen economy (Calver et al., 2022). Hydrogen production is projected to play a substantial part in the pursuit of net-zero and plays a larger role in the UK's ten-point plan (HM Government, 2020b). A phased deployment of hydrogen supply is predicted for the UK, starting with hydrogen derived from a fossil fuel source with CCS (blue hydrogen) with the objective to switch to hydrogen derived from renewable sources (green hydrogen) (HM Government, 2021; UK Parliament, 2021).
Bio-hydrogen is an example of a third-generation biofuel and may be formed via many technologies, including steam reformation, gasification, fermentation, microbial fuel cells, and algal cultivation (ETIP Bioenergy, 2021). The carbon balance of bio-hydrogen has the potential to be carbon neutral, but when combined with CCS, a form of BECCS, has the potential to produce a net-negative carbon balance (Zhou and Park, 2020).
Hydrogen has the potential to be blended with natural gas in a national grid. HyNet in the northwest of England has proposed to inject hydrogen into the national grid at a 20% volume, with the goal of reaching 100% in the future (HyNet, 2020). Other projects such as H2 Aberdeen are seeking to develop a hydrogen economy through hydrogen fuel HGVs, hydrogen district heating and national gas grid replacement (Aberdeen City Council, 2015). In line with these initiatives, the hydrogen produced by the Sawmill Residue supply chain case study was directed for national grid injection at 20% volume. The national grid pipelines and the carbon transportation costs of H2 injection into the national grid from anywhere in the UK is presented in Figure 6.
Carbon Navigation System Infrastructure Upgrade
The CCS infrastructure in the UK is quickly evolving toward the goal of efficiently and permanently storing CO2 in the offshore subsurface. The Teesside and Humberside clusters have recently formed a collaboration named the “Northern Endurance Partnership,” which plans to introduce a new CO2 compatible pipeline directly connecting the Teesside cluster to the Endurance reservoir (Zero Carbon Humber, 2020). The CNS model has been updated to incorporate this new pipeline into all calculations. The updated CO2 pipeline network is presented in Figure 6.
Carbon Navigation System Cost Matrix and Emissions Profiles
The CNS model uses a standardized carbon-based cost matrix, kgCO2/ton-km, to minimize the spatially explicit CO2 emissions produced by the supply chain. This analysis assumes that all transportation is fossil fuel derived and uses the same fossil fuel emissions profile and transport switching costs used in Freer et al. (2021). This analysis also assumes that empty return journeys do take place within the supply chains.
The three modeled BECCS supply chains all produce power as an energy output, producing negligible spatially explicit supply chain emissions from the transportation of the power through the grid. Due to this, the energy output limb of the CNS was not used for the three supply chains. The emissions produced via the construction of new powerlines to rural areas fall out of scope for this analysis, but it is important to note that there will be additional carbon costs for connecting BECCS-Power in rural areas at a rate of 6.3 ktCO2/km (Harrison et al., 2010).
Biomass Data Processing
The CNS model is capable of searching for specific quantities of biomass through the processing of biomass into standardized “Biomass Packages” through a named process of either splitting or bundling (Freer et al., 2021). Splitting the biomass points involves taking a singular biomass location representing a large quantity of biomass and splitting the singular point into multiple stacked points, each representing 27.45 tons of biomass (truck capacity with applied loading factor). Bundling the biomass is applied when a singular biomass point represents <27.45 tons of biomass and is combined with other biomass points to constitute the standardized 27.45 tons of biomass while integrating the added carbon cost of gathering the biomass points together. The process of splitting and bundling the biomass data is further explained in Freer et al. (2021).
The biomass data within the MSW supply chain and Sawmill Residue supply chain were split, while the biomass data within the Wheat Straw supply chain were split and bundled due to the distribution of high and low yield locations.
Biomass Availability and Availability Distribution
Biomass availability considered in this study is the percentage of total biomass production that is collatable and of high enough quality for energy production (Welfle et al., 2014a,b). Due to the lack of data accessibility for biomass availability for BECCS, bioenergy biomass availability was used as a proxy. The biomass availability percentages for each supply chain have been taken from the literature specific to each biomass type and are presented in Table 1 (DECC, 2012, 2014; Cadent Gas Ltd, 2017; Fantini, 2017; Townsend et al., 2018; Scarlat et al., 2019; UK Forestry Commission, 2021). The chosen biomass availability percentages are representative of the current biomass strategy but may be subject to change with future policies and climate impacts and would require future work to determine the impact of a variable biomass availability on BECCS supply chains.
An equal distribution of biomass availability was also assumed due to the lack of spatially explicit reported data for biomass use and competition distribution. Future work will be required to determine the spatial distribution of biomass availability but would require a fully transparent database of biomass users for the entire UK at high spatial resolution.
Batch Processing of the Supply Chains
Each of the supply chains was simulated over a range of locations to create the carbon performance heatmaps. The CNS model calculates the routings for one location, dissolves the routings into the point, and then automatically moves onto the next locations. Each of the supply chains were simulated over 219,878 locations to generate the heatmaps, resulting in 6.01 billion total routings between the three supply chains. Each of the supply chains also perform drastically differently for the same location. Figure 7 depicts the routings for the three supply chains from the same location, which access the Endurance storage site via the Teesside ICCS cluster, showing the range in the footprints of the supply chains.
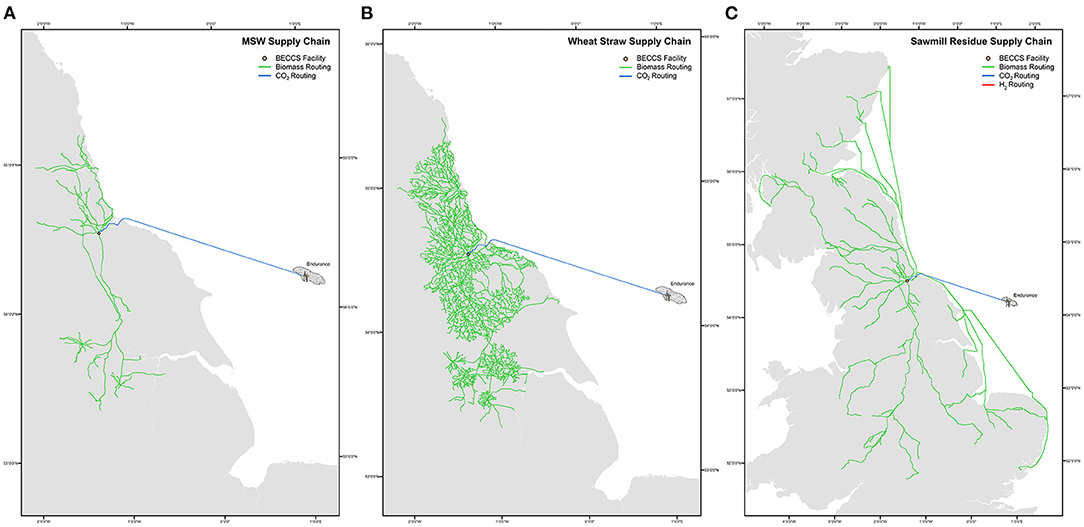
Figure 7. Biomass, CO2 and H2 routings for the three supply chains simulated from the same location accessing the endurance CO2 storage site via the Teesside ICCS cluster, (A) MSW supply chain, (B) wheat straw supply chain, (C) sawmill residue supply chain.
Each of the simulated BECCS facility emissions for the supply chains were dissolved and joined to their respective point locations, where an inverse distance weighted interpolation was applied to generate the carbon performance heatmaps.
Results and Discussion
This analysis evaluates the carbon performances of three modeled BECCS supply chains across the UK using the CNS model. The carbon performance heatmaps for the MSW, Wheat Straw and Sawmill Residue supply chains is presented in Figure 8.
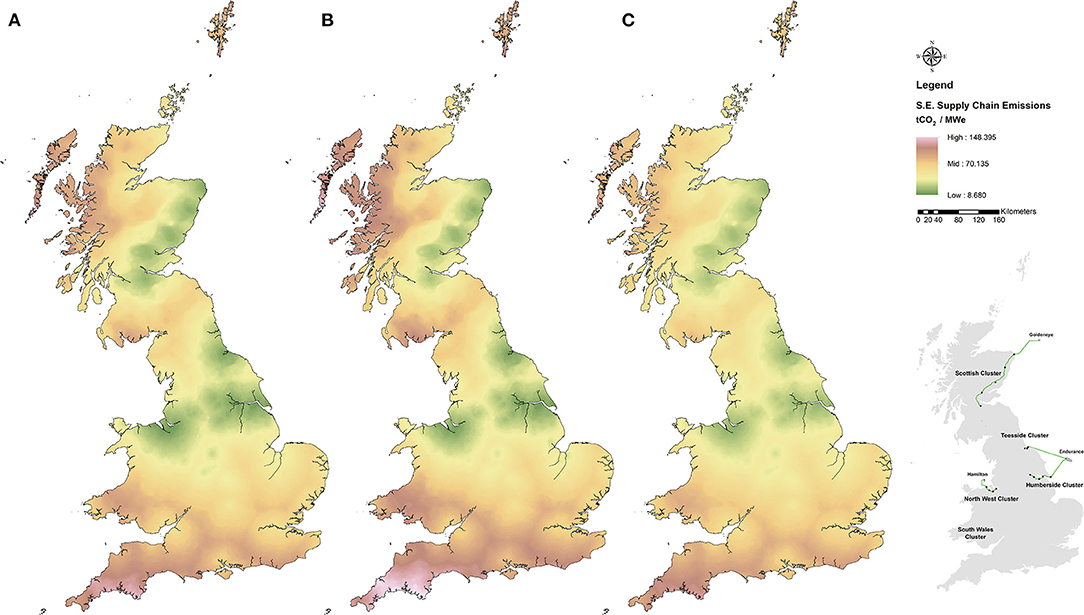
Figure 8. Carbon performance heatmaps depicting the spatially explicit supply chain emissions for the modeled BECCS supply chains, (A) MSW supply chain, (B) wheat straw supply chain, (C) sawmill residue supply chain, along with an annotated ICCS cluster map for the UK.
BECCS Supply Chains Carbon Performance Areas
Across all three supply chains, the areas of highest performance (areas that have produced the lowest amount of supply chain transportation emissions) are concentrated around the ICCS clusters that use a dedicated CO2 pipeline. Compared to the other clusters, the South Wales cluster underperforms due to the large and carbon-intensive distance required to transport CO2 for geological storage. The decarburization of the shipping or rail industry would greatly benefit the carbon performance of the area. Additionally, the conversion of an oil platform in the Irish sea into a CO2 catchment hub with a dedicated pipeline connected to the geological storage sites in the area would benefit the South Wales cluster as well as Northern Ireland, Ireland and the rural west coast of the England and Scotland.
The siting location with the highest performance for the MSW and Sawmill Residue supply chains is Connah's Quay (located within the North West ICCS cluster) due to its strong access to CO2 infrastructure, available biomass, low carbon infrastructure and energy end-user. Contrarily, the highest performing siting location for the Wheat Straw supply chain is in Barrow-Upon-Humber (located within the Humberside ICCS cluster) as this location has greater access to available biomass. Areas surrounding low carbon transport switching locations, such as shipping ports and rail terminals, have elevated performance due to the increased access to less carbon-intensive transport methods. The least optimal location for all three supply chains is Kingsbridge (30 km east of Plymouth) due to it being the furthest distance in the UK from one of the modeled geological storage sites. Great efforts in decarburization would have to be made to improve the carbon performance of the area. This is not to say that BECCS is not possible in this location, but rather these three types of BECCS do not perform well in this location. Another type of BECCS, perhaps a high purity low yield manure derived anaerobic digestor BECCS would perform better in this area but would still require major decarburization of transportation.
Carbon Impact of Shifting BECCS Siting
The optimization of the siting of a BECCS facility is critical in minimizing the positive emissions in the projects carbon balance to achieve a favorable net-negative carbon balance. It may not be possible to site a BECCS facility in its most carbon-optimal location due to competition, in terms of land and available infrastructure/resources, with other sectors or potentially other BECCS facilities. The siting of a BECCS facility is extremely important to maximize its carbon performance.
The shifting of the siting location of each supply chain results in drastic changes in their carbon performance. For each of the supply chains, multiple radial lines of points every 10 m perpendicular to the transition from green to orange to red/white regions of the carbon performance maps were used to extract the values, and the average percentage change in carbon performance from green regions to orange regions and green regions to red/white regions were recorded. These average percentage changes showcase the percentage increase in supply chain emissions for each of the supply chains if they were moved from a high performance area (green region) to a moderate performance area (orange region) and to a low performance area (red/white region).
Shifting the siting of the BECCS facilities from a green region of the performance maps to an orange region results in a 298.8–804.8% increase in spatially explicit supply chain emissions, while shifting from a green zone to a red/white zone results in a 597.5–1,609.6% increase. A breakdown of the percentage changes in shifting from a green region to an orange and red/white region by each of the supply chains is presented in Table 2. Dramatically shifting the location of the BECCS facility in such a manner will make achieving a favorable net-negative carbon balance very difficult. On average, a 10 km shift in the siting of a BECCS facility will result in between an 8.6 and 13.1% increase in spatially explicit supply chain emissions.
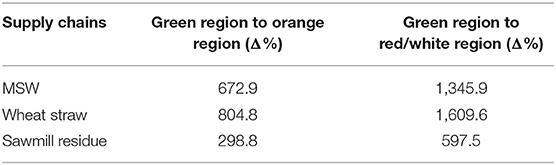
Table 2. Percentage increase in spatially explicit supply chain emissions for the MSW, wheat straw and sawmill residue supply chains from shifting the siting of the facilities from a green region to an orange region and from a green region to a red/white region.
BECCS Supply Chain Tethering
The carbon performance heatmaps for the supply chains look similar as all three of the modeled supply chains in this analysis are more tethered to their CO2 infrastructure than to their biomass and hydrogen, indicating that greater carbon savings would be made by focusing decarburization efforts on CO2 transportation rather than the other limbs of the supply chains. However, the level and scale of tethering varies dramatically between the supply chains and where the BECCS facility is located.
Whether or not a BECCS supply chain will be more tethered to its biomass or its CO2 infrastructure depends heavily on its geography, access to low carbon infrastructure, access to available biomass, distribution of its biomass and the ratios of transported tonnages. A generalized trend can be identified for the tethering of different styles of BECCS. BECCS supply chains which produce an initial high purity CO2 stream at low yields will generally be more tethered to its biomass [CPER, Decatur Illinois, Occidental White Energy, Lamberton, Plainview and Goldfield (BusinessWire, 2019; Global CCS Institute, 2021; Summit Carbon Solutions, 2021; U.S. Securities Exchange Commission, 2021)], while BECCS supply chains which produce an initial low purity CO2 stream at high yields will generally be more tethered to its CO2 infrastructure [Drax, Stockholm Exergi, Copenhill, Mikawa and Project Bright (Drax, 2019; Toshiba, 2020; Global CCS Institute, 2021; HyNet, 2021a; Stockholm Exergi, 2021)]. Although each supply chain would have to be tailored and evaluated on a case-by-case basis and depending on their relative access to available biomass and geological storage would exist on a sliding scale of tethering. Generally, high purity low yield BECCS supply chains will perform more carbon optimally in more rural areas with strong access to biomass, while low purity high yield BECCS supply chains will perform more carbon optimally in more industrial areas with strong access to geological storage sites.
The level and scale of tethering can be quantified by taking the ratio between pairs of each supply chain limb (CO2 limb, Biomass limb and H2 limb), revealing how many more times the supply chain is tethered to a specific limb. The scales of tethering for the modeled supply chains broken down by regions in the UK is presented in Figure 9. On average across the entire UK, the MSW supply chain is 6.04 times more tethered to its CO2 infrastructure compared to the other limbs, the Wheat Straw supply chain is 7.95 times more tethered and the Sawmill Residue supply chain is 2.36 times more tethered.
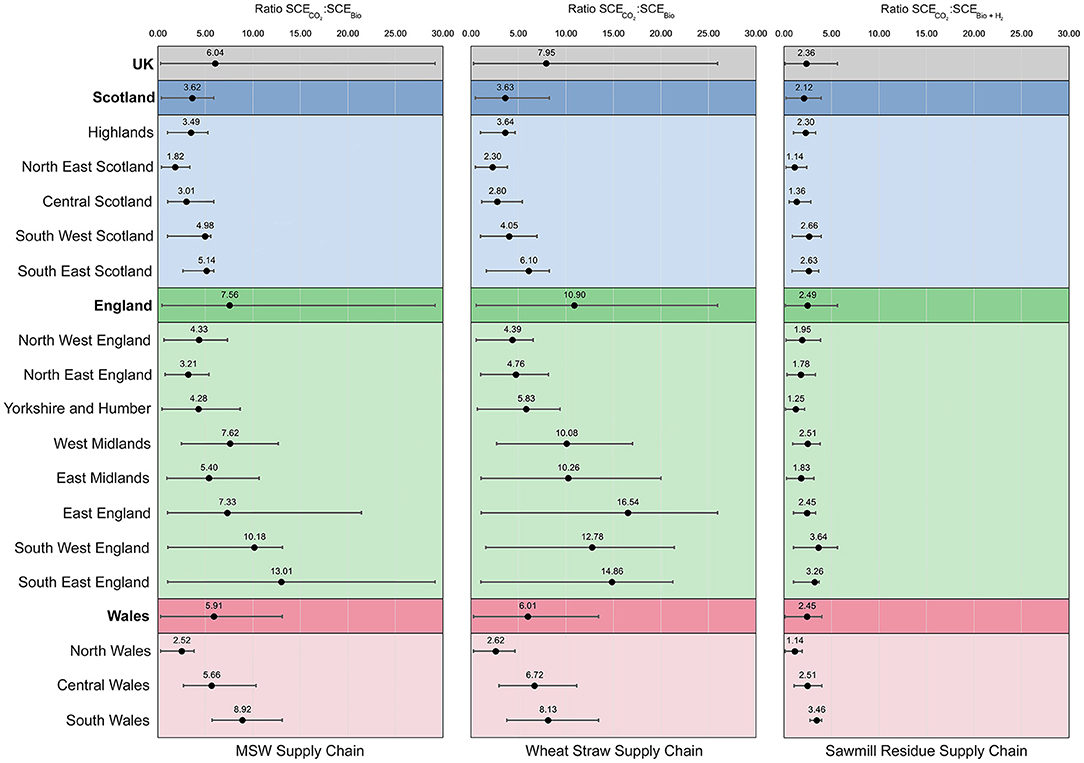
Figure 9. The scale of BECCS supply chain tethering for the MSW, wheat straw and sawmill residue supply chains across the UK broken down by regions.
The difference between the supply chain tethering across the modeled supply chains is driven by its access to available biomass, the distribution of its biomass, access to geological CO2 storage and access to its energy end-user. The MSW and Wheat Straw supply chains follow similar trajectories in their tethering as both have a well-distributed and plentiful biomass source across the UK, resulting in a wide range in the scale of supply chain tethering. The Sawmill Residue supply chain follows the same trajectory of tethering but has been skewed toward a more equal balance in supply chain tethering due to its concentrated and clustered biomass distribution and the level of access to its energy end-user. The sawmill residue across the UK are not as well distributed as the other modeled biomass types and tend to be far away from the ICCS clusters, resulting in further and more carbon-intensive biomass transportation than the other supply chains. The Sawmill Residue supply chains must also incorporate hydrogen transportation into the national grid into the tethering, while the other two supply chains do not. Other low purity high yield BECCS supply chains with concentrated biomass distributions, such as energy crop derived BECCS projects, will be expected to have similar and more equal scales of tethering to the Sawmill Residue supply chain rather than the other modeled supply chains.
Within a BECCS supply chain the scale of tethering varies greatly and exists on a spectrum depending on if the facility is located within an ICCS cluster or in a more rural location.
BECCS facilities located within ICCS clusters that have access to a CO2 pipeline tend to be more equally tethered to its biomass and CO2 but are still overall more tethered to its CO2 infrastructure. The level of tethering within 50 km of pipeline access for the Scottish, Teesside, North West and Humber clusters and 50 km from the shipping ports for the South Wales cluster are presented in Table 3. Indicating that a more balanced approach in decarbonizing the supply chain limbs would result in the most carbon savings while still having a slight focus on the CO2 limb. However, since the South Wales cluster lacks a CO2 pipeline, the facilities within the cluster are more tethered to its CO2 infrastructure than the UK average due to the large shipping distances to store CO2 within the Hamilton reservoir. On average, the MSW, Wheat Straw and Sawmill Residue supply chains are 8.92, 8.13, and 3.46 times more tethered to its CO2 infrastructure within the South Wales cluster, respectively. Indicating that greater carbon savings within the supply chains would be made if decarburization efforts were focused on decarbonizing shipping within the cluster compared to the rest of the supply chain.
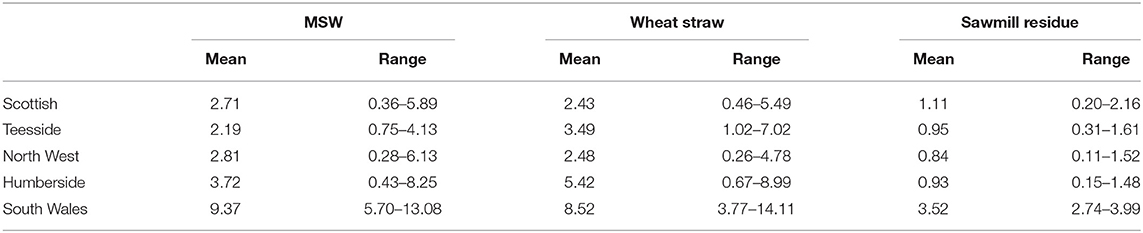
Table 3. Supply chain tethering for MSW, wheat straw and sawmill residue broken down by ICCS clusters.
Due to the lack of proximal access to CO2 storage sites, the scale of tethering for more rural areas of the UK are heavily tethered to its CO2 infrastructure for the modeled supply chains. On average, the MSW, Wheat Straw and Sawmill Residue supply chains are 3.94, 3.64, and 2.12 times more tethered to their CO2 infrastructure in the Highlands, respectively. The supply chains are 5.66, 6.72, and 2.51 times more tethered within Central Wales and 13.01, 14.86, and 3.26 times more tethered in South-East England. The decarburization of shipping and rail transportation would dramatically improve the carbon performances in these more rural areas around the UK, allowing for the wide dissemination of BECCS across the UK and not only siting the facilities within the ICCS clusters. A general trend across all supply chains is that the further south the BECCS facility is placed, the more tethered the facility is to its CO2 infrastructure. This is due to the majority of geological storage sites and CO2 compatible pipelines across the UK being in the country's northern regions, resulting in larger emissions accessing this infrastructure from the south.
BECCS Supply Chain Performances Within ICCS Clusters
The carbon performances of the modeled BECCS supply chains differ drastically within the ICCS clusters. The MSW, Wheat Straw and Sawmill Residue supply chains carbon performance maps focusing within each of the ICCS clusters is presented in Figure 10. The highest performance areas within the clusters are concentrated around the CO2 pipeline compressors. In contrast, for South Wales the highest performance areas are concentrated around the shipping ports and rail terminals since the cluster lack a CO2 compatible pipeline and proximal access to geological storage.
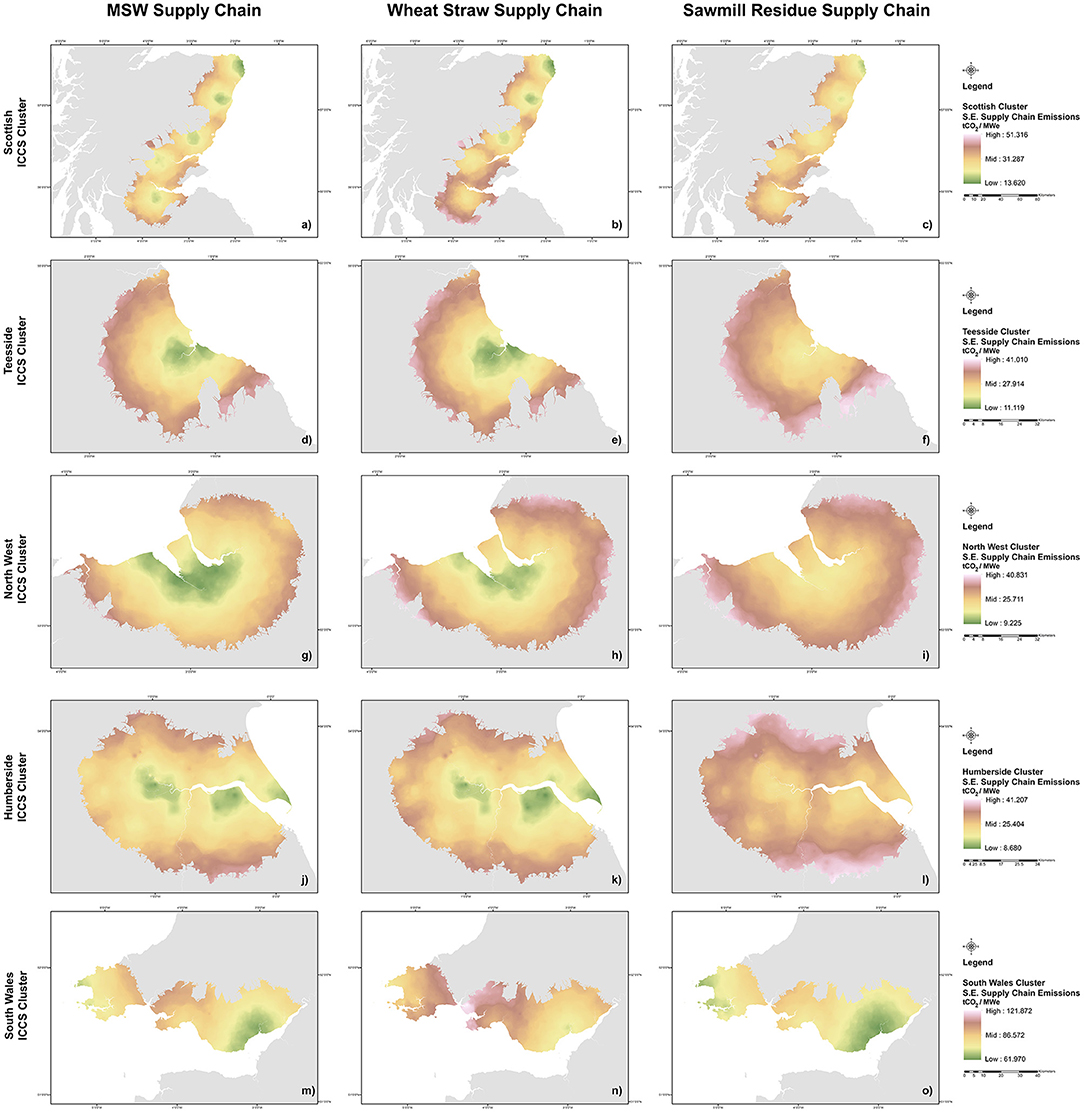
Figure 10. Carbon performance heatmaps depicting the spatially explicit supply chain emissions for the MSW, Wheat straw and sawmill residue supply chains, focusing within 50 km of an ICCS cluster, (a–c) the Scottish ICCS cluster, (d–f) the Teesside ICCS cluster, (g–i) the North West ICCS cluster, (j–l) the Humberside ICCS cluster, (m–o) the South Wales ICCS cluster.
The MSW and Wheat Straw supply chains outperform the Sawmill Residue supply chain in all ICCS clusters with a CO2 pipeline due to the comparatively increased access to available biomass, energy end-users and low carbon infrastructure. Notably, the MSW supply chain performs at a higher level along the entire length of the Feeder 10 pipeline in the Scottish cluster due to the abundance of available biomass, while the Wheat Straw supply chain performs at a higher level at the northern end of the pipeline due to the distribution of wheat straw being greater in the Northeast of Scotland. Contrarily, the Sawmill Residue supply chain outperforms both the MSW and Wheat Straw supply chains within the South Wales cluster due to the moderate access to sawmill residue and lack of large enough quantities of MSW and wheat straw to store the required 1 MtCO2/yr.
Comparative BECCS Supply Chain Decision Mapping
A decision map is a map that can choose between a selection of options under a specific set of criteria at a high spatial resolution to clearly show what should go where under the set criteria. The main objective of this decision map is to provide a heuristic to help inform stakeholders such as policymakers, NGOs, academia or industry in determining where to locate BECCS facilities.
A comparative BECCS supply chain decision map was generated for the three modeled supply chains, indicating for a given area of the UK which of the three supply chains captured 1 MtCO2/yr for the lowest amount of spatially explicit supply chain emissions per MWe. The decision map is presented in Figure 11.
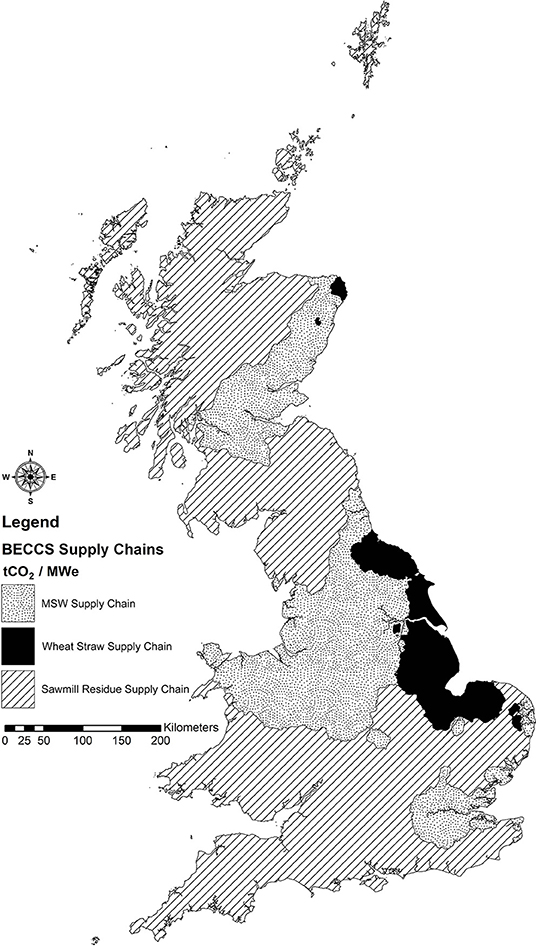
Figure 11. Comparative BECCS supply chain decision map. Identifying between the MSW, wheat straw and sawmill residue supply chains should be chosen for any given area of the UK, under the condition of capturing 1 MtCO2/yr for the least amount of spatially explicit supply chain emissions per MWe.
The phrasing of the question when generating a decision map is critical. A decision map showing which supply chains should be chosen to capture 0.5 MtCO2/yr for the lowest amount of spatially explicit supply chain emissions per MWe would show vastly different results than 1 MtCO2/yr. This is due to the smaller operational footprints of the supply chains as less distance is needed to be traveled to meet the biomass quotas to capture 0.5 MtCO2/yr compared to 1 MtCO2/yr.
The selection process deciding which supply chain is chosen is based on access to biomass, energy end-users and access to low carbon infrastructure. The MSW and Wheat Straw supply chains were selected for each location based on their biomass and low carbon infrastructure access. In comparison, the Sawmill Residue supply chain selection was based on its biomass, energy end-user and low carbon infrastructure access.
There are four main bodies of choice for the three supply chains across the UK. The Wheat Straw supply chain is the better choice across Teesside, the east side of Humberside and the coastal areas of the East Midlands and East England. The MSW supply chain is the better choice across North Wales, NW England, Yorkshire, the west side of Humberside and Scotland's east coast. The MSW supply chain has not been selected for South Wales, but it may have been chosen if the decision map was recalibrated for a smaller BECCS facility rather than 1 MtCO2/yr. The Sawmill Residue supply chain is best located in more isolated rural areas such as Southwest England, Cumbria, Scottish Highlands, the Outer Hebrides, Orkney and Shetlands.
Notably, there are a series of small zones within the decision map which identify one of the supply chains within another supply chain zone. This is most apparent for the Greater London area, where the MSW supply chain performs best within wider surroundings dominated by the Sawmill Residue. These zones are termed “enclaves” and are driven by areas with increased levels of access to biomass, energy end-users and particularly low carbon infrastructure compared to their surrounding areas. The Greater London enclave is selected due to the abundance of MSW and excellent access to shipping and rail terminals compared to the areas surrounding Greater London. There are eight groupings of enclaves across the UK, with the majority based in England, one in Scotland and none in Wales. There are more enclaves in England compared to Scotland and Wales due to the widespread distribution of low carbon transport switching locations. England has distinct areas of dense and sparse infrastructure which allow the decision map to form enclaves, while the infrastructure in Scotland and Wales is concentrated along the east coast and north and south coast, respectively, which makes enclaves less likely. The enclaves in England are driven by increased access to shipping ports and rail terminals, while the enclaves in Scotland are driven by increased access to the feeder 10 pipeline.
The information conveyed by the decision map has the potential to help inform policymakers and industry on how to best place BECCS facilities across the UK, but much more research will be needed to cover all aspects and contexts of BECCS deployment. The decision map only focuses on the carbon emissions for the modeled supply chains. Further research will be needed to ensure that BECCS facilities are right-sized into its local infrastructure, available biomass, economic environment, energy systems, land management strategies, geography, and socio-political context. The decision map has been calibrated for 1 MtCO2/yr, but many locations may be better suited for larger or more likely smaller facilities. Future research into determining the optimal BECCS facility scale for any location in the UK will be greatly needed to ensure a successful and sustainable deployment of BECCS. Future work will also be performed on the decision map to capture other constraints such as biomass availability volatility, transportation costs, integration of renewable fuels, rapidly evolving CO2 infrastructure and static supply chain emissions.
It is important to highlight that this study only considers the spatially explicit supply chain emissions for each supply chain and does not include the spatially static emissions such as biomass drying, biomass grinding, fuel combustion, CO2 compression, CO2 injection and CO2 remediation. The study also does not explore land-use change implications such as soil carbon sequestration, erosion control and consequences of climate change. This is particularly important for cereal straw derived BECCS projects like the Wheat Straw supply chain in this study. Further collaboration with LCAs, sustainability studies, economic feasibility studies and stakeholder engagement studies will be needed to ensure a sustainable and successful integration of BECCS into our energy generation, land management strategies, waste management strategies and socio-economic frameworks.
If the three modeled BECCS supply chains were to be rolled out across the UK, assuming the biomass availability used in this analysis, the theoretical output of the MSW supply chain would be 6.53 MtCO2/yr, the theoretical output of the Wheat Straw supply chain would be 12.39 MtCO2/yr and the theoretical output of the Sawmill Residue supply chain would be 3.79 MtCO2/yr. This would constitute 43.5% of the required amount of BECCS to meet Net-Zero by 2050 under the UK BECCS requirements in the Committee on Climate Change Sixth Carbon Budget balanced pathway scenario. The roll-out of these supply chains would not be as one mega facility, but rather disseminated as a series of smaller facilities that have been tailored and calibrated to be successfully integrated into their geographic, economic, environmental and social context. The realistic output of these supply chains may be less as these roll-outs calculations assume 100% utilization of available biomass, which may not be economically viable, particularly in more rural areas of the country. The theoretical outputs also assume no reduction in the level of competition with other sectors, particularly for MSW, and no reduction in biomass availability from zero-waste campaigns and policies.
Future analysis will also have to account for the impact of climate change on the supply of biomass for BECCS as some representative concentration pathways could see a 46% decrease in biomass yield (IPCC, 2021). The realistic outputs of the supply chains may also be increased through mixing the feedstocks with other sources or through importation, but this may reduce the calorific value of the fuels, reduce the supply of biomass for other countries and result in unwanted additional transportation emissions. Although, there are potential limitations in scaling up bioenergy and BECCS facilities to the required level to meet Net-Zero from what is currently available due to limited biomass supply and limited operational timeframes. These limitations may be circumvented with the recent advances in pre-treatment techniques and dry biomass storage systems to prolong the operational timeframes of the facilities and secure biomass supply (Victorin et al., 2020; Wendt and Zhao, 2020). Future analysis exploring the implications of multiple smaller decentralized BECCS facilities with reduced operational timeframes may also reveal potential pathways for BECCS deployment.
Conclusion
The work produced in this paper using the CNS model has the potential to aid the successful and sustainable deployment of BECCS across the UK. BECCS is an incredibly diverse and unique technology that needs to be carefully tailored to its local infrastructure, available biomass, economic environment, energy systems, land management strategies, socio-political context and geography for successful deployment and integration. Each BECCS facility must be evaluated on a case-by-case basis, and some supply chain designs perform better in some locations than others. The findings from this paper can be replicated for any other BECCS supply chain or simulated in any other country. The findings produced also have the potential to act as a useful heuristic for policymakers and industry when planning where to site specific BECCS projects.
The shifting of a BECCS facility will result in a drastic increase in spatially explicit supply chain emissions, where for the modeled supply chains for each 10 km shift in facility location results between an 8.6 and 13.1% increase in emissions. The optimal placement of a BECCS facility will play a significant role in achieving a favorable net-negative carbon balance and should be considered in the inception of each and every project.
A BECCS project may be more tethered to its CO2 infrastructure or biomass and, as a general rule, a BECCS project producing an initial high purity low yield CO2 stream will be overall more tethered to its biomass, and a project producing an initial low purity high yield CO2 stream will be overall more tethered to its CO2 infrastructure. All three of the modeled supply chains were more tethered to its CO2 infrastructure, but the scale of tethering varies greatly and exists on a spectrum depending on if the facility is located within an ICCS cluster or in a more rural location. Locations within ICCS clusters tend to be more equally tethered to both its biomass and CO2 infrastructure, with a slight preference for the CO2 infrastructure, and more rural areas tended to be more strongly tethered to its CO2 infrastructure; Indicating that low purity high yield BECCS project will perform at higher levels within ICCS clusters and high purity low yield BECCS projects will perform at higher levels in more rural areas.
This paper has also introduced a BECCS decision map indicating for any given area which of the modeled BECCS supply chains should be chosen to best store 1 MtCO2/yr for the least amount of spatially explicit supply chain emissions. The decision map reveals that the modeled supply chains have a performance proclivity for certain areas of the UK and that one BECCS supply chain that operates optimally in one location cannot be transplanted or replicated into a new location without tailoring the supply chain to its new geographic, economic, environmental and social context.
Data Availability Statement
The raw data supporting the conclusions of this article will be made available by the authors, without undue reservation.
Author Contributions
MF created and calibrated the model and software, performed the statistical analysis, and wrote the first draft of the manuscript. MF, CG, AW, and AL-L wrote sections of the manuscript. All authors contributed to conception and design of the study, contributed to manuscript revision, read, and approved the submitted version.
Funding
The work was funded by the scholarship support provided by the Department of Mechanical Aerospace and Civil Engineering (MACE) DTP Allocation at the University of Manchester, UKRI projects UKCCSRC (EP/PO26214/1), FABGGR (NE/P019951/1), IDRIC (EP/V027050/1) and UK Supergen Bioenergy Hub (EP/S000771/1).
Conflict of Interest
The authors declare that the research was conducted in the absence of any commercial or financial relationships that could be construed as a potential conflict of interest.
Publisher's Note
All claims expressed in this article are solely those of the authors and do not necessarily represent those of their affiliated organizations, or those of the publisher, the editors and the reviewers. Any product that may be evaluated in this article, or claim that may be made by its manufacturer, is not guaranteed or endorsed by the publisher.
References
Aberdeen City Council (2015). Aberdeen City Region Hydrogen Strategy. Available online at: https://committees.aberdeencity.gov.uk/documents/s45267/AberdeenCityRegionHydrogenStrategy2015-2025.pdf (accessed November 17, 2021).
Agrawal, H., and Rao, A. B. (2020). Chapter 61: Techno-economic potential of pre-combustion CO2 capture in bio-energy pathways, in Advances in Energy Research, Vol. 1 (Singapore: Springer).
Akhurst, M., Mallows, T., Pearce, J., and Mackay, E. (2017). Assessing interactions between multiple geological CO2 storage sites to optimize capacity in regionally extensive storage sandstones. Energy Proc. 114, 4571–4582. doi: 10.1016/j.egypro.2017.03.1577
Albanito, F., Hastings, A., Fitton, N., Richards, M., Martin, M., mac Dowell, N., et al. (2019). Mitigation potential and environmental impact of centralized versus distributed BECCS with domestic biomass production in Great Britain. GCB Bioenergy. 11, 1234–1252. doi: 10.1111/gcbb.12630
Ali, I., Cawkwell, F., Dwyer, E., and Green, S. (2017). Modeling managed grassland biomass estimation by using multitemporal remote sensing data-a machine learning approach. IEEE J. Select. Topics Appl. Earth Observ. Remote Sens. 10, 3254–3264. doi: 10.1109/JSTARS.2016.2561618
Baik, E., Sanchez, D. L., Turner, P. A., Mach, K. J., Field, C. B., and Benson, S. M. (2018). Geospatial analysis of near-term potential for carbon-negative bioenergy in the United States. Proc. Natl. Acad. Sci. U. S. A. 115, 3290–3295. doi: 10.1073/pnas.1720338115
BEIS (2018). Clean Growth The UK Carbon Capture Usage and Storage Deployment Pathway an Action Plan. London.
BEIS (2021). Cluster Sequencing for Carbon Capture, Usage and Storage (CCUS) Deployment: Phase-1: Track-1 Clusters Confirmed. Available online at: https://www.gov.uk/government/publications/cluster-sequencing-for-carbon-capture-usage-and-storage-ccus-deployment-phase-1-expressions-of-interest/october-2021-update-track-1-clusters-confirmed (accessed November 9, 2021).
Bello, S., Galán-Martín, Á., Feijoo, G., Moreira, M. T., and Guillén-Gosálbez, G. (2020). BECCS based on bioethanol from wood residues: potential towards a carbon-negative transport and side-effects. Appl. Energy 279, 115884. doi: 10.1016/j.apenergy.2020.115884
BGS (2020). British Geological Survey - Our Data. Available online at: https://www.bgs.ac.uk/data/home.html (accessed March 17, 2020).
Brevik, P. (2017). The Full Scale CCS-Project at Norcem Brevik Can It Be Realised? Oslo: Norsk Industri.
BusinessWire (2019). Occidental Petroleum and White Energy to Study Feasibility of Capturing CO2 for Use in Enhanced Oil Recovery Operations. Available online at: https://www.businesswire.com/news/home/20180619005792/en/Occidental-Petroleum-White-Energy-Study-Feasibility-Capturing (accessed November 5, 2019).
Cadent Gas Ltd (2017). Review of Bioenergy Potential: Technical Report. Available online at: https://cadentgas.com/nggdwsdev/media/media/reports/futureofgas/Cadent-Bioenergy-Market-Review-TECHNICAL-Report-FINAL-amended.pdf
Calver, P., Mander, S., and Abi, D. (2022). Energy research & social science Low carbon system innovation through an energy justice lens: exploring domestic heat pump adoption with direct load control in the United Kingdom. Energy Res. Soc. Sci. 83, 102299. doi: 10.1016/j.erss.2021.102299
CCC (2020b). The Sixth Carbon Budget: The UK's path to Net Zero. Committee on Climate Change. London.
Chiesa, P., Consonni, S., Kreutz, T., and Williams, R. (2005). Co-production of hydrogen, electricity and CO2 from coal with commercially ready technology. Part A: performance and emissions. Int. J. Hydrogen Energy 30, 747–767. doi: 10.1016/j.ijhydene.2004.08.002
Clery, D. S., Vaughan, N. E., Forster, J., Lorenzoni, I., Gough, C. A., and Chilvers, J. (2021). Bringing greenhouse gas removal down to earth: stakeholder supply chain appraisals reveal complex challenges. Glob. Environ. Change 71, 102369. doi: 10.1016/j.gloenvcha.2021.102369
da Silva, F. T. F., Carvalho, F. M., Corrêa, J. L. G., Merschmann PR de, C., Tagomori, I. S., Szklo, A., et al. (2018). CO2 capture in ethanol distilleries in Brazil: designing the optimum carbon transportation network by integrating hubs, pipelines and trucks. Int. J. Greenhouse Gas Control 71, 168–183. doi: 10.1016/j.ijggc.2018.02.018
Dawood, F., Anda, M., and Shafiullah, G. M. (2020). Hydrogen production for energy: an overview. Int. J. Hydrog. Energy 45, 3847–3869. doi: 10.1016/j.ijhydene.2019.12.059
DECC (2012). Bioenergy Strategy Analytical Annex. Available online at: https://www.gov.uk/government/uploads/system/uploads/attachment_data/file/48338/5136-bioenergy-strategy-analytical-annex.pdf%5Cnhttps://www.gov.uk/government/publications/uk-bioenergy-strategy (accessed November 7, 2021).
DEFRA (2019). Farming Statistics Provisional Crop Areas, Yields and Livestock Populations. National Statistics. London 1–22.
Dixon, T. (2019). Positive Result on the London Protocol's CCS Export Amendment. IEAGHG. Available from: https://ieaghg.org/ccs-resources/blog/positive-result-on-the-london-protocol-s-ccs-export-amendment (accessed June 28, 2021).
Dolan, K. A., Stoy, P. C., and Poulter, B. (2020). Land management and climate change determine second-generation bioenergy potential of the US Northern Great Plains. GCB Bioenergy 12, 491–509. doi: 10.1111/gcbb.12686
Donnison, C., Holland, R. A., Hastings, A., Armstrong, L. M., Eigenbrod, F., and Taylor, G. (2020). Bioenergy with Carbon Capture and Storage (BECCS): finding the win–wins for energy, negative emissions and ecosystem services—size matters. GCB Bioenergy 12, 586–604. doi: 10.1111/gcbb.12695
ECN Biomass Energy Efficiency (2021). Phyllis2. Available online at: https://phyllis.nl/ (accessed November 9, 2021).
Emenike, O., Michailos, S., Finney, K. N., Hughes, K. J., Ingham, D., and Pourkashanian, M. (2020). Initial techno-economic screening of BECCS technologies in power generation for a range of biomass feedstock. Sustain. Energy Technol. Assess. 40, 100743. doi: 10.1016/j.seta.2020.100743
ETIP Bioenergy (2021). Bio-Hydrogen. Available online at: https://www.etipbioenergy.eu/value-chains/products-end-use/products/biohydrogen (accesesd June 5, 2021).
Fajardy, M., and Mac Dowell, N. (2018). The energy return on investment of BECCS: is BECCS a threat to energy security? Energy Environ. Sci. 11, 1581–1594. doi: 10.1039/C7EE03610H
Fantini, M. (2017). Biomass availability, potential and characteristics, in Biorefineries: Targeting Energy, High Value Products and Waste Valorisation, 21–54. Available online at: http://www.springer.com/series/8874
FCH (2020). Opportunities for Hydrogen Energy Technologies Considering the National Energy & Climate Plans. Brussels: Fuel Cells and Hydrogen.
Felgenhauer, M. F., Pellow, M. A., Benson, S. M., and Hamacher, T. (2016). Evaluating co-benefits of battery and fuel cell vehicles in a community in California. Energy 114, 360–368. doi: 10.1016/j.energy.2016.08.014
Forster, J., Vaughan, N. E., Gough, C., Lorenzoni, I., and Chilvers, J. (2020). Mapping feasibilities of greenhouse gas removal: Key issues, gaps and opening up assessments. Global Environ. Change. 63, 102073. doi: 10.1016/j.gloenvcha.2020.102073
Freer, M., Gough, C., Welfle, A., and Lea-langton, A. (2021). Carbon optimal bioenergy with carbon capture and storage supply chain modelling: How far is too far? Sustain. Energy Technol. Assess. 47, 101406. doi: 10.1016/j.seta.2021.101406
Gabrielli, P., Charbonnier, F., Guidolin, A., and Mazzotti, M. (2020). Enabling low-carbon hydrogen supply chains through use of biomass and carbon capture and storage: a Swiss case study. Appl. Energy 275, 115245. doi: 10.1016/j.apenergy.2020.115245
García-Freites, S., Gough, C., and Röder, M. (2021). The greenhouse gas removal potential of bioenergy with carbon capture and storage (BECCS) to support the UK's net-zero emission target. Biomass Bioenergy 151, 106164. doi: 10.1016/j.biombioe.2021.106164
Gerboni, R. (2016). Introduction to hydrogen transportation, in Compendium of Hydrogen Energy. Torino: Elsevier Ltd. p. 283–99.
Ghiat, I., AlNouss, A., McKay, G., and Al-Ansari, T. (2020). Biomass-based integrated gasification combined cycle with post-combustion CO2 recovery by potassium carbonate: techno-economic and environmental analysis. Comput. Chem. Eng. 135, 106758. doi: 10.1016/j.compchemeng.2020.106758
Global CCS Institute (2018). Bioenergy and Carbon Capture and Storage. Washington, DC: Global CCS Institute.
Global CCS Institute (2021). Global Status of CCS 2021: CCS Accelerating to Net Zero. Washington, DC.
Gondal, I. A. (2016). Hydrogen transportation by pipelines, in Compendium of Hydrogen Energy. Rawalpindi: Elsevier Ltd, 301–322.
Gough, C., Thornley, P., Mander, S., Vaughan, M., and Lea-Langton, A. (2018). Biomass Energy with Carbon Capture and Storage (BECCS): Unlocking Negative Emissions (Manchester: John Wiley & Sons Ltd.), 119–127.
Greenberg, S. E., Bauer, R., Will, R., Locke, R., Carney, M., Leetaru, H., et al. (2017). Geologic carbon storage at a one million tonne demonstration project: lessons learned from the illinois basin - decatur project. Energy Proc. 114, 5529–5539. doi: 10.1016/j.egypro.2017.03.1913
Hakl, J., Hrevušová, Z., Hejcman, M., and Fuksa, P. (2012). The use of a rising plate meter to evaluate lucerne (Medicago sativa L.) height as an important agronomic trait enabling yield estimation. Grass Forage Sci. 67, 589–596. doi: 10.1111/j.1365-2494.2012.00886.x
Harrison, G. P., Maclean, E. J., Karamanlis, S., and Ochoa, L. F. (2010). Life cycle assessment of the transmission network in Great Britain. Energy Policy 38, 3622–3631. doi: 10.1016/j.enpol.2010.02.039
HM Government (2020a). Find Your Open Data. Available online at: https://data.gov.uk/ (accessed March 18, 2020).
HM Government (2020b). The Ten Point Plan for a Green Industrial Revolution. London: Department for Business, Energy and Industrial Strategy.
IEA (2008). Co-Production of Hydrogen and Electricity by Coal Gasification with CO2 Capture – Updated Economic Analysis. Available online at: www.ieagreen.org.uk (accessed October 10, 2021).
IPCC (2018). Global Warming of 1.5°C: An IPCC Special Report on the Impacts of Global Warming of 1.5°C Above Pre-industrial Levels and Related Global Greenhouse Gas Emission Pathways, in the Context of Strengthening the Global Response to the Threat of Climate Change. Geneva.
IPCC (2021).Climate change 2021: the physical science basis, in Contribution of Working Group I to the Sixth Assessment Report of the Intergovernmental Panel on Climate Change, editors Masson-Delmotte, V., Zhai, P., Pirani, A., Connors, S. L., Péan, C., Berger, S., Caud, N., and Chen, Y., (Geneva: Cambridge University Press), 3949.
Jin, M., Mackay, E., Akhurst, M., Hitchen, K., and Quinn, M. (2012). Evaluation of the CO2 storage capacity of the captain sandstone formation, in 74th European Association of Geoscientists and Engineers Conference and Exhibition 2012 Incorporating SPE EUROPEC 2012: Responsibly Securing Natural Resources.
Jones, A. R., Raja Segaran, R., Clarke, K. D., Waycott, M., Goh, W. S. H., and Gillanders, B. M. (2020). Estimating mangrove tree biomass and carbon content: a comparison of forest inventory techniques and drone imagery. Front. Marine Sci. 6, 1–13. doi: 10.3389/fmars.2019.00784
Kemper, J. (2017). IEAGHG: Biomass With Carbon Capture and Storage (BECCS/Bio-CCS). Cheltenham: IEAGHG.
Krause, A., Knoke, T., and Rammig, A. (2020). A regional assessment of land-based carbon mitigation potentials: bioenergy, BECCS, reforestation, and forest management. GCB Bioenergy 12, 346–360. doi: 10.1111/gcbb.12675
Kuo, P. C., and Wu, W. (2015). Design, optimization and energetic efficiency of producing hydrogen-rich gas from biomass steam gasification. Energies 8, 94–110. doi: 10.3390/en8010094
Lask, J., Rukavina, S., Zori,ć, I., Kam, J., Kiesel, A., Lewandowski, I., et al. (2021). Lignocellulosic ethanol production combined with CCS—A study of GHG reductions and potential environmental trade-offs. GCB Bioenergy 13, 336–347. doi: 10.1111/gcbb.12781
Laude, A., Ricci, O., Bureau, G., Royer-Adnot, J., and Fabbri, A. (2011). CO2 capture and storage from a bioethanol plant: carbon and energy footprint and economic assessment. Int. J. Greenhouse Gas Control 5, 1220–1231. doi: 10.1016/j.ijggc.2011.06.004
Melara, A. J., Singh, U., and Colosi, L. M. (2020). Is aquatic bioenergy with carbon capture and storage a sustainable negative emission technology? Insights from a spatially explicit environmental life-cycle assessment. Energy Conv. Manage. 224, 113300. doi: 10.1016/j.enconman.2020.113300
Mendiara, T., Pérez-Astray, A., Izquierdo, M. T., Abad, A., de Diego, L. F., García-Labiano, F., et al. (2018). Chemical looping combustion of different types of biomass in a 0.5 kWth unit. Fuel. 211, 868–875. doi: 10.1016/j.fuel.2017.09.113
Moldenhauer, P., Linderholm, C., Rydén, M., and Lyngfelt, A. (2020). Avoiding CO2 capture effort and cost for negative CO2 emissions using industrial waste in chemical-looping combustion/gasification of biomass. Mitigat. Adapt. Strateg. Glob. Change 25, 1–24. doi: 10.1007/s11027-019-9843-2
Moreira, J. R., Romeiro, V., Fuss, S., Kraxner, F., and Pacca, S. A. (2016). BECCS potential in Brazil: achieving negative emissions in ethanol and electricity production based on sugar cane bagasse and other residues. Appl. Energy 179, 55–63. doi: 10.1016/j.apenergy.2016.06.044
Muresan, M., Cormos, C. C., and Agachi, P. S. (2013). Techno-economical assessment of coal and biomass gasification-based hydrogen production supply chain system. Chem. Eng. Res. Design 91, 1527–1541. doi: 10.1016/j.cherd.2013.02.018
Negri, V., Galán-Martín, Á., Pozo, C., Fajardy, M., Reiner, D. M., Mac Dowell, N., et al. (2021). Life cycle optimization of BECCS supply chains in the European Union. Appl. Energy 298:117252. doi: 10.1016/j.apenergy.2021.117252
Newnham, G. J., Grant, I. F., Martin, D. N., and Anderson, S. A. J. (2010). Improved methods for assessment and prediction of grassland curing, Satellite Based Curing Methods and Mapping. Final Report: Project A, Vol. 1.
Norwegian Ministry of Petroleum and Energy (2016a). Summary – Feasibility Studies - CCS Project in Norway. Oslo.
Norwegian Ministry of Petroleum and Energy (2016b). Feasibility Study for Full-Scale CCS in Norway. Oslo: Ministry of Petroleum and Energy.
Occidental Petroleum Organisation (2017). Occidental Petroleum Corporation: Leader In CO2 EOR & CCUS Deployment. Los Angeles, CA.
Pale Blue Dot (2016). Progressing Development of the UK's Strategic Carbon Dioxide Storage Resource: A Summary of Results From the Strategic UK CO2 Storage Appraisal Project. Aberdeen.
Pale Blue Dot (2020). CO2 Stored Database. Aberdeen. Available online at: http://www.co2stored.co.uk/home/index (accessed February 20, 2020).
Patzschke, C. F., Bahzad, H., Boot-Handford, M. E., and Fennell, P. S. (2020). Simulation of a 100-MW solar-powered thermo-chemical air separation system combined with an oxy-fuel power plant for bio-energy with carbon capture and storage (BECCS). Mitigat. Adapt. Strategies Glob. Change 25, 539–557. doi: 10.1007/s11027-019-09879-0
Pour, N., Webley, P. A., and Cook, P. J. (2018). Potential for using municipal solid waste as a resource for bioenergy with carbon capture and storage (BECCS). Int. J. Greenhouse Gas Control 68, 1–15. doi: 10.1016/j.ijggc.2017.11.007
Rashid, M. M., Mesfer, M. K., Al Naseem, H., and Danish, M. (2015). Hydrogen production by water electrolysis: a review of alkaline water electrolysis, PEM water electrolysis and high temperature water electrolysis. Int. J. Eng. Adv. Technol. 4, 2249–8958. doi: 10.1016/j.mset.2019.03.002
Rosa, L., Sanchez, D. L., and Mazzotti, M. (2021). Assessment of carbon dioxide removal potential: Via BECCS in a carbon-neutral Europe. Energy Environ. Sci. 14, 3086–3097. doi: 10.1039/D1EE00642H
Sagues, W. J., Jameel, H., Sanchez, D. L., and Park, S. (2020). Prospects for bioenergy with carbon capture & storage (BECCS) in the United States pulp and paper industry. Energy Environ. Sci. 13, 2243–2261. doi: 10.1039/D0EE01107J
Sanchez, D. L., and Callaway, D. S. (2016). Optimal scale of carbon-negative energy facilities. Appl. Energy 170, 437–444. doi: 10.1016/j.apenergy.2016.02.134
Scarlat, N., Fahl, F., and Dallemand, J. F. (2019). Status and opportunities for energy recovery from municipal solid waste in Europe. Waste Biomass Valoriz. 10, 2425–2444. doi: 10.1007/s12649-018-0297-7
Stockholm Exergi (2021). Bio-CCS – Considerable Potential. Available online at: https://www.stockholmexergi.se/om-stockholm-exergi/about-stockholm-exergi/negative-emissions/bio-ccs/#::~text=Bio-CCS is one possible,the Värtan area of Stockholm (accessed October 14, 2021).
Summit Carbon Solutions (2021). Growth Energy Carbon Spotlight. Available online at: https://www.summitcarbonsolutions.com/news/growthenergycarbonspotlight (accessed October 14, 2021).
Tagomori, I. S., Rochedo, P. R. R., and Szklo, A. (2019). Techno-economic and georeferenced analysis of forestry residues-based Fischer-Tropsch diesel with carbon capture in Brazil. Biomass Bioenergy 123, 134–148. doi: 10.1016/j.biombioe.2019.02.018
Toshiba (2020). Toshiba Starts Operation of Large-Scale Carbon Capture Facility. Available online at: https://www.toshiba-energy.com/en/info/info2020_1031.htm (accessed October 14, 2021).
Townsend, T. J., Sparkes, D. L., Ramsden, S. J., Glithero, N. J., and Wilson, P. (2018). Wheat straw availability for bioenergy in England. Energy Policy 122, 349–357. doi: 10.1016/j.enpol.2018.07.053
UK Forestry Commission (2021). Forestry Yields. Available online at: https://www.forestresearch.gov.uk/tools-and-resources/fthr/forest-yield/ (accessed November 24, 2021).
UKCEH (2019). UKCEH Land Cover® plus: Crops. Available online at: https://www.ceh.ac.uk/crops2015 (accessed October 22, 2020).
Victorin, M., Davidsson, Å., and Wallberg, O. (2020). Characterization of mechanically pretreated wheat straw for biogas production. Bioenergy Res. 13, 833–844. doi: 10.1007/s12155-020-10126-7
Welfle, A., Gilbert, P., and Thornley, P. (2014a). Increasing biomass resource availability through supply chain analysis. Biomass Bioenergy 70, 249–266. doi: 10.1016/j.biombioe.2014.08.001
Welfle, A., Gilbert, P., and Thornley, P. (2014b). Securing a bioenergy future without imports. Energy Policy 68, 1–14. doi: 10.1016/j.enpol.2013.11.079
Wendt, L. M., and Zhao, H. (2020). Review on bioenergy storage systems for preserving and improving feedstock value. Front. Bioeng. Biotechnol. 8, 370. doi: 10.3389/fbioe.2020.00370
Williams, J. D. O., Fellgett, M. W., and Quinn, M. F. (2016). Carbon dioxide storage in the Captain Sandstone aquifer: Determination of in situ stresses and fault-stability analysis. Petroleum Geosci. 22, 211–222. doi: 10.1144/petgeo2016-036
Xing, X., Wang, R., Bauer, N., Ciais, P., Cao, J., Chen, J., et al. (2021). Spatially explicit analysis identifies significant potential for bioenergy with carbon capture and storage in China. Nat. Commun. 12, 1–12. doi: 10.1038/s41467-021-23282-x
Yi, Q., Zhao, Y., Huang, Y., Wei, G., Hao, Y., Feng, J., et al. (2018). Life cycle energy-economic-CO2 emissions evaluation of biomass/coal, with and without CO2 capture and storage, in a pulverized fuel combustion power plant in the United Kingdom. Appl. Energy 225, 258–272. doi: 10.1016/j.apenergy.2018.05.013
Yu, M., Wang, K., and Vredenburg, H. (2021). Insights into low-carbon hydrogen production methods: green, blue and aqua hydrogen. Int. J. Hydrogen Energy 46, 21261–21273. doi: 10.1016/j.ijhydene.2021.04.016
Zero Carbon Humber (2020). Leading Energy Companies Form Partnership to Accelerate the Development of Offshore Transport and Storage Infrastructure for Carbon Emissions in UK North Sea. Scunthorpe.
ZeroCarbonHumber (2019). Capture for Growth: A Roadmap for the World's First Zero Carbon Industrial Cluster. A Roadmap for the World's First Zero-Carbon Industrial Cluster. Scunthorpe: Zero Carbon Humber.
Zhang, D., Bui, M., Fajardy, M., Patrizio, P., Kraxner, F., and Mac Dowell, N. (2019). Unlocking the potential of BECCS with indigenous sources of biomass at a national scale. Sustain. Energy Fuels 4, 226–253. doi: 10.1039/C9SE00609E
Zhang, X., Shen, J., Saini, P. K., Lovati, M., Han, M., Huang, P., et al. (2021). Digital twin for accelerating sustainability in positive energy district: a review of simulation tools and applications. Front. Sustain. Cities 3, 663269. doi: 10.3389/frsc.2021.663269
Keywords: BECCS, negative emissions, supply chains, macro-energy systems, GIS, carbon-optimal transportation
Citation: Freer M, Gough C, Welfle A and Lea-Langton A (2022) Putting Bioenergy With Carbon Capture and Storage in a Spatial Context: What Should Go Where? Front. Clim. 4:826982. doi: 10.3389/fclim.2022.826982
Received: 01 December 2021; Accepted: 07 February 2022;
Published: 07 March 2022.
Edited by:
Mijndert Van Der Spek, Heriot-Watt University, United KingdomReviewed by:
Daniel L. Sanchez, University of California, Berkeley, United StatesMarcelo Galdos, University of Leeds, United Kingdom
Christian Moretti, ETH Zürich, Switzerland
Copyright © 2022 Freer, Gough, Welfle and Lea-Langton. This is an open-access article distributed under the terms of the Creative Commons Attribution License (CC BY). The use, distribution or reproduction in other forums is permitted, provided the original author(s) and the copyright owner(s) are credited and that the original publication in this journal is cited, in accordance with accepted academic practice. No use, distribution or reproduction is permitted which does not comply with these terms.
*Correspondence: Muir Freer, muir.freer@manchester.ac.uk