- 1Middle Path EcoSolutions, Boulder, CO, United States
- 2The Ronin Institute, Montclair, NJ, United States
- 3Independent Scholar, New York, NY, United States
- 4Department of Forestry, North Carolina State University, Raleigh, NC, United States
- 5Bureau of Ocean Energy Management, Washington, DC, United States
- 6School of Information, University of Michigan, Ann Arbor, MI, United States
Community resilience increases a place-based community's capacity to respond and adapt to life-changing environmental dynamics like climate change and natural disasters. In this paper, we aim to support Earth science's understanding of the challenges communities face when applying Earth science data to their resilience efforts. First, we highlight the relevance of Earth science in community resilience. Then, we summarize these challenges of applying Earth science data to community resilience:
• Inequity in the scientific process,
• Gaps in data ethics and governance,
• A mismatch of scale and focus, and
• Lack of actionable information for communities.
Lastly, we offer the following recommendations to Earth science as starting points to address the challenges presented:
• Integrate community into the scientific data pathway,
• Build capacity to bridge science and place-based community needs,
• Reconcile openness with self-governance, and
• Improve access to data tools to support community resilience.
Introduction
Climate change, natural disasters, and public health threats test the durability of our society. A place-based community's capacity to respond to and recover from life-changing events is called community resilience. Science plays a key role in providing evidence that people and groups can use to make informed decisions about their community's resilience. Earth science data, which are qualitative and quantitative products of observation representing properties of objects, events, and their environments (Rowley, 2007), have been historically produced, curated, and managed for use by scientists. Due to the nature of Earth science data, data support has been mostly provided by and restricted to large institutions with access to high computing power (Dutton et al., 1995; Ramapriyan and Behnke, 2019). However, the societal, political, technical, and cultural landscape around the meaning and use of data is changing. There are growing expectations in society for more open data (ESIP interview: Mayernik and Virapongse, 2019). Decision-makers, such as practitioners, planners, industry leaders, and the general public, seek to leverage scientific data and information to develop new tools, make decisions, and act. There is still much work to be done by Earth scientists and their data science partners to address issues of data access and use by decision-makers (Wee and Piña, 2019), and particularly for community resilience.
The Earth Science Information Partners (ESIP) Community Resilience cluster addresses how Earth science data can be better utilized to support community resilience. Over the past 5 years, members of the Community Resilience cluster (co-authors of this paper) have been leading discussions within ESIP on this topic during ESIP's biannual meetings, through monthly teleconferencing calls, and across other ESIP clusters. With over 150 member organizations, ESIP includes individuals from federal, state, and local government, non-profit organizations, and the private sector. The discussions that we had through these activities greatly informed this paper.
In this paper, we aim to facilitate the contribution of Earth science data to community resilience efforts by:
• summarizing challenges related to how data and information are accessed, trusted, and made actionable for the purposes of community resilience,
• framing how data use and community resilience can work together, and
• presenting recommendations to address the challenges.
We pose the question: How can Earth science data and information be used more effectively to enhance community resilience? We aim for this paper to be useful for people that generate, analyze, and manage Earth science data, support the translation of scientific data to information, and apply science-based information for societal benefit.
Community Resilience
Community resilience functions within a complex social-ecological system, where people, environment, climate, and other entities interact. In this context, “community” is geographically localized (place-based), while referring to the social interactions that occur among people in a place (Theodori, 2005). Communities can be defined by geopolitical units (e.g., town or neighborhood), social groupings (e.g., demographic profile, social class structure, culture, language), and entities organized around special interests. We draw on a definition of “community resilience” that describes it as the capacity of a system to prevent, adapt, and recover from shocks and stressors so that it grows stronger and is more prepared in the future [United States Agency for International Development (USAID), 2012]. This framing enables us to conceptualize “community resilience” expansively to consider a community's capacity to effectively respond to natural hazards such as flooding, wildfires, and earthquakes (e.g., Cutter et al., 2013), human-caused disasters such as mass shootings (e.g., Aldrich and Meyer, 2015), as well as systemic trauma experienced by indigenous people through repression and colonization (e.g., Kirmayer et al., 2009). While climate resilience in response to adverse climate events has been a recent focus in the literature, our definition encompasses it, acknowledging the multiple scenarios that communities must cope with in the face of external challenges. Our thesis in this paper considers this broader focus of community resilience, with recommendations that can be applied across different contexts and circumstances.
Building the capacity and flexibility of a community to adapt to an ever-changing socio-environment is central to improving community resilience (Magis, 2010). In application, community resilience goals help to frame and guide local- to global-scale decision-making to improve human livelihoods, address environmental change, and prepare communities to cope with hazards, risks, and disasters (PCAST-Executive Office of the President, 2011; Cutter et al., 2013; Bone et al., 2016). Environmental justice goals can also be addressed through community resilience efforts aiming to reduce inequitable exposure from toxic waste in industrial waterfront areas and improving residents' health and quality of life (Bautista et al., 2015); a community's resilience is dependent on the strength of the entire social-ecological system as a whole. Improving community resilience involves accounting for future change and uncertainty in decision-making, including assessing when resisting change is beneficial or detrimental, and developing plans that allow flexibility as needed. To effectively impact a community's resilience, decision-making must be informed and empowered at multiple socio-political scales (including individual, city, and national levels) and across sectors (including community members, private businesses, and government stakeholders) (Table 1).
Navigating change to community resilience is often framed within an adaptive cycle, which posits that growth is not constant and reorganization is required to maintain the community as a functioning entity (Fath et al., 2015). This emphasizes the need for communities to adapt to and build capacity for a changing suite of problems, while being aware of common pitfalls that they may experience when responding to disturbances. Efforts to create a more resilient community often require addressing the tension between the inherent qualities of a system (e.g., physical and ecological structure, function, or states) and the values that people (and often specific groups of people) associate with different components of a system (Higuera et al., 2019). Community resilience has been used to address industry changes (King, 2008), diminishing natural resources (Smith et al., 2012), climate change (Adger et al., 2005; Funfgeld and McEvoy, 2012), health crises (Chandra et al., 2011), health and wellbeing of indigenous people (Kirmayer and Valaskakis, 2009), forest fires (McWethy et al., 2019), and environmental management (Virapongse et al., 2016). Fundamental concepts of resilience can be operationalized to enhance a community's ability to use available Earth science data and information for their benefit.
Data Challenges in Community Resilience
Closing the gap between community resilience needs and Earth science data is an ongoing, multifaceted challenge. While we note some well-known barriers that already have relatively extensive literature describing these issues (for example, downscaling), we concentrate primarily on aspects that are less well-studied.
Inequity in the Scientific Process
Without equitable representation in the scientific process, it is unlikely that the resilience needs of communities—particularly among those segments of the population that are historically underrepresented across society—will be sufficiently addressed. Scientific bodies, composed of academics and professionals, largely determine who asks questions and makes decisions in science. They decide the objectives of research, the purpose and methods for data collection, the types of data products created, and what research should be funded. Inequities and lack of representation (e.g., race, ethnicity, class, political perspective; Funk, 2012) among scientific decision-makers increases the likelihood that scientific narratives and science agendas are biased and perceived as untrustworthy. Indeed, the stakes are high: scientific decision-making can determine the priority placed on issues and the types of information and knowledge generated to address them, potentially depriving more socially vulnerable groups the right to scientific benefits (Klinsky et al., 2017).
The harmful societal impact of such power discrepancies in decision-making has been recognized in sectors like environmental conservation in the US, where it is increasingly apparent that conservation agendas are being drawn from a primarily White perspective (Green2.0, 2021). Similarly, STEM disciplines (e.g., geoscience) have historically created systemic barriers and thwarted success (e.g., hostile work environments, limited access to resources and opportunities) for researchers minoritized due to their race, ethnicity, gender identity, sexual orientation, and other aspects of their identities (e.g., Berhe et al., 2022). In terms of topical areas, climate research is one where such inequities exist in production as well as implementation for impact. For example in the global context, the majority of climate change research is conducted within the developed world (Tai and Robinson, 2018), even though it is well established that climate impacts less developed countries disproportionately more (IPCC, Allen et al., 2014). The latest Intergovernmental Panel on Climate Change report further documents the miniscule amount of funding available for climate-related research in Africa, despite worsening impacts of a warmer climate in the continent such as biodiversity loss, droughts, reduced crop productivity, and economic growth (Trisos et al., 2022, Chapter 9, pp. 9–18).
Poor representation in science perpetuates environmental injustice (“a situation in which a specific social group is disproportionately affected by environmental hazards”) (Brulle and Pellow, 2006). A well-known example of environmental injustice is the drinking water crisis in Flint, Michigan, which the ESIP Community Data cluster (Diggs et al., 2021) examined as a case study for the role of Earth Science data in environmental justice. As a predominantly Black and socioeconomically depressed community, Flint represents one of the most disadvantaged and marginalized groups in our society and in the scientific process. The failure of the government to provide adequate drinking water protections resulted in disastrous health impacts and further erosion of trust between communities of color and the local, state, and federal U.S. government. With underrepresentation in the regulatory decision making process, including any advocates for them, it was extremely challenging for the Flint community to convince governmental agencies to address water contamination issues, despite the alarm being raised within the community (Butler et al., 2016). After attention was drawn to the issue by the U.S. Environmental Protection Agency (EPA), further deception of the EPA by state regulators eventually led to criminal charges (Butler et al., 2016). It also became evident that data collection protocols to test and monitor drinking water were willfully ignored or misinterpreted–not only in Flint but in other underserved communities in the US as well (Balazs and Ray, 2014; Katner et al., 2016). The Flint example highlights how scientists supporting government decision-making must recognize and mitigate the challenges that exist in applying the scientific process in communities striving for environmental justice.
Fundamental issues regarding ownership of and access to scientific data and information exacerbate inequity in the scientific process by limiting who can use data and information. Despite the fact that many scientific datasets are at least partially funded by place-based communities (e.g., via taxes), much of the scientific literature that reports Earth science research findings is published in journals that are inaccessible to the public or behind paywalls that are insurmountable by marginalized communities and nations. Open licensing presents its own unique set of challenges regarding the ownership of federally funded research data (Khayyat and Bannister, 2015). Moreover, even when the data and literature are available, it isn't always clear that results are easily and broadly usable by communities. While progress toward public access to data continues to be incremental, the equitable, technical, and legal challenges associated with data inhibit the societal benefit of science.
Social structures inherent in science create rigidity in the scientific process that inhibits the due consideration of stake and rights holder input, and the equitable distribution of benefits from science. These are especially pronounced given the social inequities that exist in society, and the fact that science has significant barriers to overcome with its implicit biases, including learning to see how inequities show up within the scientific establishment (Tanner, 2009). The question is, who do scientists take an oath to when performing their duties? Are they serving science and the continuation of its norms and practices? Community resilience applications offer an option to help scientists understand who science is being performed for, so the due representation of disadvantaged and historically underrepresented communities in the scientific process can be improved. Only by adapting the scientific process to the community context, can science address these inequities.
Gaps in Data Ethics and Governance
Improved community resilience relies on data that authentically represents the specific context and needs of a place-based community. Collecting such data, however, can be fraught and contested if people are not able to control their data and trust how it will be used. In this section, we focus on the ethical lapses that occur when managing, using, and reusing data products. Data governance entails formalizing ethical processes to ensure data are correctly managed after collection–this can include data preparation, maintenance, and security (Thompson et al., 2015).
In the community resilience context, data governance can include community members designing restrictions on data collection and use, and assigning responsibility for collecting, maintaining, and protecting data in alignment with their cultural identity. This is particularly crucial for indigenous peoples and other racial and ethnic minority groups and the institutions within which they are embedded (Smith, 2016). The ability of groups to control their own data has been defined as part of the right to self-determination as outlined in the United Nations Declaration on the Rights of Indigenous Peoples (UN General Assembly, 2007). The authority to control data collection and use are also components of more recent CARE Principles for Indigenous Data Governance (Carroll et al., 2020).
Data reuse can be ethically problematic. Interpretation and reuse of data by third parties can be harmful if such use is uninformed by the scientific and cultural context or selective in its analysis of available data (Reimsbach-Kounatze, 2021). Many datasets lack the information needed to inform data users about how data should or should not be used. For example, the U.S. Bureau of Land Management (BLM) collected oral histories from indigenous elders in Alaska Arctic boroughs in order to establish indigenous rights to federal lands. The BLM controlled access to the data for many years despite it being a valuable source of cultural information for the associated communities (Pratt, 2004).
Systematic data collection from disenfranchized communities can be intertwined with discrimination, reinforcing narratives that may be detrimental to the communities themselves. In addition, while data on their own may not disclose sensitive information, they often can be combined with other data to unintentionally or intentionally reveal sensitive information about vulnerable populations. A broadly cited example is the re-identification of individuals' names and addresses by piecing together multiple de-identified environmental quality datasets from a public health study (Sweeney et al., 2017). The availability of spatial data can similarly present a problem, such as when personal information is unintentionally re-identified through a geographic information system (GIS; Scassa, 2010).
Existing global inequality is perpetuated by data injustices through surveillance, economic exploitation, algorithmic profiling, and loss of the right to privacy (Heeks and Renken, 2018). Globally, approximately 19% of countries have no data protection laws, leaving their residents acutely vulnerable to personal security breaches and algorithmic prejudice (UNCTAD DPR., 2016; UNCTAD, 2020). Refugees are even more vulnerable. They lack access, control, and protection of their data, since they may not have rights within an asylum country to their data or be defended by the country they leave (Rolan et al., 2020). As the collection, storage, sharing, and use of Earth science data are increasingly facilitated by technological advances, ethical advances must also keep pace to ensure that the interests of vulnerable communities are adequately protected.
Despite recent interest in co-production of scientific knowledge between producers and users of science (e.g., Lemos et al., 2018; Jagannathan et al., 2020), the literature remains largely theoretical without addressing the specific challenges we have described in this section (e.g., the step-by-step process of co-development and relationship). While there are promising trends to be more inclusive of communities in co-developed climate services (i.e., customized products such as forecasts and predictions), climate adaptation projects, environmental decision making, and environmental sustainability projects (Kirchhoff et al., 2013; Laursen et al., 2018; Bremer et al., 2019; Mach et al., 2020), many projects still fail to authentically address the inequitable power dynamics that are inherent in the processes of collaboration. For initiatives aiming to enhance community resilience in response to external events, including but not limited to climate-related damage, the gap in skills, capacities, and awareness needed for data producers to build trust with users to enable co-creation continues to be a limitation.
A Mismatch of Scale and Focus
Earth science research and place-based communities often work on different time and spatial scales, as well as have differing needs and goals for data collection. Community resilience decision-makers need data to make urgent and consequential decisions, while addressing multiple spatial and temporal scales (Table 1) and the needs of diverse stakeholders. An example of such tradeoffs are near- and long-term economic stability, or a city vs. an individual's exposure to climate risks (Chelleri et al., 2015).
Earth science data products that are needed for community resilience planning (e.g., climate projections, Earth systems modeling) are often produced at vast spatial and temporal scales, which are relevant to the understanding of long-term natural phenomena (Kirchmeier-Young et al., 2019). Yet, communities and industries often require data products that incorporate long-term trends with more actionable near-term, higher accuracy data (Vera et al., 2010; Dunn et al., 2015). Therefore, additional research and processing of Earth science data are needed to make these larger-scaled data more appropriate for community-level resilience needs (Bhuvandas et al., 2014), but many communities lack such data processing capacity (O'Neill, 2011). Without correctly scaling data and information, decision-makers may be taking (or not taking) actions that ultimately reduce their community's resilience. For example, Earth science phenomena described at a coarse scale (i.e., large areas represented as uniform depicted with lower resolution) may have directionally opposite effects at a local scale (Keskitalo et al., 2016). The lack of appropriately scaled Earth science data can limit its use to community resilience efforts for larger geopolitical units (such as nations), while local communities are left out of such efforts.
While the previously described issue of downscaling is well-addressed in literature, there are other kinds of scale mismatches. One is illustrated by the 2012 U.S. National Science Foundation (NSF) sponsored 2nd Semantic Sea Ice Interoperability Initiative (SIII)—a workshop that brought together Alaskan indigenous sea ice experts, National Oceanic and Atmospheric Administration (NOAA) specialists, and researchers around the topic of sea ice. Some members of indigenous communities of the Arctic are sea ice experts. However, their ability to understand and predict sea-ice behavior has been becoming increasingly strained by climate change. For years, NOAA had been providing sea ice charts, which provide a low resolution but comprehensive picture of sea ice in the Arctic. These charts are based on a variety of much higher resolution data, including Synthetic Aperture RADAR (SAR) full-resolution imagery; imagery that is too large to be transmitted to indigenous coastal villages given their available bandwidth. During the workshop, members of the indigenous community suggested producing a cropped SAR data product covering only the sea ice within a few miles of their community. Such a product would be useful for making decisions about travel and food harvesting. Existing ice charts and SAR products extend for 100 miles or more, far outside the bounds that a typical hunter would travel, thus providing many times more data than are useful at the community level. A cropped data product, however, would fit within the bandwidth limitations of the communities and could be directly compared to what community members could see from shore and on shore fast ice; an insight that NOAA data managers appreciated and acted upon.
Scientific research is often organized as individual research projects (i.e., principal investigator-led projects) that range from global efforts to local groups focusing on a single domain area. While there is an assumption that the results of different research projects will naturally inform each other, the reality is that these connections often fail to form, resulting in a splintered scientific approach that lacks place-based synthesis and fails to address emerging disasters (Cutter et al., 2013). For example, scientists studying rare earthquakes and floods do not automatically integrate their research with those studying modern disaster response or engineering cities (Ismail-Zadeh et al., 2016). In addition, the relationships between the projects may be too weak to support upward scaling of their goals and extrapolation from their results (Taylor, 1984; Parsons et al., 2011). Data products may neglect cross-scale relationships and address communities as if they are stand-alone and isolated entities (Sharifi, 2016), or provide sweeping results that are not fine-grained enough for tangible and useful decision-making. In contrast, information useful for community resilience must be well-coordinated across various scales to accurately assess risk, illuminate knowledge gaps and solutions, and communicate hazards. The information available to decision-makers depends on the strength of the interactions between data creators operating at multiple scales (Pulsifer et al., 2020). Better interaction across scales of this “information ecosystem” can improve data for interdisciplinary, systemic research (Parsons et al., 2011), such as for community resilience.
Lack of Actionable Information for Communities
Earth science data are often created for a particular science community with the expectation that others will find a way to use them (Baker et al., 2015). The lack of attention given to the nuanced needs and worldviews of specific groups that could use Earth science data contributes to this challenge (Bhargava and Manoli, 2015). For example, different communities use different terminology, which impacts how information can reach and influence people, and in turn impacts their knowledge development (Eitzel et al., 2017). Further, existing power dynamics in knowledge generation often undermine data and knowledge that originate from outside of conventional scientific frameworks, such as among traditional and tacit knowledge systems (Brun and Schumacher, 1987; Roux et al., 2006; Dunlop, 2009). Often this issue is framed as a lack of data literacy, which implies that the solution involves improving a community's ability to read, work with, analyze, and argue with data (D'Ignazio and Bhargava, 2016). The problem with this framing is that all of the responsibility for “learning” about data is placed on community members, rather than Earth science researchers making efforts to provide data and information that are useful for those communities.
The application of Earth science data toward societal benefit is often conceptualized as a data, information, knowledge, and wisdom (DIKW) pathway (Ackoff, 1989; Sharma, 2008). This DIKW pathway emphasizes the one-directionality of data to application. An oversimplified and idealized version of the climate change discourse illustrates an example of the DIKW pathway from data to societal benefit: (1) Earth scientists notice trends and describe climate change to their peers as part of their typical scientific discourse, (2) scientists communicate technical information about climate change outside their disciplines, and their implications become evident in closely allied fields, (3) climate change begins to be recognized broadly across a number of disciplines and by a subset of the general public, and (4) climate change is now a central part of public policy discourse with analysis occurring cross-sectorally. There are many assumptions within this process, such as the trickling effect of scientific knowledge into the general public. However, it serves as a useful example of how scientists might envision how their data efforts contribute to societal benefit through the DIKW pathway.
Figure 1 depicts the data equivalent of such a DIKW pathway, where source data is processed through a number of steps into intermediate data products that become publicly accessible final data products to be interpreted for community consumption. Even with this simple, linear model it is clear that unless all of the products along the path are continuously funded and available, neither the final data products nor their interpretations will be available for any community to consume.
An unexplored assumption behind long-term investments in data management, and in today's policy and decision making context, is that the DIKW pathway justifies the initial investment and creation of structured data, for example, climate data records (Meier et al., 2021), even when existing knowledge frameworks are too rigid to address today's pressing needs and priorities. Indeed, the example of the climate change information pathway demonstrates how much easier it is to address an Earth science problem from one worldview, rather than taking on the challenge of considering the complex and nuanced perspectives of multiple user communities. The challenge here is to present more accurate conceptual frameworks that demonstrate the role of users (community) in the data pathway.
In reality, the Earth science data “pathway” is often more like a “network system” (Li and Whalley, 2002) with multiple intended products stemming from data sets or combined data sets, and people who play multiple roles on the path and in the network. Figure 2 depicts a fragment of an existing data product network currently available at the National Snow and Ice Data Center (NSIDC). Data products that are tailored to support a particular user group's needs have potential for greater use and applicability by that user group, in contrast to data products that are developed without a specific end user in mind. However, creation of such downstream data and interpretive products for end users can be challenging. Baker et al. (2015) describe a situation where data products created by a specific Earth science community (e.g., the sea ice scientific community) were confusing and unhelpful for various groups outside of that community, leading to a process of data development that continued for more than a dozen years.
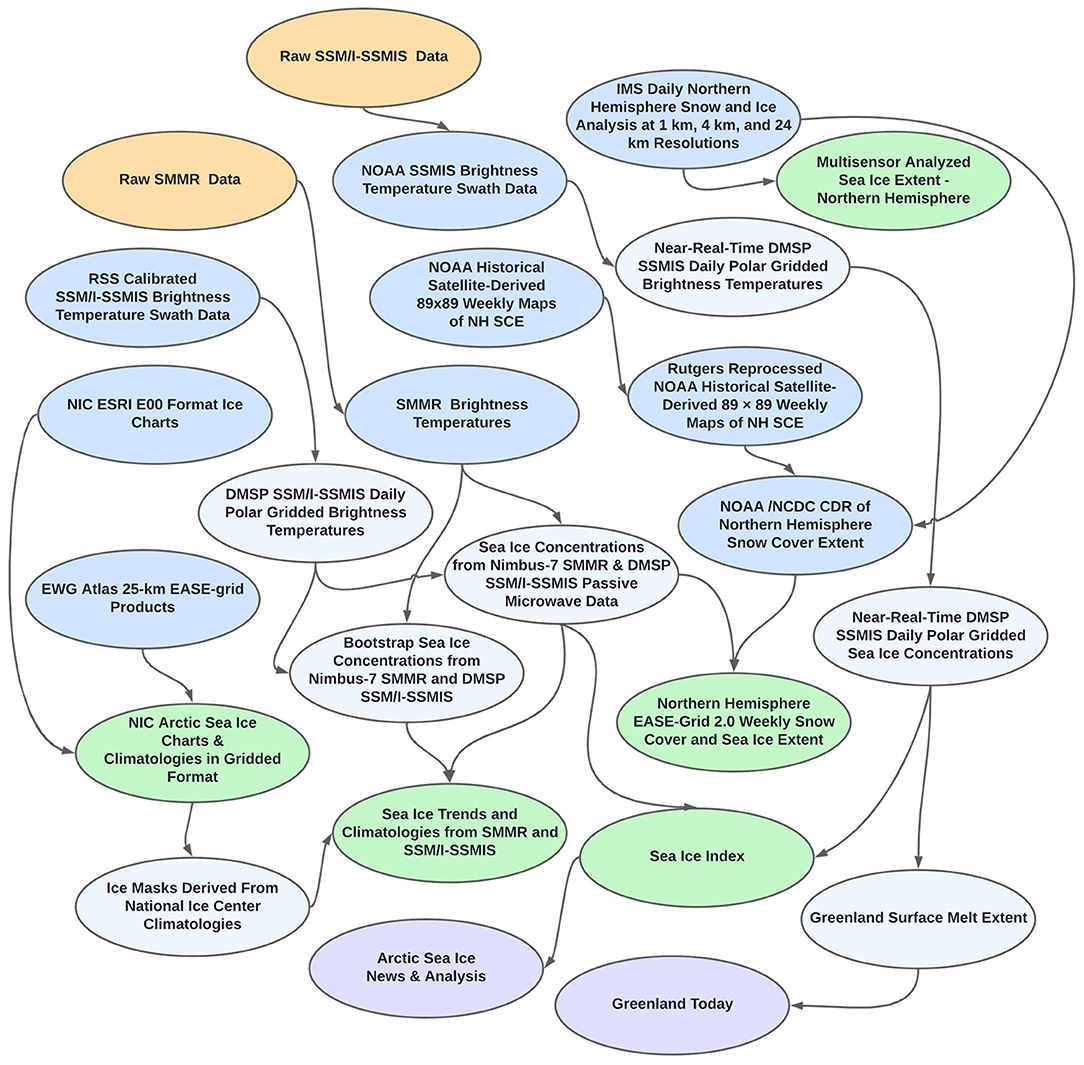
Figure 2. Each bubble represents a data product, most of which are publicly available from NSIDC. Orange products are raw data; while dark blue indicates data external to NSIDC used in creating NSIDC data products. Light blue indicates science products available from NSIDC; while Green indicates products that are NSIDC terms “Easy-to-use”. In NSIDC's view these data products are in “formats that require little or no processing or programming. These may be of particular interest to K-12 teachers and students, the press, the general public, or non-cryospheric researchers.” (NSIDC, 2022). Purple represents science products (blogs) also available from the NSIDC homepage that provide data interpretation for broad audiences. Figure adapted and updated from Baker et al. (2015).
Recommendations to Enhance Community Resilience
To address the challenges identified in the previous section, this section describes four categories of recommendations to help improve how Earth Science enhances community resilience:
• Integrate community into the scientific data pathway,
• Build capacity to bridge science and place-based community needs,
• Balance openness with self-governance, and
• Improve access to data tools to support community resilience.
These recommendations present elements of decentralized, transdisciplinary, and systems thinking, which are needed to address the complex social-ecological systems that underlie community resilience. Figure 3 provides a summary of how the recommendations (section Recommendations to Enhance Community Resilience) map to the challenges presented previously in this paper (section Data Challenges in Community Resilience).
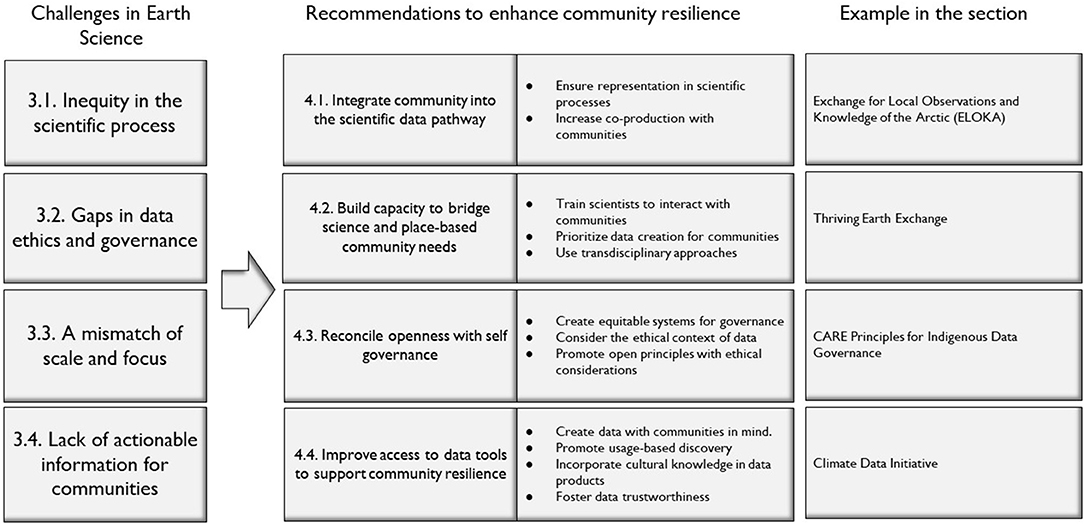
Figure 3. Recommendations to the Earth sciences as to how changes in Earth science can enhance community resilience, stemming from the challenges described in this paper.
Integrate Community Into the Scientific Data Pathway
Develop new conceptual frameworks that incorporate all relevant participants within data usage pathways for societal benefit (e.g., communities, scientists, data managers, analysts, translators, consultants, science communicators, non-profit groups, and other intermediaries).
Earth science applications affect people, the world that we live in, and the resilience of our communities. For this reason, Earth science project design and development, including data creation and curation, should be inclusive and representative of all relevant perspectives, values, and needs. It is challenging to consider the needs of different stakeholders and participants present in a community context, but by doing so, Earth science projects can be designed to ensure that the groups most vulnerable to adverse effects of climate and other environmental events are fairly represented in scientific processes. Progress to that end involves co-production within the conceptual framing of the Earth science data pathway for community resilience. Such a process encourages more interaction between scientific information and the applied context, helping to overcome the entrenched knowledge systems that support one-way linear processes where science disseminates information and knowledge as a commodity.
While programs such as ELOKA have begun to facilitate these processes (in ELOKA's case for Arctic communities), more long-term programs that facilitate co-production in individual research activities for a wide variety of community contexts are needed (Pulsifer et al., 2012). Similarly, large agency missions should explicitly consider the entire range of communities that could potentially find utility in the agency data produced and should design their initial data products accordingly. In general, it can be expected that different products will be needed for each kind of community, so such products should be developed in conjunction with those communities. Moreover, agencies should expect that as conditions change over time, new needs and uses for the data will be found. Consequently, ongoing resources should be allocated to support co-development of useful, new products as they are identified. NASA's long-standing Advancing Collaborative Connections for Earth System Science (ACCESS) program is a prototype of such a program though focused primarily on science community needs (Ramapriyan and Murphy, 2017). Their Earth Science Applications programs that call for projects in specific topic areas, such as health and air quality, use Earth observations to improve decision-making and service to the public.
Several ESIP clusters contribute in this area. For example, the long-standing Agriculture and Climate cluster is working with county and agency fire chiefs and managers on improved data for dealing with wildfires and their aftermaths. A nascent Space Weather group, whose members have worked with national grid providers, are discussing how best to represent space weather impacts on the electrical grid. The Community Data cluster also grappled with how to better include community perspectives in the analysis of data for environmental justice purposes.
Community resilience projects are diverse, and their needs for different co-production processes can vary. Such contextual variation is considered in typologies of community participation (Cornwall, 2008) that range from a more passive information-seeking approach to a more highly interactive transdisciplinary approach, where users are more deeply involved in developing the goals, methods, and analyses of a project. Similarly Meadow et al. (2015) describe strategic co-development of science knowledge through four increasingly cooperative modes of engagement ranging from contractual, consultative, collaborative, to collegial that reflect one-directional flow of information to more shared exchange of different forms of science knowledge. Their examples of conducting action research incorporating social science approaches suggests an openness to transdisciplinary learning that is critical to advance community resilience.
An often used type of co-production approach in science is citizen science, or “community science,” which centers around involving members of the public in the scientific process to both benefit the scientific process and the involved community members (Craglia and Shanley, 2015). Areas of convergence where science and communities can build beneficial co-productive relationships include motivations to benefit society, social location (i.e., the user groups that must be involved to succeed at change), and ethics to support inclusive knowledge generation (Jull et al., 2017).
Co-production in Earth science occurs as scientific data and information interfaces with existing knowledge and wisdom from communities to support decision-making for community resilience. For such a model to work, mutual respect between scientists and community members, as well as a more pluralistic perspective of research expertise is needed to provide an important starting point for successful co-production. This can look like evidence-building activities that incorporate scientific research findings and data (e.g., as expressed in the Foundations of Evidence Based Policy-making Act of 2018) with those based on traditional knowledge systems and tacit place-based knowledge (Kendall et al., 2017; Rainie et al., 2017). Strategic reframing is one approach for conflict resolution in environmental management decisions that allows government agencies to adapt to different stake and rights holder perspectives and consider different scenarios (Auad et al., 2018). Such integration of world views helps to build trust around science, while also ensuring that scientific information is relevant and useful within real-world contexts. The goal of these processes is for Earth scientists to become more sensitive to the historical colonial context of science that influences and colors their assumptions, approaches, implicit biases, and applications within their work (D'Ignazio and Klein, 2020).
Build Capacity to Bridge Science and Place-Based Community Needs
Provide more skills development for scientists, community members, and other participants within the data usage pathway to help bridge gaps and develop intermediaries.
Training and professional development for scientists can help them engage more meaningfully with communities and better understand the real-world context that their work applies to. While many scientists are unlikely to interact directly with communities, some do interact with community members that use and interface with data based on their unique skills and to fulfill different roles (as intermediaries). These include: Communicators (who make sense of and tell stories about data for others to digest), Readers (who need skills to interpret data), Makers (who need the skills to use data for problem solving), and Scientists (who are knowledgeable about the data domain and need to leverage strong technical data use and communication skills) (Wolff et al., 2016). Community members with technical skills (e.g., Scientists as described above) can be valuable brokers, representing community needs in collaboration with the Earth science data community. The Pacific Islands Climate Adaptation Science Center's University of Hawai'i's Manager Climate Corps program (Laursen et al., 2018) demonstrates the value of a careful needs assessment as researchers embarked on a collaboration with natural and cultural resource managers to co-create climate adaptation strategies–their processes of harnessing the knowledge and experiential capacities of local experts through close interpersonal engagement helped establish relationships that can jointly work toward social-ecological change.
Recent trends in data science education are promising for creating a new generation of application-focused data scientists (e.g., Irizarry, 2020). Scholars have advocated for multiple strategies and new curricular foci to train students to effectively use data to understand and tackle societal problems–these suggestions include creating opportunities for students to actively engage with real-world projects, collaborate across disciplines, and across sectors like industry and government (Song and Zhu, 2016); learn to interact with multivariate phenomena that depict the complexity and interconnectedness of variables underlying societal issues (Engel, 2017); and inculcate “habits of mind” (p. 5) in practice and theory that encourage critical thinking, inquiry-based reasoning, and problem solving (Finzer, 2013). Greater attention to skills-based education is a start to help data scientists develop data products for community members in a manner that prioritizes community members' use (rather than Earth scientists' use). However, the burden should not fall on communities to defend themselves with data, and the education of data skills and capability to work with data should not be isolated to the privileged. This would help communities overcome barriers in access and use of available information to advocate on their own behalf.
To address the problems of scale and community data needs that we identify in our challenges, new research pathways can be created by pursuing transdisciplinary approaches based on communities of practice (Wenger-Trayner and Wenger-Trayner, 2015). Such communities of practice would include Earth science and Community Resilience practitioners, who are brought together by common needs and goals. These communities of practice would act as transdisciplinary teams to “work jointly to grasp the complexity of problems from diverse scientific and societal perspectives, integrate natural and social science disciplines, alter discipline-specific approaches, and focus on problem-solving for what is perceived to be the common good” (Yates et al., 2015). These teams would require long-term and stable co-operation between Earth Scientists and the community. Working together would help develop translators or intermediaries between different stakeholders in the information pathway, such as translating between communities to understand information needs and what data is appropriate for resilience planning. As an example, the Bureau of Ocean Energy Management, a US federal agency, could integrate a multi-scale resilience framework into its scientific research and management enterprise in order for science to more clearly articulate and navigate the multi-scale dynamics of social-ecological systems (Auad et al., 2018). Building connections between Earth science and communities may benefit from intermediary organizations and initiatives, like the Thriving Earth Galkiewicz and Pandya (2014) which can help identify communities with Earth science challenges and facilitate interactions with Earth science experts.
Reconcile Openness With Self-Governance
Open science reduces barriers to information while increasing governance & agency over data to protect the interests of people and communities that are subjects of data.
We articulated two problems concerning access to data. The first is the limited access to relevant data by those outside the Earth science community. The second problem is marginalized communities' limited agency over data use. While these may seem to have different solutions, we argue that both problems require the same solution: creating equitable systems for data products, infrastructure, and governance. Such management of Earth science data is not only scientific, but also dependent on societal norms and cultural best practices. Yet transformative shifts in norms of practice or systems change are inherently challenging as highlighted by Jagannathan et al. (2020) in their analysis of outcomes of knowledge co-production processes observed in practice compared to those theorized. However, initiatives are already in place to change data systems that enable more transparency and access to data and will hopefully spur additional efforts. For example, the FAIR (Findable, Accessible, Interoperable, and Reusable) principles (Wilkinson et al., 2016) is an example of one solution that has been offered to help increase community members' access to data.
Additionally, there have been some good examples of how the consideration of the ethical context around data collection, governance, and use has occured at different scales that affect the Earth science data community. The 2019 decadal U.S. Federal Data Strategy emphasizes ethical governance, conscious design, and learning culture to “continually challenge and guide agencies, practitioners, and policymakers to improve the government's approach to data stewardship and the leveraging of data to create value” (United States Government et al., 2019). Recent findings from an open forum co-hosted by the Data Coalition and the Data Foundation concluded that future Federal Action Plans should emphasize “equity and inclusion, the importance of sharing data in a way that protects and respects privacy, and prioritizes transparency and openness” (Turbes, 2020). The American Geophysical Union (AGU) has also published ethics questions for practicing scientists to consider during the data life cycle (Gundersen, 2017) and the AGU Position Statement on Data states “all players in the science ecosystem should ensure … that relevant scientific evidence is processed, shared, and used ethically…” (American Geophysical Union, 2019). Privacy concerns can involve the release of information by different entities, so it may benefit from control by an overarching governance body (Commission on Evidence-Based Policymaking, 2017).
Openness, without governance, might infringe upon the rights and privacy of people who are subjects of data, but the CARE principles present an example of an ethical bookend to FAIR. The CARE principles were formulated to further the self-determination of indigenous people against the ongoing process of colonial oppression and exploitation of indigenous knowledge and data (Carroll et al., 2020). Similar principles could also be more broadly applied beyond the indigenous context and among other groups of people who are historically marginalized in society.
We note that principles alone will not resolve these issues. Principles must be carried over into practice. While several groups are working to translate FAIR principles into action as well as measure the FAIRness of research data (Bahim et al., 2020); practices and measures for the CARE principles are not as far along. However, it should be noted that the ESIP Sustainable Data Management cluster has been working with the Global Indigenous Data Alliance (GIDA) to define the responsibilities and actions data repositories should take to become more CARE-compliant (Global Indigenous Data Alliance, 2019). Moreover, work on translating the CARE principles into theory and action for researchers is forthcoming from IEEE (a project on “Recommended Practice for Provenance of Indigenous Peoples' Data” https://standards.ieee.org/ieee/2890/10318/) and Research Data Alliance (International Indigenous Data Sovereignty Interest Group, https://www.rd-alliance.org/groups/international-indigenous-data-sovereignty-ig).
Reconciling openness with governance offers the opportunity to be deliberative with incremental progress that carefully considers the ethics behind data use. Science and society has no other option but to reconcile these objectives, as further promotion of open principles along with due consideration for the ethical dimension are two critical components for maintaining the social contract (Lubchenco and Rapley, 2020).
Improve Access to Data Tools to Support Community Resilience
Reduce the burden for community resilience practitioners to discover and access Earth science data.
People in communities use a diverse set of sources to make decisions impacting their lives, including those that address problems that arise within the complex systems that they live in. Decisions are rapid and responsive to evolving information contexts, and may not be based in the sciences, including Earth sciences. In other words, in the absence of data that are specific to a problem, people use whatever information they have at hand. Decision-makers may base their decisions on data that have been reinterpreted within a personal or specific context. They might also seek out experts to help interpret the landscape of the Earth sciences and present contextualized information relevant to the community's predicament. Secondary data sources that provide a level of interpretation, which people in communities consume as scientific products, can form the basis of people's world views. For example, the Climate Data Initiative brought together earth science datasets and reframed them in a global resilience context to enable broader consumption by practitioners (Sisco et al., 2019). In this derived data context, discovery of scientific data may occur when pre-digested and cited secondary scientific information is cataloged to allow people to back track to originating discoveries and datasets more easily, and challenge misconceptions that may inadvertently arise about the secondary sources. Cvitanovic et al., 2014 also recommends the creation of management-oriented summaries of research articles to describe the policy implications of research outcomes in publicly meaningful ways; many journals have instituted or are in the process of instituting the concept of plain language summaries.
ESIP's Discovery cluster emphasizes that making assumptions about data's future utility should be avoided. Instead, data discovery informed by the end-user's actual usage (Usage-based data discovery; Lynnes et al., 2020) can better align existing knowledge frameworks to re-prioritize research investments. As a result of this process, intended audiences and beneficiaries of data management labors can be clearly identified, so the relevance and value of highly technical endeavors can be better targeted (e.g., decision-makers who seek to support resilience in their community). Usage-based data discovery can further increase the utility of data by providing examples of how data have been used by other communities, while communicating the larger application context for the data. With the right processes in place, a feedback mechanism to data providers would also allow for iterative improvements.
To further this process, Earth science repositories can develop controlled vocabularies to tag datasets according to specific information needs (e.g., Semnacher and Chong, 2019). This allows federal agencies to enhance discovery and demonstrate the applicability of their research products to society and sustainable development goals (e.g., the Arctic report card by Starkweather et al., 2020). In these scenarios, data products (e.g., summaries, subsets, trends, or infographic representations) that are context- and culturally-specific are more easily utilized by decision-makers within their community (Baker et al., 2015). Correctly scaled data in a format usable by communities can interact with their cultural knowledge to produce data subsets and syntheses that directly improve community resilience. For example, city governments develop resilience plans that cover different sectors of the government, including energy, food, and urban infrastructure. These plans aim to bring together government, industry, community groups, and residents using a collaborative transdisciplinary effort. Earth scientists would be more closely involved in these efforts as the developers of data being used to guide decision-making. Data are often used by communities post-hoc, so the purpose of data and their relevant metadata need to be carefully and clearly articulated so they can be used appropriately.
Information about the reliability and trustworthiness of data should also be provided. A basic overview of the database, including how it was developed, who was involved, and the audiences for whom it was intended are some basic metadata that are fundamental to improving the usability of data. For example, websites displaying analyses of Earth science can provide a level of transparency to understand data sources and versions of data used in the analytical products (Lynnes et al., 2020). The Earth sciences could provide data that have verifiable data trust measures as a means to pre-assess the quality of data for applications (see example below). While there will always be inherent uncertainty in Earth science data products, certain datasets are categorically wrong for certain applications, and an established and transparent evaluation process would help mitigate untrained users from missapplying data (Ekstrom et al., 2015; Nissan et al., 2019) and clearly communicate the uncertainty within the dataset. This would enable increased trust and reliability in external data sources by users of this data in community contexts. Recent guidelines around the trustworthiness of data can help the scientific community (Jamieson et al., 2019) and repositories (Lin et al., 2020) with their assessment of datasets. Trusted data are highly reusable, broadly applicable, of verified quality, and clarify the source of the data and its intended applicability. Data trust assessments should be provided to datasets that meet transparent guidelines or rules on data maintenance, governance, and storage that emphasize access, accountability, and long-term management. This is another area where ESIP clusters have been actively working. For example, the Information Quality Cluster recently published a paper calling for the development of practical guidelines for representing and sharing data quality information (Peng et al., 2021).
In another example, Operational Readiness Levels (ORL) developed within the ESIP Disaster Lifecyle cluster provide a ranking by which to provide decision-makers with an understanding of the operational reliability of datasets using predefined criteria (case study provided by Hicks in the ESIP webinar Moe et al., 2018). This is an example of how credentials can allow for diverse datasets to be accessed and used in decision-making, while still accounting for the level of uncertainty inherent to a given data set. This can drive community decision-making by forwarding value-neutral information as to how complete or ready a data set is. Similar ORLs could be developed for community planning data, such as climate/weather/natural disaster forecasts.
Conclusion
In this paper, we described the challenges of applying Earth science data to community resilience decision-making. Inequity in the scientific process presents challenges in identifying solutions that are both innovative and reflective of community needs. Gaps in data ethics and governance highlight the need to be aware of how misuse of data can negatively impact people who are subjects of that data. A mismatch of scale and focus emphasizes the importance of how data must address the different sociopolitical, temporal, and geographical scales that are relevant to place-based community resilience. Lack of actionable information for communities speaks to the gaps between the types of data products that are produced and the expectations of communities to use them.
We encourage the Earth science data community to develop practices for improving how Earth science data and analyses are disseminated to and used for community resilience purposes. To that end, we have suggested the following starting points:
• Integrate community into the scientific data pathway to emphasize the importance of re-framing how we think about data literacy, and re-conceptualize the DIKW pathway to be more inclusive of community world views.
• Build capacity to bridge science and place-based needs to help identify opportunities for skills development among both scientists and community decision-makers to reduce the disconnect between the groups.
• Reconcile openness with self-governance to create more ethical and equitable systems of data products, infrastructure, and governance.
• Improve access to data tools to support community resilience by prioritizing usage-based discovery to ensure data can better meet the needs of communities.
The framing that we present here can help organizations like ESIP mobilize Earth science data scientists and practitioners to develop innovative solutions to Earth science data challenges, and affect durable and effective change with the most impactful benefit across society. Such organizations have the capacity and connections to integrate the suggestions that we offer within the Earth science ecosystem. Through our work in ESIP's Community Resilience cluster and in collaboration with other ESIP clusters, we see the potential to create spaces within Earth science that better support place-based community needs.
Contributing to these Earth science data opportunities is our professional responsibility to the communities that we are a part of, since as scientists we have access to resources, skills, and tools that many in our society do not. Without more attention given to bridging the gaps between Earth science and community resilience, we risk continuing to exclude and marginalize the most vulnerable place-based communities in our world, and continue to waste scarce resources on producing data and investing in scientific initiatives that do not meet the urgent needs of our society. By working in concert with communities, Earth Scientists can contribute to making the systemic changes needed to help overcome some of the biggest challenges of our generation.
Author Contributions
AV coordinated meetings and drafts, developed initial concept of the paper, contributed original writing, contributed revisions, acted as corresponding author, and submitted the article. RG, JB, and RD developed initial concept of the paper, contributed original writing, and contributed revisions. ZR contributed original writing, contributed revisions, formatted and completed citations, and drafted and completed figure. CG contributed revisions, formatted citations, formatted paper, and drafted and completed figure. All authors contributed to the article and approved the submitted version.
Funding
CG and ZR were supported through the Earth Science Information Partners (ESIP) Community Fellowship in 2021 and 2019–2020, respectively. Funding for open access publication was provided by Earth Science Information Partners (ESIP).
Conflict of Interest
During this work, AV was the sole employee of the Middle Path EcoSolutions consultancy which aims to empower communities to become more healthy and sustainable. RG was a Researcher at Knology for the majority of the writing process.
The remaining authors declare that the research was conducted in the absence of any commercial or financial relationships that could be construed as a potential conflict of interest.
Publisher's Note
All claims expressed in this article are solely those of the authors and do not necessarily represent those of their affiliated organizations, or those of the publisher, the editors and the reviewers. Any product that may be evaluated in this article, or claim that may be made by its manufacturer, is not guaranteed or endorsed by the publisher.
Acknowledgments
We would like to thank the participants of previous community resilience-themed ESIP meeting sessions, particularly the Summer Meeting 2015 in Monterey, CA; Winter Meeting January 2017 in Bethesda, MD; Winter Meeting January 2018 in Bethesda, MD; Winter Meeting 2019 in Bethesda, MD; Winter Meeting 2021 (virtual); and Summer Meeting 2021 (virtual). We acknowledge Steve Young and the expertise he provided on Environmental Protection Agency (EPA) regulations and the environmental justice issue in Flint, Michigan. We thank Drs. Lindsay Barbieri, Brian Wee, and Christine White who were co-authors of a synthesis report entitled Community Resilience: Demonstrating the socioeconomic value of Earth Science data from ESIP Winter Meeting 2018 in Bethesda, MD that provided inspiration for this paper. Two reviewers also provided helpful comments and suggestions that improved the paper. The views expressed in this article do not necessarily represent the views of the Bureau of Ocean Energy Management, the Department of the Interior, or the United States.
References
Adger, W. N., Hughes, T. P., Folke, C., Carpenter, S. R., and Rockström, J. (2005). Social-ecological resilience to coastal disasters. Science 309, 1036–1039. doi: 10.1126/science.1112122
Aldrich, D. P., and Meyer, M. A. (2015). Social capital and community resilience. Am. Behav. Scientist 59, 254–269. doi: 10.1177/0002764214550299
Allen, M. R., Pachauri, R. K., Barros, V. R., Broome, J., Cramer, W., Christ, R., et al. (2014). “Climate change 2014: synthesis report,” in Contribution of Working Groups I, II and III to the Fifth Assessment Report of the Intergovernmental Panel on Climate Change, eds R. K. Pachauri and L. Meyer (EPIC3Geneva; IPCC), 151. Available online at: https://epic.awi.de/id/eprint/37530/
American Geophysical Union (2019). AGU Position Statement on Earth and Space Science Data. Poistion Statment on Data. Available online at: https://www.agu.org/Share-and-Advocate/Share/Policymakers/Position-Statements/Position_Data
Auad, G., Blythe, J., Coffman, K., and Fath, B. D. (2018). A dynamic management framework for socio-ecological system stewardship: a case study for the United States Bureau of Ocean Energy Management. J. Environ. Manage. 225, 32–45. doi: 10.1016/j.jenvman.2018.07.078
Bahim, C., Casorrán-Amilburu, C., Dekkers, M., Herczog, E., Loozen, N., Repanas, K., et al. (2020). The FAIR data maturity model: an approach to harmonise FAIR assessments. Data Sci. J. 19, 41. doi: 10.5334/dsj-2020-041
Baker, K. S., Duerr, R. E., and Parsons, M. A. (2015). Scientific knowledge mobilization: co-evolution of data products and designated communities. Int. J. Digital Curation 10, 110–135. doi: 10.2218/ijdc.v10i2.346
Balazs, C. L., and Ray, I. (2014). The drinking water disparities framework: on the origins and persistence of inequities in exposure. Am. J. Public Health 104, 603–611. doi: 10.2105/AJPH.2013.301664
Bautista, E., Hanhardt, E., Osorio, J. C., and Dwyer, N. (2015). New York City environmental justice alliance waterfront justice project. Local Environ. 20, 664–682. doi: 10.1080/13549839.2014.949644
Berhe, A. A., Barnes, R. T., Hastings, M. G., Mattheis, A., Schneider, B., Williams, B. M., et al. (2022). Scientists from historically excluded groups face a hostile obstacle course. Nat. Geosci. 15, 2–4. doi: 10.1038/s41561-021-00868-0
Bhargava, S., and Manoli, D. (2015). Psychological frictions and the incomplete take-up of social benefits: evidence from an IRS field experiment. Am. Econ. Rev. 105, 3489–3529. doi: 10.1257/aer.20121493
Bhuvandas, N., Timbadiya, P. V., Patel, P. L., and Porey, P. D. (2014). Review of downscaling methods in climate change and their role in hydrological studies. Int. J. Geol. Environ. Eng. 8, 713–718.
Bone, C., Moseley, C., Vinyeta, K., and Bixler, R. P. (2016). Employing resilience in the United States Forest Service. Land Use Policy 52, 430–438. doi: 10.1016/j.landusepol.2016.01.003
Bremer, S., Wardekker, A., Dessai, S., Sobolowski, S., Slaattelid, R., and van der Sluijs, J. (2019). Toward a multi-faceted conception of co-production of climate services. Climate Serv. 13, 42–50. doi: 10.1016/j.cliser.2019.01.003
Brulle, R. J., and Pellow, D. N. (2006). Environmental justice: human health and environmental inequalities. Annu. Rev. Public Health 27, 103–124. doi: 10.1146/annurev.publhealth.27.021405.102124
Brun, V., and Schumacher, T. (1987). Traditional Herbal Medicine in Northern Thailand. Berkeley, CA: University of California Press.
Butler, L. J., Scammell, M. K., and Benson, E. B. (2016). The Flint, Michigan, water crisis: a case study in regulatory failure and environmental injustice. Environ. Justice 9, 93–97. doi: 10.1089/env.2016.0014
Carroll, S. R., Garba, I., Figueroa-Rodríguez, O. L., Holbrook, J., Lovett, R., Materechera, S., et al. (2020). The CARE principles for indigenous data governance. Data Sci. J. 19, 43. doi: 10.5334/dsj-2020-043
Chandra, A., Acosta, J., Howard, S., Uscher-Pines, L., Williams, M., Yeung, D., et al. (2011). Building community resilience to disasters. Rand Health Q. 1.
Chelleri, L., Waters, J. J., Olazabal, M., and Minucci, G. (2015). Resilience trade-offs: addressing multiple scales and temporal aspects of urban resilience. Environ. Urban. 27, 181–198. doi: 10.1177/0956247814550780
Commission on Evidence-Based Policymaking (2017). The Promise of Evidence-Based Policymaking. Bipartisan Policy Center. Available online at: https://bipartisanpolicy.org/download/?file=/wp-content/uploads/2019/03/Full-Report-The-Promise-of-Evidence-Based-Policymaking-Report-of-the-Comission-on-Evidence-based-Policymaking.pdf
Cornwall, A. (2008). Unpacking 'participation': models, meanings and practices. Commun. Dev. J. 43, 269–283. doi: 10.1093/cdj/bsn010
Craglia, M., and Shanley, L. (2015). Data democracy - increased supply of geospatial information and expanded participatory processes in the production of data. Int. J. Digital Earth 8, 679–693. doi: 10.1080/17538947.2015.1008214
Cutter, S. L., Ahearn, J. A., Amadei, B., Crawford, P., Eide, E. A., Galloway, G. E., et al. (2013). Disaster resilience: a national imperative. Environ. Sci. Policy Sustain. Dev. 55, 25–29. doi: 10.1080/00139157.2013.768076
Cvitanovic, C., Fulton, C. J., Wilson, S. K., van Kerkhoff, L., Cripps, I. L., and Muthiga, N. (2014). Utility of primary scientific literature to environmental managers: an international case study on coral-dominated marine protected areas. Ocean Coast. Manag. 102, 72–78. doi: 10.1016/j.ocecoaman.2014.09.003
Diggs, S., Thomer, A., and McKenzie, M. (2021). Community Data Cluster Ideation and Gap Analysis, Facilitated by the Community Data Cluster. 2021 ESIP Winter Meeting. Available online at: https://2021esipwintermeeting.sched.com/event/g49o/community-data-cluster-ideation-and-gap-analysis
D'Ignazio, C., and Bhargava, R. (2016). DataBasic: design principles, tools and activities for data literacy learners. J. Commun. Informatics 12, 83–107. doi: 10.15353/joci.v12i3.3280
Dunlop, C. A. (2009). Policy transfer as learning - capturing variation in what decision-makers learn from epistemic communities. Policy Stud. 30, 289–311. doi: 10.1080/01442870902863869
Dunn, M. R., Lindesay, J. A., and Howden, M. (2015). Spatial and temporal scales of future climate information for climate change adaptation in viticulture: a case study of user needs in the Australian winegrape sector. Aust. J. Grape Wine Res. 21, 226–239. doi: 10.1111/ajgw.12138
Dutton, J., Bretherton, F. P., Jenne, R. L., Karin, S., Volansky, S., Webster, F., et al. (1995). “The Earth Sciences Information System” (Appendix F) in A Review of the U.S. Global Change Research Program and NASA's Mission to Planet Earth/Earth Observing System. National Research Council. Available online at: https://ntrs.nasa.gov/api/citations/19960016634/downloads/19960016634.pdf
Eitzel, M. V., Cappadonna, J. L., Santos-Lang, C., Duerr, R. E., Virapongse, A., West, S. E., et al. (2017). Citizen science terminology matters: exploring key terms. Citizen Sci. Theory Prac. 2, 1–20. doi: 10.5334/cstp.96
Ekstrom, J. A., Suatoni, L., Cooley, S. R., Pendleton, L. H., Waldbusser, G. G., Cinner, J. E., et al. (2015). Vulnerability and adaptation of US shellfisheries to ocean acidification. Nat. Clim. Chang. 5, 207–214.
Engel, J. (2017). Statistical literacy for active citizenship: a call for data science education. Stat. Educ. Res. J. 16, 44–49. doi: 10.52041/serj.v16i1.213
Fath, B. D., Dean, C. A., and Katzmair, H. (2015). Navigating the adaptive cycle: an approach to managing the resilience of social systems. Ecol. Soc. 20. doi: 10.5751/ES-07467-200224
Finzer, W. (2013). The data science education dilemma. Technol. Innov. Stat. Educ. 7.doi: 10.5070/T572013891
Funfgeld, H., and McEvoy, D. (2012). Resilience as a useful concept for climate change adaptation? Plann. Theory Prac. 13, 324–328. doi: 10.1080/14649357.2012.677124
Funk, C. (2012). Key Findings About Americans' Confidence in Science and Their Views on Scientists' Role in Society. Pew Research Center. Available online at: https://www.pewresearch. org/fact-tank/2020/02/12/key-findings-about-americans-confidence-in-science-and-their-views-on-scientists-role-in-society
Galkiewicz, J., and Pandya, R. (2014). Meeting people where they are: Thriving earth exchange. Eos Trans. Am. Geophys Union. 95, 44–44. doi: 10.1002/2014EO050006
Global Indigenous Data Alliance (2019). CARE Principles for Indigenous Data Governance. GIDA. Available online at: https://www.Gida-Global.Org/Care
Green2.0 (2021). 2021 NGO & Foundation Transparency Report Card, Green2.0. Available online at: https://diversegreen.org/transparency-cards/2021-green-2-0-ngo-foundation-transparency-report-card/
Gundersen, L. C., (ed.). (2017). Scientific Integrity and Ethics in the Geosciences. Hoboken, NJ: John Wiley & Sons, Inc.
Heeks, R., and Renken, J. (2018). Data justice for development: what would it mean? Information Dev. 34, 90–102. doi: 10.1177/0266666916678282
Higuera, P. E., Metcalf, A. L., Miller, C., Buma, B., McWethy, D. B., Metcalf, E. C., et al. (2019). Integrating subjective and objective dimensions of resilience in fire-prone landscapes. Bioscience 69, 379–388. doi: 10.1093/biosci/biz030
Irizarry, R. A. (2020). The role of academia in data science education. Harvard Data Sci. Rev. 2. doi: 10.1162/99608f92.dd363929
Ismail-Zadeh, A. T., Cutter, S. L., Takeuchi, K., and Paton, D. (2016). Forging a paradigm shift in disaster science. Natural Hazards 2, 969–988. doi: 10.1007/s11069-016-2726-x
Jagannathan, K., Arnott, J. C., Wyborn, C., Klenk, N., Mach, K. J., Moss, R. H., et al. (2020). Great expectations? Reconciling the aspiration, outcome, and possibility of co-production. Curr. Opin. Environ. Sustain. 42, 22–29. doi: 10.1016/j.cosust.2019.11.010
Jamieson, K. H., McNutt, M., Kiermer, V., and Sever, R. (2019). Signaling the trustworthiness of science. Proc. Nat. Acad. Sci. U.S.A. 116, 19231–19236. doi: 10.1073/pnas.1913039116
Jull, J., Giles, A., and Graham, I. D. (2017). Community-based participatory research and integrated knowledge translation: advancing the co-creation of knowledge. Implement. Sci. 12, 150. doi: 10.1186/s13012-017-0696-3
Katner, A., Pieper, K. J., Lambrinidou, Y., Brown, K., Hu, C. Y., Mielke, H. W., et al. (2016). Weaknesses in federal drinking water regulations and public health policies that impede lead poisoning prevention and environmental justice. Environ. Justice 9, 109–117. doi: 10.1089/env.2016.0012
Kendall, J. J. K. Jr., Brooks, J. J., Campbell, C., Wedemeyer, K. L., Coon, C. C., Warren, S. E., et al. (2017). Use of traditional knowledge by the United States Bureau of Ocean Energy Management to support resource management. Czech Polar Rep. 7, 151–163. doi: 10.5817/CPR2017-2-15
Keskitalo, E. C. H., Horstkotte, T., Kivinen, S., Forbes, B., and Käyhkö, J. (2016). “Generality of mis-fit”? The real-life difficulty of matching scales in an interconnected world. Ambio 45, 742–752. doi: 10.1007/s13280-015-0757-2
Khayyat, M., and Bannister, F. (2015). Open data licensing: more than meets the eye. Information Polity 20, 231–252. doi: 10.3233/IP-150357
King, C. A. (2008). Community resilience and contemporary agri-ecological systems: Reconnecting people and food, and people with people. Syst. Res. Behav. Sci. 25, 111–124. doi: 10.1002/sres.854
Kirchhoff, C. J., Carmen Lemos, M., and Dessai, S. (2013). Actionable knowledge for environmental decision making: broadening the usability of climate science. Annu. Rev. Environ. Resour. 38, 393–414. doi: 10.1146/annurev-environ-022112-112828
Kirchmeier-Young, M. C., Wan, H., Zhang, X., and Seneviratne, S. I. (2019). Importance of framing for extreme event attribution: the role of spatial and temporal scales. Earth's Future 7, 1192–1204. doi: 10.1029/2019EF001253
Kirmayer, L. J., Sehdev, M., Whitley, R., Dandeneau, S. F., and Isaac, C. (2009). Community resilience: models, metaphors and measures. Int. J. Indigenous Health 5, 62–117. doi: 10.3138/ijih.v5i1.28978
Kirmayer, L. J., and Valaskakis, G. G. (2009). Healing Traditions: The Mental Health of Aboriginal Peoples in Canada. Vancouver, BC: UBC Press.
Klinsky, S., Roberts, T., Huq, S., Okereke, C., Newell, P., Dauvergne, P., et al. (2017). Why equity is fundamental in climate change policy research. Global Environ. Change 44, 170–173. doi: 10.1016/j.gloenvcha.2016.08.002
Laursen, S., Puniwai, N., Genz, A. S., Nash, S. A., Canale, L. K., and Ziegler-Chong, S. (2018). Collaboration across worldviews: managers and scientists on Hawaiî Island utilize knowledge coproduction to facilitate climate change adaptation. Environ. Manage. 62, 619–630. doi: 10.1007/s00267-018-1069-7
Lemos, M. C., Arnott, J. C., Ardoin, N. M., Baja, K., Bednarek, A. T., Dewulf, A., et al. (2018). To co-produce or not to co-produce. Nat. Sustain. 1, 722–724. doi: 10.1038/s41893-018-0191-0
Li, F., and Whalley, J. (2002). Deconstruction of the telecommunications industry: from value chains to value networks. Telecomm. Policy 26, 451–472. doi: 10.1016/S0308-5961(02)00056-3
Lin, D., Crabtree, J., Dillo, I., Downs, R. R., Edmunds, R., Giaretta, D., et al. (2020). The TRUST principles for digital repositories. Scientific Data 7, 144. doi: 10.1038/s41597-020-0486-7
Lubchenco, J., and Rapley, C. (2020). Our moment of truth: the social contract realized? Environ. Res. Lett. 15, 110201. doi: 10.1088/1748-9326/abba9c
Lynnes, C., Zhu, M. Q., Blythe, J., Williamson, T. N., Burnett, J., Huffer, E., et al. (2020). Usage-Based Discovery of Earth Observations. 2020, IN012-02. Available online at: https://ui.adsabs.harvard.edu/abs/2020AGUFMIN012..02L
Mach, K. J., Lemos, M. C., Meadow, A. M., Wyborn, C., Klenk, N., Arnott, J. C., et al. (2020). Actionable knowledge and the art of engagement. Curr. Opin. Environ. Sustain. 42, 30–37. doi: 10.1016/j.cosust.2020.01.002
Magis, K. (2010). Community resilience: an indicator of social sustainability. Soc. Nat. Resources 23, 401–416. doi: 10.1080/08941920903305674
Mayernik, M., and Virapongse, A. (2019). Making Data Matter with Matt Mayernik. Making Data Matter. doi: 10.6084/m9.figshare.7914197.v1
McWethy, D. B., Schoennagel, T., Higuera, P. E., Krawchuk, M., Harvey, B. J., Metcalf, E. C., et al. (2019). Rethinking resilience to wildfire. Nat. Sustain. 2, 797–804. doi: 10.1038/s41893-019-0353-8
Meadow, A. M., Ferguson, D. B., Guido, Z., Horangic, A., Owen, G., and Wall, T. (2015). Moving toward the deliberate coproduction of climate science knowledge. Weather Climate Soc. 7, 179–191. doi: 10.1175/WCAS-D-14-00050.1
Meier, W., Fetterer, F., Windnagel, A., and Stewart, S. (2021). NOAA/NSIDC Climate Data Record of Passive Microwave Sea Ice Concentration, Version 4. doi: 10.7265/EFMZ-2T65
Moe, K., Moran, T., Jones, D., Hicks, K., Glasscoe, M., Virapongse, A., et al. (2018). ESIP Webinar #5: Managing Disasters Through Improved Data-Driven Decision-Making. doi: 10.6084/m9.figshare.7361327
Nissan, H., Goddard, L., de Perez, E. C., Furlow, J., Baethgen, W., Thomson, M. C., et al. (2019). On the use and misuse of climate change projections in international development. Wiley Interdiscip. Rev. Clim. Chang. 10, e579. doi: 10.1002/wcc.579
NSIDC (2022). Easy-to-use Data Products. NSIDC. Available online at: https://nsidc.org/data/resources/easy-to-use.
O'Neill, E. T. (2011). Frbr: functional requirements for bibliographic records. Libr. Resour. Tech. Serv. 46, 150–159. doi: 10.5860/lrts.46n4.150
Parsons, M. A., Godøy, Ø., LeDrew, E., de Bruin, T. F., Danis, B., Tomlinson, S., et al. (2011). A conceptual framework for managing very diverse data for complex, interdisciplinary science. J. Information Sci. 37, 555–569. doi: 10.1177/0165551511412705
PCAST-Executive Office of the President (2011). Sustaining Environmental Capital: Protecting Society and the Environment. President's Council of Advisors on Science and Technology. Available online at: https://obamawhitehouse.archives.gov/sites/default/files/microsites/ostp/pcast_sustaining_environmental_capital_report.pdf
Peng, G., Downs, R.R., Lacagnina, C., Ramapriyan, H., Ivánová, I., Moroni, D., et al. (2021). Call to action for global access to and harmonization of quality information of individual earth science datasets. Data Sci. J. 20, 19. doi: 10.5334/dsj-2021-019
Pratt, K. L. (2004). Observations on researching and managing alaska native oral history: a case study. Alaska J. Anthropol. 2, 138–153.
Pulsifer, P., Gearheard, S., Huntington, H. P., Parsons, M. A., McNeave, C., and McCann, H. S. (2012). The role of data management in engaging communities in Arctic research: Overview of the Exchange for Local Observations and Knowledge of the Arctic (ELOKA). Polar Geogr. 35, 271–90. doi: 10.1080/1088937X.2012.708364
Pulsifer, P. L., Kontar, Y., Berkman, P. A., and Taylor, D. R. F. (2020). “Information ecology to map the arctic information ecosystem,” in Governing Arctic Seas: Regional Lessons from the Bering Strait and Barents Sea, Vol. 1, eds O. R. Young, P. A. Berkman, and A. N. Vylegzhanin (Cham: Springer International Publishing), 269–291.
Rainie, S. C., Rodriguez-Lonebear, D., and Martinez, A. (2017). Policy Brief(Version2): Data Governance for Native Nation Rebuilding. Tucson: Native Nations Institute.
Ramapriyan, H., and Behnke, J. (2019). Importance and incorporation of user feedback in earth science data stewardship. Data Sci. J. 18, 24. doi: 10.5334/dsj-2019-024
Ramapriyan, H. K., and Murphy, K. (2017). Collaborations and partnerships in nasa's earth science data systems. Data Sci. J. 16, 51–55. doi: 10.5334/dsj-2017-051
Reimsbach-Kounatze, C. (2021). “Enhancing access to and sharing of data: striking the balance between openness and control over data,” in Data Access, Consumer Interests and Public Welfare (Nomos Verlagsgesellschaft mbH & Co. KG), 25–68.
Rolan, G., McKemmish, S., Oliver, G., Evans, J., and Faulkhead, S. (2020). “Digital equity through data sovereignty: a vision for sustaining humanity,” in IConference 2020 Proceedings.
Roux, D., Rogers, K., Biggs, H., Ashton, P., and Sergeant, A. (2006). Bridging the science-management divide: moving from unidirectional knowledge transfer to knowledge interfacing and sharing. Ecol. Soc. 11. doi: 10.5751/ES-01643-110104
Rowley, J. (2007). The wisdom hierarchy: representations of the DIKW hierarchy. J. Information Sci. 33, 163–180. doi: 10.1177/0165551506070706
Scassa, T. (2010). Geographical information as 'personal information.' Oxford Univ. Commonwealth Law J. 10, 185–214. doi: 10.5235/147293410794895322
Semnacher, C., and Chong, S. (2019). Improving Information Retrieval: The Arctic Data Center Unveils New Semantic Search Product. Witness the Arctic. Available online at: https://arcus.org/witness-the-arctic/2019/2/article/30127
Sharifi, A. (2016). A critical review of selected tools for assessing community resilience. Ecol. Indic. 69, 629–647. doi: 10.1016/j.ecolind.2016.05.023
Sharma, N. (2008). The Origin of the “Data Information Knowledge Wisdom”(DIKW) Hierarchy. Available online at: http://www.researchgate.net/publication/292335202_The_Origin_of_Data_Information_Knowledge_Wisdom_DIKW_Hierarchy
Sisco, A., Cook, K., Bugbee, K., Davidson, J., Duffy, L., Dabolt, T., et al. (2019). Changing Climate, Changing Data: Exposing Climate Data to New Users Through GeoPlatform.gov's Resilience Community. American Geophysical Union Fall Meeting, Poster Contribution: IN33B-0822, San Francisco, CA. Available online at:: agu.confex.com/agu/fm19/meetingapp.cgi/Paper/615144
Smith, D. E. (2016). “Governing data and data for governance: the everyday practice of indigenous sovereignty, “ in Indigenous Data Sovereignty: Toward an Agenda, 117–135.
Smith, J. W., Moore, R. L., Anderson, D. H., and Siderelis, C. (2012). Community resilience in Southern Appalachia: a theoretical framework and three case studies. Hum. Ecol. 40, 341–353. doi: 10.1007/s10745-012-9470-y
Song, I. Y., and Zhu, Y. (2016). Big data and data science: what should we teach?. Expert Syst. 33, 364–373. doi: 10.1111/exsy.12130
Starkweather, S., Shapiro, H., Vakhutinsky, S., and Druckenmiller, M. (2020). The Observational Foundation of the Arctic Report Card-A 15-Year Retrospective Analysis on the Arctic Observing Network (AON) and Insights for the Future System. National Oceanic and Atmospheric Administration.
Sweeney, L., Yoo, J. S., Perovich, L., Boronow, K. E., Brown, P., and Brody, J. G. (2017). Re-Identification Risks in HIPAA Safe Harbor Data: A Study of Data From One Environmental Health Study. Technology Science, 2017. Available online at: https://www.ncbi.nlm.nih.gov/pmc/articles/PMC6344041/
Tai, T. C., and Robinson, J. P. (2018). Enhancing climate change research with open science. Front. Environ. Sci. 6, 115. doi: 10.3389/fenvs.2018.00115
Tanner, K. D. (2009). Learning to see inequity in science. CBE Life Sci. Educ. 8, 265–270. doi: 10.1187/cbe.09-09-0070
Taylor, H. A. (1984). Information ecology and the archives of the 1980s. Archivaria 25–37. Available online at: https://archivaria.ca/index.php/archivaria/article/view/11075
Theodori, G. L. (2005). Community and community development in resource-based areas: operational definitions rooted in an interactional perspective. Soc. Nat. Resour. 18, 661–669. doi: 10.1080/08941920590959640
Thompson, N., Ravindran, R., and Nicosia, S. (2015). Government data does not mean data governance: lessons learned from a public sector application audit. Govern. Infm. Q. 32, 316–322. doi: 10.1016/j.giq.2015.05.001
Trisos, C. H., Adelekan, I. O., Totin, E., Ayanlade, A., Efitre, J., Gemeda, A., et al. (2022). “Africa,” in Climate Change 2022: Impacts, Adaptation, and Vulnerability. Contribution of Working Group II to the Sixth Assessment Report of the Intergovernmental Panel on Climate Change, eds H.-O. Pörtner, D.C. Roberts, M. Tignor, E.S. Poloczanska, K. Mintenbeck, A. Alegría, et al. (Cambridge: Cambridge University Press), 9–225.
Turbes, C. (2020). Continuing the Federal Data Strategy is a Must-Do for the Next Administration. Data Coalition. Available online at: http://www.datacoalition.org/continuing-the-federal-data-strategy-is-a-must-do-for-the-next-administration,/
UN General Assembly (2007). United Nations declaration on the rights of indigenous peoples. UN Wash 12, 1–18.
UNCTAD (2020). Data Protection and Privacy Legislation Worldwide. UNCTAD. Available online at: https://unctad.org/page/data-protection-and-privacy-legislation-worldwide
UNCTAD DPR. (2016). International Data Flows: Implications for Trade and Development. United Nations. Available online at: https://unctad.org/system/files/official-document/dtlstict2016d1_en.pdf
United States Agency for International Development (USAID) (2012). Building Resilience to Recurrent Crisis: USAID Policy and Program Guidance. Available online at: http://www.usaid.gov/sites/default/files/documents/1870/USAIDResiliencePolicyGuidanceDocument.pdf
United States Government, Droegemeier, K., Kelley, K., Kent, S., Potok, N., and Roat, M. (2019). Federal Data Strategy 2020 Action Plan. Available online at: https://strategy.data.gov/assets/docs/2020-federal-data-strategy-action-plan.pdf
Vera, C., Barange, M., Dube, O. P., Goddard, L., Griggs, D., Kobysheva, N., et al. (2010). Needs assessment for climate information on decadal timescales and longer. Procedia Environ. Sci. 1, 275–286. doi: 10.1016/j.proenv.2010.09.017
Virapongse, A., Brooks, S., Metcalf, E. C., Zedalis, M., Gosz, J., Kliskey, A., et al. (2016). A social-ecological systems approach for environmental management. J. Environ. Manage. 178, 83–91. doi: 10.1016/j.jenvman.2016.02.028
Wee, B., and Piña, A. (2019). A Vision for Adapting at the Pace of Socioenvironmental Change. EOS. Available online at: https://eos.org/opinions/a-vision-for-adapting-at-the-pace-of-socioenvironmental-change
Wenger-Trayner, E., and Wenger-Trayner, B. (2015). Introduction to Communities of Practice. Available online at: https://wenger-trayner.com/introduction-to-communities-of-practice/
Wilkinson, M. D., Dumontier, M., Aalbersberg, I. J., Appleton, G., Axton, M., Baak, A., et al. (2016). The FAIR Guiding Principles for scientific data management and stewardship. Sci. Data 3, 160018. doi: 10.1038/sdata.2016.18
Wolff, A., Gooch, D., Montaner, J. J. C., Rashid, U., and Kortuem, G. (2016). Creating an understanding of data literacy for a data-driven society. J. Commun. Informatics 12, 3. doi: 10.15353/joci.v12i3.3275
Keywords: community resilience, data governance, actionable data, data ethics, data pathways, Earth science data
Citation: Virapongse A, Gupta R, Robbins ZJ, Blythe J, Duerr RE and Gregg C (2022) How Can Earth Scientists Contribute to Community Resilience? Challenges and Recommendations. Front. Clim. 4:761499. doi: 10.3389/fclim.2022.761499
Received: 19 August 2021; Accepted: 31 March 2022;
Published: 09 May 2022.
Edited by:
Nazila Merati, National Oceanic and Atmospheric Administration (NOAA), United StatesReviewed by:
Tasnuva Rouf, University of California, Berkeley, United StatesKripa Jagannathan, Lawrence Berkeley National Laboratory, United States
Mathew Biddle, U.S. Integrated Ocean Observing System, United States
Copyright © 2022 Virapongse, Gupta, Robbins, Blythe, Duerr and Gregg. This is an open-access article distributed under the terms of the Creative Commons Attribution License (CC BY). The use, distribution or reproduction in other forums is permitted, provided the original author(s) and the copyright owner(s) are credited and that the original publication in this journal is cited, in accordance with accepted academic practice. No use, distribution or reproduction is permitted which does not comply with these terms.
*Correspondence: Arika Virapongse, av@middlepatheco.com