- 1Department of Geography, Portland State University, Portland, OR, United States
- 2Water Security and Sustainability Research Initiative, University of California, Merced, Merced, CA, United States
- 3Center for Law, Energy & the Environment, Berkeley School of Law, University of California, Berkeley, Berkeley, CA, United States
- 4Lawrence Berkeley National Laboratory, University of California, Berkeley, Berkeley, CA, United States
- 5School of Law, National University of Ireland Galway, Galway, Ireland
- 6Department of Urban Studies and Planning, Massachusetts Institute of Technology, Cambridge, MA, United States
- 7California Department of Water Resources, Sacramento, CA, United States
- 8School of Engineering, University of California, Merced, Merced, CA, United States
Evidence-based environmental management requires data that are sufficient, accessible, useful and used. A mismatch between data, data systems, and data needs for decision making can result in inefficient and inequitable capital investments, resource allocations, environmental protection, hazard mitigation, and quality of life. In this paper, we examine the relationship between data and decision making in environmental management, with a focus on water management. We focus on the concept of decision-driven data systems—data systems that incorporate an assessment of decision-makers' data needs into their design. The aim of the research was to examine the process of translating data into effective decision making by engaging stakeholders in the development of a water data system. Using California's legislative mandate for state agencies to integrate existing water and other environmental data as a case study, we developed and applied a participatory approach to inform data-system design and identify unmet data needs. Using workshops and focused stakeholder meetings, we developed 20 diverse use cases to assess data sources, availability, characteristics, gaps, and other attributes of data used for representative decisions. Federal and state agencies made up about 90% of the data sources, and could readily adapt to a federated data system, our recommended model for the state. The remaining 10% of more-specialized data, central to important decisions across multiple use cases, would require additional investment or incentives to achieve data consistency, interoperability, and compatibility with a federated system. Based on this assessment, we propose a typology of different types of data limitations and gaps described by stakeholders. We also propose technical, governance, and stakeholder engagement evaluation criteria to guide planning and building environmental data systems. Data-system governance involving both producers and users of data was seen as essential to achieving workable standards, stable funding, convenient data availability, resilience to institutional change, and long-term buy-in by stakeholders. Our work provides a replicable lesson for using decision-maker and stakeholder engagement to shape the design of an environmental data system, and inform a technical design that addresses both user and producer needs.
Introduction
Evidence-based environmental management requires data that are sufficient, accessible, useful and used (California Department of Water Resources, 2020). If data systems are to effectively inform environmental decision making, then development of such systems can be improved through assessment and incorporation of decision-makers' data needs. The concept of data-driven decision making describes the practice of making decisions based on analysis of data (Provost and Fawcett, 2013). In this paper, we develop a related and equally important concept of decision-driven data systems: data systems that are designed based on an understanding of decision-makers' data needs. Development of such systems can be improved through first assessing these needs and then incorporating this assessment into system design and content prioritization.
We define “data systems” broadly as the assemblage of hardware, software, people, and institutions that collect, organize, archive, distribute, integrate, process, analyze, and synthesize data and information. There are a growing number of efforts that seek to advance earth and environmental data systems through integration and collaboration in order to maximize applicability to both research and decision making. For example, National Science Foundation (NSF) has supported Hydroshare, a collaborative environment for sharing hydrologic and critical-zone data and models geared toward research users. In the European Union, the INSPIRE Directive seeks to create a spatial-data infrastructure to inform E.U. environmental policies, and the Copernicus project focuses on meeting earth-science data-user needs. Copernicus developers have created a use case library demonstrating how data are applied to real-world problem solving.
Water management presents an important case for strengthening the relationship between environmental data and decision making. Provisioning and use of adequate information are central to effectively making investments in water infrastructure, confirming environmental regulatory compliance, managing risks and uncertainties, guiding operations, evaluating and encouraging innovation, and making rapid and effective decisions during droughts, floods, or crisis events (Kiparsky et al., 2013; Escriva-Bou et al., 2016; Larsen et al., 2016; Green Nylen et al., 2018a,b). Researchers have worked to strengthen connections between data and decision making related to water. For example, researchers have assessed decision-makers' demand for and use of forecasting data for water resources management (Viel et al., 2016; Neumann et al., 2018). Researchers and computational/data scientists are advancing new approaches to quantify watershed behavior to inform management decisions. Recent examples highlight the promise of machine learning for advancing tractable watershed-data processing, parameter estimation, sensor optimization, early warning, groundwater-level prediction, and process understanding (e.g., Ahmad et al., 2010; Oroza et al., 2016; Pau et al., 2016; Mosavi et al., 2018; Schmidt et al., 2018; Müller et al., 2019). Researchers are also developing watershed-centric data tools that seek to improve integration of data management, analysis, modeling and interpretation of diverse watershed datasets (Varadharajan et al., 2019; Hubbard et al., 2020). These examples indicate significant potential for new tools to aid in the tractable translation of water data into information for decision making.
The complexity of water systems means that managers must integrate and analyze multiple types of data and information (Kallis et al., 2006; Bakker, 2012; Vogel et al., 2015). Modern information technology promises, in concept, to make such multi-faceted integration possible, but providing data does not in and of itself ensure that data can or will be used for more effective and sustainable water management. Here, water data refers to a broad suite of data and information used to inform water-related research and decision making. Water data includes both measured data and model-output data, and can be used both to characterize systems and to monitor conditions over time. Our definition of water data goes beyond hydrologic data such as streamflow, precipitation, and groundwater-level measurements to include many related and relevant areas, such as land use, ecological, and agricultural data. We primarily address public data sources in this paper.
As a case study, we focus on California water, which is one of the most complex and politically contentious environmental management challenges in the world. California's water challenges require a wide range of data to solve problems including managing drought and climate change, balancing environmental and agricultural water demands, and meeting water needs of endangered species and cities alike (Hanak, 2011). Yet despite California's prominence in the technology sphere, the state's water data have not proven up for these challenges (California Council on Science and Technology, 2014; Escriva-Bou et al., 2016). California water data are diverse and fragmented, and are produced, housed, and maintained by multiple entities from disparate sectors. Recent legislation has attempted to address this issue. California's Open and Transparent Water Data Act (Assembly Bill, or AB 1755), passed in 2016 (Cal. Water Code §12,400 et seq.), requires California state agencies to integrate existing water and other environmental data from local, state, and federal agencies for the purpose of creating and maintaining a statewide integrated water data platform. In this research, we developed a process to systematically explore data needs for decision making to inform the design of data systems, focusing on California.
The aim of this paper is to contribute a better understanding of the practice of translating data into effective decision making by engaging stakeholders in data system development. The research has three main contributions. First, we develop the concept of a decision-driven data system, and assess how it might support improvements in informing management across a wide range of environmental sectors. Second, we examine and illustrate the concept's application in the California case study by defining attributes of a user-centered data and information system through stakeholder engagement. Third, we identify and characterize types of data limitations, and evaluate how a decision-driven, user-defined data system can address the data limitations experienced by users.
We first describe our methods, which involved working with stakeholders in California water management to develop and analyze a set of “use cases,” short descriptions of decision making and the data needed to inform those decisions. We then develop a typology of different types of data limitations and gaps described by stakeholders, including gaps in data availability, accessibility, interoperability, and resolution. We propose technical, governance, and stakeholder engagement evaluation criteria to guide planning and building environmental data systems that account for these needs. By developing and describing a method for engaging stakeholders in the development of data systems, this article contributes to a better understanding of a crucial but understudied aspect of the practice of translating data into effective decision making, and offers recommendations applicable to a broad range of environmental and climate data and information systems.
Methods
Leaders from the California Department of Water Resources (DWR), the California Council on Science and Technology (CCST) and researchers from University of California collaborated on a process of engaging stakeholders and evaluating data needs with the goal of ensuring that California's Open and Transparent Water Data Act results in an effective data system that improves water management in practice1. Our stakeholder engagement was centered around identification and analysis of “use cases”—brief descriptions of decision making associated with a specific outcome (such as balancing a basin water budget or responding to a harmful algal bloom) and the data needed to inform those decisions (fully described in Cantor et al., 2018). The idea of use cases was initially articulated in the field of computer sciences, based on the concept of developing data systems by starting with the end users' goals in mind in order to increase efficiency and efficacy (Alexander and Maiden, 2005; Kulak and Guiney, 2012). We adapted the use case approach from computer sciences to first systematically assess the data needs of California's water decision makers and other data users, then evaluate whether existing data and data systems met these needs, and finally to communicate these needs with technical developers of data systems and applications.
Use Case Development
We developed our application of the use case concept in collaboration with technical data system developers as well as data users. To begin, we asked the interrelated questions of who needs what data in what form to make what decisions (Kiparsky and Bales, 2017). We created a template (Table 1) to guide stakeholders in answering these questions in a systematic way, centered around a particular decision or goal.
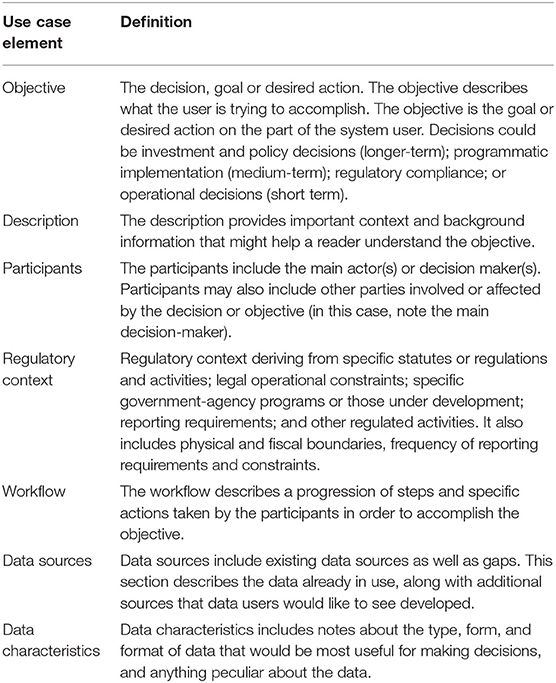
Table 1. Use case template: Elements and definitions of a use case (adapted from Cantor et al., 2018).
Using the template in Table 1, we identified and developed 20 use cases (see Cantor et al., 2018). The use cases were compiled during three full-day-long facilitated workshops as well as additional meetings with stakeholders. We defined “stakeholder” broadly as including data producers and consumers with an interest in the outcomes of California's progress on water data, including academics, state and local agency representatives, non-governmental-organization representatives, community members, the private sector, and other water management practitioners. Workshop participants were selected through purposive sampling (Aarons et al., 2012; Ritchie et al., 2013) based on their relevant experience with data use or production related to the selected use cases.
The first two workshops, which produced eight use cases in total, each included 60–80 attendees. The majority of attendees worked with one of the state agencies named in California's Open and Transparent Water Data Act (AB 1755), so they attended in the capacity of their agencies, which had a direct stake in the process. Other attendees included academics, non-profit organization representatives, and others who saw themselves as having an interest in participating in water data system design and development. Lunch and opportunities for networking were provided as part of the workshops. Workshops began with an overview of the concept of data for decision making and the specific task of informing development of a data system. Participants then formed smaller breakout groups of 10–20 participants to develop use cases on pre-identified topics. Each group was given the use case template (Table 1) and had an assigned facilitator and note taker from the project team. We next identified and developed four additional use cases through a series of more-targeted, facilitated meetings with smaller groups of water data users and data producers with specific subject area expertise (for example, employees at the California State Water Resources Control Board involved in water rights), and worked directly with a range of non-governmental organizations and state agencies to identify and develop the remaining eight use cases using the template. Finally, a third, larger workshop was held toward the end of the use case process to present the initial use cases and findings to ~100 attendees, and to solicit their feedback. The process thus evolved over time—from medium-sized workshops with a variety of water data users, to targeted meetings and one-on-one work to generate specific use cases, to a more general forum to present initial results.
The use cases encompassed a diversity of topics relevant to California water management, including groundwater management, environmental restoration, wetland monitoring, fishery management, urban and agricultural water management, water rights and water availability, capital investment, and drought contingency planning2. For example, some of the specific use case topics included “Management of environmental flows to protect salmon habitat,” “Groundwater basin water budgets,” “Water shortage contingency planning vulnerability assessment,” and “Decision support system for harmful algal bloom response, communication, and mitigation.” To provide a more detailed example, Table 2 shows a completed use case on the topic of groundwater recharge project planning, and Table 3 summarizes the specific data sources listed by stakeholders for this example use case.
While the sample of use cases does not comprehensively represent the entire landscape of California water management (for example, the cases covered many themes related to water quality, habitat, and water allocation, but water treatment utilities were largely unaddressed in the overall use case portfolio), the cases represent the complexity and breadth of water-management topics, and the selection of use cases was deliberately aligned with broader goals for California water (California Natural Resources Agency, 2016).
Analysis of Use Cases
We analyzed the collected use cases to identify patterns. We compiled the data sources listed for each use case and coded them according to thematic categories, including data topic and data provider. At least two members of the research team coded each data source and cross-checked their categorizations to enhance reliability. An emergent coding scheme (Holton, 2007) was used in order to capture the wide range of stakeholder-generated themes that were included in the use cases. Use case information was then cross checked and verified to remove errors and redundancy. We then identified data gaps, which we defined as data that were unavailable, inconsistently available, available only in formats that did not allow for interoperability, or that contained gaps in measurement or analysis. Data gaps were also coded and checked by multiple researchers for reliability. Finally, qualitative comments and feedback were coded using an emergent coding scheme, and were grouped according to themes to better understand stakeholder perspectives (see Cantor et al., 2018 for more detail). These classifications allowed us to systematically examine the availability of data sources, origin of data sources, the thematic topics covered, and gaps in data.
Results
Data Types and Sources
Stakeholders used (or saw potential to use) water-related data for a wide variety of decisions. Some use cases were oriented toward directly answering a question, while other use cases involved collecting and integrating data into models or decision support tools that in turn could be used to inform a number of different decisions. Some use cases focused on high-level investment and policy decisions, some on mid-level programmatic implementation, and others on day-to-day operational decisions, and regulatory compliance. Some cases represented concrete, already-existing decision processes, while others were more aspirational in describing desired goals.
Analysis of the use cases confirmed that water decision makers require a wide diversity of data types. While this may be no surprise to those versed in environmental management, it is important to consider the implications for data-system design. Water decision making requires a variety of data related to various natural, built, and socioeconomic systems in addition to data more traditionally associated with the hydrologic cycle (including precipitation and streamflow, water demand, groundwater, water quality, and water storage data) (Table 4). As illustrated in Table 4, the heterogeneity of data included in the use cases underscores the point that water data systems need to incorporate not only data obviously related to water (e.g., precipitation, streamflow), but also a wide range of related data—from agricultural land use to population data to climate-change projections—to fully support water-related decisions. The diversity of data and their associated spatial and temporal resolutions presents a challenge to data-system designers seeking to prioritize accessibility and interoperability for water decision making.
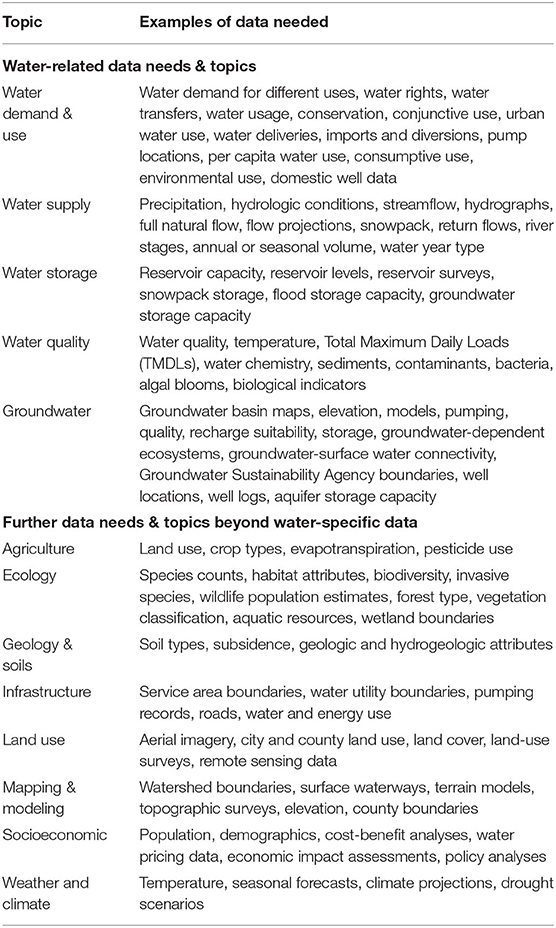
Table 4. Broad range of data needs and topics represented within data needed for water decision making (adapted from Cantor et al., 2018).
A relatively small number of state and federal public agencies provided the bulk of the data: just six federal and state agencies (including, at the federal level, the U.S. Geological Survey, the U.S. Department of Agriculture, and the National Oceanic and Atmospheric Administration, and at the California state level, the Department of Water Resources, the State Water Resource Control Board, and the Department of Fish and Wildlife) provided ~two-thirds of the data sources mentioned by decision makers. Federal and state agencies made up about 90% of the data sources, while a variety of university, private, and non-governmental sources together made up the remaining 10%. Data systems seeking to integrate public data from the full range of federal and state data providers contributing to water management will need to rely upon common data standards between public agencies to ensure interoperability—a large task currently underway in California. At the same time, there was a long list of more specialized data that were cited for specific use in a single case. Water data users drew not only from public data from state and federal agencies, but also from a wide range of less-frequently-used other sources that were still highly important in certain decisions.
Data Limitations
Stakeholder input and use cases revealed significant limitations in data and information availability (Figure 1). Some critical data were not available at all (limitation type 1). For example, data about groundwater extraction by individual water users was not systematically collected. As another example, data related to water demand by different interests such as recreation, or socioeconomic data such as valuation by different interests, pricing, or willingness to pay, was not readily available.
Other data were inaccessible or hard to use (limitation type 2). For example, some datasets were only published as PDF files or were not machine readable, and other data were password protected, required a fee to access, or were otherwise inaccessible. Other data had been transformed into maps or visualization tools, but the underlying data were not readily available. In one notable example, most information on California water rights only existed in paper form in a vault in the state capitol, rather than in an accessible digital database (although there have since been efforts to digitize this information).
Other data had low interoperability (limitation type 3). For example, stakeholders described datasets that were collected for specific purposes and were therefore not intended for interoperability. Multiple data producers had their own processes for data collection, storage, and documentation. The result was that data and IT systems could not exchange information with each other in standard ways allowing for comparison, aggregation, and analysis.
Finally, some data were not gathered using standardized approaches, or were not collected at useful time intervals or consistent spatial resolutions (limitation type 4). For example, data can be collected seasonally, monthly, or daily but this may not line up with decision-making needs. As another specific example, the California Department of Water Resources divides California into different hydrologic regions, but these boundaries did not exactly match USGS hydrologic boundaries, making it difficult to integrate multiple data sets.
Limitations in accessibility, interoperability, and resolution (types 2, 3, and 4) mean that some data sources can effectively constitute data gaps even if data technically exist.
Discussion
Scholarship from environmental science and management has outlined guiding principles for how data can ideally guide decision making (Cortner, 2000; Cash et al., 2003; Holmes and Clark, 2008; Lemos and Rood, 2010). Data and information, beyond providing a snapshot of the state of the environment, should be useful, which refers to functionality and desirability for decision makers, as well as usable, which refers to how well data inform decision making processes in practice (Lemos and Rood, 2010). Data and information must also be salient (relevant to decision makers), credible (accurate from a scientific perspective), and legitimate (produced in a way that is perceived as respectful, unbiased, and fair) (Cash et al., 2003).
In this paper, we apply these principles to the mechanisms through which data are stored, published, accessed, and used. Drawing from our stakeholder engagement and analysis, we identified three categories of considerations for developing useful and usable water data systems that are salient, credible, and legitimate: (1) technical elements, including data interoperability, spatiotemporal resolution, documentation and quality; (2) governance, including funding and operating of systems across institutions; and (3) stakeholder engagement. Here we discuss each of these categories, then use them to inform criteria to evaluate a water data system.
Technical Considerations
Most of the use cases in our analysis integrated multiple data sources spanning a variety of thematic categories and sourced from a range of different data providers. The extraordinary heterogeneity of water data (Table 4) reflects how water decisions must often consider hydrologic, ecological, climate and other natural-system phenomena (e.g., streamflow, groundwater levels, species abundance, temperature, etc.) as well as characteristics associated with human and built systems (e.g., land use, crop types, built infrastructure, etc.). It also reflects institutional realities: water data are produced, housed, and maintained by multiple entities from disparate sectors.
Our analysis showed that there are significant limitations in data availability (Figure 1), including non-existent data and available but difficult-to-access data. Interoperability (limitation type 3) presented a particularly significant problem, and based on our analysis, it became evident that interoperability of multiple data sources from different providers is key to the success of an environmental data system (Figure 1). The current lack of uniform, accessible, interoperable, and ultimately usable data hampers evidence-based water management in California (Escriva-Bou et al., 2016). Datasets are produced for a variety of primary purposes, and thus do not always share metadata or data-quality standards. Given our finding that a relatively small number of state and federal agencies provided a large fraction of needed data, there is significant potential for interoperability to improve by focusing on those agencies. Stakeholders also noted challenges related to spatial and temporal resolution of data collection (limitation type 4), which are related to interoperability (Gibson et al., 2000).
To address the interoperability challenge, participants in our project discussed the relative benefits of centralized vs. federated data systems. A centralized system such as those used by multiple federal agencies can readily implement uniform data standards and respond to diverse user needs. Yet federated data systems were preferred by many participants. Federated data systems connect multiple independent data systems through common standards, conventions, and protocols, while keeping those independent systems autonomous (Busse et al., 1999; Blodgett et al., 2016). Our research showed that data users relied upon a wide range of data produced and distributed by a variety of state and federal agencies and other data producers. Given the reliance on a range of distributed data sources from independent organizations, a federated data system may have advantages. A successful interoperable federated system requires clear standards for data quality, metadata, and technical requirements. Standards do not have to be created from scratch: for example, projects such as Hydroshare and the Environmental Systems Science Data Infrastructure for a Virtual Ecosystem (ESS-DIVE), a cyberinfrastructure system to integrate diverse environmental datasets, have laid significant groundwork for methods to define and store metadata (Peckham and Goodall, 2013; Agarwal et al., 2017; Varadharajan et al., 2019). Here, it is worth highlighting the importance of clear standards, as data managers across different agencies and organizations may believe their standards are aligned but in practice, they may not be aligned sufficiently to support an effective federated system.
Workshop participants emphasized the importance of traceability, clear identification of sources, and documentation of uncertainties, all of which contribute to an assessment of data limitations (Figure 1). A data system drawing from multiple sources requires clear protocols for data quality assurance and documentation throughout all stages of the data life cycle. Structuring data according to set standards can facilitate integration between multiple data providers (Blodgett et al., 2016). Georeferencing of data is also critical for many water-related analyses. Archiving practices also require thought, as they are important to prevent data losses. One solution is the use of unique digital object identifiers (DOIs) for data sets (Paskin, 2010; Wilkinson et al., 2016), which can address traceability concerns by ensuring that data sets persist even if websites are reorganized and can assist with versioning, quality assistance/quality control, and referencing. For continually updated datasets, making versioned DOI sets of data would be a helpful best practice across agencies.
The range of use cases identified in this research also showed that different data users need data in different formats. In some cases, stakeholders and researchers preferred raw data which they could analyze and translate themselves into information. In other cases, stakeholders required quality-controlled data with transformed formats that could be readily input into decision-support systems, hydrologic models, workflows, visualization software, water-budget calculation, or other analytical tools.
Governance Considerations
Open data are important for sustainable and inclusive environmental management and water governance in particular (De Stefano et al., 2012; Chini and Stillwell, 2020), and can help make environmental governance more transparent, accountable, and efficient (Blodgett et al., 2016; Mayton and Story, 2018). Stakeholders in our research emphasized that developing and maintaining an open and transparent water data system requires not just making existing data more readily available, but also requires thoughtful governance and sustainable funding. Strategies for generating a sustainable funding source and governance model for a water data system have been proposed and adopted by the state of California. These involve a consortium of state, NGO, and private-sector actors working collaboratively (Huttner et al., 2018).
Participants in our stakeholder engagement noted that resources are needed throughout the information pipeline: this includes data system design, quality control, decision support and analysis tools, archiving, user support and continued system innovation. Building and maintaining a sustainable data system will therefore require investment in addressing limitations in data availability, accessibility, interoperability and resolution (Figure 1). To maximize usability over time, long-term funding models must be carefully thought out, with special consideration given to openness of data systems. Again, a federated system has benefits in this area: while a federated system with multiple funding streams may be vulnerable to losing one or more data streams, it also provides resilience by being distributed. It can also incorporate incremental additions from legislative actions that introduce new data sources or systems that meet new or emerging needs.
In addition to funding, an effective data system relies upon robust institutions to coordinate decision making and actions around how the data system is structured and used (Huttner et al., 2018). A framework that does not address institutional concerns increases the risk of data system failure from lack of coordination, underinvestment, or lack of trust and buy-in. Stakeholders noted the importance of trust, confidence, and credibility within and between institutions, which are widely recognized as important in water resources management generally, but can be forgotten when the focus is on the technical aspects of data systems (Jackson, 2006).
Data systems benefit from participation of data providers because their adherence to standards is important for interoperability and their involvement in those standards is a way to facilitate that adherence. Governance mechanisms such as mandates for incorporating standard metadata and data-quality procedures could help ensure that agencies participate in a federated system. The bulk of the data used by stakeholders in our analysis came from public agencies. Legislative and regulatory mandates could be a way to encourage participation of these agencies. Still, a large handful of data sources identified as useful or necessary came from a wide variety of non-governmental stakeholders. Such smaller data providers may require incentives to fully participate in a system if adhering to protocols involves costs. For example, “intervener funding” (financial support that helps stakeholders to effectively participate in agency proceedings) could help support engagement of non-governmental data producers (Kiparsky et al., 2016). Another mechanism to encourage participation could involve requiring that state-funded projects make data interoperable and publicly available (similar to current National Science Foundation requirements for data management plans and data publication).
This raises a particular conundrum for environmental data systems design: the distinction between public and non-public data. While it may be possible (although far from straightforward) to require openness and transparency of data from federal, state, and local agencies, there remains a large category of non-public data. Other sources of data include nonprofit data sources, but also private data sources that present additional complications with regards to openness and transparency. It also may be more difficult to enact requirements or incentives for interoperability with these non-public data sources, meaning that they are likely to be more difficult to integrate, even though they may provide valuable information.
Stakeholder Engagement
Ensuring that an environmental data system is sufficient, accessible, useful and used (California Department of Water Resources, 2020) hinges on meaningful, ongoing relationships with data users. Successful stakeholder engagement requires many things: recognition of common goals, time to develop functional relationships, common vocabulary, careful facilitation and ongoing maintenance of relationships, and resources. Developing environmental data systems that are sufficient, accessible, useful, and used requires both usable technical cyberinfrastructure, good governance, and funding sufficient to support both technical infrastructure and governance.
We found that engaging knowledgeable stakeholders with detailed understanding of data needs and workflows involved in different aspects of water-related decision making is essential to identifying key aspects of data system usability. We also note the importance of engaging those who hold a stake in water decisions but do not have in-depth technical knowledge. To support communication, we used professional facilitation in larger meetings to ensure that project goals were articulated clearly and concisely. We also found it useful to engage stakeholders through different formats to serve different project goals. Larger workshops were helpful in communicating overall aims to a broader audience, including those with influence over policy decisions. Smaller meetings enabled focused conversations with specific groups of people with targeted technical knowledge. Working directly with organizations to identify use cases was an effective way to engage additional stakeholders.
User-focused data-system development can thus be framed as an adaptive management cycle (Pahl-Wostl, 2007) that includes multiple iterations of planning, implementation, and evaluation. Stakeholder engagement should be formally integrated into this cycle from an early stage to increase usability of the data system (Welp et al., 2006; Reed, 2008). Because decision-maker needs and technological capacities change over time, a data system must be adaptable (McNie, 2007; Hanseth and Lyytinen, 2016), and as new decision-maker needs and new technologies arise, a data system must evolve to remain useful. The process of identifying stakeholder objectives, translating these objectives into functional and technical requirements, and using these objectives to inform the development of data systems, can be built into the life cycle of data system design.
Evaluating Decision-Driven Data Systems
To integrate the technical, governance, and stakeholder-engagement considerations identified during our research and outlined here, we propose a set of questions to guide evaluating the success of an environmental data system (Table 5). This set of evaluation criteria incorporates the multiple types of data limitations identified in this paper (see Figure 1) and includes technical considerations, governance considerations, and stakeholder engagement considerations.
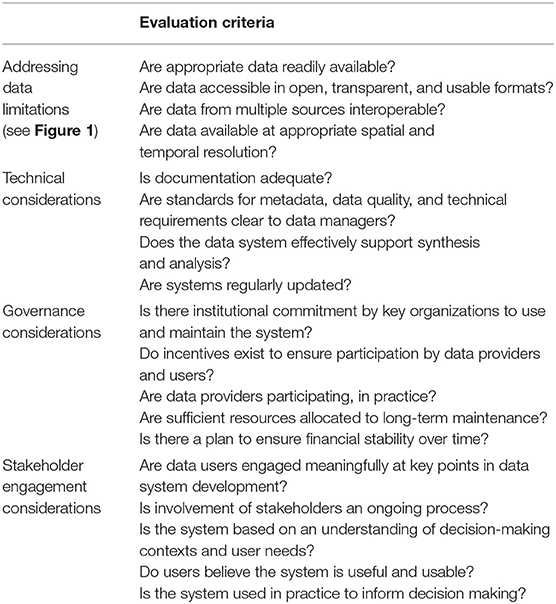
Table 5. Proposed criteria for evaluating success of an environmental data system (adapted from Cantor et al., 2018).
These evaluation questions are in line with those developed by others, such as the “FAIR” (Findable, Accessible, Interoperable, Reusable) Guiding Principles (Wilkinson et al., 2016), but also add to these guiding principles through inclusion of governance and stakeholder engagement criteria, which we argue are crucial to data system success and should therefore be included alongside the more technical considerations. These questions are targeted at data providers, although many of the evaluation questions require the input of data users. The questions do not provide quantitative measurements or metrics, which would need to be specific to an individual data system; instead, these questions provide a guide for data providers to consider how well their system is serving users. Our evaluation criteria include the very important question of whether the data system is ultimately used in practice to inform decision making—perhaps the key indicator of success.
A crucial indicator of the success of our process can be found in the formal uptake of the concepts of decision-driven water data systems into state processes required by statute (California Department of Water Resources, 2020). Based on the results of our workshops and analysis, our recommendation of a federated, use case-driven water data platform that connects independent databases while prioritizing and managing data based on how data will be used has been adopted by California's AB 1755 Partner Agency Team. Another indicator of success is in the influence of other subsequent processes. For example, organizers of a recent workshop on water data in Texas used a use case approach based on our template and model (Rosen and Roberts, 2018). Drawing from our approach, the Texas workshop organizers also started from the basic principle that water data systems must be responsive to stakeholder needs in order to support decision making in practice (Rosen and Roberts, 2018).
Challenges and Limitations
In the course of our study, we experienced inevitable obstacles related to the challenges of working with stakeholders. We found that (as might be expected) engaging with stakeholders meaningfully is time consuming and takes resources, and it is important not to underestimate the capacity needed to conduct effective stakeholder engagement. We also learned that developing a sufficiently clear articulation of an objective or decision around which to anchor a use case was not a simple task. In practice, it proved difficult for larger groups with greater diversity in their topical expertise to agree upon objectives. At the same time, engaging participants in groups helped ensure that different stakeholders with various types of expertise could provide different types of knowledge.
The work presented in this paper has several limitations. First, many problems in the water sector are highly complex. They may involve multiple levels or stages of decisions: in this project we mainly tested the use case approach on single-stage decisions and the concept would need to be adapted or used iteratively to account for multi-stage decisions. Second, the use case framework is helpful for identifying data gaps, but does not necessarily provide a mechanism for evaluating the relevance or significance of such gaps. That is, some limitations represent a critical bottleneck to decision processes, while other limitations do not actively constrain decisions from going forward but still impact the quality of those decisions. Future efforts to implement use cases and identify data limitations could ask participants about the relative impact of a particular data limitation. Third, we developed this methodology with the creation of a new data system in mind; we did not test the applicability of the methodology to existing data systems that already have established formats and tools. Future work could test our proposed evaluation criteria by applying it to an existing system. Finally, given growing interest in water data from global organizations (for example, the World Water Data Initiative, led by the World Meteorological Organization) there may be opportunity for future research to examine how these concepts apply to different scales.
We also acknowledge that conflicts in water management go beyond data. Water issues and proposed solutions frequently evoke controversy and can be hotly contested. In this project we did not directly address the complex politics and disagreements between different stakeholder groups that frequently emerge in environmental governance and problem-solving. While data can, ideally, help inform and evaluate solutions to difficult and controversial issues, we recognize that lack of data is not the only issue preventing good water governance, and that conflict will not be resolved solely through data availability.
Conclusions
Applying the concept of decision-driven data systems to environmental management is an important contribution to the overarching goal of enhancing data-informed environmental decision making. Our case study of water data in California identified specific ways in which less-than-adequate data sources and systems are currently constraining decision making, resulting in data gaps, ineffective delivery of overlapping data needs across sectors, and limiting secondary uses of data. Based on this research, we argue that to effectively inform water management, data systems must begin with a strong understanding of decision makers' data needs, and should engage decision makers to identify and address different types of data gaps and limitations. Otherwise, data systems risk being of limited utility, an inefficient use of resources, and a source of frustration for users.
Our work shows that useful and usable environmental data systems must consider not only technical elements, but also data system governance and stakeholder engagement. In the case we examined, given the distributed nature of data required by stakeholders, the independence of disparate agencies, and the need for interoperability, federated data systems have the potential to address technical and governance issues. In terms of stakeholder engagement, a responsive data system requires ongoing analysis of stakeholder objectives and translation of those objectives into functional and technical requirements. Resources for engagement should be considered part of infrastructure investment, because they ultimately can help inform usability of a data system and prevent wasting future resources.
Supporting environmental decision making through decision-driven data systems is a long-term project involving ongoing attention to meaningful engagement with decision makers and other data stakeholders. As is true of other forms of infrastructure, the full value of investments in environmental data may only become apparent when it is sorely needed: for example, the value of water data becomes apparent during droughts, floods, or other crisis events. In such events, access to information may be a crucial factor in determining whether or not rapid and effective decisions can be reached. This prospect alone justifies the forward-looking efforts described in this article, and, more generally, greater attention to the role of data in environmental management and sustainability.
Data Availability Statement
A full, detailed compilation of all 20 use cases developed for this project and the specific data sources associated with each is available online at: https://doi.org/10.15779/J28H01. Further inquiries can be directed to the corresponding author.
Ethics Statement
Ethical review and approval was not required for the study on human participants in accordance with the local legislation and institutional requirements. Written informed consent for participation was not required for this study in accordance with the national legislation and the institutional requirements.
Author Contributions
AC: conceptualization, methodology, investigation, data curation, analysis, and writing—original draft. MK: conceptualization, methodology, investigation, analysis, writing—original draft, supervision, project administration, and funding acquisition. SH and RK: conceptualization and writing—review and editing. LP: analysis, data curation, and writing—review and editing. KG: project administration, investigation, and writing—review and editing. GD and CM: resources, investigation, and writing—review and editing. RB: conceptualization, supervision, project administration, funding acquisition, and writing—review and editing. All authors contributed to the article and approved the submitted version.
Funding
This work was supported by the University of California Office of the President (UCOP), through the UC Water Security and Sustainability Research Initiative (UCOP Grant No. 13941), and by the Water Foundation. Support for SH was provided by U.S. Department of Energy, Office of Science, Office of Biological and Environmental Research under Award Number DE-AC02-05CH1123.
Conflict of Interest
The authors declare that the research was conducted in the absence of any commercial or financial relationships that could be construed as a potential conflict of interest.
Publisher's Note
All claims expressed in this article are solely those of the authors and do not necessarily represent those of their affiliated organizations, or those of the publisher, the editors and the reviewers. Any product that may be evaluated in this article, or claim that may be made by its manufacturer, is not guaranteed or endorsed by the publisher.
Acknowledgments
An earlier report on this study, including a complete description of the California use case development process can be found in a 2018 report written by the authors of this article and published as a report by UC Berkeley School of Law, Center for Law, Energy & the Environment. The 2018 report is available at https://doi.org/10.15779/J28H01. Thanks to the workshop participants, facilitators, and use case contributors for sharing their time and expertise. We thank workshop sponsors, including the California Council on Science and Technology (CCST), UC Water, Lawrence Berkeley National Laboratory, the California Department of Water Resources, and the Water Foundation, Leigh Bernacchi, Luke Sherman, and Amber Mace for assistance in organizing the workshops, John Helly, Richard Roos-Collins, Holly Doremus, and Nell Green Nylen for discussions on the concepts presented in this paper, and reviewers for helpful comments on versions of this paper.
Footnotes
1. ^In this article, we build on and extend a 2018 report published by the Center for Law, Energy & the Environment at Berkeley Law, available at: https://doi.org/10.15779/J28H01. The initial report was published as a white paper intended largely for a California-based water policy and decision-maker audience. In this article, we strive to speak to a broader scholarly audience by expanding the theoretical framing, putting key ideas from the 2018 report into a more in-depth conversation with scholarly literature, extending the generalizable observations, and more fully developing and discussing the typology of data limitations.
2. ^A full, detailed compilation of all 20 use cases and the specific data sources associated with each is available online at: https://doi.org/10.15779/J28H01.
References
Aarons, G. A., Fettes, D. L., Sommerfeld, D. H., and Palinkas, L. A. (2012). Mixed methods for implementation research: application to evidence-based practice implementation and staff turnover in community-based organizations providing child welfare services. Child Maltreat. 17, 67–79. doi: 10.1177/1077559511426908
Agarwal, D., Varadharajan, C., Cholia, S., Snavely, C., Hendrix, V., Gunter, D., et al. (2017). “Environmental System Science Data Infrastructure for a Virtual Ecosystem (ESS-DIVE)-a new US DOE data archive,” in American Geophysical Union Fall Meeting (New Orleans, LA).
Ahmad, S., Kalra, A., and Stephen, H. (2010). Estimating soil moisture using remote sensing data: a machine learning approach. Adv. Water Resour. 33, 69–80. doi: 10.1016/j.advwatres.2009.10.008
Alexander, I. F., and Maiden, N. (2005). Scenarios, Stories, Use Cases: Through the Systems Development Life-Cycle. Hoboken, NJ: John Wiley & Sons.
Bakker, K. (2012). Water security: research challenges and opportunities. Science (80-.) 337, 914–915. doi: 10.1126/science.1226337
Blodgett, D., Read, E., Lucido, J., Slawecki, T., and Young, D. (2016). An analysis of water data systems to inform the open water data initiative. JAWRA 52, 845–858. doi: 10.1111/1752-1688.12417
Busse, S., Kutsche, R. D., Leser, U., and Weber, H. (1999). Federated information systems: Concepts, terminology and architectures. Forschungsberichte des Fachbereichs Informatik 99, 1–38.
California Council on Science and Technology (2014). Achieving a Sustainable California Water Future Through Innovations in Science and Technology. Sacramento, CA.
California Department of Water Resources (2020). Open and Transparent Water Data Act- Implementation Journal. Sacramento, CA.
Cantor, A., Kiparsky, M., Kennedy, R., Hubbard, S., Bales, R., Pecharroman, L. C., et al. (2018). Data for Water Decision Making: Informing the Implementation of California's Open and Transparent Water Data Act through Research and Engagement. Berkeley, CA: UC Berkeley Law, Center for Law, Energy & the Environment.
Cash, D. W., Clark, W. C., Alcock, F., Dickson, N. M., Eckley, N., Guston, D. H., et al. (2003). Knowledge systems for sustainable development. Proc. Natl. Acad. Sci. 100, 8086–8091. doi: 10.1073/pnas.1231332100
Chini, C. M., and Stillwell, A. S. (2020). Envisioning blue cities: urban water governance and water footprinting. J. Water Resour. Plan. Manag. 146:4020001. doi: 10.1061/(ASCE)WR.1943-5452.0001171
Cortner, H. J. (2000). Making science relevant to environmental policy. Environ. Sci. Policy 3, 21–30. doi: 10.1016/S1462-9011(99)00042-8
De Stefano, L., Hernández-Mora, N., López Gunn, E., Willaarts, B., and Zorrilla-Miras, P. (2012). “Public participation and transparency in water management,” in Water, agriculture and the environment in Spain: Can we square the circle?, eds L. De Stefano and M. Ramon Llamas (Boca Raton, FL: CRC Press/Balkema; Taylor & Francis Group), 217–225. doi: 10.1201/b13078-22
Escriva-Bou, A., McCann, H., Hanak, E., Lund, J., and Gray, B. (2016). Accounting for California's Water. San Francisco, CA: Public Policy Institute of California. doi: 10.5070/P2CJPP8331936
Gibson, C. C., Ostrom, E., and Ahn, T.-K. (2000). The concept of scale and the human dimensions of global change: a survey. Ecol. Econ. 32, 217–239. doi: 10.1016/S0921-8009(99)00092-0
Green Nylen, N., Kiparsky, M., Owen, D., Doremus, H., and Hanemann, M. (2018a). Addressing Institutional Vulnerabilities in California's Drought Water Allocation, Part 1: Water Rights Administration and Oversight During Major Statewide Droughts, 1976–2016. Berkeley, CA: California's Fourth Climate Change Assessment, California Natural Resources Agency.
Green Nylen, N., Kiparsky, M., Owen, D., Doremus, H., and Hanemann, M. (2018b). Addressing Institutional Vulnerabilities in California's Drought Water Allocation, Part 2: Improving Water Rights Administration and Oversight for Future Droughts. Berkeley, CA: UC Berkeley Law, Center for Law, Energy & the Environment.
Hanak, E. (2011). Managing California's Water: From Conflict to Reconciliation. San Francisco, CA: Public Policy Institute of CA.
Hanseth, O., and Lyytinen, K. (2016). “Design theory for dynamic complexity in information infrastructures: the case of building internet,” in Enacting Research Methods in Information Systems (Berlin: Springer), 104–142. doi: 10.1007/978-3-319-29272-4_4
Holmes, J., and Clark, R. (2008). Enhancing the use of science in environmental policy-making and regulation. Environ. Sci. Policy 11, 702–711. doi: 10.1016/j.envsci.2008.08.004
Holton, J. A. (2007). “The coding process and its challenges,” in The SAGE Handbook of Grounded Theory, ed K.Charmaz (Thousand Oaks, CA: SAGE Publications), 265–290. doi: 10.4135/9781848607941.n13
Hubbard, S. S., Varadharajan, C., Wu, Y., Wainwright, H., and Dwivedi, D. (2020). Emerging technologies and radical collaboration to advance predictive understanding of watershed hydrobiogeochemistry. Hydrol. Process. 34, 3175–3182. doi: 10.1002/hyp.13807
Huttner, N., King, K., and Whitney, J. (2018). Governance and Funding for Open and Transparent Water Data. Redwood City, CA: Redstone Strategy Group.
Jackson, S. (2006). “Water models and water politics: design, deliberation, and virtual accountability,” in Proceedings of the 2006 International Conference on Digital Government Research (San Diego, CA: Digital Government Society of North America), 95–104. doi: 10.1145/1146598.1146632
Kallis, G., Kiparsky, M., Milman, A., and Ray, I. (2006). Glossing over the complexity of water. Science (80-.) 314, 1387–1388. doi: 10.1126/science.314.5804.1387c
Kiparsky, M., and Bales, R. (2017). Advanced data would improve how California manages water. Sacramento Bee.
Kiparsky, M., Owen, D., Green Nylen, N., Doremus, H., Christian-Smith, J., Cosens, B., et al. (2016). Designing Effective Groundwater Sustainability Agencies: Criteria for Evaluation of Local Governance Options. Berkeley, CA: UC Berkeley Law, Center for Law, Energy & the Environment.
Kiparsky, M., Sedlak, D. L., Thompson, B. H. Jr, and Truffer, B. (2013). The innovation deficit in urban water: the need for an integrated perspective on institutions, organizations, and technology. Environ. Eng. Sci. 30, 395–408. doi: 10.1089/ees.2012.0427
Larsen, S., Hamilton, S., Lucido, J., Garner, B., and Young, D. (2016). Supporting diverse data providers in the open water data initiative: communicating water data quality and fitness of use. JAWRA 52, 859–872. doi: 10.1111/1752-1688.12406
Lemos, M. C., and Rood, R. B. (2010). Climate projections and their impact on policy and practice. Wiley Interdiscip. Rev. Clim. Chang. 1, 670–682. doi: 10.1002/wcc.71
Mayton, H., and Story, S. D. (2018). Identifying common ground for sustainable water data management: the case of California. Water Policy 20, 1191–1207. doi: 10.2166/wp.2018.047
McNie, E. C. (2007). Reconciling the supply of scientific information with user demands: an analysis of the problem and review of the literature. Environ. Sci. Policy 10, 17–38. doi: 10.1016/j.envsci.2006.10.004
Mosavi, A., Ozturk, P., and Chau, K. (2018). Flood prediction using machine learning models: literature review. Water 10:1536. doi: 10.3390/w10111536
Müller, J., Park, J., Sahu, R., Varadharajan, C., Arora, B., Faybishenko, B., et al. (2019). Surrogate Optimization of Deep Neural Networks for Groundwater Predictions. arxiv [preprint].arxiv:1908.10947.
Neumann, J., Arnal, L., Emerton, R., Griffith, H., Hyslop, S., Theofanidi, S., et al. (2018). Can seasonal hydrological forecasts inform local decisions and actions? A decision-making activity. Geosci. Commun. 1, 35–57. doi: 10.5194/gc-1-35-2018
Oroza, C. A., Zheng, Z., Glaser, S. D., Tuia, D., and Bales, R. C. (2016). Optimizing embedded sensor network design for catchment-scale snow-depth estimation using LiDAR and machine learning. Water Resour. Res. 52, 8174–8189. doi: 10.1002/2016WR018896
Pahl-Wostl, C. (2007). Transitions towards adaptive management of water facing climate and global change. Water Resour. Manag. 21, 49–62. doi: 10.1007/s11269-006-9040-4
Paskin, N. (2010). Digital object identifier (DOI®) system. Encycl. Libr. Inf. Sci. 3, 1586–1592. doi: 10.1081/E-ELIS3-120044418
Pau, G. S. H., Shen, C., Riley, W. J., and Liu, Y. (2016). Accurate and efficient prediction of fine-resolution hydrologic and carbon dynamic simulations from coarse-resolution models. Water Resour. Res. 52, 791–812. doi: 10.1002/2015WR017782
Peckham, S. D., and Goodall, J. L. (2013). Driving plug-and-play models with data from web services: a demonstration of interoperability between CSDMS and CUAHSI-HIS. Comput. Geosci. 53, 154–161. doi: 10.1016/j.cageo.2012.04.019
Provost, F., and Fawcett, T. (2013). Data science and its relationship to big data and data-driven decision making. Big data 1, 51–59. doi: 10.1089/big.2013.1508
Reed, M. S. (2008). Stakeholder participation for environmental management: a literature review. Biol. Conserv. 141, 2417–2431. doi: 10.1016/j.biocon.2008.07.014
Ritchie, J., Lewis, J., Nicholls, C. M., and Ormston, R. (2013). Qualitative Research Practice: A Guide for Social Science Students and Researchers. Thousand Oaks, CA: Sage Publications.
Rosen, R. A., and Roberts, S. V. (2018). Connecting Texas Water Data Workshop: Building an Internet for Water. San Antonio, TX: Water Resources Science and Technology Book and E-Book Publications and Reports.
Schmidt, F., Wainwright, H. M., Faybishenko, B., Denham, M., and Eddy-Dilek, C. (2018). In situ monitoring of groundwater contamination using the Kalman filter. Environ. Sci. Technol. 52, 7418–7425. doi: 10.1021/acs.est.8b00017
Varadharajan, C., Agarwal, D. A., Brown, W., Burrus, M., Carroll, R. W. H., Christianson, D. S., et al. (2019). Challenges in building an end-to-end system for acquisition, management, and integration of diverse data from sensor networks in watersheds: lessons from a mountainous community observatory in East River, Colorado. IEEE Access 7, 182796–182813. doi: 10.1109/ACCESS.2019.2957793
Viel, C., Beaulant, A.-L., Soubeyroux, J.-M., and Céron, J.-P. (2016). How seasonal forecast could help a decision maker: an example of climate service for water resource management. Adv. Sci. Res. 13, 51–55. doi: 10.5194/asr-13-51-2016
Vogel, R. M., Lall, U., Cai, X., Rajagopalan, B., Weiskel, P. K., Hooper, R. P., et al. (2015). Hydrology: the interdisciplinary science of water. Water Resour. Res. 51, 4409–4430. doi: 10.1002/2015WR017049
Welp, M., de la Vega-Leinert, A., Stoll-Kleemann, S., and Jaeger, C. C. (2006). Science-based stakeholder dialogues: theories and tools. Glob. Environ. Chang. 16, 170–181. doi: 10.1016/j.gloenvcha.2005.12.002
Keywords: water management, data systems, stakeholder engagement, environmental decision making, California
Citation: Cantor A, Kiparsky M, Hubbard SS, Kennedy R, Pecharroman LC, Guivetchi K, Darling G, McCready C and Bales R (2021) Making a Water Data System Responsive to Information Needs of Decision Makers. Front. Clim. 3:761444. doi: 10.3389/fclim.2021.761444
Received: 19 August 2021; Accepted: 07 October 2021;
Published: 17 November 2021.
Edited by:
Tiffany C. Vance, U.S. Integrated Ocean Observing System, United StatesReviewed by:
Nancy Wilkinson, San Francisco State University, United StatesAustin Becker, University of Rhode Island, United States
Copyright © 2021 Cantor, Kiparsky, Hubbard, Kennedy, Pecharroman, Guivetchi, Darling, McCready and Bales. This is an open-access article distributed under the terms of the Creative Commons Attribution License (CC BY). The use, distribution or reproduction in other forums is permitted, provided the original author(s) and the copyright owner(s) are credited and that the original publication in this journal is cited, in accordance with accepted academic practice. No use, distribution or reproduction is permitted which does not comply with these terms.
*Correspondence: Alida Cantor, acantor@pdx.edu