- 1School of Environmental Sciences, University of Liverpool, Liverpool, United Kingdom
- 2LOCEAN-IPSL, Sorbonne Université, CNRS, IRD, MNHN, Paris, France
- 3LMD-IPSL, Ecole normale supérieure / Université PSL, CNRS, Sorbonne Université, Ecole Polytechnique, Paris, France
- 4Ocean Modeling and Data Assimilation Division, Fondazione Centro Euro-Mediterraneo sui Cambiamenti Climatici, Bologna, Italy
- 5Institute for the Oceans and Fisheries, The University of British Columbia, Vancouver, BC, Canada
- 6UMR9190 Centre Pour la Biodiversité Marine, l'Exploitation et la Conservation, University of Montpellier, CNRS, IFREMER, IRD, Sete, France
Ocean net primary production (NPP) results from CO2 fixation by marine phytoplankton, catalysing the transfer of organic matter and energy to marine ecosystems, supporting most marine food webs, and fisheries production as well as stimulating ocean carbon sequestration. Thus, alterations to ocean NPP in response to climate change, as quantified by Earth system model experiments conducted as part of the 5th and 6th Coupled Model Intercomparison Project (CMIP5 and CMIP6) efforts, are expected to alter key ecosystem services. Despite reductions in inter-model variability since CMIP5, the ocean components of CMIP6 models disagree roughly 2-fold in the magnitude and spatial distribution of NPP in the contemporary era, due to incomplete understanding and insufficient observational constraints. Projections of NPP change in absolute terms show large uncertainty in CMIP6, most notably in the North Atlantic and the Indo-Pacific regions, with the latter explaining over two-thirds of the total inter-model uncertainty. While the Indo-Pacific has previously been identified as a hotspot for climate impacts on biodiversity and fisheries, the increased inter-model variability of NPP projections further exacerbates the uncertainties of climate risks on ocean-dependent human communities. Drivers of uncertainty in NPP changes at regional scales integrate different physical and biogeochemical factors that require more targeted mechanistic assessment in future studies. Globally, inter-model uncertainty in the projected changes in NPP has increased since CMIP5, which amplifies the challenges associated with the management of associated ecosystem services. Notably, this increased regional uncertainty in the projected NPP change in CMIP6 has occurred despite reduced uncertainty in the regional rates of NPP for historical period. Improved constraints on the magnitude of ocean NPP and the mechanistic drivers of its spatial variability would improve confidence in future changes. It is unlikely that the CMIP6 model ensemble samples the complete uncertainty in NPP, with the inclusion of additional mechanistic realism likely to widen projections further in the future, especially at regional scales. This has important consequences for assessing ecosystem impacts. Ultimately, we need an integrated mechanistic framework that considers how NPP and marine ecosystems respond to impacts of not only climate change, but also the additional non-climate drivers.
Importance of Ocean Net Primary Production to Ocean Ecosystem Services
Photosynthesis by marine phytoplankton drives ocean net primary production (NPP, defined usually as photosynthesis minus respiration), which is a major planetary flux, accounting for half of global-scale photosynthesis (Field, 1998) and supporting major ocean ecosystem services. As a de novo source of fixed organic carbon, NPP primes the biological carbon pump and carbon sequestration, as well as supporting ecosystem structure and function (Falkowski et al., 2003; Bindoff et al., 2019). As such, changes to NPP in response to changes in climate act alongside other direct climate impacts, such as warming, to catalyse the response of these key services (Cheung et al., 2009; Lotze et al., 2019; Boyce et al., 2020; Tagliabue et al., 2020).
The net consumption of inorganic carbon and the production of particulate organic carbon during NPP perturbs the air-sea CO2 partial pressure gradient, influencing atmosphere ocean carbon dioxide exchange. Subsequent transfer of this particulate organic carbon into the ocean interior via an array of particle injection pumps sequester carbon away from the atmosphere over various timescales (Boyd et al., 2019) and thus has an acknowledged major role in the global carbon cycle (Volk and Hoffert, 1985; Ito and Follows, 2005; Kwon et al., 2009). On the timescales of multiple centuries NPP acts to modulate atmospheric carbon dioxide levels via its control on the biological carbon pump intensity and ocean carbon inventories (Falkowski et al., 2003; Ito and Follows, 2005).
NPP is essential for marine biodiversity and associated ecosystem services. It is one of the main inputs of nutrients and energy that supports consumer growth and survival in marine food webs. Consumer diversity and their ecosystem functions then deliver important ecosystem services and societal benefits (Bindoff et al., 2019). These benefits include fisheries, climate regulation, supporting cultural, and intrinsic values. As an example, NPP is closely related to the distribution and magnitude of marine fisheries catches (Stock et al., 2017), which provide food, nutrition, income, and livelihoods for many millions of people globally (FAO, 2018). Changes in the transfer of NPP-derived particulate organic carbon from the ocean surface to the seafloor also affects the food supply to benthic ecosystems (Yool et al., 2017).
To achieve high rates of NPP, phytoplankton require light, and a range of resources for enzyme cofactors, as well as the structural components that permit high population levels (Falkowski et al., 1998; Saito et al., 2008). Alongside variable light conditions and the response of metabolic rates to temperature, deficiencies in the availability of these essential resources are the main “bottom up factors” limiting rates of NPP and driving its response to climate change (Laufkötter et al., 2015). Key resources to consider are nitrogen and iron, with phosphorus, silicon, and others playing roles in particular regions or for particular phytoplankton groups (Moore et al., 2013). Importantly, as the overall rates of NPP are affected by the combination of specific rates and population levels, they are also controlled by changes in predation by zooplankton (known as grazing) that affect phytoplankton standing stocks.
The Intergovernmental Panel on Climate Change (IPCC) made a range of assessments regarding NPP and associated ecosystem services in the recent Special Report on Oceans and Cryosphere in a Changing Climate (SROCC) report. In the SROCC, and other IPCC assessment reports, various different degrees of specific language are carefully used to assign confidence and likelihood to the assessments (Mastrandrea et al., 2011). Confidence is assigned based on the strength of the evidence and the level of agreement, with high confidence reserved for high agreement across robust evidence. Likelihood is used when enough confidence exists for there to be a probabilistic assessment, with likely reflecting 66–100% probability, very likely reflecting 90–100% probability and virtually certain related to 99–100% probability. When the results across multiple models are assessed, it is common to examine the likely or very likely range, either by using one or two standard deviations, respectively, or the appropriate confidence intervals (Collins et al., 2013; Bindoff et al., 2019).
NPP projections are available as part of the 5th and 6th Coupled Model Intercomparison Projects (CMIP5 and CMIP6, respectively). Due to the timing, SROCC relied on CMIP5 datasets and made the following assessments regarding the contemporary trends in NPP and future projections under the high emissions RCP8.5 scenario (Bindoff et al., 2019): (i) there was high confidence that the ongoing changes to stratification and nutrient cycles was having a regionally variable impact on NPP, (ii) there was low confidence assigned to the very likely range of NPP decline between 4 and 11% by 2081–2100, relative to 2006–2015, with medium confidence associated with projected declines of 7–16% (very likely range) in the tropical ocean that were constrained by historical variability. The projected declines in NPP were also assessed to lead to alterations of community structure (high confidence), reduce global marine animal biomass (medium confidence), and the maximum catch potential of fisheries (medium confidence), which may elevate the risk of impacts on income, livelihood, and food security of the dependent human communities (medium confidence). Taken together, these raise significant challenges for ocean ecosystem services in the face of ocean NPP changes.
Processes Governing Ocean Net Primary Production and Their Representation in Earth System Models
Generally, the types of ocean model used for climate change projections as part of CMIP6 and CMIP5 exercises follow a relatively similar approach to representing NPP despite showing a range of model configurations. They consider a discrete set of phytoplankton and zooplankton functional types (in rare cases also heterotrophic bacteria) and represent NPP as the product of the phytoplankton growth rate and biomass, with some inter-model differences in the representation of carbon loss due to respiration. In rare cases, gross primary production (GPP) is computed, with NPP the result of GPP minus autotrophic respiration and other loss terms (Vichi et al., 2007).
Phytoplankton growth rates are controlled by limitation by light and a range of nutrients using a Liebig “law of the minimum” approach. Across different models, these range from single nutrient limitation by N or P to accounting for multiple limiting factors (N, P, Si, and Fe). Fractional limitation of temperature dependent maximum growth rates is based on either external or cellular nutrient levels and when more than one nutrient is considered, the “most” limiting nutrient is used. Phytoplankton biomass is grazed by zooplankton, as a function of prey density following classical functional responses. The nutrient levels themselves are an emergent property of the ocean physical model and the balance between consumption during NPP and other processes, as well as the release and recycling by zooplankton, bacteria and degradation of sinking particulate organic matter, and sediment remineralisation in some models, which operate alongside external sources to the ocean (see Laufkötter et al., 2015; Séférian et al., 2020 for a more detailed description of NPP parameterizations in CMIP5 and CMIP6 Earth system models).
Earth system models show good skill in reproducing the distribution of major nutrients (nitrate, phosphate and silicate) (Séférian et al., 2020), with the notable exception of iron (Tagliabue et al., 2016), but less skill for indices of biological activity, like surface chlorophyll (Séférian et al., 2020). This issue is compounded for NPP, since the different types of observational algorithms and their various dependencies introduce additional uncertainty (beyond that for chlorophyll) in remote sensing estimates e.g., (Sathyendranath et al., 2020). Moreover, intercomparisons compare full Earth system models and thus the impact of differences in the specific NPP closures cannot be isolated from parallel differences in the accompanying ocean physical models and, more so, their atmospheric and land components. To date, the regional skill of Earth system models against estimates of NPP derived from remote sensing algorithms has not been conducted in depth, with global assessments available (Séférian et al., 2020).
Model projections of NPP at regional scales under different emissions scenarios are important because they inform community-level assessments, such as those by the IPCC, and form the basis of risk assessments for critical open ocean marine ecosystems and ecosystem services, such as fisheries (Bindoff et al., 2019). To date, uncertainty in NPP projections conducted as part of CMIP5 and CMIP6 at regional scale has been typically assessed by averaging changes across multiple years around the end of the century (2081–2100), relative to the recent historical era (1996–2015 for CMIP6) across models and considering whether more than 80% of the models agreed on the sign of change or with the statistical significance at regional scale (Bopp et al., 2013; Kwiatkowski et al., 2020). As a more quantitative assessment that is in line with IPCC assessments, the standard deviation around the multi-model mean can be used as the “likely” uncertainty range (Bindoff et al., 2013; Collins et al., 2013).
From the initial analysis of 13 CMIP6 Earth system models published by Kwiatkowski et al. (2020), global rates of NPP are projected to decline under the SSP5-8.5 high emissions scenario by a multi-model mean of 2.99% by the end of the 21st century, with a “likely” uncertainty range of ±9.1%. This spread has increased from the 10 models assessed for CMIP5 under the RCP8.5 scenario (Bopp et al., 2013), where there was a multi-model mean decline of 8.54%, with a “likely” uncertainty range around one-third lower at ±5.9%. The only regions where more than 80% of the CMIP6 models agreed on the sign of change under SSP5-8.5 were for the projected declines in the North Atlantic and increases in the Southern Ocean, with agreement on this metric more common for CMIP5 (Bopp et al., 2013). There is to date, no quantitative assessment of the regional uncertainty in absolute terms from the more recent CMIP6 models. Some efforts to assess regional change were performed as part of CMIP5 (Cabré et al., 2014; Laufkötter et al., 2015; Leung et al., 2015; Fu et al., 2016; Rickard et al., 2016; Kwiatkowski et al., 2017; Nakamura and Oka, 2019), highlighting the role of uncertainty and distinct regional drivers. Inter-model spread in the magnitude of NPP projections was noted in CMIP5 and was found to be localised in the tropics, but the regional expression and consequences for ecosystem services were not examined in great detail (Laufkötter et al., 2015).
As marine ecosystem projections are driven by changes in NPP or planktonic biomass, any inter-model differences and uncertainty in NPP projections then feeds into projected risk assessments under different climate change scenarios. For instance, alterations to the projected NPP response due to modified phytoplankton nutrient supply in the tropical Pacific has been shown to increase uncertainty in projected impacts of climate change on total consumer biomass (Tagliabue et al., 2020). In another study, accounting for greater complexity in phytoplankton physiology was found to enhance the amplified climate driven responses of higher trophic levels (Kwiatkowski et al., 2019a). Ultimately, ecosystem services depend on changes in absolute NPP rather than the percentage changes that are usually presented. To accommodate the divergent absolute mean state conditions of Earth system models, upper trophic level and fisheries projections are currently forced to rely on proportional changes that are appropriately benchmarked for a subset of Earth system models (e.g., Tittensor et al., 2018).
Variability in Global and Regional Net Primary Production Projections From CMIP5 and CMIP6 Models
In this section, we examine and review NPP changes from 16 CMIP6 models newly assembled for this study (only 13 were used in Kwiatkowski et al., 2020) and 10 CMIP5 models previously assessed (Bopp et al., 2013). This focus on absolute, as well as relative changes from CMIP6 and CMIP5 models at regional scale is important as they underpin the associated ecosystem services and our understanding of a key component of upper ocean carbon turnover. To facilitate this regional analysis, we re-gridded CMIP6 and CMIP5 models onto a uniform 1 × 1 degree horizontal grid as in Kwiatkowski et al. (2020) and used 11 broad biogeographic provinces based on Longhurst (2007) and summarised previously (Vichi et al., 2011) to represent 11 key oceanic regions (Table 1). We address the change in depth-integrated annual NPP averaged between 2081–2100 and 1995–2014 in three ways, the relative change (in percentage terms), the absolute change in mol C m−2 yr−1 and integrated over the province in Tmol C yr−1. A common reference period is applied to both CMIP6 and CMIP5 models. In all cases, we assessed uncertainty across models in two ways, via the one standard deviation “likely” range that links to IPCC assessments and the full inter-model range. We generally focus on using the one standard deviation “likely” range, but note the importance of the inter-model range in quantifying the total range of uncertainty across all models. In this way, we update the recent assessments based on CMIP5 and CMIP6 as part of the IPCC sixth assessment reports.
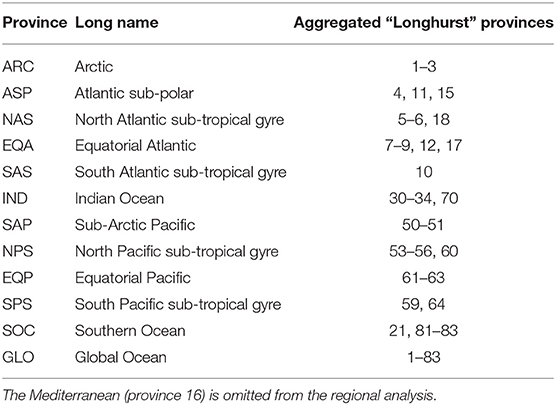
Table 1. Summary of the ocean provinces used in this study and the codes of the 83 Longhurst provinces (Longhurst, 2007) used by Vichi et al. (2011), with the numbers corresponding to their Table 1 (Vichi et al., 2011).
Before addressing the projected changes, we focus on the degree of inter-model difference in the levels of historical (1995–2014) NPP. For example, the multi-model mean global annual NPP is 41.4 Pg (or 3.45 Pmol), but the likely and inter-model ranges across the 16 CMIP6 models are 10 and 33 Pg, respectively. Notably, this inter-model range of 33 Pg C, is over an order of magnitude greater than the projected absolute change (see below). Some models show rates of global NPP as high as 56 Pg C in the historical era, while others are as low as 23 Pg C. These inter-model differences in historical levels of NPP are also present at a regional level, with areas of high absolute NPP in multi-model mean terms (Figure 1A), also showing an elevated likely uncertainty (Figure 1B) and inter-model range (Figure 1C). Notably, the magnitudes of both the likely and inter-model range-based uncertainties are of similar order to the multi-model mean NPP, indicating a high level of inter-model difference (note similar scale range for Figure 1 panels). In general, the degree of inter-model uncertainty in historical levels of NPP has declined between CMIP5 and CMIP6, with the largest declines in uncertainty across models in regions such as the tropical Atlantic, Pacific and Indian Oceans (Figure 2A). Despite their reduction since CMIP5, these inter-model differences in ocean NPP remain substantial and reflect major mean state biases across models, as well as indicating a large knowledge gap in the magnitude of this key planetary flux.
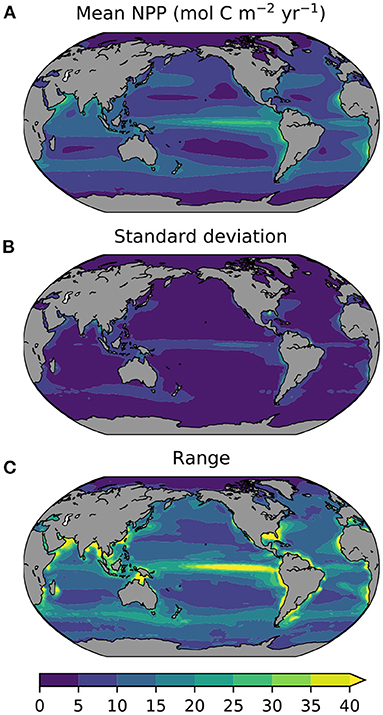
Figure 1. Absolute annual NPP from 16 CMIP6 models for the historical period (1995–2014) presented as (A) multi-model mean, (B) 1σ, and (C) the full inter-model range. All plots show annual NPP in mol C m−2 yr−1.
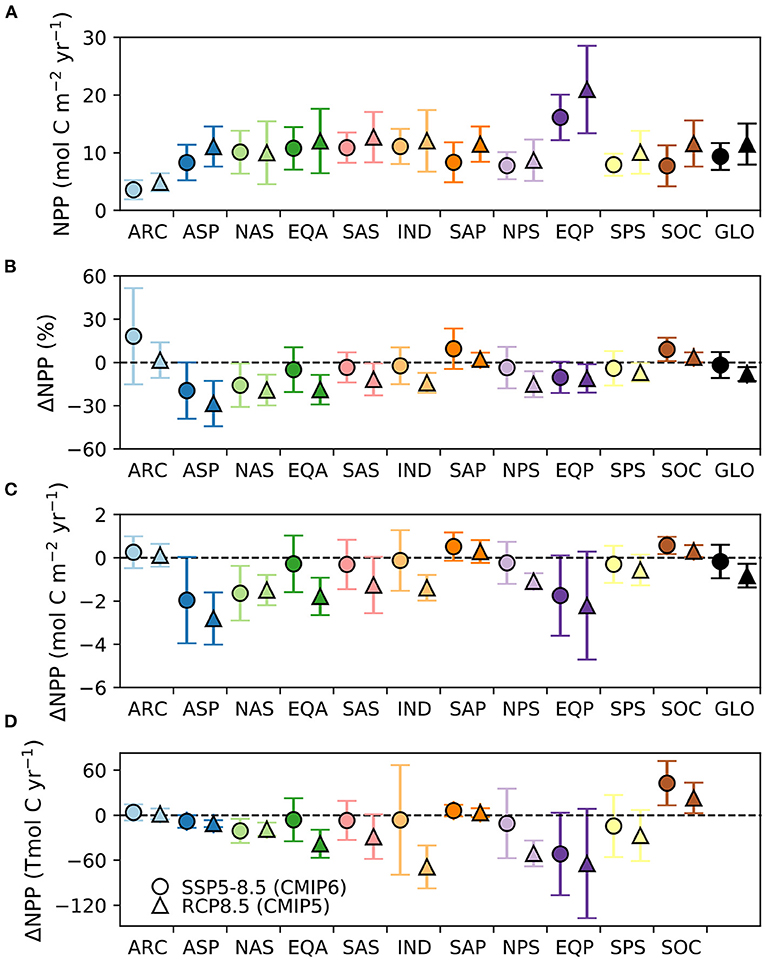
Figure 2. Multi model mean and 1σ uncertainty for 16 CMIP6 and 10 CMIP5 models on a province by province basis for (A) NPP levels during the historical era (1995–2014), and projected changes in annual NPP on a (B) percent, (C) mol C m−2 and (D) Tmol C basis (all relative to 1995–2014). CMIP6 results are from the 16 models using the SSP5-8.5 scenario, while CMIP5 results are from the 10 models using the RCP8.5 scenario. The global results are not included on panel d as they distort the scale.
Projected Changes in NPP at Global and Regional Scales
Relative Changes
In our analysis extended to 16 CMIP6 models, global NPP is projected to decline by 1.76% under SSP5-85, with a likely uncertainty range of ±8.06% or a total inter-model range of 33.96% across all models (relative to 1995–2014). The multi-model change for CMIP6 is slightly less than the analysis of Kwiatkowski et al. (2020), due to the inclusion of three additional Earth system models. The inter-model range of >30% around this small average change highlights the wide array of responses in projections at the global scale and the lack of confidence in even the sign of change for CMIP6. Globally, the multi-model mean change in NPP as declined from around −8.06% for CMIP5 to −1.76% for CMIP6, due to the much larger inter-model spread (Table 2). The slight change in the global percentage change for CMIP5, compared to Bopp et al. (2013), arises due to our use of a later reference period for CMIP5 of 1995–2014.
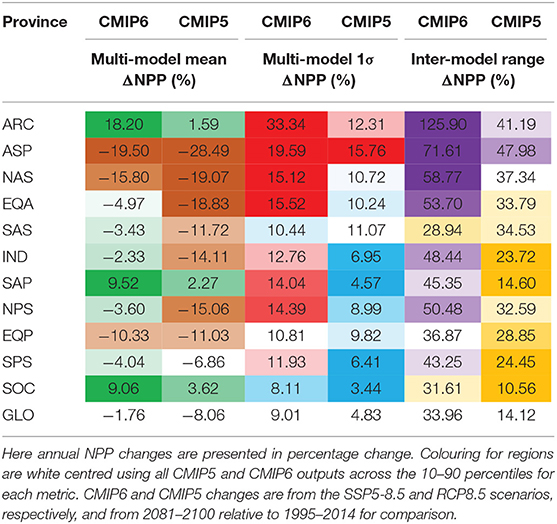
Table 2. Statistics (as percentage changes) for the multi-model mean, 1σ “likely” range and the full inter-model range across different regions and globally for 16 CMIP6 and 10 CMIP5 models.
Except for a few regions, the uncertainty in percentage changes in NPP, measured either by the likely or inter-model range, is broadly similar across regions (Figure 3, Table 2). Exceptions to this are the Arctic, which shows the highest likely and inter-model range, and the North Atlantic sub-polar gyre (Figure 3, Table 2). The Southern Ocean and, to a lesser extent, the South Atlantic sub-tropical Gyre, display uncertainties (both in terms of likely and inter-model ranges) that are slightly smaller than the global average. Compared to CMIP5, uncertainties in the projected percentage changes in NPP at regional scale have increased by up to 3-fold under CMIP6, most notably in the sub-Arctic Pacific, Arctic and Southern Ocean, with the exception of the South Atlantic Sub-Tropical Gyre where uncertainty has declined since CMIP5 (Table 2, Figure 2B).
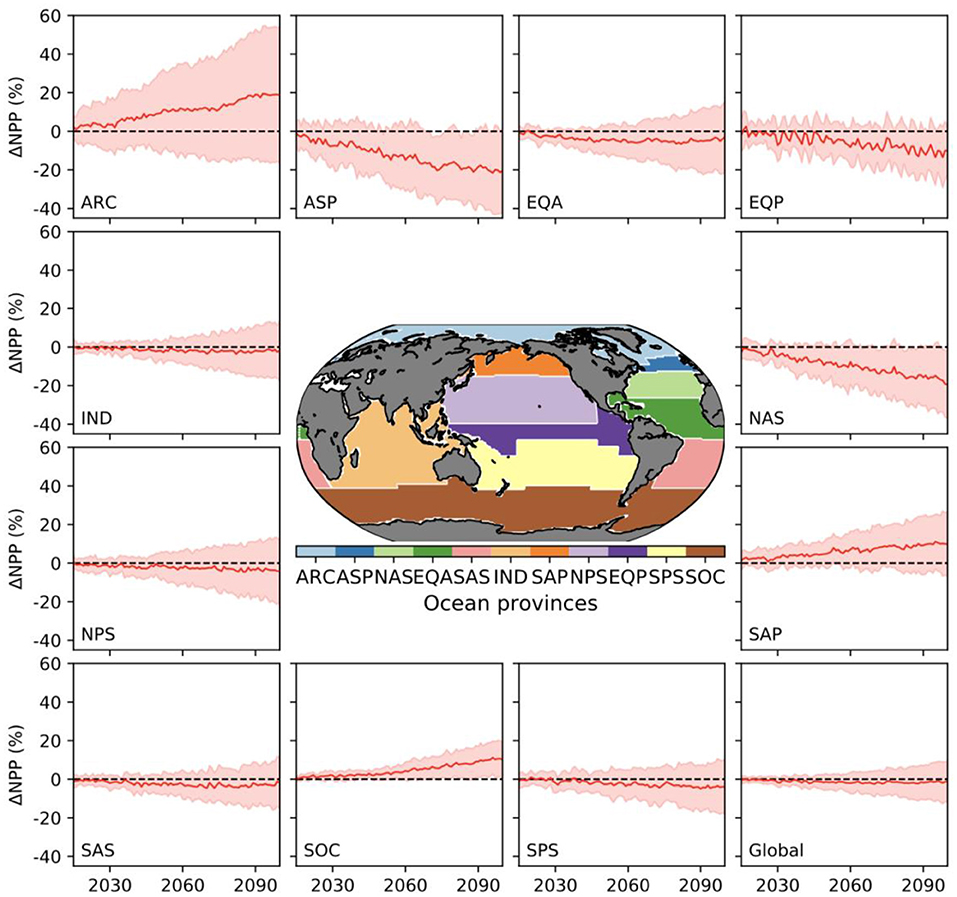
Figure 3. Percentage change in annual NPP (relative to 1995–2014) across different biogeochemical provinces and globally from 16 CMIP6 models. The red line represents the multi-model mean and the shaded area the 1σ “likely” uncertainty range. The map shows the location of the provinces.
Absolute Changes
The projected changes in absolute NPP under the high emissions SSP5-8.5 scenario also show inter-model differences that are significant. At the global scale, the projected annual NPP changes are −0.17 mol C m−2 (or −63.34 Tmol C) and are associated with likely and inter-model ranges of 0.77 and 2.72 mol C m−2 (or 286.40 and 1010.00 Tmol C), respectively (Tables 3, 4). Similar to percentage changes, we find uncertainties in the global projections that are very large, relative to the multi-model mean change. Importantly, the degree of uncertainty in projections of global NPP in absolute terms has grown from CMIP5 to CMIP6 by around 1.5-fold (Tables 3, 4). However, regionally, even stronger levels of uncertainty in absolute NPP changes emerge from the CMIP6 models (Figure 4). Regions that hosted high NPP in the historical period (Figure 1A) show the largest likely uncertainty and inter-model range for their projected NPP changes in absolute terms (Figures 4B,C). The inter-model range in the projected NPP change is amplified 5-fold, relative to the multi-model mean change (note the 5-fold increase in scale for Figure 4C, as compared to Figure 4A), while the inter-model range was of similar order to the multi-model mean in the historical era (similar range for Figures 1A,C).
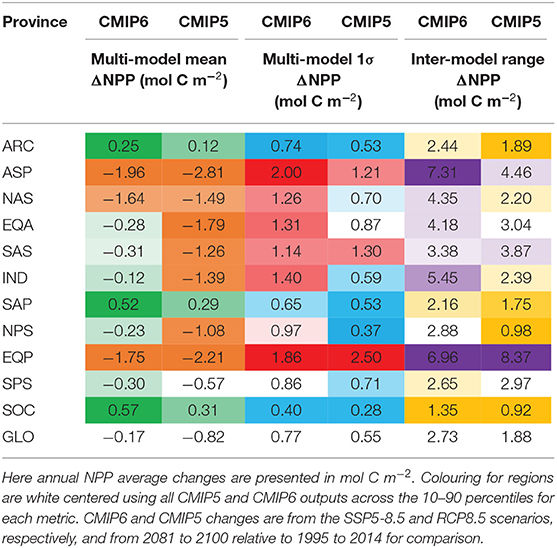
Table 3. Statistics (as mol C m−2 changes) for the multi-model mean, 1σ “likely” range and the full inter-model range across different regions and globally for 16 CMIP6 and 10 CMIP5 models.
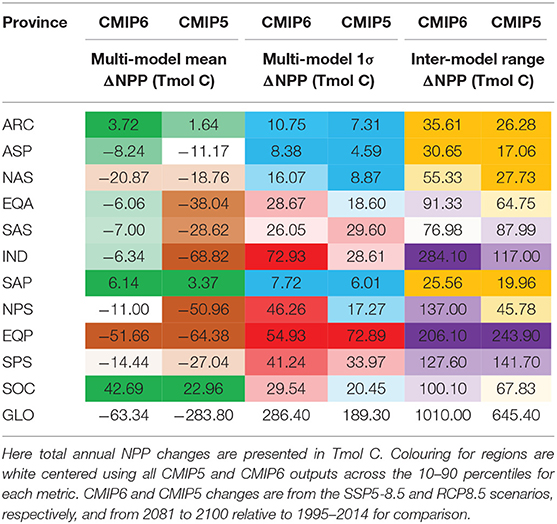
Table 4. Statistics (as Tmol C changes) for the multi-model mean, 1σ ‘likely' range and the full inter-model range across different regions and globally for 16 CMIP6 and 10 CMIP5 models.
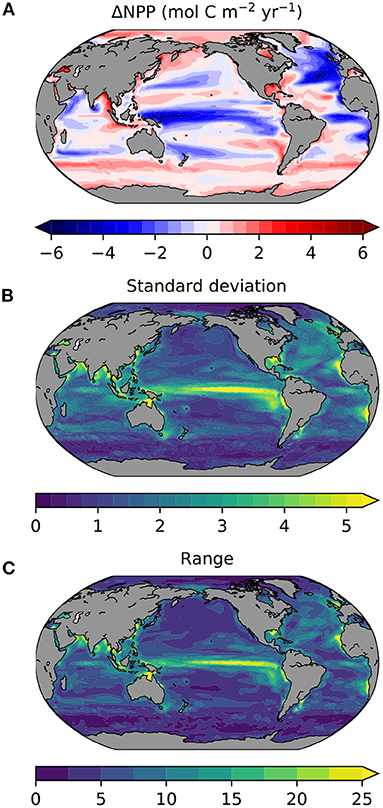
Figure 4. The SSP5-8.5 change in NPP from 16 CMIP6 models by 2081-2100 (relative to 1995–2014) for (A) multi-model mean, (B) 1σ, and (C) the full inter-model range. All plots show annual NPP in mol C m−2 yr−1. Note scale change for (C).
Turning to the absolute changes at the regional scale in more detail, we see that some key regions emerge as contributing substantially to the uncertainty in projected absolute changes on a per square metre basis (Figure 5). Relative to the global uncertainty, the North Atlantic sub-polar Gyre, Equatorial Pacific and the tropical Indian Ocean display the largest uncertainty in the mol C m−2 changes in annual NPP for both the likely and inter-model range (Table 3, Figure 2C). In a regionally integrated sense, the area of the different regions is important and the Indian Ocean, Equatorial Pacific, northern and southern sub-tropical Pacific regions emerge as hotspots of uncertainty (Table 4, Figure 2D). Indeed, around two-thirds (67%) of the overall inter-model range in the total NPP change across models in a total integrated sense can be explained simply by the summed contributions from the tropical Pacific and Indian Oceans (Table 4). Uncertainties, in terms of both the likely and inter-model range, at the regional scale have also expanded markedly since CMIP5, especially for the Indian Ocean and north Pacific sub-tropical gyre where uncertainty has increased around 2–3-fold (Tables 3, 4, Figures 2C,D).
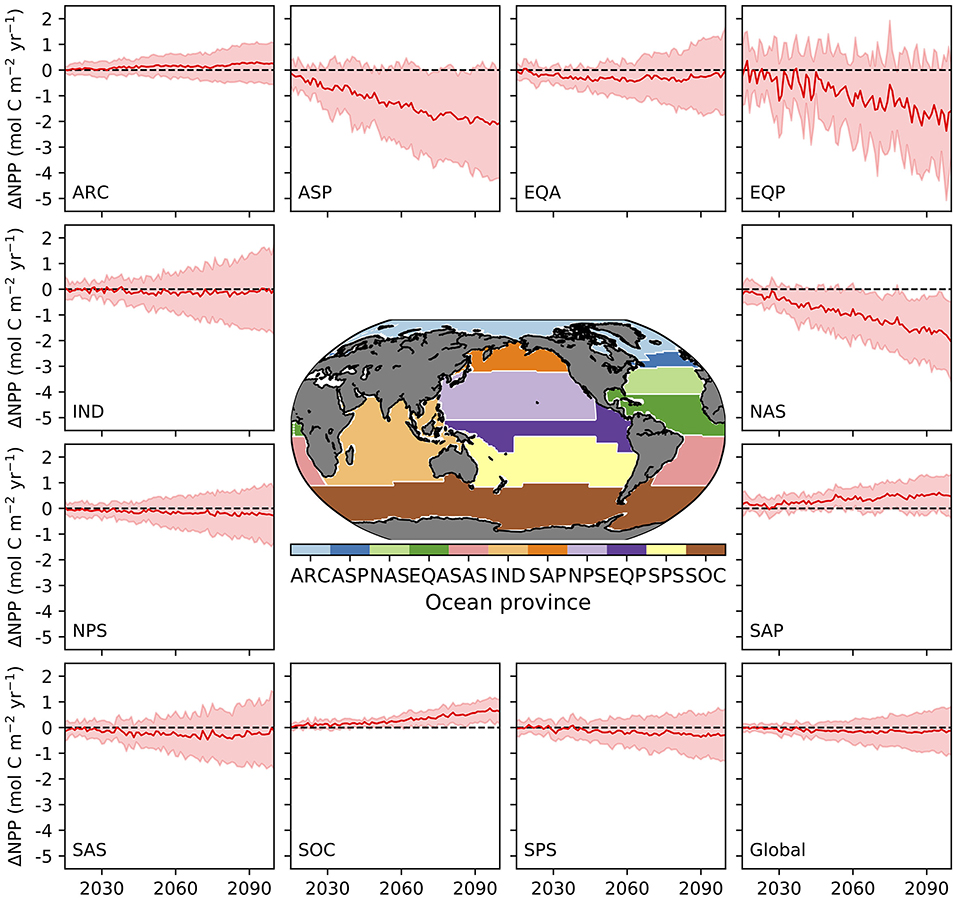
Figure 5. Absolute change in annual NPP (relative to 1995–2014) in mol C m−2 across different biogeochemical provinces and globally from 16 CMIP6 models. The red line represents the multi-model mean and the shaded area the 1σ “likely” uncertainty range. The map shows the location of the provinces.
Overall, this indicates that a large proportion of the inter-model uncertainty around global NPP projections in absolute terms resides in the response of the Tropical Pacific, Indian and to a lesser extent the North Atlantic Oceans. Some regions that have strong uncertainties in relative changes, like the Arctic (Figure 3), are less significant in absolute changes. For the Tropical Pacific and Indian Oceans, the uncertainty in the projections of absolute NPP change concerns the sign of the change, while for the North Atlantic Ocean it relates to the magnitude of the projected decline, which is common across models (Figure 5). Lastly, the increase in uncertainty regarding projected changes since CMIP5, despite less uncertainty in the mean state, is notable (Figure 2) and will lower confidence in NPP projections made in SROCC due to the reduced inter-model agreement.
It is particularly notable that despite higher equilibrium climate sensitivity (Zelinka et al., 2020), greater surface warming and enhanced upper ocean nutrient decline in CMIP6 than CMIP5, global NPP decline over the 21st century is less, not more, extensive (Kwiatkowski et al., 2020). The lower magnitude of global NPP decline in CMIP6 is not attributable to an individual region, but rather a result of enhanced NPP increases in the Southern and Arctic Oceans and less extensive declines in multiple other regions, notably the Indian Ocean, the equatorial Atlantic and Pacific, and the sub-tropical gyres in the Pacific and south Atlantic (Figure 2). This suggests that the temporal evolution of phytoplankton resource limitation and or grazing pressure due to climate-driven changes in atmosphere/ocean physics and/or ocean biogeochemical cycling may have significantly altered between CMIP5 and CMIP6 in these regions.
Key Mechanisms Underpinning Uncertainty
The dominant conceptual view of how climate change affects NPP in the predominantly nutrient limited low to mid latitude regions is via changes to ocean stratification, which reduce nutrient supplies to the surface ocean (Behrenfeld et al., 2006; Doney, 2006). In contrast, light limited regions at high latitudes benefit from extended growing seasons due to sea ice removal and the maintenance of ocean mixing within the euphotic layer when waters are more stratified. However, this emphasis on stratification is incomplete (e.g., Whitt and Jansen, 2020) and clearly does not account for the variety of physical and biogeochemical mechanisms that produce the inter-model uncertainty in NPP projections for both CMIP5 and CMIP6. In our analysis on the projected changes in NPP in absolute terms, three key regions of the ocean emerged as hotspots of inter-model disagreement: (i) Indian Ocean, (ii) Tropical Pacific (encompassing the equatorial and northern and southern Pacific sub-tropical gyres), and the (iii) North Atlantic (including the sub-polar gyre). Ultimately, the source of inter-model uncertainty in all three regions is 2-fold and linked to the response of (i) atmospheric and ocean circulation changes, and (ii) ocean biogeochemical cycling, including nutrient supply, resource limitation and grazing.
Uncertainties in Atmospheric and Oceanic Circulation Changes
Changes in the tropical Indo-Pacific atmospheric circulation known as the Walker Cell are a good example of how other factors, such as changing wind patterns, interact with warming to drive NPP change. With easterlies over the equatorial Pacific and Westerlies over the equatorial Indian Ocean, the Indo-Pacific Walker Cell sustains NPP in the eastern Pacific and western Indian Ocean by shallowing the nutricline and making nutrients more available. Any Walker Cell intensification would thus tend to oppose the effect of enhanced water column stability linked to warming, while a Walker Cell slowdown would amplify the NPP decrease in the Pacific by reducing nutrient availability (Matear et al., 2015). Currently, the evolution of the Walker Cell in Earth system models is uncertain, with most projecting a weakening (Cai et al., 2020), but some suggesting an intensification (Plesca et al., 2018). Although observations suggest the Walker Cell has intensified over recent decades, there is a debate regarding whether it can be attributed to a longer term trend that is not consistently reproduced by Earth system models or to internal atmospheric variability (Kociuba and Power, 2015). An improved understanding of how changes in winds, that are poorly constrained, interact with ongoing stratification changes, that are better understood, in driving the overall NPP response in the Indo-Pacific is required.
The western Arabian sea is one of the most productive regions in the entire tropics (Naqvi et al., 2010; Moffett and Landry, 2020) and although changes in stratification contribute to the NPP changes (Roxy et al., 2016), alterations to winds may also be important. Notably, high rates of NPP in the Arabian Sea during summer are supported by the Somalia and Oman upwellings that are driven by alongshore monsoon winds and offshore Ekman pumping, with mesoscale processes also playing an important role in its horizontal extent (e.g., Marra and Barber, 2005). In contrast, during winter, Arabian Sea NPP is regulated by convective mixing in response to winter monsoon evaporative cooling (e.g., Keerthi et al., 2017). Most Earth system models project a decrease in the monsoon winds over the Arabian Sea during both winter (Parvathi et al., 2017) and summer (Sooraj et al., 2014), as well as anomalous easterlies at the equator in response to the Indo-Pacific Walker circulation weakening. These changes will contribute to an NPP decline during both seasons, but are highly variable across models (as discussed above). Ultimately, significant biases and uncertainties in the representation of the Asian monsoon and its oceanic response in Earth system models hamper the reliability of NPP projections in the Indian Ocean (Singh et al., 2019). As fine-scale processes, such as mesoscale eddies, are very important in this region, improvements to model resolution during CMIP6 (Eyring et al., 2016) have the potential to improve the reliability of future NPP projections.
Changes in coastal upwelling in the Eastern Boundary Upwelling Systems (EBUS) due to shifts in wind patterns will also be important in shaping the response of NPP and the coupling to ecosystem services. Early studies suggested strengthening winds due to climate change would lead to consistent increases in upwelling in EBUS, with implications for the response of NPP (Bakun, 1990). Current Earth system models, and some observations, tend to project reduced winds and upwelling intensity for low latitudes and enhancements at higher latitudes under a high emissions scenario during CMIP5 (García-Reyes et al., 2015; Rykaczewski et al., 2015). However, the interaction of changing winds with coastal warming can produce complex responses in upwelling at local scales, with an important role for mesoscale dynamics not resolved in Earth system models (García-Reyes et al., 2015; Wang et al., 2015; Renault et al., 2016; Xiu et al., 2018).
Interannual changes in NPP in the North Atlantic have been shown to be poorly correlated with parallel modifications to stratification (Lozier et al., 2011; Dave and Lozier, 2013), highlighting the role of other physical mechanisms. The Gulf Stream plays a critical role in nutrient supply in the North Atlantic via the nutrient stream (Pelegrí et al., 1996). This means that projected changes to nutrient concentrations in the upper ocean from Earth system models result from the combination of the nutrient stream and its sensitivity to the Atlantic meridional overturning circulation (AMOC), which interact with the effect of changes to ocean stratification (Tagklis et al., 2020; Whitt and Jansen, 2020; Couespel et al., 2021). Differences in the mean state representation of the nutrient stream and the extent of AMOC slowdown across CMIP5 (Cheng et al., 2013) and CMIP6 (Weijer et al., 2020) are therefore major contributors to the magnitude and uncertainty of projected NPP declines in the region (Tagklis et al., 2020; Whitt and Jansen, 2020).
Uncertainties in the Representation of Biogeochemical Processes
In the tropical Pacific, in particular, part of the inter-model uncertainty is driven by the relative role played by iron and nitrogen limitation among models. A set of mechanistic experiments within a single model demonstrated that minor parameter adjustments that altered the strength of iron limitation and its replacement by nitrogen limitation in the tropical Pacific led to large changes in both the magnitude and sign of changes to regional NPP (Tagliabue et al., 2020). It is highly likely that there is divergence in the representation of nutrient limitation regimes across CMIP5 and CMIP6 models, in part due to the paucity of observational constraints (Moore et al., 2013; Ustick et al., 2021). Indeed, it was notable that the emergent constraint that assessed NPP projections from CMIP5 using the co-variation of sea surface temperature and NPP anomalies (Kwiatkowski et al., 2017), was not able to strongly discriminate among different iron-nitrogen limitation scenarios (Tagliabue et al., 2020). New understanding to inform on Earth system model parameterisations and performance are clearly needed for this key aspect of model structural uncertainty.
Poor knowledge of nutrient limitation regimes is not restricted to the tropical Pacific, but also the Indian Ocean, with local physical processes also being important. Our knowledge of Indian Ocean biogeochemical cycling and nutrient limitation regimes remains limited but has been growing in recent years (Grand et al., 2015; Chinni et al., 2019; Twining et al., 2019). For instance, iron limitation and zooplankton grazing have been suggested to modulate NPP in the western Arabian Sea (Moffett et al., 2015; Moffett and Landry, 2020), which highlights the need for a deeper understanding of how grazing processes and iron limitation interact in this highly productive region. Additionally, if iron limitation is important, then climate-driven changes in dust fluxes will also be a key component of projected changes in Earth system models. Of course, these biogeochemical changes also interact with the atmospheric and ocean physics changes mentioned in section Uncertainties in Atmospheric and Oceanic Circulation Changes to control the fate of NPP. As suggested by Moffett and Landry (2020), any strengthening of the monsoonal circulation should exacerbate iron limitation, leading to an eastward shift in the utilization of upwelled nutrients, while a weakening of the monsoonal circulation, as projected by most climate models (Singh et al., 2019), will probably reduce the importance of iron limitation. It is likely that there is ultimately a strong mosaic of nutrient limitation regimes in the region, linked to both the underlying physical finescales, but also the role of dust, river and margin supplies that needs to be assessed further in Earth system models.
Relevant across all the tropical regions showing high uncertainty is the contribution played by nitrogen fixation by diazotrophs in modulating the NPP response to climate change. As a new source of nitrogen to the upper ocean, nitrogen fixation may counterbalance changes in nutrient supply due to changing ocean stratification, but only if sufficient iron and phosphorus is present (Zehr and Capone, 2020). Projected changes to nitrogen fixation are highly variable among models at regional scale and because modifications to nitrogen fixation due to climate change emerge rapidly, they can contribute to the changes in NPP in nitrogen limited regions that establish themselves outside of internal variability later in the century (Wrightson and Tagliabue, 2020). CMIP5 and CMIP6 models take different approaches to representing diazotrophy, which introduces an additional source of uncertainty in the evolution of the upper ocean fixed nitrogen supply.
In the Arctic, NPP projections are particularly uncertain in areas of present-day sea ice, where initial nitrate concentrations and the rate of sea ice retreat determine how reduced light limitation and enhanced nutrient limitation interact to determine the future NPP response in a given model (Popova et al., 2012; Vancoppenolle et al., 2013). In the Arctic also, a recent study has also shown that nutrient input from the land, through riverine fluxes and coastal erosion, may sustain around one third of contemporary Arctic Ocean NPP (Terhaar et al., 2021). This suggests that changes in nutrient input may be a key factor affecting future Arctic NPP, in accord with recent satellite derived trends (Lewis et al., 2020).
More broadly, additional sensitivities around the linkage between phytoplankton and zooplankton, as well as upper ocean nutrient cycling will contribute to inter-model uncertainties. The ability of Earth system models to resolve variations in the nutrient contents of phytoplankton plays a role in their responses to changing nutrient supply (Kwiatkowski et al., 2018), with implications for zooplankton grazers (Kwiatkowski et al., 2019a). Differences in the changes in grazing rates across Earth system models contributes additional uncertainty to NPP projections, due to their impact on overall phytoplankton biomass (Laufkötter et al., 2015). Moreover, adjustments to zooplankton grazing efficiencies due to climate change induced changes in food quality can lead to alterations to and feedbacks on upper ocean recycling, especially for essential micronutrients (Richon et al., 2020; Richon and Tagliabue, 2021). Whether or not temperature sensitivities are applied to nutrient recycling pathways in Earth system models may also affect the temporal evolution of NPP (Taucher and Oschlies, 2011). Finally, explicit representation of the “end to end” coupling between phytoplankton and upper trophic levels is not yet widespread, but may also be important in shaping the response of NPP (Lefort et al., 2015; Aumont et al., 2018).
Implications for Ocean Biodiversity and Ecosystem Services
The large uncertainties regarding the projected changes in NPP in extent and sign, as well as their increase from CMIP5 we report here, have important implications for fisheries management. This is particularly the case because uncertainties increase at regional scales and are accentuated in regions where fisheries contribute substantially to food provision (e.g., the Tropical Pacific and Indian Ocean) (Golden et al., 2016; Bindoff et al., 2019). Temperature has been found to be a principal driver of changes in the biogeography of marine fish and invertebrates, while NPP interacts with temperature to affect the productivity of the populations (Brander, 2007; Blanchard et al., 2012; Cheung et al., 2019). Thus, production of marine species that expand poleward in response to warming may be limited by the decrease in NPP in their future range, resulting in a decline in total fish production as the low latitude edge of the distribution range retract poleward (Jones and Cheung, 2015; Villarino et al., 2015; Cheung et al., 2019). In contrast, increase in NPP may increase the rate of range expansion. Simultaneously, changes in NPP, as the basic energy production, will also result in cascading effects through the food chains from zooplankton to fish and top predators such as seabirds and marine mammals (Bindoff et al., 2019; Tagliabue et al., 2020; du Pontavice et al., 2021). In relation to this, an added dimension of uncertainties in NPP projections that are important for understanding the effects on marine foodwebs is the nutritional quality associated with NPP (e.g., size, structure and nutrient content). These changes will impact the availability of seafood from fisheries, both globally and regionally. Because the uncertainty range of NPP is high, and has increased since the CMIP5 results available for the SROCC, there are rising challenges for the long-term planning of fisheries development. These include the need for strategies that are more adaptive to an increasingly uncertain future. Similar considerations would also hold for other ecosystem services, based on the fact that coastal and shelf seas are providing up to 50% of global ocean primary production, thereby supporting a variety of rich habitats, such as wetlands, mangroves, kelp forests, and seagrass meadows that support biodiversity and provide food, coastal protection, carbon sequestration, and other services (Bindoff et al., 2019).
In terms of the mitigation of climate change, the uncertainties in the reduction of the biological carbon pump translate to differences spanning orders of magnitude in abatement and social costs, particularly given the increased uncertainty with respect to previous estimates from CMIP5 and related cost estimates (Barange et al., 2017). For instance, in the Barange et al. (2017) study, the projected decrease in carbon sequestration in the North Atlantic of 27–41% was estimated to represent a loss of 170–3,000 billion USD in abatement (mitigation) costs and 23–401 billion USD in social costs. While NPP is a key first step, an array of additional processes lead to the eventual change in the biological carbon pump. For instance, parallel changes in the plankton community composition, sinking rates and the remineralisation of particles will decouple changes in the biological carbon pump from NPP (De La Rocha and Passow, 2014; Sanders et al., 2014). Nevertheless, due to their connectivity, increased uncertainty in NPP will certainly not increase confidence in projections of the biological carbon pump. However, it is possible that additional constraints, e.g., from ocean interior nutrient and oxygen levels, can better constrain the biological carbon pump and thus bolster confidence in assessments.
Future Efforts to Reduce Uncertainty in NPP Projections
A major question is whether the community will be able to constrain ocean NPP better in the future, so model skill and inter-model agreement for NPP looks more like it does for nitrate and phosphate. The major issue for NPP is the high degree of variability across different spatial and temporal scales (compared to whole ocean nutrient reservoirs) and large divergence in contemporary direct constraints, which rely on different remote sensing algorithms applied across different satellite sensors (Sathyendranath et al., 2020). Unlike the biological carbon pump, there is only limited ability to constrain the magnitude and distribution of NPP indirectly from nutrient and oxygen distributions. This suggests that new approaches, such as using measurements like carbon isotopes or genomics, allied to improved observational coverage from bioArgo, are needed to provide the improved picture of both the magnitude and variability of upper ocean NPP that is required to drive improved models.
Associated with the challenge of constraining the magnitude of present-day NPP is uncertain knowledge of the drivers of change and how inter-model differences in atmospheric and ocean physics cascade through ocean biogeochemical cycles to drive resource limitation of NPP. Knowledge is growing regarding the role of multiple concurrent factors regulating microbial activity (Saito et al., 2014; Browning et al., 2017; Caputi et al., 2019; Ustick et al., 2021). But how the emergence of these multiple drivers are affected by the climate responses of key physical components, like winds, in the Indo-Pacific region in particular, is a major knowledge gap. Future efforts that conduct additional model experiments aimed at addressing the role of key mechanisms (e.g., Tagliabue et al., 2020), rather than the ensemble of differences (across land, atmosphere, and ocean components) between different Earth system models, are urgently needed to advance this area.
Largely absent from discussions around changing NPP in response to changing nutrient supply is the role of modification to external nutrient inputs to the ocean. While there have been some efforts including dynamic coupling with aerosol nutrient supply at the sea surface (e.g., Yool et al., 2021), future work that accounts for additional external inputs may introduce additional uncertainty in NPP changes. For instance, the inclusion of dynamic Greenland and Antarctic ice sheets in Earth system models is rapidly becoming computationally permissible and the associated changes to freshwater fluxes due to climate change have the potential to influence projections of NPP via both physical and biogeochemical mechanisms (Hopwood et al., 2020). These include changes to stratification and associated consequences for nutrient supply (Vizcaíno et al., 2008), but also direct supply of key nutrients, such as iron (Laufkötter et al., w2018; Person et al., 2019) and low latitude impacts via atmospheric teleconnections (Kwiatkowski et al., 2019b). Earth system models are also moving toward dynamic representations of land-ocean riverine nutrient fluxes (Séférian et al., w2020), which have a strong sensitivity to anthropogenic activity and management choices (Seitzinger et al., 2010). The choice of which riverine nutrients to resolve and how these fluxes are coupled to land surface models has the potential to substantially alter projections of NPP in coastal regions (e.g., Terhaar et al., 2019).
At present, each CMIP Earth system model typically differs from its parent generation in terms of both physical and biogeochemical ocean models, not to mention differences in other components of the coupled Earth system (Bopp et al., 2013; Kwiatkowski et al., 2020; Séférian et al., 2020). This presents an inherent traceability issue when trying to identify what determines differences in NPP projections both within and across CMIP generations. One approach that has been previously adopted is to couple a suite of ocean biogeochemical models with the same physical ocean model (Friedrichs et al., 2007; Kriest et al., 2010; Popova et al., 2012; Kwiatkowski et al., 2014) in order to isolate the role of biogeochemistry. Although often practically difficult, this can be a valuable exercise, identifying the limitations of specific model parameterisations. Another area where improvements could be made is in the documentation of Earth system models, specifically, with respect to model spin-up procedures (e.g., Séférian et al., 2016) and validation. Currently each ocean biogeochemical modelling group makes independent and undocumented validation decisions. Knowledge of what observational constraints have been used to constrain ocean biogeochemical models would critically improve our understanding of the uncertainty in NPP projections. In this respect, much could be learned from the terrestrial biogeochemistry community (e.g., Spafford and MacDougall, 2021).
Concluding Remarks
Overall, the increase in model uncertainty between CMIP5 and CMIP6 for projections of NPP dramatically exceeds that of other ecosystem drivers such as sea surface temperature (Kwiatkowski et al., 2020). However, one might also consider whether the analysis we have made here constitutes a realistic assessment as to the magnitude of the uncertainty in the projected changes to ocean NPP. Despite progress in recent years, the ocean biology components of Earth system models remain very simple, relative to the complex picture of the structure and function of the ocean microbiome that is emerging from observations (Sunagawa et al., 2015). Future work that explores the consequences of new processes in Earth system models, including (but not limited to) microbial co-limitation, acclimation or adaptation, mixotrophy, and predator-prey dynamics will raise challenges for constraining model uncertainty (especially at regional scales) and model democratisation (Sanderson et al., 2015). This will be especially true for efforts using ocean biology changes from Earth system models to project upper trophic levels and provide assessments of fish stock changes. More broadly speaking, making the link between upper trophic levels and NPP in the context of a changing climate would benefit from moving beyond the single forcing of anthropogenic climate change toward a holistic framework of impacts on ocean ecosystems, ranging from pollutants (e.g., mercury, persistent organic pollutants, plastics) to disease (e.g., Vibrio) to overfishing.
Data Availability Statement
Publicly available datasets were analyzed in this study. This data can be found at: https://esgf.llnl.gov/.
Author Contributions
The study was designed and led by AT. Analysis of CMIP5 and CMIP6 models was performed by AT and LK. AT led the writing of the manuscript with contributions from LK, LB, MB, WC, ML, and JV. All authors contributed to the article and approved the submitted version.
Funding
AT received funding from the European Research Council (ERC) under the European Union's Horizon 2020 research and innovation programme (grant agreement no. 724289). LK received funding from the Agence Nationale de la Recherché (grant no. ANR-18-ERC2-0001-01) and the EU Horizon 2020 4C project (grant no. 821003). MB received funding from the EU H2020 project FutureMARES, grant agreement No 869300. JV acknowledges financial support from Institut de Recherche pour le Développement (IRD) for research related to the theme of this article. LB received funding from EU Horizon 2020 COMFORT (grant no. 820989) and TRIATLAS (grant no. 817578) projects.
Conflict of Interest
The authors declare that the research was conducted in the absence of any commercial or financial relationships that could be construed as a potential conflict of interest.
Publisher's Note
All claims expressed in this article are solely those of the authors and do not necessarily represent those of their affiliated organizations, or those of the publisher, the editors and the reviewers. Any product that may be evaluated in this article, or claim that may be made by its manufacturer, is not guaranteed or endorsed by the publisher.
Acknowledgments
We thank two reviewers for their comments that improved the manuscript. We acknowledge the World Climate Research Programme's Working Group on Coupled Modelling, which is responsible for CMIP.
References
Aumont, O., Maury, O., Lefort, S., and Bopp, L. (2018). Evaluating the potential impacts of the diurnal vertical migration by marine organisms on marine biogeochemistry. Glob. Biogeochem. Cycles 32, 1622–1643. doi: 10.1029/2018GB005886
Bakun, A. (1990). Global climate change and intensification of coastal ocean upwelling. Science 247, 198–201. doi: 10.1126/science.247.4939.198
Barange, M., Butenschön, M., Yool, A., Beaumont, N., Fernandes, J. A., Martin, A. P., et al. (2017). The cost of reducing the North Atlantic ocean biological carbon pump. Front. Marine Sci. 3:290. doi: 10.3389/fmars.2016.00290
Behrenfeld, M. J., O'Malley, R. T., Siegel, D. A., McClain, C. R., Sarmiento, J. L., Feldman, G. C., et al. (2006). Climate-driven trends in contemporary ocean productivity. Nature 444, 752–755. doi: 10.1038/nature05317
Bindoff, N. L., Cheung, W. W., Kairo, J. G., Arístegui, J., Guinder, V. A., Hallberg, R., et al. (2019). “Changing ocean, marine ecosystems, dependent communities,” in IPCC Special Report on the Ocean and Cryosphere in a Changing Climate, eds D. C. R. Pörtner, H-O. Masson-Delmotte, V. Zhai, P. Tignor, M. Poloczanska, and E. Mintenbeck (Cambridge, UK: Cambridge University Press), 447–587.
Bindoff, N. L., Stott, P. A., AchutaRao, K. M., Allen, M. R., Gillett, N., Gutzler, D., et al. (2013). Detection and attribution of climate change: from global to regional,” in Climate Change 2013: The Physical Science Basis. Contribution of Working Group I to the Fifth Assessment Report of the Intergovernmental Panel on Climate Change, eds T. F. Stocker, D. Qin, G. K. Plattner, M. Tignor, S. K. Allen, J. Boschung, et al. (Cambridge, New York, NY: Cambridge University Press), 867–952.
Blanchard, J. L., Jennings, S., Holmes, R., Harle, J., Merino, G., Allen, J. I., et al. (2012). Potential consequences of climate change for primary production and fish production in large marine ecosystems. Philos. Trans. R. Soc. Lond,. B,. Biol. Sci. 367, 2979–2989. doi: 10.1098/rstb.2012.0231
Bopp, L., Resplandy, L., Orr, J. C., Doney, S. C., Dunne, J. P., Gehlen, M., et al. (2013). Multiple stressors of ocean ecosystems in the 21st century: projections with CMIP5 models. Biogeosciences 10, 6225–6245. doi: 10.5194/bg-10-6225-2013
Boyce, D. G., Lotze, H. K., Tittensor, D. P., Carozza, D. A., and Worm, B. (2020). Future ocean biomass losses may widen socioeconomic equity gaps. Nat. Commun. 11:2235. doi: 10.1038/s41467-020-15708-9
Boyd, P. W., Claustre, H., Levy, M., Siegel, D. A., and Weber, T. (2019). Multi-faceted particle pumps drive carbon sequestration in the ocean. Nature 568, 327–335. doi: 10.1038/s41586-019-1098-2
Brander, K. M. (2007). Global fish production and climate change. Proc. Natl. Acad. Sci. U.S.A. 104, 19709–19714. doi: 10.1073/pnas.0702059104
Browning, T. J., Achterberg, E. P., Rapp, I., Engel, A., Bertrand, E. M., Tagliabue, A., et al. (2017). Nutrient co-limitation at the boundary of an oceanic gyre. Nature 551, 242–246. doi: 10.1038/nature24063
Cabré, A, Marinov, I., and Leung, S. (2014). Consistent global responses of marine ecosystems to future climate change across the IPCC AR5 earth system models. Clim. Dynam. 109, 33–57. doi: 10.1007/s00382-014-2374-3
Cai, W., Santoso, A., Wang, G., Wu, L., Collins, M., Lengaigne, M., et al. (2020). “ENSO response to greenhouse forcing,” in El Niño Southern Oscillation in a Changing Climate, Geophysical Monograph Series, 289–307. doi: 10.1002/9781119548164.ch13
Caputi, L., Carradec, Q., Eveillard, D., Kirilovsky, A., Pelletier, E., Pierella Karlusich, J. J., et al. (2019). Community-level responses to iron availability in open ocean plankton ecosystems. Glob. Biogeochem. Cycles 33, 391–419. doi: 10.1029/2018GB006022
Cheng, W., Chiang, J. C. H., and Zhang, D. (2013). Atlantic Meridional Overturning Circulation (AMOC) in CMIP5 models: RCP and historical simulations. J. Clim. 26, 7187–7197. doi: 10.1175/JCLI-D-12-00496.1
Cheung, W. W. L., Bruggeman, J., and Butenschön, M. (2019). “Projected changes in global and national potential marine fisheries catch under climate change scenarios in the twenty-first century,” in Impacts of Climate Change on Fisheries and Aquaculture: Synthesis of Current Knowledge, Adaptation and Mitigation Options, eds T. B. M. Barange, M. C. M. Beveridge, K. Cochrane, S. Funge-Smith, and F. Poulain (Rome: Food and Agriculture Organization of the United Nations), 63–86.
Cheung, W. W. L., Lam, V. W. Y., Sarmiento, J. L., Kearney, K., Watson, R., and Pauly, D. (2009). Projecting global marine biodiversity impacts under climate change scenarios. Fish Fisheries 10, 235–251. doi: 10.1111/j.1467-2979.2008.00315.x
Chinni, V, Singh, S. K., Bhushan, R. R, Rengarajan, V., and Sarma, V. S. S. (2019). Spatial variability in dissolved iron concentrations in the marginal and open waters of the Indian Ocean. Mar. Chem. 208, 11–28. doi: 10.1016/j.marchem.2018.11.007
Collins, M., Knutti, R., Arblaster, J., Dufresne, J. L., Fichefet, T., Friedlingstein, P., et al. (2013). “Long-term climate change: projections, commitments and irreversibility,” in Climate Change 2013: The Physical Science Basis. Contribution of Working Group I to the Fifth Assessment Report of the Intergovernmental Panel on Climate Change, eds T. F. Stocker, D. Qin, G. K. Plattner, M. Tignor, S. K. Allen, J. Boschung, et al. (Cambridge, New York, NY: Cambridge University Press), 1029–1136.
Couespel, D., Lévy, M., and Bopp, L. (2021). Oceanic primary production decline halved in eddy-resolving simulations of global warming. Biogeosci. Discussions 18, 4321–4349. doi: 10.5194/bg-18-4321-2021
Dave, A. C., and Lozier, M. S. (2013). Examining the global record of interannual variability in stratification and marine productivity in the low-latitude and mid-latitude ocean. J. Geophys. Res. Oceans 118, 3114–3127. doi: 10.1002/jgrc.20224
De La Rocha, C. L., and Passow, U. (2014). The biological pump. Treatise Geochem. 6, 93–122. doi: 10.1016/B978-0-08-095975-7.00604-5
Doney, S. C. (2006). Oceanography: plankton in a warmer world. Nature 444, 695–696. doi: 10.1038/444695a
du Pontavice, H., Gascuel, D., Reygondeau, G., Stock, C., and Cheung, W. W. L. (2021). Climate-induced decrease in biomass flow in marine food webs may severely affect predators and ecosystem production. Glob. Chang. Biol. 27, 2608–2622. doi: 10.1111/gcb.15576
Eyring, V., Bony, S., Meehl, G. A., Senior, C. A., Stevens, B., Stouffer, R. J., et al. (2016). Overview of the Coupled Model Intercomparison Project Phase 6 (CMIP6) experimental design and organization. Geosci. Model Dev. 9, 1937–1958. doi: 10.5194/gmd-9-1937-2016
Falkowski, P. G., Barber, R. T., and Smetacek, V. (1998). Biogeochemical controls and feedbacks on ocean primary production. Science 281, 200–206. doi: 10.1126/science.281.5374.200
Falkowski, P. G., Laws, E. A., Barber, R. T., and Murray, J. W. (2003). “Phytoplankton and their role in primary, new, export production,” in Ocean Biogeochemistry: The Role of the Ocean Carbon Cycle in Global Change, ed M. J. R. Fasham (Berlin, Heidelberg: Springer Berlin Heidelberg), 99–121. doi: 10.1007/978-3-642-55844-3_5
Field, C. B. (1998). Primary production of the biosphere: integrating terrestrial and oceanic components. Science 281, 237–240. doi: 10.1126/science.281.5374.237
Friedrichs, M. A., Dusenberry, J. A., Anderson, L. A., Armstrong, R. A., Chai, F., Christian, J. R., et al. (2007). Assessment of skill and portability in regional marine biogeochemical models: role of multiple planktonic groups. J. Geophys. Res. 112. doi: 10.1029/2006JC003852
Fu, W., Randerson, J. T., and Moore, J. K. (2016). Climate change impacts on net primary production (NPP) and export production (EP) regulated by increasing stratification and phytoplankton community structure in the CMIP5 models. Biogeosciences 13, 5151–5170. doi: 10.5194/bg-13-5151-2016
García-Reyes, M., Sydeman, W. J., Schoeman, D. S., Rykaczewski, R. R., Black, B. A., Smit, A. J., et al. (2015). Under pressure: climate change, upwelling, and eastern boundary upwelling ecosystems. Front. Marine Sci. 2:109. doi: 10.3389/fmars.2015.00109
Golden, C. D., Allison, E. H., Cheung, W. W., Dey, M. M., Halpern, B. S., McCauley, D. J., et al. (2016). Nutrition: fall in fish catch threatens human health. Nature 534, 317–320. doi: 10.1038/534317a
Grand, M. M., Measures, C. I., Hatta, M., Morton, P. L., Barrett, P., Milne, A., et al. (2015). The impact of circulation and dust deposition in controlling the distributions of dissolved Fe and Al in the south Indian subtropical gyre. Mar. Chem. 176, 110–125. doi: 10.1016/j.marchem.2015.08.002
Hopwood, M. J., Carroll, D., Dunse, T., Hodson, A., Holding, J. M., Iriarte, J. L., et al. (2020). Review article: how does glacier discharge affect marine biogeochemistry and primary production in the Arctic?. Cryosphere 14, 1347–1383. doi: 10.5194/tc-14-1347-2020
Ito, T., and Follows, M. J. (2005). Preformed phosphate, soft tissue pump and atmospheric CO2. J. Mar. Res. 63, 813–839. doi: 10.1357/0022240054663231
Jones, M. C., and Cheung, W. W. L. (2015). Multi-model ensemble projections of climate change effects on global marine biodiversity. ICES J. Marine Sci. 72, 741–752. doi: 10.1093/icesjms/fsu172
Keerthi, M. G., Lengaigne, M., Levy, M., Vialard, J., Parvathi, V., Boyer Montégut, C. D., et al. (2017). Physical control of interannual variations of the winter chlorophyll bloom in the northern Arabian Sea. Biogeosciences 14, 3615–3632. doi: 10.5194/bg-14-3615-2017
Kociuba, G., and Power, S. B. (2015). Inability of CMIP5 models to simulate recent strengthening of the walker circulation: implications for projections. J. Clim. 28, 20–35. doi: 10.1175/JCLI-D-13-00752.1
Kriest, I., Khatiwala, S., and Oschlies, A. (2010). Towards an assessment of simple global marine biogeochemical models of different complexity. Prog. Oceanogr. 86, 337–360. doi: 10.1016/j.pocean.2010.05.002
Kwiatkowski, L., Aumont, O., and Bopp, L. (2019a). Consistent trophic amplification of marine biomass declines under climate change. Glob. Chang. Biol. 25, 218–229. doi: 10.1111/gcb.14468
Kwiatkowski, L., Aumont, O., Bopp, L., and Ciais, P. (2018). The impact of variable phytoplankton stoichiometry on projections of primary production, food quality and carbon uptake in the global ocean. Glob. Biogeochem. Cycles 32, 516–528. doi: 10.1002/2017GB005799
Kwiatkowski, L., Bopp, L., Aumont, O., Ciais, P., Cox, P. M., Laufkötter, C., et al. (2017). Emergent constraints on projections of declining primary production in the tropical oceans. Nat. Climate Change 7, 355–358. doi: 10.1038/nclimate3265
Kwiatkowski, L., Naar, J., Bopp, L., Aumont, O., Defrance, D., and Couespel, D. (2019b). Decline in atlantic primary production accelerated by greenland ice sheet melt. Geophys. Res. Lett. 46, 11347–11357. doi: 10.1029/2019GL085267
Kwiatkowski, L., Torres, O., Bopp, L., Aumont, O., Chamberlain, M., Christian, J. R., et al. (2020). Twenty-first century ocean warming, acidification, deoxygenation, and upper-ocean nutrient and primary production decline from CMIP6 model projections. Biogeosciences 17, 3439–3470. doi: 10.5194/bg-17-3439-2020
Kwiatkowski, L., Yool, A., Allen, J. I., Anderson, T. R., Barciela, R., Buitenhuis, E. T., et al. (2014). iMarNet: an ocean biogeochemistry model intercomparison project within a common physical ocean modelling framework. Biogeosciences 11, 7291–7304. doi: 10.5194/bg-11-7291-2014
Kwon, E. Y., Primeau, F., and Sarmiento, J. L. (2009). The impact of remineralization depth on the air–sea carbon balance. Nat. Geosci. 2, 630–635. doi: 10.1038/ngeo612
Laufkötter, C., Stern, A. A., John, J. G., Stock, C. A., and Dunne, J. P. (2018). Glacial iron sources stimulate the southern ocean carbon cycle. Geophys. Res. Lett. 45, 13377–313385. doi: 10.1029/2018GL079797
Laufkötter, C., Vogt, M., Gruber, N., Aita-Noguchi, M., Aumont, O., Bopp, L., et al. (2015). Drivers and uncertainties of future global marine primary production in marine ecosystem models. Biogeosciences 12, 6955–6984. doi: 10.5194/bg-12-6955-2015
Lefort, S., Aumont, O., Bopp, L., Arsouze, T., Gehlen, M., and Maury, O. (2015). Spatial and body-size dependent response of marine pelagic communities to projected global climate change. Glob. Chang. Biol. 21, 154–164. doi: 10.1111/gcb.12679
Leung, S., Cabr,é, A, and Marinov, I. (2015). A latitudinally banded phytoplankton response to 21st century climate change in the Southern Ocean across the CMIP5 model suite. Biogeosciences 12, 5715–5734. doi: 10.5194/bg-12-5715-2015
Lewis, K. M., van Dijken, G. L., and Arrigo, K. R. (2020). Changes in phytoplankton concentration now drive increased Arctic Ocean primary production. Science 369, 198–202. doi: 10.1126/science.aay8380
Longhurst, A. R. (2007). “Chapter 1 - toward an ecological geography of the sea,” in Ecological Geography of the Sea (Second Edition), ed A. R. Longhurst (Burlington: Academic Press), 1–17. doi: 10.1016/B978-012455521-1/50002-4
Lotze, H. K., Tittensor, D. P., Bryndum-Buchholz, A., Eddy, T. D., Cheung, W. W., Galbraith, E. D., et al. (2019). Global ensemble projections reveal trophic amplification of ocean biomass declines with climate change. Proc. Natl. Acad. Sci. U.S.A. 116, 12907–12912. doi: 10.1073/pnas.1900194116
Lozier, M. S., Dave, A. C., Palter, J. B., Gerber, L. M., and Barber, R. T. (2011). On the relationship between stratification and primary productivity in the North Atlantic. Geophys. Res. Lett. 38:L18609. doi: 10.1029/2011GL049414
Marra, J., and Barber, R. T. (2005). Primary productivity in the Arabian Sea: a synthesis of JGOFS data. Prog. Oceanogr. 65, 159–175. doi: 10.1016/j.pocean.2005.03.004
Mastrandrea, M. D., Mach, K. J., Plattner, G.-K., Edenhofer, O., Stocker, T. F., Field, C. B., et al. (2011). The IPCC AR5 guidance note on consistent treatment of uncertainties: a common approach across the working groups. Clim. Change 108, 675–691. doi: 10.1007/s10584-011-0178-6
Matear, R. J., Chamberlain, M. A., Sun, C., and Feng, M. (2015). Climate change projection for the western tropical Pacific Ocean using a high-resolution ocean model: implications for tuna fisheries. Deep Sea Res. Topic. Stud. Oceanography 113, 22–46. doi: 10.1016/j.dsr2.2014.07.003
Moffett, J. W., and Landry, M. R. (2020). Grazing control and iron limitation of primary production in the Arabian Sea: Implications for anticipated shifts in Southwest Monsoon intensity. Deep Sea Res. Topic. Stud. Oceanography 179:104687. doi: 10.1016/j.dsr2.2019.104687
Moffett, J. W., Vedamati, J., Goepfert, T. J., Pratihary, A., Gauns, M., and Naqvi, S. W. A. (2015). Biogeochemistry of iron in the Arabian Sea. Limnol. Oceanogr. 60, 1671–1688. doi: 10.1002/lno.10132
Moore, C. M., Mills, M. M., Arrigo, K. R., Berman-Frank, I., Bopp, L., Boyd, P. W., et al. (2013). Processes and patterns of oceanic nutrient limitation. Nat. Geosci. 6, 701–710. doi: 10.1038/ngeo1765
Nakamura, Y., and Oka, A. (2019). CMIP5 model analysis of future changes in ocean net primary production focusing on differences among individual oceans and models. J. Oceanography 75, 441–462. doi: 10.1007/s10872-019-00513-w
Naqvi, S. W. A., Moffett, J. W., Gauns, M. U., Narvekar, P. V., Pratihary, A. K., Naik, H., et al. (2010). The Arabian Sea as a high-nutrient, low-chlorophyll region during the late Southwest Monsoon. Biogeosciences 7, 2091–2100. doi: 10.5194/bg-7-2091-2010
Parvathi, V., Suresh, I., Lengaigne, M., Izumo, T., and Vialard, J. (2017). Robust projected weakening of winter monsoon winds over the arabian sea under climate change. Geophys. Res. Lett. 44, 9833–9843. doi: 10.1002/2017GL075098
Pelegrí, J. L., Csanady, G. T., and Martins, A. (1996). The North Atlantic nutrient stream. J. Oceanography 52, 275–299. doi: 10.1007/BF02235924
Person, R., Aumont, O., Madec, G., Vancoppenolle, M., Bopp, L., and Merino, N. (2019). Sensitivity of ocean biogeochemistry to the iron supply from the Antarctic Ice Sheet explored with a biogeochemical model. Biogeosciences 16, 3583–3603. doi: 10.5194/bg-16-3583-2019
Plesca, E., Grützun, V., and Buehler, S. A. (2018). How robust is the weakening of the pacific walker circulation in CMIP5 idealized transient climate simulations?. J. Clim. 31, 81–97. doi: 10.1175/JCLI-D-17-0151.1
Popova, E. E., Yool, A., Coward, A. C., Dupont, F., Deal, C., Elliott, S., et al. (2012). What controls primary production in the Arctic Ocean? Results from an intercomparison of five general circulation models with biogeochemistry. J. Geophys. Res. 117:C00D12. doi: 10.1029/2011JC007112
Renault, L., Deutsch, C., McWilliams, J. C., Frenzel, H., Liang, J.-H., and Colas, F. (2016). Partial decoupling of primary productivity from upwelling in the California Current system. Nat. Geosci. 9, 505–508. doi: 10.1038/ngeo2722
Richon, C., Aumont, O., and Tagliabue, A. (2020). Prey stoichiometry drives iron recycling by zooplankton in the global ocean. Front. Marine Sci. 7:451. doi: 10.3389/fmars.2020.00451
Richon, C., and Tagliabue, A. (2021). Biogeochemical feedbacks associated with the response of micronutrient recycling by zooplankton to climate change. Glob. Chang. Biol. 27, 4758–4770. doi: 10.1111/gcb.15789
Rickard, G. J., Behrens, E., and Chiswell, S. M. (2016). CMIP5 earth system models with biogeochemistry: an assessment for the southwest Pacific Ocean. J. Geophys. Res. 121, 7857–7879. doi: 10.1002/2016JC011736
Roxy, M. K., Modi, A., Murtugudde, R., Valsala, V., Panickal, S., Prasanna Kumar, S., et al. (2016). A reduction in marine primary productivity driven by rapid warming over the tropical Indian Ocean. Geophys. Res. Lett. 43, 826–833. doi: 10.1002/2015GL066979
Rykaczewski, R. R., Dunne, J. P., Sydeman, W. J., García-Reyes, M., Black, B. A., and Bograd, S. J. (2015). Poleward displacement of coastal upwelling-favorable winds in the ocean's eastern boundary currents through the 21st century. Geophys. Res. Lett. 42, 6424–6431. doi: 10.1002/2015GL064694
Saito, M. A., Goepfert, T. J., and Ritt, J. T. (2008). Some thoughts on the concept of colimitation: three definitions and the importance of bioavailability. Limnol. Oceanogr. 53, 276–290. doi: 10.4319/lo.2008.53.1.0276
Saito, M. A., McIlvin, M. R., Moran, D. M., Goepfert, T. J., DiTullio, G. R., Post, A. F., et al. (2014). Multiple nutrient stresses at intersecting Pacific Ocean biomes detected by protein biomarkers. Science 345, 1173–1177. doi: 10.1126/science.1256450
Sanders, R., Henson, S. A., Koski, M., Christina, L. D. L. R., Painter, S. C., Poulton, A. J., et al. (2014). The biological carbon pump in the North Atlantic. Prog. Oceanogr. 129, 200–218. doi: 10.1016/j.pocean.2014.05.005
Sanderson, B. M., Knutti, R., and Caldwell, P. (2015). A representative democracy to reduce interdependency in a multimodel ensemble. J. Clim. 28, 5171–5194. doi: 10.1175/JCLI-D-14-00362.1
Sathyendranath, S., Platt, T., Kovac, Z., Dingle, J., Jackson, T., Brewin, R. J. W., et al. (2020). Reconciling models of primary production and photoacclimation [Invited]. Appl. Opt. 59, C100–C114. doi: 10.1364/AO.386252
Séférian, R., Berthet, S., Yool, A., Palmieri, J., Bopp, L., Tagliabue, A., et al. (2020). Tracking improvement in simulated marine biogeochemistry between CMIP5 and CMIP6. Curr. Clim. Change Rep. 6, 1–25. doi: 10.1007/s40641-020-00160-0
Séférian, R., Gehlen, M., Bopp, L., Resplandy, L., Orr, J. C., Marti, O., et al. (2016). Inconsistent strategies to spin up models in CMIP5: implications for ocean biogeochemical model performance assessment. Geosci. Model Dev. 9, 1827–1851. doi: 10.5194/gmd-9-1827-2016
Seitzinger, S. P., Mayorga, E., Bouwman, A. F., Kroeze, C., Beusen, A. H., Billen, G., et al. (2010). Global river nutrient export: a scenario analysis of past and future trends. Glob. Biogeochem. Cycles 24. doi: 10.1029/2009GB003587
Singh, D., Ghosh, S., Roxy, M. K., and McDermid, S. (2019). Indian summer monsoon: extreme events, historical changes, and role of anthropogenic forcings. Wiley Interdisciplinary Rev. Climate Change 10:e571. doi: 10.1002/wcc.571
Sooraj, K. P., Terray, P., and Mujumdar, M. (2014). Global warming and the weakening of the Asian summer monsoon circulation: assessments from the CMIP5 models. Climate Dynamics 45, 233–252. doi: 10.1007/s00382-014-2257-7
Spafford, L., and MacDougall, A. H. (2021). Validation of terrestrial biogeochemistry in cmip6 earth system models: a review. Geosci. Model Dev. Discussions 14, 2939–2957. doi: 10.5194/gmd-2021-150
Stock, C. A., John, J. G., Rykaczewski, R. R., Asch, R. G., Cheung, W. W., Dunne, J. P., et al. (2017). Reconciling fisheries catch and ocean productivity. Proc. Natl. Acad. Sci. U.S.A. 114, E1441–E1449. doi: 10.1073/pnas.1610238114
Sunagawa, S., Coelho, L. P., Chaffron, S., Kultima, J. R., Labadie, K., Salazar, G., et al. (2015). Ocean plankton. structure and function of the global ocean microbiome. Science 348:1261359. doi: 10.1126/science.1261359
Tagklis, F., Ito, T., and Bracco, A. (2020). Modulation of the North Atlantic deoxygenation by the slowdown of the nutrient stream. Biogeosciences 17, 231–244. doi: 10.5194/bg-17-231-2020
Tagliabue, A., Aumont, O., DeAth, R., Dunne, J. P., Dutkiewicz, S., Galbraith, E., et al. (2016). How well do global ocean biogeochemistry models simulate dissolved iron distributions?. Global Biogeochem. Cycles 30, 149–174. doi: 10.1002/2015GB005289
Tagliabue, A., Barrier, N., Du Pontavice, H., Kwiatkowski, L., Aumont, O., Bopp, L., et al. (2020). An iron cycle cascade governs the response of equatorial Pacific ecosystems to climate change. Glob. Chang. Biol. 26, 6168–6179. doi: 10.1111/gcb.15316
Taucher, J., and Oschlies, A. (2011). Can we predict the direction of marine primary production change under global warming?. Geophys. Res. Lett. 38. doi: 10.1029/2010GL045934
Terhaar, J., Lauerwald, R., Regnier, P., Gruber, N., and Bopp, L. (2021). Around one third of current Arctic Ocean primary production sustained by rivers and coastal erosion. Nat. Commun. 12:169. doi: 10.1038/s41467-020-20470-z
Terhaar, J., Orr, J. C., Ethé, C, Regnier, P., and Bopp, L. (2019). Simulated arctic ocean response to doubling of riverine carbon and nutrient delivery. Global Biogeochem. Cycles 33, 1048–1070. doi: 10.1029/2019GB006200
Tittensor, D. P., Eddy, T. D., Lotze, H. K., Galbraith, E. D., Cheung, W., Barange, M., et al. (2018). A protocol for the intercomparison of marine fishery and ecosystem models: Fish-MIP v1.0. Geosci. Model Dev. 11, 1421–1442. doi: 10.5194/gmd-11-1421-2018
Twining, B. S., Rauschenberg, S., Baer, S. E., Lomas, M. W., Martiny, A. C., and Antipova, O. (2019). A nutrient limitation mosaic in the eastern tropical Indian Ocean. Deep Sea Res. II Topic Stud. Oceanography 166, 125–140. doi: 10.1016/j.dsr2.2019.05.001
Ustick, L. J., Larkin, A. A., Garcia, C. A., Garcia, N. S., Brock, M. L., Lee, J. A., et al. (2021). Metagenomic analysis reveals global-scale patterns of ocean nutrient limitation. Science 372, 287–291. doi: 10.1126/science.abe6301
Vancoppenolle, M., Bopp, L., Madec, G., Dunne, J., Ilyina, T., Halloran, P. R., et al. (2013). Future Arctic Ocean primary productivity from CMIP5 simulations: uncertain outcome, but consistent mechanisms. Global Biogeochem. Cycles 27, 605–619. doi: 10.1002/gbc.20055
Vichi, M., Allen, J. I., Masina, S., and Hardman-Mountford, N. J. (2011). The emergence of ocean biogeochemical provinces: a quantitative assessment and a diagnostic for model evaluation. Glob. Biogeochem. Cycles 25. doi: 10.1029/2010GB003867
Vichi, M., Pinardi, N., and Masina, S. (2007). A generalized model of pelagic biogeochemistry for the global ocean ecosystem. Part I: theory. J. Marine Syst. 64, 89–109. doi: 10.1016/j.jmarsys.2006.03.006
Villarino, E., Chust, G., Licandro, P., Butenschön, M., Ibaibarriaga, L., Larrañaga, A., et al. (2015). Modelling the future biogeography of North Atlantic zooplankton communities in response to climate change. Mar. Ecol. Prog. Ser. 531, 121–142. doi: 10.3354/meps11299
Vizcaíno, M., Mikolajewicz, U., Gröger, M., Maier-Reimer, E., Schurgers, G., and Winguth, A. M. E. (2008). Long-term ice sheet–climate interactions under anthropogenic greenhouse forcing simulated with a complex Earth System Model. Clim. Dynamics 31, 665–690. doi: 10.1007/s00382-008-0369-7
Volk, T., and Hoffert, M. I. (1985). “Ocean carbon pumps: analysis of relative strengths and efficiencies in ocean-driven atmospheric CO2 changes,” in The Carbon Cycle and Atmospheric CO2: Natural Variations Archean to Present, Geophysical Monograph Series, 99–110. doi: 10.1029/GM032p0099
Wang, D., Gouhier, T. C., Menge, B. A., and Ganguly, A. R. (2015). Intensification and spatial homogenization of coastal upwelling under climate change. Nature 518, 390–394. doi: 10.1038/nature14235
Weijer, W., Cheng, W., Garuba, O. A., Hu, A., and Nadiga, B. T. (2020). CMIP6 models predict significant 21st century decline of the atlantic meridional overturning circulation. Geophys. Res. Lett. 47. doi: 10.1029/2019GL086075
Whitt, D. B., and Jansen, M. F. (2020). Slower nutrient stream suppresses Subarctic Atlantic Ocean biological productivity in global warming. Proc. Natl. Acad. Sci. U.S.A. 117, 15504–15510. doi: 10.1073/pnas.2000851117
Wrightson, L., and Tagliabue, A. (2020). Quantifying the impact of climate change on marine diazotrophy: insights from earth system models. Front. Marine Sci. 7:635. doi: 10.3389/fmars.2020.00635
Xiu, P., Chai, F., Curchitser, E. N., and Castruccio, F. S. (2018). Future changes in coastal upwelling ecosystems with global warming: the case of the California Current System. Sci. Rep. 8:2866. doi: 10.1038/s41598-018-21247-7
Yool, A., Martin, A. P., Anderson, T. R., Bett, B. J., Jones, D. O. B., and Ruhl, H. A. (2017). Big in the benthos: future change of seafloor community biomass in a global, body size-resolved model. Glob. Chang. Biol. 23, 3554–3566. doi: 10.1111/gcb.13680
Yool, A., Palmiéri, J., Jones, C. G., de Mora, L., Kuhlbrodt, T., Popova, E. E., et al. (2021). Evaluating the physical and biogeochemical state of the global ocean component of UKESM1 in CMIP6 historical simulations. Geosci. Model Dev. 14, 3437–3472. doi: 10.5194/gmd-14-3437-2021
Zehr, J. P., and Capone, D. G. (2020). Changing perspectives in marine nitrogen fixation. Science 368:eaay9514. doi: 10.1126/science.aay9514
Keywords: climate change, ocean net primary production, earth system model (ESM), climate projections, ocean modeling, oceanography, ocean biogeochemical cycles, ocean biogeochemical model
Citation: Tagliabue A, Kwiatkowski L, Bopp L, Butenschön M, Cheung W, Lengaigne M and Vialard J (2021) Persistent Uncertainties in Ocean Net Primary Production Climate Change Projections at Regional Scales Raise Challenges for Assessing Impacts on Ecosystem Services. Front. Clim. 3:738224. doi: 10.3389/fclim.2021.738224
Received: 08 July 2021; Accepted: 14 October 2021;
Published: 25 November 2021.
Edited by:
Zita Sebesvari, United Nations University, JapanReviewed by:
Kewei Lyu, Oceans and Atmosphere (CSIRO), AustraliaAlessandro Stocchino, Hong Kong Polytechnic University, Hong Kong SAR, China
Copyright © 2021 Tagliabue, Kwiatkowski, Bopp, Butenschön, Cheung, Lengaigne and Vialard. This is an open-access article distributed under the terms of the Creative Commons Attribution License (CC BY). The use, distribution or reproduction in other forums is permitted, provided the original author(s) and the copyright owner(s) are credited and that the original publication in this journal is cited, in accordance with accepted academic practice. No use, distribution or reproduction is permitted which does not comply with these terms.
*Correspondence: Alessandro Tagliabue, YS50YWdsaWFidWVAbGl2ZXJwb29sLmFjLnVr