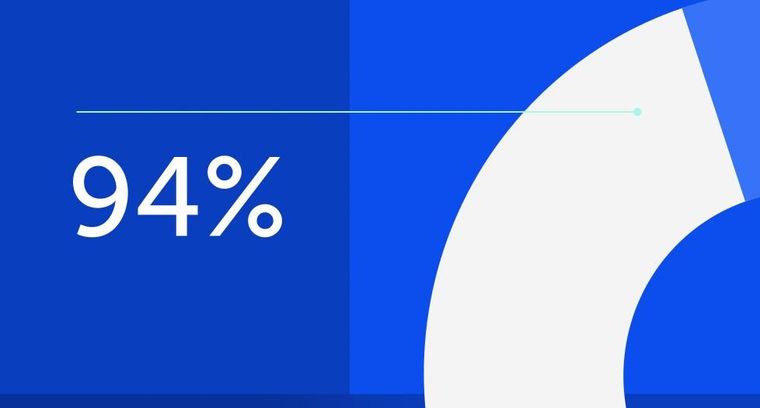
94% of researchers rate our articles as excellent or good
Learn more about the work of our research integrity team to safeguard the quality of each article we publish.
Find out more
PERSPECTIVE article
Front. Clim., 25 March 2021
Sec. Climate Risk Management
Volume 3 - 2021 | https://doi.org/10.3389/fclim.2021.650760
This article is part of the Research TopicOpen Citizen Science Data and MethodsView all 28 articles
Citizen science is an important vehicle for democratizing science and promoting the goal of universal and equitable access to scientific data and information. Data generated by citizen science groups have become an increasingly important source for scientists, applied users and those pursuing the 2030 Agenda for Sustainable Development. Citizen science data are used extensively in studies of biodiversity and pollution; crowdsourced data are being used by UN operational agencies for humanitarian activities; and citizen scientists are providing data relevant to monitoring the sustainable development goals (SDGs). This article provides an International Science Council (ISC) perspective on citizen science data generating activities in support of the 2030 Agenda and on needed improvements to the citizen science community's data stewardship practices for the benefit of science and society by presenting results of research undertaken by an ISC-sponsored Task Group.
Citizen science is an important vehicle for democratizing science and promoting the goal of universal and equitable access to scientific data and information. While the benefits of civic engagement and the contributions of citizen science (CS) to societal goals such as environmental justice are widely recognized, perhaps less understood is the critical importance of data as an output of citizen science projects. Yet, data need to be recognized as a long-lived legacy of CS activities and an important contribution to scientific research. The International Science Council's (ISC; formerly ICSU) acts as the “voice of science”, with the vision that scientific knowledge, data and expertise are universally accessible, and their benefits universally shared. Accessibility to scientific knowledge and sharing its benefits are also values associated with citizen science. Through its work the ISC is promoting data stewardship and dissemination in the CS community so as to magnify the impact of citizen science on policy and programs related to (among other things) attainment of the U.N. Sustainable Development Goals (SDGs) (see also Fritz et al., 2019; Fraisl et al., 2020).
To that end, in 2016 the ISC established a joint Task Group on Citizen Science Data under the auspices of its two data-related bodies, the Committee on Data (CODATA), which focuses on data policy and capacity building in data science, and the World Data System (WDS), which focuses on promoting the value and sustainability of Trustworthy Data Repositories (TDRs) that provide data stewardship, long-term preservation, and access to quality-assured data. In its first incarnation, the CODATA–WDS Task Group focused on understanding the ecosystem of data-generating CS and crowdsourcing projects so as to characterize the potential of and challenges for science as a whole and data science in particular.1 The interest was in evaluating CS practices throughout the data lifecycle. To that end, the Task Group (TG) conducted a survey of data collection, validation, curation, and management practices for a sample of 36 CS projects globally representing different research domains, types of CS practices, and regions (results published in Bowser et al., 2020).
In its second incarnation, with some change in membership, the TG turned to the question of how CS can contribute to the evidence base for monitoring and driving progress toward achievement of the SDGs.2 To advance research in this area, in 2020 the TG collected data on 44 CS projects in Sub-Saharan Africa linked to the water and sanitation (SDG 6) and urban development (SDG 11) SDGs. The TG also developed guidance for CS groups that wish to contribute data to SDG monitoring efforts by “unpacking” the often opaque language surrounding the SDG goal, target, and indicator framework by presenting key information in layperson's terms. The purpose of this work is to provide “handles” that allow citizen groups to contribute to filling data gaps and tracking the progress of government agencies and other actors in monitoring and fulfilling the SDGs.
This article provides an ISC perspective on the topic based on these efforts and the views of the co-authors, who have all served as TG members. From an ISC perspective, citizen science is an important vehicle for democratizing science and promoting the goal of universal and equitable access to scientific data and information. Beyond evaluating citizen science and its data products from the perspective of its utility to professional scientists (a primary focus of the work of the first TG), the ISC understands that CS can be a vehicle for addressing interlinked environmental and development issues that are of the highest concern to communities (a major focus of the second TG) (International Council for Science (ISC), 2017). These include environmental justice and equitable access to basic services such as clean water, food, education and health services.
It should be noted that CS is an evolving practice that covers many disciplinary areas and types of citizen contributions, from crowdsourcing using online platforms to relatively passive modes of data collection using sensors to extreme CS (often conducted under the auspice of terms like “community based participatory research”), in which citizens are involved in all phases from problem definition to protocol development and implementation (Haklay, 2013). This complexity makes generalizations perilous. Hence findings presented in this perspective must necessarily be seen as partial, though still helpful for highlighting common data practices among CS projects and understanding the potential that CS holds for democratizing data.
In 2017 the TG launched a research project to understand “the State of the Data in Citizen Science.” The TG developed a sampling framework for capturing the diversity of citizen science projects, including topical areas, geographic scale or scope, location, type of data collection or data analysis, and project governance model. This resulted in a sample of 36 CS projects. TG members then surveyed CS project principals using an interview instrument designed to elicit self-reported practices on aspects of the data lifecycle and data management, including information on data quality assurance and quality control (QA/QC), technical infrastructure, and data governance, documentation, and access.
Some of the most vocal criticisms of citizen science involve the perceived quality of citizen science data (e.g., Nature, 2015). We found that many of the projects in our sample had robust mechanisms for ensuring data quality −94% of projects surveyed used one method or more, and 56% used five methods or more. This suggests that data quality itself is not a major issue in CS, but rather the documentation (or lack thereof) of publicly reported QA/QC and practices is a main opportunity for improvement. We also found opportunities for improvement around data storage, management, and access. For example, compared to the large number of projects employing a diverse range of QA/QC mechanisms, fewer projects provided easy access to open data, offered a persistent unique identifier (UID), or selected an open license. Still, in line with norms around providing feedback to guide and motivate continued participation, the majority of projects (83%) found some way to share findings with their volunteers.
The complete description of research findings can be found in the journal article by Bowser et al. (2020). In addition, as a complementary practical resource the TG offered a summary of recommendations in six areas of the data lifecycle. Here, based in part on the article and on findings from ongoing work, we offer some updated recommendations for at least two audiences: citizen science projects seeking to improve their own data-related practices, and therefore elevate the value of their data for reuse, and a growing number of supporting platforms, infrastructures, and communities that are supporting citizen science projects in data curation, validation, and management.
Many projects already ensure that volunteers receive training, sensors undergo initial calibration checks, and assessments are made for individual devices and contributors. Some projects are also leveraging “big data” quality strategies, including methods to flag outliers for further checks, or incorporating uncertainty metrics for devices, volunteers, and individual measurements (e.g., Kelling et al., 2015). For projects that seek to promote the re-use of their data, or for supporting platforms, initial analysis on the quality of the collection, sampling approaches, and triangulation against other datasets encourages reuse and further increases the credibility of CS data in the scientific community. To improve data quality assessments, we recommend that CS projects with minimal privacy concerns could store the data in its most disaggregated form, explicitly state likely biases in sampling [e.g., over-sampling in nature preserves or on weekends (Cooper, 2014)], and document these assessments along with their QA/QC practices on websites and/or through formal QA/QC plans.
Many CS platforms, such as iNaturalist, OpenStreetMap, BioCollect, and CitSci.org, already offer existing “infrastructures” of technological platforms and communities. Other projects may develop their own technological platforms and systems. To the degree possible, we encourage new projects to consider leveraging existing, already tested infrastructures across the data lifecycle rather than establishing entirely new and distinct platforms. In cases where new developments become necessary, it is critical to partner with existing open-source technology and standards development communities to ensure that best practices are achieved. For example, working groups of the U.S. based Citizen Science Association (CSA) and Open Geospatial Consortium (OGC) have already established guidelines for metadata documentation and/or standards for data collection and sharing.
Both within and beyond citizen science, there are benefits to data archiving in large and stable data repositories, where they can be aggregated with data from other CS efforts as well as data from other research methodologies (see data access below). Ideally, to ensure long-term data preservation, an archiving strategy should involve more than one copy, use different media technologies, and preserve the datasets at different locations (Eynden et al., 2011; Parsons et al., 2011). Raw data and metadata should also be retained to allow subsequent reprocessing (Danielsen et al., 2020).
Relevant considerations include privacy and ethical data use, including ensuring the protection of sensitive location-based information, personally identifiable information (PII), and proper use of licensing. CS projects should carefully consider tradeoffs between openness and privacy. For example, while many citizen science projects embrace openness as a scientific ideal and support data re-use, there are also legitimate concerns around the safety of endangered or threatened species and the privacy of citizen science volunteers who may share data from sensitive locations (Bowser et al., 2017; Johnson et al., 2021). Moreover, CS projects should ensure that data ownership and data use rights are clearly stated and reflect the priorities of the volunteers (see also data licenses below).
As discussed earlier, assessments of data quality and fitness-for-purpose can be supported with documentation on QA/QC methods on project websites. Documentation is also needed to describe exactly how the data were collected, including information on specific protocols (Assumpção et al., 2018). One opportunity for sharing this information is posting it along with QA/QC methods on project websites. In addition, as existing work on data and metadata standards and supporting platforms continues to evolve, tools such as data catalogs could document standardized information on methodologies for external parties to discover and assess. The field of CS would benefit from increased resources to support data documentation, which promotes confidence in the data as well as reuse.
In terms of data discovery and access, 28% of projects surveyed made data available through a topical or field-based repository (such as the Global Biodiversity Information Facility), 22% through an institutional repository, 11% through a public sector data repository, and 6% through a publication-based repository. This broadly corresponds with the practices by scientists more generally (Tenopir et al., 2015). CS projects can encourage re-use by providing easy access to their data in standardized formats. Multiple download options such as raw and cleaned data, temporal and spatial subsets, and format options such as spreadsheets, geographic formats, and API access can help to eliminate the barriers to use and meet the needs of data users. The ability to subset the data is particularly beneficial in regions with limited bandwidth. Note that broader open science efforts are required to promote open access to citizen science data, along with other types of scientific knowledge.
In addition to making data open, additional mechanisms are required to make data findable, accessible, interoperable, and reusable (FAIR; Wilkinson et al., 2016). We recommend the adoption of open, machine-readable licenses. Our research found that Creative Commons licenses are frequently used in citizen science (e.g., CC BY 4.0, which promotes attribution of the data authors but otherwise does not restrict use). While seemingly “progressive” and in keeping with the community ethos of some CS initiatives, the restriction on commercial uses (such as CC BY-NC 2.0) or the inappropriate application of share-alike licenses (such as CC BY-SA 3.0) can prevent third parties from providing value-added data and services based on raw data that are of benefit to society. Other licenses, such as the Open Data Commons Open DataBase License (ODbL), may also be appropriate for projects seeking to maximize data reuse (see Cooper et al., 2021, this Research Topic).
In 2019, as the above work was being finalized, the TG turned its attention to understanding challenges and opportunities for citizen science to contribute to the SDGs. Our findings in this area, based on a 2020 survey of 44 CS initiatives in Sub-Saharan Africa, are more preliminary. We focused on water supply and access (SDG 6) and urban planning and sanitation (SDG 11) out of a recognition that these two areas are of high concern in Africa (Stren, 2019), and the fact that projects in these domains are more likely to be driven by community concerns rather than donor interests (Jameson et al., 2020). The survey was of a representative mix of projects across regions of sub-Saharan Africa, with roughly 39% of projects from West Africa, 27% each from Central and East Africa, and 7% from Central Africa.3 The authors identified respondents in a number of countries through TG members and regional CS experts, then employed snowball sampling to identify additional respondents. All respondents were directed to the link for a Google form or interviewed in person using the same instrument.
Examples of surveyed projects include the Nigeria Slum/Informal Settlement Federation, the Clean and Green Congo project in the Democratic Republic of Congo, the Citizen Land and Service Project in Ghana, Map Kibera in Nairobi, Kenya, and the AfriWatSan project in Uganda. Domains represented by the CS projects (in descending order of frequency considered in our survey) include mapping of resources, urban planning, urban sanitation, ecosystems and ecology, disaster risk management, and transportation, among others. Common tools used by the projects include smartphones, sensors, test kits, and a variety of geospatial tools (GPS, GIS, OpenStreetMap, etc.) and the primary purposes were to educate the public, advance research and ensure that evidence-based policies are enacted.
Our findings suggest that CS projects have the potential to contribute to SDG tracking through participatory data collection, standardized data collection across cities, and improved data accessibility for decision making and science. Perhaps the two most important contributions from an equity lens are in understanding community perspectives and generating data at local levels (which are critical for the Leave No One Behind focus of the 2030 Agenda), and promoting the empowerment of communities to negotiate with authorities on service delivery. However, barriers still remain to getting citizen science used in SDG reporting, due to issues such as an inherent lack of trust in citizen-generated data, as well as (in some cases) inconsistent adherence to best practices for data management, including those described above.
While the use of citizen-generated data by decision makers is not yet widespread, trust and acceptability have been found to increase the chances of data use. City officials in Lagos have used CS generated data to select communities for revitalization and service provision, and National Statistical Offices (NSOs) in Kenya and Ghana have expressed openness to CS generated data on the grounds that data are scarce, no agency can monitor all 17 SDGs, and such data can mobilize community and government cooperation. Some specific examples of data use by governments came out of SDG6-related projects in southern Africa. For example, the uMkhomazi Landscape Restoration Project in South Africa states that the government is supportive and is seeking to integrate data from citizen science into catchment management, whereas WaterAid in Eswatini (former Swaziland) mentioned decision makers' understanding of the potential to use the data to inform better planning and budgeting for water supplies, however financial constraints have limited government action.
Recognizing that most citizen groups are not trained in the processes developed by NSOs to ensure the consistent collection of robust data, citizen-generated data may need to be validated by an NSO before inclusion in official SDG reporting. It has been suggested that such data may therefore be viewed mainly as a complement to data from conventional sources and could be provided alongside official statistics. Viewed from the CS perspective, Jameson et al. (2020) argue that citizen science in low-income contexts should not only be viewed in terms of the value of data production but also as a means of empowering and engaging communities. Thus, rather than requiring that citizen scientists adhere to rigorous protocols and sustain data collection efforts over long periods, CS projects are perhaps best positioned to identify gaps in data acquisition and to highlight community concerns, and as a tool for lobbying for better services and hopefully sustained and consistent data collection by government agencies on issues of importance to communities.
The complexity of the SDG indicators suggests that they have not been developed with a view to enabling lay-people to monitor them (Fritz et al., 2019). Where CS groups do wish to contribute to sustained monitoring of SDG progress, they need tools to do so. Thanks to multiple interactions of members of the TG with experts at the UN, governments and NSOs on one side, and citizens in the field on the other, it became clear that some of the limits to engagement and adoption of improved data collection practices lie in misunderstanding and miscommunication between the two groups. The requirements that bodies like the UN and NSOs have for data, including quantity, quality, collection procedures, and the needs for specific measurements are quite strict. For CS data to be useful in this context, CS groups need to be aware of such criteria. However, the jargon and complexity of official requirements is often impenetrable to citizen groups, which can represent a barrier to engagement.
In order to explore the extent of this challenge, we sought to demystify the official requirements for a selection of SDG indicators by translating them into layperson's language. The TG worked with five indicators and produced for each a compendium4 including concepts and definitions of the goal, target and indicator; a global overview on the current progress in attaining the target; the computation method and an example of implementation; the rationale, significance and consequences of implementing the indicator; and suggestions on how a citizen can participate and contribute. Documentation is necessary to raise awareness on how data need to be collected for selected SDG indicators, and to present citizen science projects with clear opportunities for participation.
Also, those seeking to re-use CS data—particularly for national or international reporting and assessment processes—need to meet citizen science projects halfway (Eicken et al., 2021). Even when citizen science projects are following scientific best practices for collecting, analyzing, and sharing data, governing bodies like the UN typically have additional requirements for monitoring and assessment processes. For example, efforts are underway to promote CS contributions to reporting progress on SDG 14.1.1.b, which assesses plastics pollution in oceans, by including an indicator for citizen science collected data on beach litter (Campbell et al., 2019). A UN advisory group produced an 138-page report on plastics pollution (Joint Group of Experts on the Scientific Technical Aspects of Marine Environmental Protection (GESAMP), 2019) that is too dense and detailed for most individuals or citizen science groups. Recognizing a gap to be filled between such detailed guidelines and the need for actionable, on-the-ground guidelines, UNEP convened a workshop in December 2020 to discuss how to effectively leverage citizen science for SDG reporting that considered both UNEP and CS perspectives. Similar efforts around SDGs 6, 11, and others could further bridge lingering gaps.
That said, whereas some types of indicators are amenable to involvement of local stakeholders in their monitoring (Danielsen et al., 2013), others are best suited to expert-driven assessment (e.g., indicators that require a national overview or detailed knowledge of administrative or legislative aspects). This suggests that just as citizen science data may be fit for a particular purpose, participation through citizen science should also be conducted with explicit acknowledgment of achievable end goals that benefit data users and citizens alike.
In order to leverage the potential of citizen science to address grand challenges like the SDGs, more work is needed, both on good data practices, and on alignment between data and decision-making. The ISC's action plan for 2019–2021, Advancing Science as a Global Public Good (International Science Council (ISC), 2019), revolves around four domains considered as the major challenges for society to which science—and the ISC as a global voice for science—must respond. The fact that the first of these challenge domains is “The 2030 Agenda for Sustainable Development” highlights the ISC's leadership role in the post-2015 development processes of the United Nations, and its strong commitment to work with its members and other international scientific organizations, funders, government agencies, NGOs and the prívate sector toward meeting the SDGs.
CODATA's strategic plan focuses, among other things, on the contribution of research data and analysis to indicators supporting the 2030 Agenda and the Sendai Framework for Disaster Risk Reduction. This is part of a broader effort on making data work for cross-domain grand challenges, including data interoperability and reuse—i.e., FAIR data. It is important for the CS community and domain experts to continue to develop agreed upon standards and ontologies for data access and integration (i.e., accessibility and interoperability). The CODATA-RDA School of Research Data Science, a strategic program to train early career researchers from low and middle income countries in data skills, has developed short courses and held summer schools over the last 5 years. The school is open to CS practitioners and could be a valuable mechanism for them to gain additional data science skills.
For its part, WDS underscores in its 2019–2023 Strategic Plan the importance of all scientific data being preserved for the long-term in trustworthy data repositories, including citizen-generated data (World Data System (WDS), 2019). This is vital to both the integrity and the acceleration of science, since it moves toward FAIR data practices for current and future generations of scientists seeking to address the grand challenges. WDS encourages citizen science groups that maintain their own data holdings to become TDRs by becoming CoreTrustSeal certified,5 and ultimately WDS Regular Members. This would ensure that they become more integral parts of the research data infrastructure through involvement in international collaborative programmes sponsored by ISC and beyond.
The work by the Task Group has contributed to a better understanding of the data management practices and needs of the CS community, including practical challenges facing smaller groups with limited financial and human resources. Clearly, given the range in scales and foci of activities among CS groups globally, a one-size-fits-all strategy will not work. And, as mentioned, the primary goal of all CS projects is often not data generation (Johnson et al., 2021). But, for medium- to large-sized data generating CS projects, the TG supports efforts to develop standards and to incorporate CS data into global research data infrastructure–as is already happening with ornithological data collected by eBird, which is deposited in the Global Biodiversity Information Facility (GBIF), a WDS regular member (Chandler et al., 2017). The TG also recognizes that citizen science projects often unfold in environments with limited resources. While we believe that identifying and recommending good data practices will help advance the field and enable more scientific research, we also understand that additional work will be needed to help citizen science projects translate these recommendations into concrete practices.
In 2021, the TG is developing a report on CS for SDGs 6 and 11 in Sub-Saharan Africa that will include practical guidelines for CS groups wanting to contribute to SDG monitoring in the urban water, sanitation and environmental planning domains. This can support the work of urban managers and UN agencies such as UN-HABITAT, as well as highlight the way citizen engagement can improve the lot of millions of urban residents across the continent.
The data analyzed in this study is subject to the following licenses/restrictions: The data are preliminary. Requests to access these datasets should be directed to cGVsaWFzQHVuaWxhZy5lZHUubmc=.
The article development was led by AdS, AB, T-RC, CC, FD, RE, EF, CH, RM, and IP contributed text to the article. AdS, AB, CC, PE, EF, RM, AS, and KS contributed to research efforts the findings from which are presented in this article. All authors contributed to the article and approved the submitted version.
Ads acknowledges funding from the NASA contract NNG13HQ04C for the continued operation of the Socioeconomic Data and Applications Center (SEDAC). AB recognizes support from the Alfred P. Sloan Foundation. AB, AS, and KS received stipends from CODATA for research activities. TRC acknowledges support from the Research Center for Information Technology Innovation, Academia Sinica, for the project Data Collaboration and Digital Preservation. PE acknowledges funding from the LIRA 2030 Africa Programme by the International Science Council and NASAC Grant No: LIRA2030-GR01/18 for research work. FD received support from EC H2020 projects INTAROS, CAPARDUS, and FRAMEwork (Grants 727890, 869673, and 862731).
The authors declare that the research was conducted in the absence of any commercial or financial relationships that could be construed as a potential conflict of interest.
The authors would like to thank CODATA and particularly Simon Hodson for support of the Task Group, as well as other members of the two CODATA-WDS Task Groups who contributed to the thinking that underlies this paper.
1. ^The full TG remit and membership can be found at https://codata.org/initiatives/task-groups/previous-tgs/citizen-science-and-crowdsourced-data/.
2. ^The remit and membership of the second TG can be found at https://codata.org/initiatives/task-groups/citizen-science-for-the-sustainable-development-goals/.
3. ^Almost half of the projects were in Nigeria and South Africa, which reflects TG member contacts but may also very well reflect high engagement with CS in these two countries. The team found it difficult to locate CS representatives without in-country contacts who could identify the main actors and provide up-to-date email addresses or cell phone numbers, since most information on the Internet is unreliable.
4. ^To access these “how to” guides, follow the link in footnote 2. Goal 3: good health and well-being - target 3.1: by 2030, reduce the global maternity ratio to less than 70 per 100,000 live births—indicator 3.1.1: maternal mortality ratio; Goal 11: sustainable cities and communities—target 11.6: by 2030, reduce the adverse per capita environmental impact of cities, including by paying special attention to air quality and municipal and other waste management—indicator 11.6.1: proportion of municipal solid waste collected and managed in controlled facilities out of total municipal waste generated, by cities; Goal 13: climate action—target 13.1: strengthen resilience and adaptive capacity to climate related hazards and natural disasters in all countries—indicator 13.1.2: number of countries that adopt and implement disaster risk reduction (DRR) strategies in line with the Sendai Framework for DRR 2015-2030; Goal 15: life on land—target 15.5: take urgent and significant action to reduce the degradation of natural habitats, halt the loss of biodiversity and, by 2020, protect and prevent the extinction of threatened species—indicator 15.5.1: red list index.
5. ^For more information visit https://www.coretrustseal.org/.
Assumpção, T. H., Popescu, I., Jonoski, A., and Solomatine, D. P. (2018). Citizen observations contributing to flood modelling: Opportunities and challenges. Hydrol. Earth Syst. Sci. 22, 1473–1489. doi: 10.5194/hess-22-1473-2018
Bowser, A., Cooper, C., de Sherbinin, A., Wiggins, A., Brenton, P., Chuang, T. R., et al. (2020). Still in need of norms: the state of the data in citizen science. Citizen Sci. Theor. Pract. 5:303. doi: 10.5334/cstp.303
Bowser, A., Shiltonk, K., Preece, J., and Warrick, E. (2017). “Accounting for privacy in citizen science: ethical research in a context of openness,” in Proceedings of the 2017 ACM Conference on Computer Supported Cooperative Work and Social Computing (CSCW '17) (Portland, OR), 2124–2136. doi: 10.1145/2998181.2998305
Campbell, J., Bowser, A., Fraisl, D., and Meloche, M. (2019). “Citizen science and data integration for understanding marine litter,” in Data for Good Exchange (New York, NY: IIASA). Available online at: https://pure.iiasa.ac.at/id/eprint/16095/ (accessed March 10, 2021).
Chandler, M., See, L., Copas, K., Bonde, A. M., López, B. C., Danielsen, F., et al. (2017). Contribution of citizen science towards international biodiversity monitoring. Biol. Conserv. 213, 280–294. doi: 10.1016/j.biocon.2016.09.004
Cooper, C. B. (2014). Is there a weekend bias in clutch-initiation dates from citizen science? Implications for studies of avian breeding phenology. Int. J. Biometeorol. 58, 1415–1419. doi: 10.1007/s00484-013-0742-z
Cooper, C. B., Jones, E., and Rasmussen, L. (2021). The power (dynamics) of open data in citizen science. Frontiers. (submitted for the Research Topic on Citizen Science Data and Methods).
Danielsen, F., Johnson, N., Lee, O., Fidel, M., Iversen, L., Poulsen, M. K., et al. (2020). Community-Based Monitoring in the Arctic. Fairbanks, AK: University of Alaska Press.
Danielsen, F., Pirhofer-Walzl, K., Adrian, T., Kapijimpanga, D., Burgess, N. D., Jensen, P. M., et al. (2013). Linking public participation in scientific research to the indicators and needs of international environmental agreements. Conserv. Lett. 7, 12–24. doi: 10.1111/conl.12024
Eicken, H., Danielsen, F., Sam, J.-M., Fidel, M., Johnson, N., Poulsen, M. K., et al. (2021). Connecting top-down and bottom-up approaches in environmental observing. BioScience. (in press).
Eynden, V., van den Corti, L., Woollard, M., Bishop, L., and Horton, L. (2011). Managing and Sharing Data; A Best Practice Guide for Researchers. Essex: University of Essex Press.
Fraisl, D., Campbell, J., See, L., Wehn, U., Wardlaw, J., Gold, M., et al. (2020). Mapping citizen science contributions to the UN sustainable development goals. Sustain. Sci. 15, 1735–1751. doi: 10.1007/s11625-020-00833-7
Fritz, S., See, L., and West, S. (2019). Citizen science and the United Nations sustainable development goals. Nat. Sustain. 2, 922–930. doi: 10.1038/s41893-019-0390-3
Haklay, M. (2013). “Citizen science and volunteered geographic information: overview and typology of participation,” in Crowdsourcing Geographic Knowledge (Dordrecht: Springer), 105–122. Available online at: https://link.springer.com/chapter/10.1007/978-94-007-4587-2_7 (accessed March 10, 2021).
International Council for Science (ISC). (2017). A Guide to SDG Interactions: From Science to Implementation, eds D. J. Griggs, M. Nilsson, A. Stevance and D. McCollum (Paris: ISC). Available online at: https://council.science/wp-content/uploads/2017/05/SDGs-Guide-to-Interactions.pdf (accessed March 10, 2021).
International Science Council) (ISC). (2019). Advancing Science as a Global Public Good—Action Plan 2019- 2021. Paris: ISC. Available online at: https://council.science/wp-content/uploads/2019/12/Advancing-Science-as-a-Global-Public-Good_ISC-Action-Plan-landscape.pdf (accessed March 10, 2021).
Jameson, S. D., Lammerhirt, E., and Prasetyo (2020). Acting locally, Monitoring Globally? How to Link Citizen-Generated Data to SDG Monitoring. Report by CIVICUS, The Open Institute, Wingu, and The Engine Room.
Johnson, N., Druckenmiller, M. L., Danielsen, F., and Pulsifer, P. L. (2021). The use of digital platforms for community-based monitoring. BioScience. (in press).
Joint Group of Experts on the Scientific Technical Aspects of Marine Environmental Protection (GESAMP). (2019). Guidelines for the Monitoring and Assessment of Plastic Litter in the Ocean. United Nations Office Nairobi (UNON), Publishing Services Section. Available online at: https://environmentlive.unep.org/media/docs/marine_plastics/une_science_dvision_gesamp_reports.pdf (accessed March 10, 2021).
Kelling, S., Fink, D., La Sorte, F., Johnston, A., Bruns, N., and Hochachka, W. (2015). Taking a “Big Data” approach to data quality in a citizen science project. Ambio 44, 601–611. doi: 10.1007/s13280-015-0710-4
Parsons, M. A., Godøy, Ø., LeDrew, E., De Bruin, T. F., Danis, B., Tomlinson, S., et al. (2011). A conceptual framework for managing very diverse data for complex, interdisciplinary science. J. Inf. Sci. 37, 555–569. doi: 10.1177/0165551511412705
Stren, R. E. (Ed.). (2019). African Cities in Crisis: Managing Rapid Urban Growth. New York, NY: Routledge.
Tenopir, C., Dalton, E. D., Allard, S., Frame, M., Pjesivac, I., Birch, B., et al. (2015). Changes in data sharing and data reuse practices and perceptions among scientists worldwide. PLoS ONE 10:e0134826. doi: 10.1371/journal.pone.0134826
Wilkinson, M., Dumontier, M., Aalbersberg, J. J., Appleton, G., Axton, M, Baak, A., et al. (2016). The FAIR guiding principles for scientific data management and stewardship. Sci. Data 3:160018. doi: 10.1038/sdata.2016.18
World Data System (WDS) (2019). WDS Strategic Plan 2019–2023. Available online at https://www.worlddatasystem.org/files/WDS_Strategic_Plan_2019-2023.pdf (accessed March 10, 2021).
Keywords: data management, data life cycle, citizen science, sustainable development goals, applied research
Citation: de Sherbinin A, Bowser A, Chuang T-R, Cooper C, Danielsen F, Edmunds R, Elias P, Faustman E, Hultquist C, Mondardini R, Popescu I, Shonowo A and Sivakumar K (2021) The Critical Importance of Citizen Science Data. Front. Clim. 3:650760. doi: 10.3389/fclim.2021.650760
Received: 07 January 2021; Accepted: 02 March 2021;
Published: 25 March 2021.
Edited by:
Jose A. Marengo, Centro Nacional de Monitoramento e Alertas de Desastres Naturais (CEMADEN), BrazilReviewed by:
Sara Hughes, University of Michigan, United StatesCopyright © 2021 de Sherbinin, Bowser, Chuang, Cooper, Danielsen, Edmunds, Elias, Faustman, Hultquist, Mondardini, Popescu, Shonowo and Sivakumar. This is an open-access article distributed under the terms of the Creative Commons Attribution License (CC BY). The use, distribution or reproduction in other forums is permitted, provided the original author(s) and the copyright owner(s) are credited and that the original publication in this journal is cited, in accordance with accepted academic practice. No use, distribution or reproduction is permitted which does not comply with these terms.
*Correspondence: Alex de Sherbinin, YWRlc2hlcmJpbmluQGNpZXNpbi5jb2x1bWJpYS5lZHU=
Disclaimer: All claims expressed in this article are solely those of the authors and do not necessarily represent those of their affiliated organizations, or those of the publisher, the editors and the reviewers. Any product that may be evaluated in this article or claim that may be made by its manufacturer is not guaranteed or endorsed by the publisher.
Research integrity at Frontiers
Learn more about the work of our research integrity team to safeguard the quality of each article we publish.