- 1Graduate School of Engineering, Kyoto University, Kyoto, Japan
- 2Disaster Prevention Research Institute, Kyoto University, Kyoto, Japan
Projecting the sea level rise (SLR), storm surges, and related inundation in the Pacific Islands due to climate change is important for assessing the impact of climate change on coastal regions as well as the adaptation of the coastal regions. The compounding effects of storm surges and SLR are one of the major causes of flooding and extreme events; however, a quantitative impact assessment that considers the topographical features of the island has not been properly conducted.
Therefore, this study projects the impact of storm surge and SLR due to climate change on Viti Levu, which is the biggest and most populous island in Fiji. The impact of SLR on the inundation in coastal areas was simulated using a dynamic model based on the IPCC SROCC scenarios and the 1/100 years return period storm surge implemented based on the RCP8.5 equivalent scenario. The affected inundation area and population due to storm surges and SLRs are discussed based on the compound effects of SLR and storm surge.
Although the contribution of SLR to the inundation area was quite significant, the 1/100 year storm surge increased by 10 to 50% of the inundation area. In addition, a narrow and shallow bay with a flat land area had the largest impact of storm surge inundation. Furthermore, the western wind direction had the most severe storm surge inundation and related population exposure due to the topographic and bathymetric characteristics of Viti Levu Island.
Introduction
Since Broecker first projected a rise in temperature due to global warming in 1975, the climate change impact on human activities has received increased interest in various fields (Broecker, 1975). For example, recently, the impact of anthropogenic climate change has received political and diplomatic interest. The United Nations has set “taking urgent action to combat climate change and its impacts” as one of its sustainable development goals (SDGs) for the year 2030 United Nations (2015), naming climate change as the biggest potential threat to the global economy during the World Economic Forum (2016). Additionally, environmental risks such as “failure of climate-change mitigation and adaptation” and “extreme events” are perceived as the biggest sources of concern, according to the newest Global Risks Perception Survey (GRPS), compiled by the Global Risks Report 2019 (World Economic Forum, 2019).
The effects of climate change on society are varied and not limited to the economy. For example, one of the repercussions of climate change-induced sea level rise (SLR) on the society is involuntary migration or displacement of the inhabitants. In addition, other climatic drivers may also serve as natural hazards. The areas most affected by natural hazards due to climate change-induced SLR include the low-lying coastal regions. Meanwhile, 10% of the world's population lives in low-lying coastal regions within a 10 m elevation of the present sea level (Carrasco et al., 2016). Muis et al. (2016) estimated that 1.3% of the world's population is at risk of coastal flooding. Particularly, in Oceania, where the SLR is estimated to have the greatest relative impact on the land area and GDP, as well as in urban areas, agricultural land, and wetlands, where the relative impact of SLR is the second highest under the least severe SLR scenario (Dasgupta et al., 2009).
The most recent data from the 5th Assessment Report (AR5) predicted an SLR of 43–84 cm with a 5% range confidence interval by the end of this century under the highest greenhouse gas concentration scenario, which will, in turn, pose a serious threat to coastlines and several islands (IPCC, 2014). The effect of SLR on small islands can be especially severe since coastal zones often harbor a significant proportion of the human population and infrastructure; in particular, the atoll islands are the most vulnerable because of a lack of resilience caused by limited relocation opportunities further inland (Woodroffe, 2008; Nurse et al., 2014). The situation is further aggravated in the tropical Western Pacific (~12 mm/yr between 1993 and 2009 have been reported) where the SLR is not uniform and is four times above the average, and remains at least 1 mm higher throughout the South Pacific region (Fasullo and Nerem, 2018), which is known for its numerous inhabited islands (Meyssignac et al., 2012; Nurse et al., 2014). To accurately evaluate the SLR impact, several studies have reported the importance of applying a synergetic approach, wherein the coastal morphology, as well as the complex interactions with the ecological environments are considered (Passeri et al., 2015; Hagen et al., 2017).
In addition to the SLR, extreme events such as storm surges have a significant impact on extreme inundation, particularly its compounding effects with SLR (Little et al., 2015). According to a study on Florida's Big Bend Region, storm surge is enhanced by the shore elevation, as well as other physical characteristics such as coastline angle, wide continental shelf, and basin geology (Hagen and Bacopoulos, 2012). Storm surges are mainly induced by tropical cyclones (TCs) in the Pacific, North Atlantic, and Indian Ocean and cause catastrophic hazards in the coastal region (Mori et al., 2014). Storm surge occurs when water is pushed onshore by wind and can also be caused by the atmospheric pressure gradient (Kim, 2019). The amplitude of the storm surge depends on the orientation of the storm track in relation to the coastline, intensity, size, wind speed, and bathymetric features [National Oceanic Atmospheric Administration (NOAA), 2020]. The impact of climate change on TC is expected to increase in intensity, but to decrease in frequency (IPCC AR5). The increase in the intensity of TC causes an increase in the storm surge height, thus resulting in severe inundation. Bilskie et al. (2019) estimated the 500-year return period of extreme events near the northern Gulf of Mexico to be a 100-year return period by the year 2100. Studies evaluating the impact of climate change on storm surges typically use three methodologies: the global climate model (GCM) simulation (direct storm surge simulation based on wind fields and sea-level pressure of GCM output) (Yasuda et al., 2010, 2014; Yang et al., 2018; Mori et al., 2019); the pseudo-global warming technique (Takayabu et al., 2015; Ninomiya et al., 2016); and the statistical modeling, which is based on a synthetic tropical cyclone model (McInnes et al., 2014; Nakajo et al., 2014). Although the importance of the impact assessment of TC and storm surge has been discussed (e.g., Mori and Takemi, 2016), a regional impact assessment combining SLR and storm surge has not yet been achieved. Here, using Fiji as an example, we assessed the impact of SLR and storm surge on coastal inundation.
Fiji is the largest island nation in the Pacific Islands after Australia and New Zealand, however, it has its vulnerabilities such as relatively higher population density in urbanized areas, as well as in low-lying regions, some of which are inhabited or serve as economic hubs. Furthermore, the island can be severely impacted by cyclones and other weather-related events. For example, as many as 76,000 inhabitants were internally displaced during Tropical Cyclone Winston, which struck the country in February 2016. In addition, 40,000 houses were damaged or destroyed with over 350,000 people significantly affected, wherein a significant part of them were living in the northern part of Viti Levu [World Meteorological Organization (WMO), 2016]. The Internal Displacement Monitoring Center (DMC) estimates that 3,614 Fiji inhabitants are annually displaced due to storm surges and another 2,076 due to cyclonic winds (Internal Displacement Monitoring Center (IDMC), 2020). The most recent tropical cyclones: Cyclone Mona and Cyclone Sarai, which hit Fiji on January 2, 2019, and December 12, 2019, respectively, internally displaced a total of 4,800 people (Internal Displacement Monitoring Center, 2020). Several studies have discussed the impact of SLR and storm surges on Fiji. For example, McInnes et al. (2014) researched the impact of TC on the coastal areas of Viti Levu and Vanua Levu islands using the Monte Carlo method, based on historical cyclone occurrences. They found that storm tide risk is higher on the northwest coasts of both islands, while the southwestern coasts of Viti Levu may have been affected during the El Niño and La Niña years. Meanwhile, the study conducted by Gravelle and Mimura (2008) on the vulnerability of the coastal areas of Fiji to SLR identified Suva, Lautoka, and Nadi as being among the most vulnerable areas in Fiji, while the villages in the north, Tavua, and Nailaga are vulnerable due to the lack of adaptive measures. In addition, they found that in many cases, retreat from the affected areas is more realistic than building protection structures or applying other related methods. However, there are no studies on the compound effect of SLR and storm surges on coastal areas.
Therefore, the objective of this study is to estimate the total inundation and affected population due to SLR and storm surge on an island under different future climate change scenarios and extreme storm surge levels. Viti Levu, which is a major island in Fiji was selected as the case study. First, a numerical model was developed to estimate the inundation area due to SLR and storm surge using topography data, atmospheric forcing, and population dataset. Second, a series of numerical simulations was conducted by combining two SLR changes and extreme wind forcing for storm surges. Finally, the compound effects of SLR and storm surge on the inundation extent are discussed and the extent of population to be affected based on exposure in vulnerable areas was estimated.
Materials and Methods
The section describes the methods developed in this study for assessing the impact of storm surge and SLR on inundation. The numerical modeling of storm surges was performed using linear shallow water equations (SWE) with spherical coordinates. Both SLR and storm surge were simulated for the inundation area. The comprehensive dataset including the topography, bathymetry, and population density was set up by combining different sources coordinating grid size. Finally, the population dataset was integrated into the comprehensive dataset for the social impact assessment.
Numerical Modeling of the Storm Surge and SLR Inundation
This study is aimed at estimating the inundation area due to storm surge, SLR, and their compound effects. Therefore, the dynamic modeling of inundation is necessary. However, since a wide area of computation is required, a model with a low computational load is necessary. Thus, inundation was simulated using in-house SWE with a spherical coordinate system in θ and φ, latitude, and longitude. The numerical model was validated using the analytical solution for ideal bathymetry. The governing equations for the free surface η(θ, φ) and momentum flux P and Q in the latitudinal and longitudinal directions are as follows:
where t is the time, h is the water depth, D is the total depth (D = η+h), P is the atmospheric pressure, R is the Earth radius, f is the Coriolis force, ρw is the seawater density (1,025 kg/m3), and g is the gravitational acceleration (9.8 m/s2). The terms τs and τb in Equation (2, 3) are the surface stress and bottom stress along the directions of the θ and φ coordinates, respectively. The origin of the free surface η is located at the mean water level and toward the upward level.
The surface wind stress, τs, is calculated using the standard quadratic law of wind speed:
where CD is the wind drag coefficient, ρa is the air density (1.225 kg/m3), and U is the wind speed at 10 m elevation (subscript i indicates latitudinal or longitudinal component). The surface drag coefficient CD given by Honda and Mitsuyasu (1980) is:
The bottom stress, τb, represents the bottom friction and is expressed by a quadratic law, as shown in Equation (6).
where k is the bottom friction coefficient and is typically k = 0.025 for the sea bottom (Flather and Tippett, 1984). The governing equations are discretized by the standard explicit first-order upwind scheme.
The open boundary condition offshore is given by the Flather type equation (Flather and Tippett, 1984)
where c = , and is the long-wave velocity, qn is the discretized depth-averaged velocity at grid n. Assuming long wave propagation outward, Equation (7) gives a non-reflected boundary condition. The inundation due to storm surges was considered using the moving boundary scheme (e.g., Liu et al., 1995; Kotani et al., 1998). Figure 1 illustrates a schematic view of the inundation scheme. The total depth Dn+1 at grid n+1 for calculating Pn+1 is given by:
where the surface elevation ηn+1 in the land includes the ground level at grid n, ηn in the ocean is defined from the mean water level at grid n, and hn+1 is bathymetry at grid n + 1 (inland area hn+1 < 0). There are two cases when the grid point n is located in the inundated area. Pn+1 is calculated using (2) if Dn+1 > 0 (grid n + 1 becomes newly inundated as shown in Figure 1A) and Pn+1 is zero if Dn+1 ≤ 0 (grid n + 1 is not inundated as in Figure 1B). The minimum total water depth hmin for the calculation of friction terms is 0.03 m in this model.
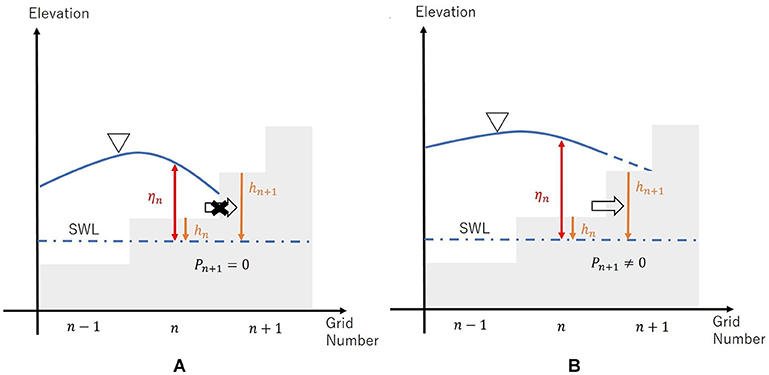
Figure 1. Schematic view of the inundation scheme [(A) the case grid n + 1 is not inundated, (B) the case grid n + 1 becomes inundated].
Figure 2 shows the schematic illustration of the inundation modeling used in this study. To simulate changes in the inundation edge/area, we considered the SLR, storm surge, as well as their compound effects with storm surges. The inundation due to storm surges was considered by the numerical model due to dynamic momentum and mass transfer of sea surface to land. The inundation area can be estimated using the altitude without considering the houses and other small structures. The accuracy of the numerical model was verified using an ideal uniform slope condition with changing slopes and wind speeds. The error of maximum storm surge height ranged from 5.3 to 5.9% for most cases.
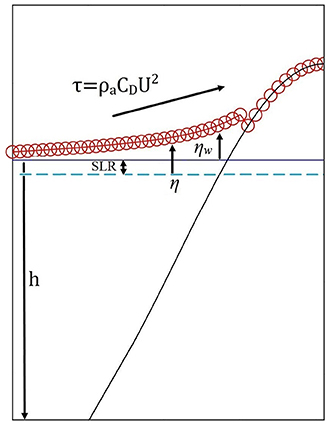
Figure 2. Schematic cross-sectional view of the coastal inundation simulation considering SLR and storm surge, where ηw is the inundation due to storm surge and η is the cumulative inundation due to storm surge and SLR.
Model Configuration and Dataset
Computational Setup
The domain size of the numerical simulation ranged from 18°24′ S to −17°8′30″ S in the latitudinal direction and 176°56′ W to 178°48′ W in the longitudinal direction. The total modeled area was approximately 28,000 km2. The computation domain includes the Viti Levu Island (10,388 km2), which is a major island in Fiji, as well as numerous smaller islands, including Ovalau (106.4 km2).
The spatial resolution of the computation was 3 arc-s (~90 m) and the details of bathymetry, topography, and forcing data are explained in the following section. The temporal discretization was 1 s and integrates 1 h for each forcing condition. Herein, we assessed the impact of SLR and maximum storm surge height on the inundation extent in Fiji. The effects of SLR were implemented as changes in the mean water level in the numerical model, and the storm surge was considered based on the dynamic model described in Section Numerical modeling of the storm surge and SLR inundation. Since the inundation process requires a dynamic model, the compound effects of SLR and storm surge on inundation were considered by changing the combination of the two phenomena using the numerical model. The combined effect of SLR using two different representative concentration pathways (RCP) scenarios and storm surges were examined by changing the wind directions. The details of the wind forcing and SLR scenarios are discussed in the following section.
Bathymetry and Topography Data
The topography and bathymetry data of the target region were compiled using the NASA's Shuttle Radar Topography Mission (SRTM) and General Bathymetric Chart of the Oceans (GEBCO) databases, respectively. The SRTM digital elevation model (DEM) provides high-resolution topography data (3 arc-s) used for land elevation data input (Jarvis et al., 2008). GEBCO, a global bathymetric grid with 30 arc-s spacing is used for ocean bathymetry data input (Becker et al., 2009). Since the two datasets have different grid coordinates and spatial resolutions, both bathymetric and topographic data were linearly interpolated and merged into the same grid with 3-arcs resolution for the computation. Subsequently, a global self-consistent hierarchical high-resolution geography (GSHHG) database was utilized for the shoreline validation.
Figure 3A shows the combined bathymetry and topography data for the Fiji area, while Figure 3B illustrates the topography data with a focus on the low-lying area of Fiji, lower than 20 m. Despite being mountainous (86.9% of the territory is located higher than 20 m above sea level), prominent low-lying areas are also present (3.3% of the island is lower than 5 m above sea level, Figure 3B). The areas in the south-eastern, southern, western, north-western, and northern parts all have a significant portion of areas below 5 m (Figure 3B). As discussed later in the text, these areas are usually more densely populated. Coastal areas are also economically significant for the country, as some of them serve as tourist beaches or resort locations. Six target locations in the low-lying area were selected for detailed analysis, as shown in the boxes in Figure 3A.
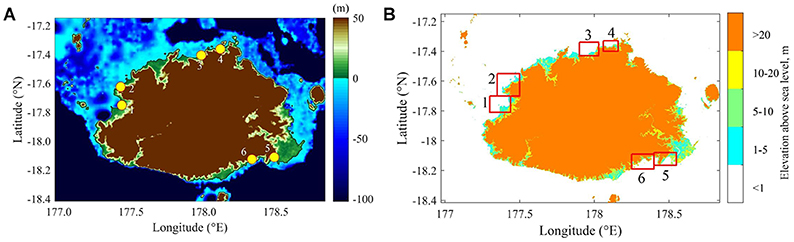
Figure 3. Computational domain, bathymetry, and topography with the selected low-lying locations [contour indicates elevation (m), (A)] and the map indicating altitude changes in Viti Levu (B).
Forcing for the Numerical Model
For the impact assessment of inundation by storm surge and SLR, we considered both effects for the numerical modeling. Extreme wind input is necessary for the storm surge modeling of Fiji, however, there are only few available historical data on forcing. Atmospheric analysis such as Climate Forecast System Reanalysis (CFSR) or European Center for Medium-Range Weather Forecasts Re-Analysis (ERA) product can be used for estimating extreme TCs, however, the available reanalysis data is too short and less accurate for the TC events and related storm surges (e.g., Mori and Takemi, 2016). In contrast, synthetic TC models used to increase the number of TC events have been used for the estimation of extreme TCs and storm surges (e.g., McInnes et al., 2014; Nakajo et al., 2014). These statistical approaches are straightforward approaches used to estimate the intensity and probability of extreme storm surges. However, the computational cost is quite high due to the large number of computations (e.g., 10,000 years simulation). Moreover, the estimation of TCs on small islands can be regarded as random processes. Thus, to estimate the maximum storm surge heights for a given return period, we assumed that constant strong winds blow over the island. This approach can estimate the hot spot of the vulnerable areas of the island, however, it neglects the inhomogeneous distribution of the wind fields of TC. Additionally, we used extreme wind speeds based on climate projection as follows.
We used the extreme sea surface wind speed data of mega ensemble projection by atmospheric GCM with 60 km resolution to estimate the extreme storm surge along the coasts of Fiji. The mega ensemble climate projections, (so-called d4PDF) (Mizuta et al., 2017) is a set of long-term ensemble projections for historical and warmer future climate conditions for a period of over 5,000 years. The projections assume a +4K global mean atmospheric temperature compared to the pre-industrial climate. The +4K climate corresponds to the temperature change under the RCP8.5 scenario by the end of the 21st century. Mori et al. (2019) estimated the future changes in the wind speed and storm surge for a 100-year return period using the d4PDF. The projections for the historical climate and +4K future climate integrated 6,000 and 5,400 years, respectively, in the d4PDF. Therefore, extreme events (e.g., wind speed in a 100-year return value) can be used to easily estimate non-parametric analysis without standard extreme value analysis.
The estimated weakening of TCs, and a consequent decrease in the wind speed and storm surge heights in the future in the Southern hemisphere, including Fiji, was discussed by Mori et al. (2019). In this study, we used the same dataset around Fiji, as shown in Figure 5. The wind speed in a 100-year return value around Viti Levu was estimated at 38.7 and 45.6 m/s for the +4K future and historical climate condition, respectively. The decreasing wind speed in the future climate can be attributed to the weaker TC intensity in the Southern hemisphere (Mori et al., 2019). Despite the weakening trend of the wind speed, the storm speed around Fiji remains the mightiest in the region for extreme wind speeds in the Southern Pacific, with the most prominent ones located in the Coral Sea (latitudes 20° to 15° S, and longitudes 150° to 160° E), followed by New Caledonia and parts of Vanuatu (Figure 4). Since this study focuses on estimations of future climate, the corresponding value is used throughout the study.
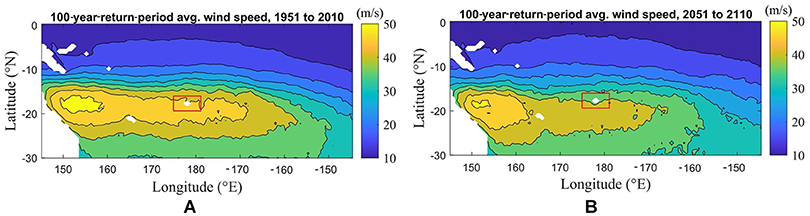
Figure 4. Extreme wind speed at 100-year-return-period around Fiji, according to historical climate (A) and the warmer future climate (B) according to d4PDF climate projection (unit: m/s).
To estimate the impact of the storm surge on the coastal areas of Fiji, the TC characteristics should be considered for the storm surge modeling. However, it is difficult to implement variability for an island due to the TC's track, translation speed, and other factors. Therefore, for simplicity, we assumed that spatially uniform wind was blowing from all four cardinal directions with the same probability.
In this study, we considered the SLR and storm surge. The SLR was implemented using the global SLR projections based on Special Report on the Ocean and Cryosphere in a Changing Climate (SROCC, 2019) by the end of the Twenty-first century. The Intergovernmental Panel on Climate Change (IPCC) AR5 summarizes the relationship between the RCPs and SLR. There are several RCP scenarios, however, we selected two typical scenarios for this analysis. First, the RCP2.6, which relates to the Paris agreement for 2-degree target in mitigation. In addition, the RCP8.5, which reflects for the worst-case scenario. The future rise in global mean sea level (GMSL) differs depending on the different radiative forcing from RCP2.6 to RCP8.5. As discussed in Chapter 4 of IPCC SROCC, the estimated sea level by the year 2,100 is 0.43 m under the RCP2.6 scenario and 0.84 m under the RCP8.5 scenario under medium confidence, compared to the years 1986–2005 (Table 1). In addition, according to the aforementioned report (Oppenheimer et al., 2019), there is a certain likelihood of a rise in the GMSL and an acceleration in the high confidence. For example, for the RCP8.5 scenario, the annual rate of SLR is predicted to be 15 mm/year by 2100. Meanwhile, there is high confidence that the dominant cause of GMSL since 1970 is anthropogenic forcing. In addition, anthropogenic drivers are not limited to the climatic drivers and may include demographic and urbanization trends. There is very high confidence that such drivers have played a significant role in negatively affecting the exposure and vulnerability of low-lying coastal communities to SLR and storm surge. In addition, there is high confidence that anthropogenic coastline modifications can outpace the SLR effects. There is a wide range of regional variability in the SLR speed, reaching up to 30%. Despite regional differences, the globally averaged SLR values were applied in this study, as there is no regional SLR projection available for the target region. To analyze the differences between the sea surface elevation depending on the SLR in detail, six low-lying territories in inhabited areas were selected (Figure 2).
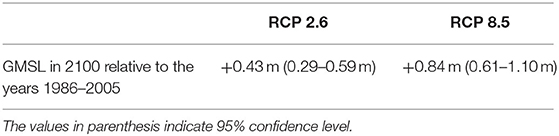
Table 1. GMSL rise estimates depending on different RCP scenarios according to the Special Report on the Ocean and cryosphere in a changing climate (Oppenheimer et al., 2019).
These areas are located in territories that are prone to the combined effect of storm surge and SLR. The largest settlements are located in the southeastern and western parts of the island. Consequently, two locations in the most urbanized and low-lying areas in Nadi (location #1 in Figure 3) and Lautoka (location #2) were selected to represent the affected areas on the western coast. The affected areas on the populated southern coast were represented by areas in Suva (location #5) and Na Vasi (location #6). The northern coast does not have any bigger settlements, but has several low-lying areas, which were represented by areas in Draunivi (location #3) and Narewa (location #4). The eastern coast was not analyzed in detail even though it has some low-lying areas, as all populated areas are mostly limited to the capital (Suva) area.
Population Data
The social impact of coastal flooding was estimated using the inundation model. The Gridded Population of the World, Version 4 (GPWv4) data provided by NASA Socioeconomic Data and Applications Center (SEDAC) was used in the model to estimate the impact of SLR and storm surge on the population [Center for International Earth Science Information Network (CIESIN), 2018]. The GPWv4 data models the distribution of the human population in most countries in the world, including Fiji. The GPWv4 is gridded with an output resolution of 30 arcs, or ~1 km at the equator. These models have been used for vulnerability mapping, health dimensions of environmental change, and disaster impacts (Doxsey-Whitfield et al., 2015). The precision of the international boundaries of census data sets was compared against the Global Administrative Areas version 2 (GADMv2). The GPWv4 used in this study is a raster data collection, which includes the population data from the national population and housing censuses conducted between 2005 and 2014. The dataset used for Fiji population estimates was based on the Final Census conducted in 2007 by Fiji Islands Bureau and Statistics, 2008, with the national estimates adjusted to the United Nations World Population Prospects to avoid potential overreporting or underreporting (United Nations, 2013).
The population dataset of GPWv4 was interpolated linearly to the same grid system as the topography dataset, from 30 arcs to 3 arcs, as described in section Bathymetry and topography data. The population distribution was then compared to the topography and inundation maps in general, as well as to those in the selected areas. This enabled the direct estimation of the affected population numbers after flagging. Conclusions were made based on the vulnerability of Viti Levu Island. Since the spatial interpolation from 30 arcs to 3 arcs gives large biases, we paid attention to the uncertainties of the analysis for the vulnerability results. The population numbers acquired from GPWv4 enabled distinguishing the principal tendencies of the population distribution on Viti Levu Island. The population density in Viti Levu (58.8 people/km2) slightly exceeds the national average (46.4 people/km2). However, despite the relatively low population density, there are areas where the population density is higher than 200 people/km2 and may exceed 5,000 people/km2. Such areas are prevalent in the western, northwestern, and southeastern parts of the island, all of which are predominantly located in coastal zones (Figure 5). The highest population density was observed in the southeastern part of the island, which is home to the capital city of Fiji.
Results and Discussions
Individual SLR and Storm Surge Contribution to Inundation
First, we examined the permanent inundation due to SLR by submerging the bathymetry and topography data. According to the RCP2.6 scenario, the SLR alone would cause an inundation of 20.5 km2, or 0.2% of the total land area, while the RCP8.5 estimated that the inundation area would be approximately twice as large at 39.9 km2 (or 0.38%) of the total land area. Figure 6 shows an example of the inundation change by SLR estimated by RCP2.6 and 8.5 in location #2 (see its location in Figure 3) in the northwestern part of Viti Levu. Changes in the coastline in location #2 (Lautoka area), as shown in Figure 6, indicate that a higher SLR induces larger permanent inundation; however, the affected area is highly dependent on the local topographical and bathymetrical features. The details of local inundation characteristics are discussed later in the manuscript.
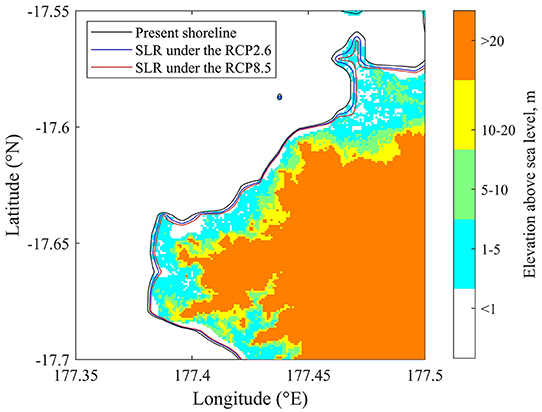
Figure 6. Changes in the shoreline by SLR in location #2, Lautoka area, (see Figure 3B) under different SLR scenarios. The black line corresponds to the coastline under present climate condition, the blue line corresponds to the retreat of coastline due to SLR under the RCP2.6 scenario, while the red line corresponds to the shift in the shoreline due to SLR under the RCP8.5 scenario.
The storm surge heights greatly differ depending on the wind speed. To estimate the maximum inundation, we changed the wind directions over the island. Figure 7 depicts a combined map for the maximum storm surge height in the simulations with four different wind directions. Owing to bathymetric and topographic features, the spatial distribution of the surface elevation was considerably smaller in the southern part of the island. However, extreme storm surges were estimated to be as high as 2.9 m on the eastern and northern coasts, while the storm surges were significantly more moderate in the southern part of the island, with a maximum estimated value of 1.42 m in the Suva area with an exception of the Vunanu area. In addition, a significant difference was observed in the total area (i.e., the marine and coastal areas where a certain sea surface elevation anomaly was observed). In all the modeled areas, for the southern wind, the total area where the sea surface was elevated above 1 m was merely 4.4 km2; however, the total area was as large as 93.42 km2 for the eastern wind, and as much as 184.55 km2 for the western wind. In addition, all the wind directions except the southern direction were capable of generating surface elevation higher than 2 m. Furthermore, 2.69 km2 of the total area had a sea surface elevation exceeding 2 m under the western wind, 1.07 km2 under the eastern wind, and 0.54 km2 under the northern wind. Differences in storm surge height for Viti Levu was due to the differences in the bathymetry and coastal morphological characteristics. The ocean area south of Fiji is noted for its depth, while the coast is either very steep or barely elevated, thus preventing a higher storm surge height for extreme events. In contrast, the northern and western coasts are characterized mostly by shallow waters and either very less elevated or 1–2 m elevated coasts, thus, generating extreme storm surges.
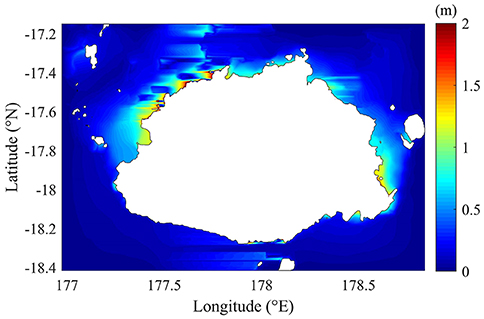
Figure 7. Spatial distribution of the maximum storm surge level combining different wind directions (unit: m above MWL).
It is important to note that the storm surge alone would lead to 7.29 km2 (0.07%)–28.55 km2 (0.28%) of inundated areas, depending on the wind direction. In addition, an average of 17.95 km2 of the territory is going to be inundated due to the storm surge alone, with the western wind resulting in the largest inundations and the southern wind resulting in the smallest ones.
Compound Effects of SLR and Storm Surge for Inundation
We investigated the compound effects of SLR and storm surge on the total inundation. First, we examined the time series of the sea surface elevation with respect to the future MSL. For this, we selected the wind direction that caused the highest storm surge for each location. Figure 8 shows an example of the time series of η at selected locations for the individual effect of the storm surge and those with the addition of the SLR for the RCP2.6 and RCP8.5 scenarios. As shown in Figure 8, the SLR decreased the time needed for coastal inundation and resulted in an earlier arrival of the peak surge. This is consistent with the results of Bilskie et al. (2016). In addition, relatively steep coasts (1 m or higher) offered favorable conditions for high storm surge-related surface elevation, as observed in Locations #2, #3, and #5 (see Figure 3) with maximum surface elevation of 1.67, 1.81, and 1.31 m, respectively. Meanwhile, locations #1, #4, and #6 exhibited more modest surface elevation values (i.e., 1.22, 1.07, and 1.06 m, respectively). The bathymetric configuration significantly affected the maximum surface elevation in locations #2 and #3. Depending on the location, the maximum elevation value was attained after 1,000–2,450 s. The maximum sea surface elevation for location #2 was achieved after 2,450 s, while that for location #6 with no simulated SLR (storm surge only) was achieved after 1,000 s. In addition, the saturation period was longer for the locations with no simulated SLR and for the locations with a larger height above sea level. The highest surface elevation values were observed in a model with no simulated SLR. Storm surges as high as 1.81 m were achieved for location #3 under the northern wind, followed by 1.67 m for location #2 under the western wind. The maximum estimated surface height for the other locations are as follows: 1.22 m for location #1, 1.07 m for location #4, 1.31 m for location #5, and 1.06 m for location #6. In comparison to the mean sea level, the estimated sea surface elevation was 8–22% lower for the SLR occurring under the RCP8.5 scenario compared to models with no SLR simulation. Only location #1 recorded almost no difference regardless of the SLR scenario.
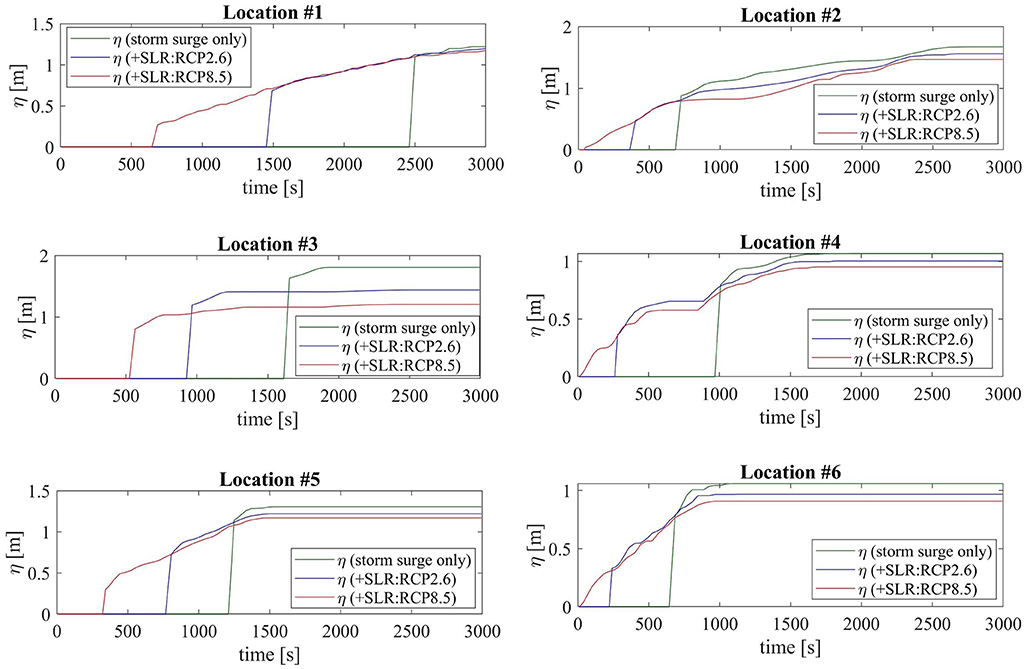
Figure 8. Time series of surface elevation due to storm surge with and without SLR in selected locations. The green line corresponds to the maximum sea surface elevation above MSL in the case of storm surge alone. The blue line corresponds to the maximum sea surface elevation (above the future MSL) in the case of storm surge+RCP2.6 scenario. The red line corresponds to the maximum sea surface elevation (above the future MSL) in the case of storm surge+RCP8.5 scenario.
The compound effects of SLR and storm surge play a significant role in sea surface elevation. The inundated area was examined for all wind directions. The most devastating results for Viti Levu were observed for the western winds (88.53 km2 of the inundated area), followed by the eastern, northern, and southern winds, wherein the estimated inundated area was 7.3, 42.8, and 79.16 km2, respectively (out of the total area of 10,388 km2). As expected, the narrow and shallow bay with a flat land area had the largest impact on the storm surge inundation.
In comparison, the northern part of Fiji showed significantly more inundated areas due to SLR, particularly under the western wind, as shown in Figure 9. It is important to note that low-lying areas, as well as shallow bathymetry are prevalent in these areas. In addition, it is important to note that some of the observed inundated areas are low-lying uninhabited small islets and rocks that are present in the bathymetry but were excluded from the topography dataset. Some of these areas are rocks separated from the mainland islands. Regardless, a relatively significant part of the Lautoka area was vulnerable to the individual effect of the SLR, including the northeastern part of Lautoka city and Vitogo Bay. Location #2 is noted for its low-lying coastline, which mostly lies below 1 m above mean sea level (AMSL). The elevation of a large part of the urbanized Lautoka area did not exceed 3 m AMSL. Therefore, SLR plays an important role in the compound surface elevation in such areas. In contrast, the SLR effect was almost negligible in the southern coast, wherein the storm surge effect was more significant, particularly for the Vunanu area under the eastern wind. In addition, the changes in the inundated area were minimal for the southern coast irrespective of the direction of the wind. Furthermore, most parts on the southern coast and the southwestern shore in particular, are noted for steep cliffs, thus making them less prone to the hazards of extreme events.
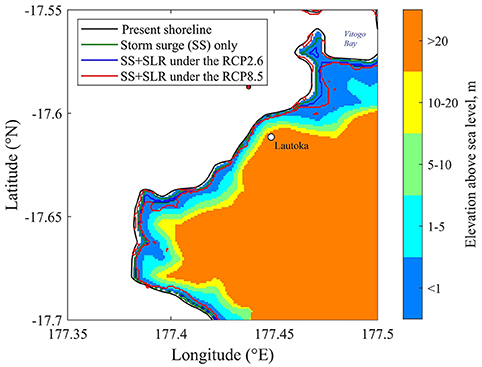
Figure 9. Inundated area due to the compound effects in location #2, Lautoka area, (see Figure 3B). The black line corresponds to the coastline under present climate condition, the green line corresponds to storm surge inundation only, the blue and red lines correspond to the maximum inundation edge due to storm surge with SLR under the RCP2.6 and RCP8.5 scenarios, respectively.
The compound effects by the SLR and storm surge changed the total inundated area of the island, however, the individual effect of these factors was relatively mild. As discussed in the previous section, the SLR inundated 20.5–39.9 km2 of the coastal territory, whereas the total area of the island is 10,390 km2. In contrast, without the SLR, the storm surge inundated only an average of 17.9 km2.
Figure 10 summarizes the compound effects of SLR and storm surge on the total inundation of Fiji. For the RCP8.5 scenario, the average compound effect was 82.99 km2 of the inundated area, with relatively minor differences depending on the wind direction (79.16–88.53 km2). Depending on the wind direction, the SLR contribution to the cumulative inundation was 45–50% (Figure 10). For the RCP2.6 scenario, the average compound effect was 48.27 km2 of the inundated area, with the analogical differences depending on the wind direction (42.84–57.97 km2). Depending on the wind direction, the SLR impact is reduced, since it contributes 35–47% to the cumulative effect. SLR is an important factor for the inundation of the northern areas. The individual effect of SLR on the inundated area is more significant than that of the storm surge when a different approach is used. When the storm surge scenario is used as a baseline, and the additional surface elevation levels from the RCP2.6 and RCP8.5 scenarios are assumed to be attributed to the SLR, the storm surge contribution to the compound effect becomes smaller. Under this interpretation, the storm surge alone contributes an average of 36% to the surface elevation (17-49%) when the SLR scenario is based on the RCP2.6, but only an average of 22% to the surface elevation (9–32%) when the SLR is based on the RCP8.5 scenario.
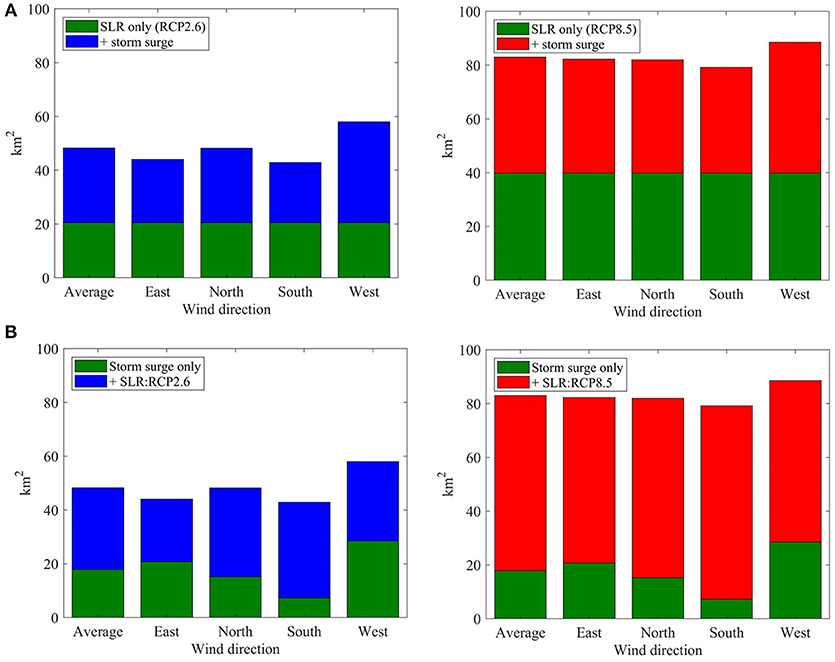
Figure 10. Compound effects on the total inundation area by SLR and storm surge (left: RCP2.6 scenario, right: RCP8.5 scenario). (A) Contribution of storm surge to total inundation area, (B) Contribution of SLR to total inundation area.
From the results, it can be concluded that the relationship between the inundation scope and SLR is not linear. This finding is similar to those of Bilskie et al. (2014) for the study on Mississippi and Alabama.
The significance of the SLR on the total inundation was confirmed by comparing the inundation depth in the selected locations under no SLR, RCP2.6, and RCP8.5 scenarios (1–6), as shown in Figure 11. The largest inundation was observed in location #2 under the RCP8.5 scenario, which was estimated to be submerged up to 1.42 m due to the compound effect. Other locations submerged above 1 m include location #1 (1.22 m), location #6 (1.05 m), and location #4 (1.00 m). Meanwhile, all observed territories were submerged below 0.8 m without SLR simulations, with the most significant inundation occurring in location #2 (0.79 m).
Exposure: Population
In this section, the population affected by the total inundation discussed in the previous section is discussed with and without the storm surge effects. Figure 12 shows the relationship between the population and the inundated area due to the storm surge and SLR. Despite the very long shoreline, the number of people estimated to be directly affected (i.e., would have their homes inundated) is limited. For the Viti Levu Island, SLR did not pose a significant threat to the island population. The total population estimated to be directly affected by the effects of SLR and storm surge was an average of 5,430 people. However, the indirectly affected population can be speculated to be significantly larger. In addition, the retreat of the coast in location #2 may presumably affect the local population more significantly than in other areas due to the lower altitude of the areas around the shore, as well as the population density.
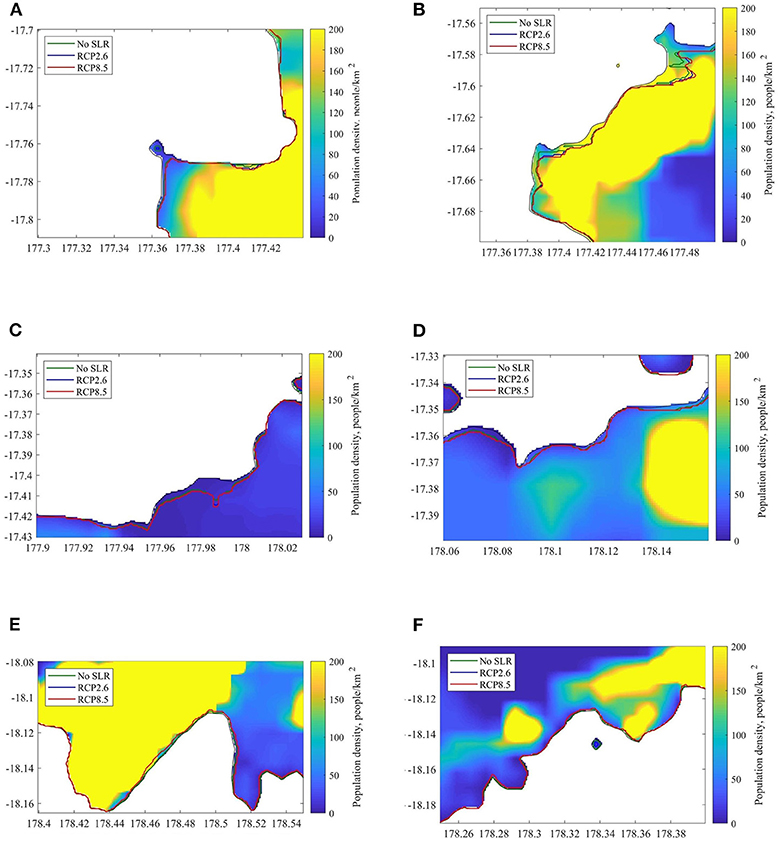
Figure 12. Relationship between the population density and inundation area in the selected 6 locations. The area of the 6 locations are shown in Figure 3B (unit: a number of people/km2). (A) Location #1, (B) Location #2, (C) Location #3, (D) Location #4, (E) Location #5, and (F) Location #6.
The most potentially vulnerable areas for the population to the impact of SLR and storm surge are located on the western side of the island. Not only were these areas estimated to have the widest inundation scale, as shown in Figure 10B, but they are also known for a higher population density in locations #1 and #2 (Figure 12). This finding is consistent with that of McInnes et al. (2014), in which the northwestern part of the island was identified to possess the highest storm tide risk. The examined locations #1 and #2 have an estimated population of 23,080 and 70,700, respectively, out of which 430 and 3,060 people, were estimated to be directly affected by inundations, respectively. Despite not being subject to inundations as wide as that on the northern side, the capital Suva area is known to have the highest population density on the island, and was denoted as location #5. Approximately, 179,230 and 11,460 people inhabit the 5th and 6th locations, respectively, while the territories inhabited by 730 and 150 people, respectively, were estimated to be inundated. Meanwhile, the northern part of Fiji is known for having smaller settlements, and the vulnerability of the directly exposed population due to hazards is low. The 3rd and 4th selected locations have an estimated population of 660 and 5,990 inhabitants, respectively, and the directly exposed population accounted for 115 people altogether.
While the estimated directly affected population number is 5,430, it is worth noting that the urbanized territory is located very close to the potentially inundated area (Figure 12). In addition, the population living in proximity to the inundated area is ~70,700. Since in certain cases, the inhabitants may become displaced due to loss of jobs when the coastal infrastructure is destroyed, it is important to consider this in further studies. Such inhabitants may have to flee even if their houses are not flooded or damaged after an extreme event. For example, the inhabitants whose income relies on the infrastructure near the coastline or where the coastal areas are extensively used such as in the agricultural sector, the shore recession and consequent soil degradation and salinization may lead to permanent displacement. In addition, some displaced people may return to their locations afterwards, while some displacement may be permanent. People who are forced to flee their home and are displaced either internally or outside their country are occasionally referred to as 'climate refugees' (Berchin et al., 2017). However, a limited number of studies regarding the relationship between climate change and human population displacement have been conducted, as this discourse is sometimes even considered controversial due to a lack of empirical studies (Mortreux and Barnett, 2009; Lilleør and den Broeck, 2011). Several sociological studies conclude that displaced people are subject to physical, economic, and social exclusion (Cernea, 2005), and are forced to abandon their usual ways of life (Berchin et al., 2017) and customs, with the vulnerability and exposure also depending on wealth and education. However, due to numerous complexities, the indirectly exposed population is beyond the scope of this study.
The SLR was confirmed to be a very significant factor for people displacement in all cases in this study. The population displacement numbers were three times higher under the RCP2.6+storm surge model, compared to the model with the storm surge alone. Consequently, the directly affected population numbers were 1.8 times higher in the RCP8.5 model than in the RCP2.6 model. In addition, the biggest difference was observed under the eastern wind. The difference in the number of the directly affected population between the no SLR model and that of the RCP8.5+storm surge differed by ~50 times (Figure 13A). This could be explained by the very scarcely populated eastern coast of the island. Meanwhile, when comparing the selected locations, the difference in the displaced population numbers differed by approximately 1.8 times or more (i.e., close to the island average). The sharpest difference was spotted in the more populated locations #6 (4.4 times) and #5 (2.5 times). In contrast, another urbanized area (location #2) exhibited the smallest increase among all the observed locations (1.6 times, Figure 13B). This can be explained by the differences in tendencies to inhabit coastal areas.
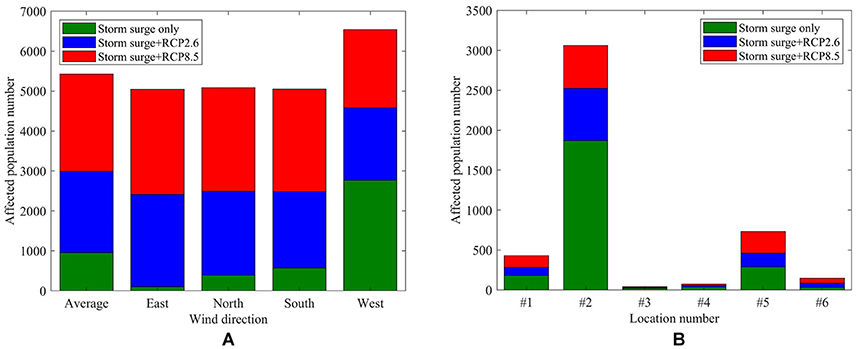
Figure 13. Graphs showing the directly affected population scope trends under different wind directions (A) and in different selected locations (B).
Conclusions
This study presents an impact assessment of coastal inundation by the SLR and storm surge on Viti Levu Island of Fiji. A numerical method was developed for computing the inundation area by the SLR and storm surge. Based on the analysis, the storm surge significantly increased the inundation area (hazard intensity) and the related affected population (exposure) depends on the topography and bathymetry.
The effects of the western wind direction equipped with the SLR effect on the western coast appeared to be the most disastrous due to the topographic and bathymetric characteristics of the island. Furthermore, the effect of the storm surge alone was limited, inundating 17.9 km2 (or 0.17%) of the island territory, whereas the compound effect under the RCP8.5 scenario resulted in 4.7 times higher inundation values for the future climate condition. A narrow and shallow bay with a flat land area had the largest impact on the storm surge inundation. In conclusion, we found that the SLR accelerated the inundation of the island compared to the present climate situation. While the storm surge caused by extreme events is projected to decrease in future climate conditions, the sea level is expected to rise continuously with time. The most potentially vulnerable parts of the island are the western urbanized areas. The settlements on the western side are subjected to the highest storm surges with the largest inundated areas, while the settlements in the south are estimated to experience smaller scales of inundation. The results of the displaced people in the south are not negligible, as it is the most densely populated area on the island. While the inundated areas are estimated to pose a direct threat to a relatively small number of the island population (5,429), Viti Levu Island, and Fiji by extension can still be categorized as vulnerable to extreme events due to the importance of the coastal areas to the country's economy, especially if the SLR intensifies in the future. In contrast, the SLR plays an important role in the displacement, as the estimated directly affected population numbers differ five times between the SLR under RCP8.5 and that of the storm surge only. In summary, this study concludes that depending on the future scenario, the biggest island of Fiji may be vulnerable to the effects of climate change, and understanding the compound effects of SLR and storm surge is necessary for adaptation planning.
For future studies, it is important to include the characteristics of TC wind fields, tracks, and other parameters, although we considered the homogeneous extreme wind speeds blowing over the island. This study used the 1/100 years extreme wind fields based on the d4PDF ensemble dataset. The projection of the extremes by GCMs has a large uncertainty. Further analysis will be required using CMIP6 or other high-resolution projections. A limitation of the proposed model for vulnerability analysis is the use of interpolated data from a coarse 1 km resolution to 100 m scale of the population density data. The precise population data can improve the vulnerability analysis and related displacement estimations in coastal areas. In addition, this model did not consider human behavior when assessing the potentially displaced population, therefore a conservative discourse was followed, in which only people living in the potentially submerged areas are considered to be directly affected. Future studies may need to conduct more detailed research regarding the coastal morphology change due to SLR and storm surge inundation, particularly for smaller low-lying islands.
Data Availability Statement
The original contributions presented in the study are included in the article/supplementary materials, further inquiries can be directed to the corresponding author/s.
Author Contributions
AS: numerical simulation, validation, analysis of the results, preparation of the manuscript, and revisions. NM: supervising, coding of model, revisions, methodology, and the final editing. NF: SWE storm surge model stability and figures related to inundation. TS: input data for the storm surge model and the revision. TM: consultation regarding the coding and visualization of figures. All authors contributed to the article and approved the submitted version.
Funding
AS would like to express utmost gratitude to the Ministry of Education, Culture, Sports, Science, and Technology of Japan (MEXT) for providing with a scholarship. A part of this research is supported by climate change adaptation research programs, National Institute for Environmental Studies of Japan and the Integrated Research Program for Advancing Climate Models (TOUGOU Program) Grant Number JPMXD0717935498 supported by MEXT, Japan.
Conflict of Interest
The authors declare that the research was conducted in the absence of any commercial or financial relationships that could be construed as a potential conflict of interest.
Acknowledgments
The authors are also grateful to Dr. Adrean Webb for contributing to solving the issues regarding the programming in the primary stages of my research, as well as his help with proof-reading.
References
Becker, J. J., Sandwell, D. T., Smith, W. H. F., Braud, J., Binder, B., Depner, J., et al. (2009). Global bathymetry and elevation data at 30 arc seconds resolution: SRTM30_PLUS. Marine Geodesy 32, 355–371. doi: 10.1080/01490410903297766
Berchin, I. I., Valduga, I. B., Garcia, J., and Andrade Guerra, J. B. S. O. (2017). Climate change and forced migrations: an effort towards recognizing climate refugees. Geoforum 84, 147–150. doi: 10.1016/j.geoforum.2017.06.022
Bilskie, M. V., Hagen, S. C., Alizad, K., Medeiros, S. C., Passeri, D. L., Needham, H. F., et al. (2016). Dynamic simulation and numerical analysis of hurricane storm surge under sea level rise with geomorphologic changes along the northern Gulf of Mexico. Earth's Future 4, 177–193. doi: 10.1002/2015EF000347
Bilskie, M. V., Hagen, S. C., and Irish, J. L. (2019). Development of return period stillwater floodplains for the northern gulf of Mexico under the coastal dynamics of sea level rise. J. Waterw. Port Coast. Ocean Eng. 145:04018043. doi: 10.1061/(ASCE)WW.1943-5460.0000468
Bilskie, M. V., Hagen, S. C., Medeiros, S. C., and Passeri, D. L. (2014). Dynamics of sea level rise and coastal flooding on a changing landscape. Geophys. Res. Lett. 41, 927–934. doi: 10.1002/2013GL058759
Broecker, W. (1975). Climatic change: are we on the brink of a pronounced global warming? Science 189, 460–46. doi: 10.1126/science.189.4201.460
Carrasco, A. R., Ferreira, Ó., and Roelvink, D. (2016). Coastal lagoons and rising sea level: a review. Earth Sci. Rev. 154, 356–368. doi: 10.1016/j.earscirev.2015.11.007
Center for International Earth Science Information Network (CIESIN) (2018). Documentation for the Gridded Population of the World, Version 4 (GPWv4), Revision 11 Data Sets. Palisades, NY: NASA Socioeconomic Data and Applications Center (SEDAC), Columbia University.
Cernea, M. M. (2005). 'Restriction of access' is displacement: a broader concept and policy: a broader concept and policy. Force. Migr. Rev. 23, 48–49. Available online at: https://www.refworld.org/docid/50c5db302.html
Dasgupta, S., Laplante, B., Meisner, C., Wheeler, D., and Yan, J. (2009). The impact of sea level rise on developing countries: a comparative analysis. Climat. Change 93, 379–388. doi: 10.1007/s10584-008-9499-5
Doxsey-Whitfield, E., MacManus, K., Adamo, S. B., Pistolesi, L., Squires, J., Borkovska, O., et al. (2015). Taking advantage of the improved availability of census data: a first look at the gridded population of the world, version 4. Papers Appl. Geogr. 1, 226–234. doi: 10.1080/23754931.2015.1014272
Fasullo, J. T., and Nerem, R. S. (2018). Altimeter-era emergence of the patterns of forced sea-level rise in climate models and implications for the future. PNAS 115, 12944–12949. doi: 10.1073/pnas.1813233115
Fiji Islands Bureau and Statistics (2008). Census 2007 Results: Population Size, Growth, Structure and Distribution. Statistical News. Suva: Fiji Islands Bureau and Statistics.
Flather, R. A., and Tippett, L. H. C. (1984). A numerical model investigation of the storm surge of 31 January and 1 February 1953 in the North Sea. Q. J. Roy. Meteor. Soc. 110, 591–612. doi: 10.1002/qj.49711046503
Gravelle, G., and Mimura, N. (2008). Vulnerability assessment of sea-level rise in Viti Levu, Fiji Islands. Sustain Sci. 3, 171–180. doi: 10.1007/s11625-008-0052-2
Hagen, S.C., and Bacopoulos, P. (2012). Coastal flooding in Florida's big bend region with application to sea level rise based on synthetic storms analysis. Terr. Atmos. Ocean. Sci. 23:481–500. doi: 10.3319/TAO.2012.04.17.01(WMH)
Hagen, S. C., Passeri, D. L., Bilskie, M. V., DeLorme, D. E., and Yoskowitz, D. (2017). “Systems approaches for HondHole-filled seamless Sa and coastal hazard assessment and resilience,” in Oxford Research Encyclopedia of Natural Hazard Science (Oxford: Oxford University Press), 28.
Honda, T., and Mitsuyasu, H. (1980). “Suimen ni oyobasu kaze no sayou ni kansuru jikkenntekikenkyu,” in Journal of the 27th Japanese Conference on Coastal Engineering, 90–93.
Internal Displacement Monitoring Center (2020). Fiji. Available online at: https://www.internal-displacement.org/countries/fiji (accessed June 29, 2020).
Internal Displacement Monitoring Center (IDMC) (2020). Fiji. Internal Displacement Monitoring Center (IDMC). Available online at: https://www.internal-displacement.org/countries/fiji (accessed November 02, 2020).
IPCC (2014). “Climate change 2014: mitigation of climate change,” in Contribution of Working Group III to the Fifth Assessment Report of the Intergovernmental Panel on Climate Change, editors O. Edenhofer, R. Pichs-Madruga, Y. Sokona, E. Farahani, S. Kadner, K. Seyboth, A. Adler, I. Baum, S. Brunner, P. Eickemeier, B. Kriemann, J. Savolainen, S. Schlömer, C. von Stechow, T. Zwickel and J.C. Minx (Cambridge, New York, NY: Cambridge University Press).
Jarvis, A., Reuter, H., Nelson, A., and Guevara, E. (2008). Hole-Filled Seamless SRTM Data v4. International Centre for Tropical Agriculture (CIAT). Retrieved from http://srtm.csi.cgiar.org/
Kim, S. Y. (2019). “Reference module in earth systems and environmental sciences,” in Encyclopedia of Ocean Sciences, 3rd Edn (Storm Surges), 663–671.
Kotani, M., Imamura, F., and Shuto, N. (1998). New method of tsunami runup and estimation of damage using GIS data Proceedings of the Coastal Engineering. Jpn. Soc. Civil Eng. 45, 356–360.
Lilleør, H. B., and den Broeck, K. V. (2011). Economic drivers of migration and climate change in LDCs. Global Environ. Change 21 (1 Suppl), S70–S81. doi: 10.1016/j.gloenvcha.2011.09.002
Little, C., Horton, R., Kopp, R., Oppenheimer, M., Vecchi, G., and Villarini, G. (2015). Joint projections of US East Coast sea level and storm surge. Nat. Clim. Change 5, 1114–1120. doi: 10.1038/nclimate2801
Liu, P. L.-F., Cho, Y-S., Briggs, M. J., Synolakis, C. E., and Kanoglu, U. (1995). Run-up of solitary waves on a circular island. J. Fluid Mech. 302, 259–285. doi: 10.1017/S0022112095004095
McInnes, K., Walsh, K., Hoeke, R., O' Grady, J., Colberg, F., and Hubbert, G. (2014). Quantifying storm tide risk in Fiji due to climate variability and change. Glob. Planet. Change 116, 115–129. doi: 10.1016/j.gloplacha.2014.02.004
Meyssignac, B., Becker, M., Llovel, W., and Cazenave, A. (2012). An assessment of two-dimensional past sea-level reconstructions over 1950-2009 based on tide-gauge data and different input sea level grids. Surv. Geophys. 33, 945–972. doi: 10.1007/s10712-011-9171-x
Mizuta, R., Murata, A., Ishii, M., Shiogama, H., Hibino, K., Mori, N., et al. (2017). Over 5000 years of ensemble future climate simulations by 60 km global and 20 km regional atmospheric models. Bull. Am. Meteorol. Soc. 98, 1383–1398. doi: 10.1175/BAMS-D-16-0099.1
Mori, N., Kato, M., Kim, S., Mase, H., Shibutani, Y., Takemi, T., et al. (2014). Local amplification of storm surges by super typhoon haiyan in the Leyte Gulf. Geophys. Res. Lett. 41, 5106–5113. doi: 10.1002/2014GL060689
Mori, N., Shimura, T., Yoshida, K., Mizuta, R., Okada, Y., Fujita, M., et al. (2019). Future changes in extreme storm surges based on mega-ensemble projection using a 60-km resolution atmospheric global circulation model. Coast. Eng. J. 61, 295–307. doi: 10.1080/21664250.2019.1586290
Mori, N., and Takemi, T. (2016). Impact assessment of coastal hazards due to future changes in tropical cyclones in the North Pacific Ocean. Weather Clim. Extrem. 11, 53–69. doi: 10.1016/j.wace.2015.09.002
Mortreux, C., and Barnett, J. (2009). Climate change, migration, and adaptation in Funafuti, Tuvalu. Glob. Environ. Change 19, 105–112. doi: 10.1016/j.gloenvcha.2008.09.006
Muis, S., Verlaan, M., Winsemius, H. C., Aerts, J. C., and Ward, P. J. (2016). A global reanalysis of storm surges and extreme sea levels. Nat. Commun. 7:11969. doi: 10.1038/ncomms11969
Nakajo, S., Mori, N., Yasuda, T., and Mase, H. (2014). Global stochastic tropical cyclone model based on principal component analysis and cluster analysis. J. Appl. Meteorol. Climatol. 53, 1547–1577. doi: 10.1175/JAMC-D-13-08.1
National Oceanic Atmospheric Administration (NOAA) (2020). What is Storm Surge? Available online at: https://oceanservice.noaa.gov/facts/stormsurge-stormtide.html (accessed June 29, 2020).
Ninomiya, J., Mori, N., Takemi, T., and Arakawa, O. (2016). Impact assessment of future storm surges due to typhoon Vera as revealed by pseudo-global warming experiments. J. Jpn. Soc. Civil Eng. Ser. B2 72, I_1501–I_1506. doi: 10.2208/kaigan.72.I_1501
Nurse, L.A., McLean, R.F., Agard, J. P., Duvat-Magnan, V., Pelesikoti, N., et al. (2014). “Small islands. Climate change 2014: impacts, adaptation, and vulnerability. Part B: regional aspects,” Contribution of Working Group II to the Fifth Assessment Report of the Intergovernmental Panel on Climate Change, editors V. R. Barros, C. B. Field, D. J. Dokken, M. D. Mastrandrea, K. J. Mach, T. E. Bilir, M. Chatterjee, K. L. Ebi, Y. O. Estrada, R. C. Genova, B. Girma, E. S. Kissel, A. N. Levy, S. MacCracken, P. R. Mastrandrea and L. L. White (Cambridge, New York, NY: Cambridge University Press), 1613–1654.
Oppenheimer, M., Glavovic, B. C., Hinkel, J., van de Wal, R., Magnan, A. K., Abd-Elgawad, A., et al. (2019). Sea Level Rise and Implications for Low-Lying Islands, Coastal Areas, and Communities. IPCC
Passeri, D. L., Hagen, S. C., Medeiros, S. C., Bilskie, M. V., Alizad, K., and Wang, D. (2015). The dynamic effects of sea level rise on low-gradient coastal landscapes: a review. Earth's Future 3, 159–181. doi: 10.1002/2015EF000298
SROCC (2019). Special Report on the Ocean and Cryosphere in a Changing Climate, editors H. O. Pörtner, D. C. Roberts, V. Masson-Delmotte, P. Zhai, M. Tignor, E. Poloczanska, K. Mintenbeck, A. Alegría, M. Nicolai, A. Okem, J. Petzold, B. Rama, N.M. Weyer. SROCC.
Takayabu, I., Hibino, K., Sasaki, H., Shiogama, H., Mori, N., Shibutani, Y., et al. (2015). Effects of climate change on the worst-case storm surge: a case study of Typhoon Haiyan. Environ. Res. Lett. 10:064011. doi: 10.1088/1748-9326/10/6/064011
United Nations (2013). Overview of National Experiences for Population and Housing Censuses of the 2010 Round. New York, NY: United Nations, Statistics Division.
United Nations (2015). Transforming Our World: The 2030 Agenda for Sustainable Development A/RES/70/1. United Nations.
Woodroffe, C. (2008). Reef-island topography and the vulnerability of atolls to sea level rise. Glob. Planet. Change 62, 77–96. doi: 10.1016/j.gloplacha.2007.11.001
World Economic Forum (2016). Global Risks Report 2016. Geneva. Available online at: http://reports.weforum.org/global-risks-2016/ (accessed June 29, 2020).
World Economic Forum (2019). The Global Risks Report 2019, 14th edition. Geneva. Available online at: http://www3.weforum.org/docs/WEF_Global_Risks_Report_2019.pdf (accessed June 29, 2020).
World Meteorological Organization (WMO) (2016). Report of the Sixteenth Session of the WMO RA V Tropical Cyclone Committee for the South Pacific and South-East Indian Ocean. Available online at: https://www.wmo.int/pages/prog/www/tcp/documents/RAV_TCC-16_FinalReport_Approved.pdf (accessed June 29, 2020).
Yang, J. A., Kim, S. Y., Mori, N., and Mase, H. (2018). Assessment of long-term impact of storm surges around the Korean Peninsula based on a large ensemble of climate projections. Coast. Eng. 142, 1–8. doi: 10.1016/j.coastaleng.2018.09.008
Yasuda, T., Mase, H., and Mori, N. (2010). Projection of future typhoons landing on Japan based on a stochastic typhoon model utilizing AGCM projections. Hydrol. Res. Lett. 4, 65–69. doi: 10.3178/hrl.4.65
Keywords: inundation, Fiji, storm surge, sea level rise (SLR), climate change vulnerability, population displacement
Citation: Sabūnas A, Mori N, Fukui N, Miyashita T and Shimura T (2020) Impact Assessment of Climate Change on Storm Surge and Sea Level Rise Around Viti Levu, Fiji. Front. Clim. 2:579715. doi: 10.3389/fclim.2020.579715
Received: 03 July 2020; Accepted: 02 November 2020;
Published: 26 November 2020.
Edited by:
Emma Rosa Mary Archer, University of Pretoria, South AfricaReviewed by:
Johnna Maria Infanti, National Oceanic and Atmospheric Administration (NOAA), United StatesChris C. Funk, United States Geological Survey (USGS), United States
Copyright © 2020 Sabūnas, Mori, Fukui, Miyashita and Shimura. This is an open-access article distributed under the terms of the Creative Commons Attribution License (CC BY). The use, distribution or reproduction in other forums is permitted, provided the original author(s) and the copyright owner(s) are credited and that the original publication in this journal is cited, in accordance with accepted academic practice. No use, distribution or reproduction is permitted which does not comply with these terms.
*Correspondence: Audrius Sabūnas, sabunas.audrius.78x@st.kyoto-u.ac.jp