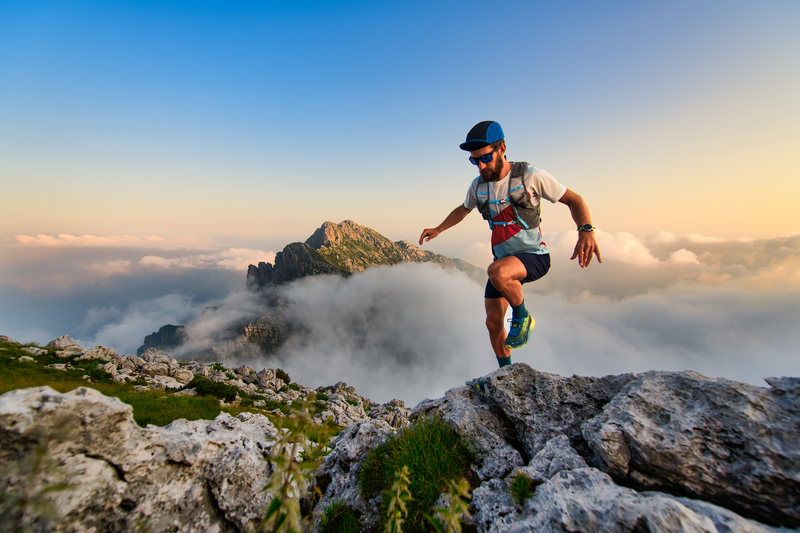
94% of researchers rate our articles as excellent or good
Learn more about the work of our research integrity team to safeguard the quality of each article we publish.
Find out more
ORIGINAL RESEARCH article
Front. Chem. , 05 February 2025
Sec. Theoretical and Computational Chemistry
Volume 13 - 2025 | https://doi.org/10.3389/fchem.2025.1548269
Multiple sclerosis (MS) is a chronic autoimmune illness characterized by demyelination, neurodegeneration, and inflammation in the central nervous system. The AXL gene, which codes for a receptor tyrosine kinase, has emerged as a promising therapeutic target due to its involvement in neuroinflammation and oligodendrocyte dysfunction. In the current study, we employed in silico techniques to design Antisense Oligonucleotides (ASOs) that selectively target AXL gene transcripts to modulate AXL expression and mitigate MS pathology. Three ASOs, A1, A2, and A3, were designed to specifically target the 5′ untranslated region (5′UTR) and coding region of the AXL gene transcripts. The ASOs were optimized with a focus on stability, binding affinity, and specificity towards AXL mRNA while minimizing off-target effects. To investigate ASO-mRNA interactions and gauge their ability to alter AXL expression, Molecular Docking was performed. Our analyses showed that A1, A2, and A3 had substantial interactions with AXL mRNA, with binding affinities of −9.5 kcal/mol, −10.8 kcal/mol, and −10.6 kcal/mol, respectively. The targeting of AXL gene transcripts through ASOs shows promise in reducing MS symptoms. Precision ASO-based therapies could effectively manage MS by targeting the essential pathways involved in the disease. ASOs provide a highly targeted approach for treating MS and offer a precise therapeutic strategy for this debilitating condition. The study lays the groundwork for future in vitro and in vivo studies to confirm the therapeutic potential of these ASOs for the treatment of MS.
Multiple sclerosis (MS) is a chronic inflammatory demyelinating autoimmune disorder of central nervous system (CNS) with unknown etiology (Al-Ghezi et al., 2019). It was considered as a rare disease in India in the past, studies from AIIMS Delhi (2015) over time suggest an increased incidence of MS in India. The precise prevalence of MS in India is not well known due to a dearth of epidemiological research, diagnoses, and registries that have been properly undertaken. However, by the current report from World Health Organization (WHO) and Multiple Sclerosis International Federation (MSIF) 2013, the crude prevalence rate of MS in India is about 5–20 per 100,000 which is much higher than the studies reported previously. Globally, MS prevalence has increased dramatically, affecting more than 2.3 million people (Bhatia et al., 2015; Zahoor and Haq, 2017).
The development of growth and survival factors that prevent axonal and oligodendrocyte loss and inflammation as well as guard against these effects is a priority in the treatment of multiple sclerosis (Baecher-Allan et al., 2018). Growth-arrest specific protein 6 (Gas6) is one growth factor related to the development, survival, and suppression of oligodendrocyte immune response (Noseworthy et al., 2000; Franklin, 2002; Raine, 1997; Fontoura et al., 2006; Frohman et al., 2006). Gas6 is a secreted protein that is widely produced in the central and peripheral nervous systems by endothelial cells and neurons. It plays a variety of physiological and pathological roles, including cell proliferation, survival, and apoptosis (Torii and Yamauchi, 2016) (Figure 1).
Tyro3 (Rse/Dtk/Sky), Axl (Ufo), and Mer (Eyk) are members of the TAM family of receptor tyrosine kinases, which Gas6 binds to and activates (Stitt et al., 1995). All three receptors are expressed by a wide variety of cell types, and both homophilic and heterophilic interactions can activate a receptor. The interactions between these TAM and Gas6 are shown in Figure 2 (O’Bryan et al., 1995; Thorp et al., 2011; Bellan et al., 2016). The major and minor Gas6 binding grooves are found in Axl. Tyro3 and Mer only share the minor groove, therefore the response to Gas6 is mediated in a concentration-dependent manner with a preference for Axl over Tyro3 over Mer. TAM receptor cleavage is carried out by two metalloproteinases called ADAM10 and ADAM17. In close proximity to the transmembrane region, their proteolytic domain cleaves TAM receptors, generating soluble TAM (sTAM). sTAM receptors reduce the number of ligand sites on the cell membrane and serve as decoy receptors to suppress the Gas6 function (Goruppi et al., 1999).
Figure 2. Interaction between TAM (Tyro, Axl, and Mer) receptors and growth arrest-specific receptor 6 (Gas6).
Over the years, various treatments and drugs have been developed to suppress the immune system and provide potential benefits such as myelin preservation and remission of symptoms. However, these therapies are not without their drawbacks, as they can lead to adverse effects (AE) ranging from mild to severe (Talanki Manjunatha et al., 2022). In the current study, a promising approach involving the use of Antisense Oligonucleotides (ASOs) targeting the mRNA of the AXL gene to mitigate adverse effects associated with MS treatment. The advantages of ASOs over small molecules are mentioned in Table 1.
Table 1. Advantages of antisense oligo therapeutics over small molecule therapeutics (Quemener et al., 2022; Yin and Rogge, 2019; Shen and Corey, 2018).
We have targeted the AXL gene for the prevention of Multiple Sclerosis using ASOs (antisense oligonucleotides), which provides a rapid and high-throughput method for silencing AXL gene expression. The antisense technology relies on Watson-Crick base-pairing interactions for its specificity, and the most successful knockdowns alongside this method are frequently seen with an RNase H-dependent and RNase H-independent cleavage mechanism of the mRNA targets. ASOs targeting the 5′ and 3′ UTR Sterically blocks ASOs can also physically hinder or prevent translation or splicing (Dhuri et al., 2020; Boros et al., 2022; Baharlooi et al., 2022; Bilanges and Stokoe, 2005).
The specificity of the biological effects shown by ASOs has, however, been the subject of debate over the past several years. The oligonucleotide backbone has been modified to reduce harmful and non-specific effects, limit oligonucleotide degradation (from endogenous nucleases), and increase specificity and affinity (Scoles et al., 2019; Kulkarni et al., 2021).
AXL protein-protein interaction network was performed using the String database. Publication involving Multiple sclerosis data was selected for further constructing a protein-protein interaction network. The network was imported into Cytoscape for further examination. Following this, the network analyzer tool was used to obtain the expression data for all nodes in the network (Shannon et al., 2003; Szklarczyk et al., 2011).
The mRNA sequence of the targeted Homo sapiens AXL receptor tyrosine kinase (transcript variant 3 mRNA) was retrieved in FASTA format from NCBI Gene Bank with an accession no: >NM_001278599.2. The open reading frame was predicted using the Translate tool from Expasy.
ASOs were designed using the sfold (http://sfold.wadsworth.org/) web server and the ones with the highest Binding energy and minimum cross-reaction with other gene transcripts were chosen for the analysis. The optimal ASO were selected based on factors such as binding energy, GC content, and avoidance of GGGG motifs. Stronger binding is indicated by a smaller binding energy, with a desired value of −8 kcal/mol for designing potent ASOs. This threshold is based on established guidelines for antisense oligonucleotide design, where binding energies in this range have been shown to ensure strong hybridization with the target RNA, enhancing efficacy while minimizing off-target interactions. The binding energy of the ASO is determined by the weighted sum of DNA/RNA stacking energies for the hybrid formed between the ASO and the target sequence. The sfold software provides computational tools for the rational design of RNA-targeting nucleic acids such as small interfering RNAs (siRNAs), antisense oligonucleotides, and trans-cleaving ribozymes for gene knock-down investigations. The process for designing siRNAs is based on a mix of siRNA duplex thermodynamic parameters, RNA target accessibility prediction, and empirical design principles. In order to take into account, the likelihood that a population of structures for the target mRNA exists, our method for evaluating target accessibility is a novel modification of the underlying RNA folding algorithm. The application module Srna includes complete features for statistical representation of sampled structures in addition to the application modules Sirna, Soligo, and Sribo for siRNAs, antisense oligos, and ribozymes, respectively (Ding et al., 2004; Bennett et al., 2019; Crooke et al., 2018; Hendling and Barišić, 2019; Crooke et al., 2021).
Molecular docking was performed using Schrodinger protein-protein docking PIPER. Molecular docking involves the preparation of three-dimensional structure prediction of target mRNA, Antisense oligos and Docking.
Secondary structure prediction from the sequence: the mRNA secondary structures were predicted using RNA folding form of mfold web server (http://www.unafold.org/mfold/applications/rna-folding-form.php) based on energy minimisation techniques. The sequences were selected at ionic concentrations of Na+ at 120 mM and Mg at 5 mM. So, the prediction was also done keeping the same ionic concentration. In mfold, all possible secondary structures are approximated based on Watson-Crick base pairing and the most thermodynamically stable structures are selected. The structure with minimum free energy was selected. Mfold gives the predicted results in various formats such as ct file, vienna, pdf, png, etc, (Zuker, 2003).
The three-dimensional structure of target RNA was predicted using RNA composer: the input for RNA composer is ct file from mfold secondary structure prediction which is converted to dot-bracket notation by the server itself and is sent to the home page for prediction of 3D structure of the RNA. This web server operates on the RNA FRABASE database which has RNA 3D structures. This server searches the fragment of the RNA input in secondary structure in dot-bracket notation and gives the 3D structure in pub format. The output is a pdb structure of the RNA the same structure was utilized for Docking (SantaLucia, 1998).
Secondary structure prediction from the sequence: ssDNA secondary structures were predicted using the DNA folding form of mfold web server (http://unafold.rna.albany.edu/?q=mfold/DNA-Folding-Form) which uses the free energies from the laboratory of SantaLucia (1998). The sequences were selected at ionic concentration of Na+ at 120 mM and Mg at 5 mM. So, the prediction was also done keeping the same ionic concentration. In mfold, all possible secondary structures are approximated based on Watson-Crick base pairing and the most thermodynamically stable structures are selected. In addition to the predicted secondary structure of the ssDNA this step provides the minimum free energy of the fold. The structure with minimum free energy was selected. Mfold gives the predicted results in various formats such as ct file, vienna, pdf, png, etc, (Zuker, 2003).
ssRNA tertiary structure prediction using RNA composer: the input for RNA composer is ct file from mfold secondary structure prediction which is converted to dot-bracket notation by the server itself and is sent to home page for prediction of 3D structure of ssRNA. This server automatically takes “t or T” as ‘U’ and gives the tertiary structure of RNA. This web server operates on the RNA FRABASE database which has RNA 3D structures. This server searches the fragment of the RNA input in secondary structure in dot-bracket notation and gives the 3D structure in pub format (SantaLucia, 1998).
Conversion of ssRNA to ssDNA 3D structure: the conversion of three-dimensional structure of ssRNA to ssDNA was performed using an in-house developed tool RNA2DNA which takes the input as.pdb files of ssRNA 3D structure and converts it to the ssDNA by editing the uracil residues of RNA to thymine residues and Deoxygenating the Ribose sugar to deoxy ribose sugar (Biesiada et al., 2016).
Energy minimization of the predicted structure using the UCSF chimera tool (Jeddi and Saiz, 2017).
Three-dimensional structures of both target RNA and AXL-ASO were incorporated into the Schrodinger Maestro workspace and were prepared using protein preparation workflow. The target mRNA fragment and the ASO were docked using the PIPER protein-protein docking platform. The mRNA fragment was chosen for receptor and ASO was chosen as the ligand. PIPER uses FFT-based docking approach to efficiently predict the interactions where it employs a grid-based representation of the docking search space and computes the correlation between Fourier transforms of receptor surface to estimate interaction energies. Stochastic optimization methods are used to sample conformational space, and generated docking poses are ranked based on correlation scores. Optionally, refinement steps may be included to improve accuracy. The top 30 poses were incorporated for docking analysis and the best pose was chosen based on the number of H-bonds and the binding pose.
The string database was searched for the AXL gene in the query for Pathway/Process/Disease/Publication. A publication that involves data on multiple sclerosis was chosen to aid in the development of a protein-protein interaction network. The publication involved six proteins GAS6, FURIN, ADAM10, ADAM17, TYRO3 and AXL, which formed a network consisting of a specified number of clusters. The number of nodes, edges, an average node of degree, avg. local clustering was found to be 6,9,3,0.75 respectively. The network was imported into Cytoscape for further examination. Following this, the network analyzer tool and the cytoHubba plugin were used to obtain the expression data for all nodes in the network. Top expressed nodes were colour-coded to highlight their expression degrees, facilitating further investigation and analysis. The continuous mapping option in the node-filling feature was used to identify the most highly expressed nodes based on the degree parameter. The protein networks were colour-coded according to the degree of expression. The FURIN, ADAM10, and GAS6 were highly expressed when compared to ADAM17, TYRO3 and AXL are mentioned in Figure 3.
Figure 3. AXL protein-Protein interaction network showing ADAM10, FURIN, GAS6 as highly expressed genes.
The ASOs were designed to target the AXL gene transcripts using sfold server. The server gave an output of 157 ASOs. The obtained ASOs were filtered based on the criteria of GC content of 50% and above, antisense oligo binding energy above −8 kcal/mol, and minimal cross-reaction with non-target genes which was checked by performing NCBI-BLAST for the sequence similarity check and three sequences with minimum sequence similarity with non-target genes were selected as final ASOs. A1 and A2 target the 5′ UTR region of the mRNA with target position of 229–248 and 230–249 with binding energy of −9.5 kcal/mol and −10.8 kcal/mol respectively and A3 targeting the coding sequence with target position of 923–942 and Binding energy of −10.6 kcal/mol with the AXL mRNA. The final three ASOs are depicted in Table 2.
The secondary structure and tertiary structure of the designed ASOs were predicted and are depicted in Figure 4. The secondary structure of the anti-AXL-ASOs had typically bulge loops, hairpin loops and single-stranded structures which are essential for binding and interaction with the target.
The three ASOs were docked to their respective target mRNA fragments. A1 was found to be forming 13 H-bonds with the corresponding target, A2 was found to be forming 8 H-bonds with the corresponding target and A3 was found to be forming 15 H-bonds with the corresponding target. The binding residues and their interactions of the respective ASOs with their targets are depicted in Figure 5.
Multiple Sclerosis (MS) is a persistent autoimmune condition that attacks myelin and obstructs communication in the central nervous system. The TAM receptors (Tyro3, Axl, and Mer) and their ligand Gas6 play critical roles in human biology by controlling apoptosis, inflammation, and cell proliferation (Pettersen et al., 2004). In a study on multiple sclerosis by Weinger et al. (2009) found that in both chronic active and chronic silent, elevated levels of membrane-bound Mer, soluble Mer, and soluble Axl were found compared to normal tissue. While Gas6 positively correlated with soluble Axl and Mer in normal tissue, an inverse negative correlation was observed between Gas6 and soluble Axl and Mer in established multiple sclerosis lesions (Kozakov et al., 2006). Several mechanisms, including neurotrophic effects, anti-inflammatory effects on microglia, trophic effects on oligodendrocytes mediated by Axl, and pro-phagocytic activities mediated by Axl and MerTK, are probably responsible for this protection. TAM activation has a positive effect on MS lesions supported by limited but reliable evidence from human research.
The FDA approved drugs like Glatiramer Acetate, Alemtuzumab, Dimethyl Fumarate, Laquinimod, Teriflunomide, Rituximab, Daclizumab, Cladribine has shown efficacy in reducing relapse rates, functions by modulating the immune response to myelin antigens and disability progression. Adverse effects mainly include lymphopenia, increased risk of infections and other effects (Lemke, 2013). To overcome the adverse effects associated with MS treatments, a novel approach involving Antisense Oligonucleotides (ASOs) targeting the mRNA of the AXL gene has been proposed. The antisense technology relies on Watson-Crick base-pairing interactions for its specificity, and the most successful knockdown alongside this method is frequently seen with an RNase H-dependent and RNase H-independent cleavage mechanism of the mRNA targets (Wei et al., 2021).
In the current study, a protein-protein interaction network was built using string and Cytoscape tools. We specifically designed three Antisense oligos targeting AXL gene transcripts at the 5′UTR region and coding region. The sequence and target region of the ASOs with the binding affinity are given in Table 2. The secondary and tertiary structures of the ASOs were predicted. The binding affinities of the ASOs (−9.5, −10.8, and −10.6 kcal/mol) were influenced by the number and distribution of hydrogen bonds formed between the ASOs and target mRNA. For instance, A3, with the highest number of hydrogen bonds (Goruppi et al., 1999), showed the strongest interaction, indicating its potential for efficient target hybridization. Residues were critical for stabilizing these interactions, aligning with findings from previous studies that highlight the importance of hydrogen bonding in ASO-mRNA binding stability (Crooke et al., 2021; Liang et al., 2017; Lange et al., 2022). These results suggest that A1, A2, and A3 could effectively modulate AXL expression, a critical factor in neuroinflammation and oligodendrocyte dysfunction associated with MS. While the computational design and docking analyses confirm the theoretical feasibility of these ASOs, further experimental validation is necessary to evaluate their therapeutic potential. Importantly, this study lays the groundwork for exploring ASOs as a targeted approach to managing MS, complementing existing therapies that primarily focus on immune modulation. The three AXO ASOs are selected based on their binding energy to the respective target sites: A1, which has a target site between 229 and 248; A2, which has a target site between 230 and 249 binding to the 5′ UTR, and A3, which has a target site between 923 and 942, which is a coding region with an open reading frame in between the 377 and 2,257 nucleotides translating to a 626aa. ASOs targeting the 5′ and 3′ UTR Sterically block ASOs can also physically hinder or prevent translation or splicing. For instance, in human umbilical vein endothelial cells, Baker et al. (1997) created RNase H-independent 2′-O-2-methoxyethyl ASOs that were complementary to the 5′ cap region of the intercellular adhesion molecule 1 (ICAM-1) transcript. By preventing the assembly of the 80S translation initiation complex, these ASOs prevented protein production of the targeted transcript (Baharlooi et al., 2022).
This is the first approach for the treatment of Multiple Sclerosis using Antisense Oligonucleotide therapeutics targeting AXL gene. This approach aims to modulate the immune response and potentially prevent MS development with minimal adverse effects. However, further research and clinical trials are necessary to validate the efficacy and safety of this innovative strategy.
MS treatment options, such as Glatiramer acetate, Alemtuzumab, Dimethyl Fumarate, Teriflunomide, Laquinimod, Rituximab, Daclizumab, and Cladribine, have demonstrated varying efficacies and associated adverse effects. Although these drugs have improved the lives of many MS patients, the pursuit of safer and more effective treatments remains ongoing. The exploration of novel approaches, such as Antisense Oligonucleotides targeting the AXL gene, holds promise for minimizing adverse effects while effectively managing MS. Continued research and advancements in MS therapeutics will ultimately provide improved outcomes and better quality of life for individuals affected by this debilitating disease.
The original contributions presented in the study are included in the article/supplementary material, further inquiries can be directed to the corresponding authors.
BS: Conceptualization, Data curation, Formal Analysis, Investigation, Writing–original draft, Writing–review and editing. AN: Data curation, Formal Analysis, Methodology, Writing–original draft, Writing–review and editing. CD: Investigation, Software, Validation, Writing–original draft, Writing–review and editing. AJ: Investigation, Software, Writing–original draft, Writing–review and editing. BH: Investigation, Visualization, Writing–original draft, Writing–review and editing. SS: Validation, Visualization, Writing–original draft, Writing–review and editing. HA-M: Methodology, Validation, Writing–original draft, Writing–review and editing. SA: Validation, Visualization, Writing–original draft, Writing–review and editing. SP: Project administration, Writing–original draft, Writing–review and editing. CSr: Project administration, Visualization, Writing–original draft, Writing–review and editing. CSh: Supervision, Writing–original draft, Writing–review and editing. SK: Supervision, Validation, Visualization, Writing–original draft, Writing–review and editing.
The author(s) declare that financial support was received for the research, authorship, and/or publication of this article. This research was supported by King Saud University, Riyadh, Saudi Arabia, Project Number (RSPD2025R709).
The authors BS, acknowledge JSS AHER for JSS AHER Overseas Research Fellowship 2023–24 to conduct the research. The authors AN, CD, AJ, BH, SVS and CS acknowledge the support and infrastructure provided by JSS AHER. SPK thank Amrita Vishwa Vidyapeetham for support extended towards the collaborations. CSS acknowledge Davangere University for their extended support. The author SNS acknowledges the JSS Science and Technology University, Mysuru for their extended support. The authors HAA and SFA acknowledge and extend their appreciation to the Researchers Supporting Project Number (RSPD2025R709), King Saud University, Riyadh, Saudi Arabia, for supporting this study.
The authors declare that the research was conducted in the absence of any commercial or financial relationships that could be construed as a potential conflict of interest.
The reviewer HPN declared a shared affiliation with the author CSr at the time of review.
The author(s) declare that no Generative AI was used in the creation of this manuscript.
All claims expressed in this article are solely those of the authors and do not necessarily represent those of their affiliated organizations, or those of the publisher, the editors and the reviewers. Any product that may be evaluated in this article, or claim that may be made by its manufacturer, is not guaranteed or endorsed by the publisher.
MS, multiple sclerosis; ASOs, Antisense Oligonucleotides; CNS, Central Nervous System; AE, Adverse Effects; AD, Alzheimer’s Disease; HD, Huntington’s Disease; ALS, Amyotrophic Lateral Sclerosis, ICAM-1, Intercellular Adhesion Molecule 1.
Al-Ghezi, Z. Z., Miranda, K., Nagarkatti, M., and Nagarkatti, P. S. (2019). Combination of cannabinoids, Δ9- tetrahydrocannabinol and cannabidiol, ameliorates experimental multiple sclerosis by suppressing neuroinflammation through regulation of miRNA-mediated signaling pathways. Front. Immunol. 10, 1921. doi:10.3389/fimmu.2019.01921
Baecher-Allan, C., Kaskow, B. J., and Weiner, H. L. (2018). Multiple sclerosis: mechanisms and immunotherapy. Neuron 97 (4), 742–768. doi:10.1016/j.neuron.2018.01.021
Baharlooi, H., Mansourabadi, A. H., Minbashi Moeini, M., Mohamed Khosroshahi, L., and Azimi, M. (2022). Nucleic acids as novel therapeutic modalities to address multiple sclerosis onset and progression. Cell. Mol. Neurobiol. 42 (8), 2611–2627. doi:10.1007/s10571-021-01158-4
Baker, B. F., Lot, S. S., Condon, T. P., Cheng-Flournoy, S., Lesnik, E. A., Sasmor, H. M., et al. (1997). 2'-O-(2-Methoxy)ethyl-modified anti-intercellular adhesion molecule 1 (ICAM-1) oligonucleotides selectively increase the ICAM-1 mRNA level and inhibit formation of the ICAM-1 translation initiation complex in human umbilical vein endothelial cells. J. Biol. Chem. 272 (18), 11994–12000. doi:10.1074/jbc.272.18.11994
Bellan, M., Pirisi, M., and Sainaghi, P. P. (2016). The Gas6/TAM system and multiple sclerosis. Int. J. Mol. Sci. 17 (11), 1807. doi:10.3390/ijms17111807
Bennett, C. F., Krainer, A. R., and Cleveland, D. W. (2019). Antisense oligonucleotide therapies for neurodegenerative diseases. Annu. Rev. Neurosci. 42, 385–406. doi:10.1146/annurev-neuro-070918-050501
Bhatia, R., Bali, P., and Chaudhari, R. M. (2015). Epidemiology and genetic aspects of multiple sclerosis in India. Ann. Indian Acad. Neurology 18 (Suppl. 1), S6–S10. doi:10.4103/0972-2327.164814
Biesiada, M., Purzycka, K. J., Szachniuk, M., Blazewicz, J., and Adamiak, R. W. (2016). Automated RNA 3D structure prediction with RNAComposer. Methods Mol. Biol. Clift. N.J. 1490, 199–215. doi:10.1007/978-1-4939-6433-8_13
Bilanges, B., and Stokoe, D. (2005). Direct comparison of the specificity of gene silencing using antisense oligonucleotides and RNAi. Biochem. J. 388 (Pt 2), 573–583. doi:10.1042/BJ20041956
Boros, B. D., Schoch, K. M., Kreple, C. J., and Miller, T. M. (2022). Antisense oligonucleotides for the study and treatment of ALS. Neurother. J. Am. Soc. Exp. Neurother. 19 (4), 1145–1158. doi:10.1007/s13311-022-01247-2
Crooke, S. T., Baker, B. F., Crooke, R. M., and Liang, X. H. (2021). Antisense technology: an overview and prospectus. Nat. Rev. Drug Discov. 20, 427–453. doi:10.1038/s41573-021-00162-z
Crooke, S. T., Witztum, J. L., Bennett, C. F., and Baker, B. F. (2018). RNA-targeted therapeutics. Cell metab. 27 (4), 714–739. doi:10.1016/j.cmet.2018.03.004
Dhuri, K., Bechtold, C., Quijano, E., Pham, H., Gupta, A., Vikram, A., et al. (2020). Antisense oligonucleotides: an emerging area in drug discovery and development. J. Clin. Med. 9 (6), 2004. doi:10.3390/jcm9062004
Ding, Y., Chan, C. Y., and Lawrence, C. E. (2004). Sfold web server for statistical folding and rational design of nucleic acids. Nucleic acids Res. 32 (Web Server issue), W135–W141. doi:10.1093/nar/gkh449
Fontoura, P., Steinman, L., and Miller, A. (2006). Emerging therapeutic targets in multiple sclerosis. Curr. Opin. neurology 19 (3), 260–266. doi:10.1097/01.wco.0000227035.93199.e0
Franklin, R. J. (2002). Why does remyelination fail in multiple sclerosis? Nat. Rev. Neurosci. 3 (9), 705–714. doi:10.1038/nrn917
Frohman, E. M., Racke, M. K., and Raine, C. S. (2006). Multiple sclerosis--the plaque and its pathogenesis. N. Engl. J. Med. 354 (9), 942–955. doi:10.1056/NEJMra052130
Goruppi, S., Ruaro, E., Varnum, B., and Schneider, C. (1999). Gas6-mediated survival in NIH3T3 cells activates stress signalling cascade and is independent of Ras. Oncogene 18, 4224–4236. doi:10.1038/sj.onc.1202788
Hendling, M., and Barišić, I. (2019). In-silico design of DNA oligonucleotides: challenges and approaches. Comput. Struct. Biotechnol. J. 17, 1056–1065. doi:10.1016/j.csbj.2019.07.008
Jeddi, I., and Saiz, L. (2017). Three-dimensional modeling of single stranded DNA hairpins for aptamer-based biosensors. Sci. Rep. 7, 1178. doi:10.1038/s41598-017-01348-5
Kozakov, D., Brenke, R., Comeau, S. R., and Vajda, S. (2006). PIPER: an FFT-based protein docking program with pairwise potentials. Proteins 65 (2), 392–406. doi:10.1002/prot.21117
Kulkarni, J. A., Witzigmann, D., Thomson, S. B., Chen, S., Leavitt, B. R., Cullis, P. R., et al. (2021). The current landscape of nucleic acid therapeutics. Nat. Nanotechnol. 16, 630–643. doi:10.1038/s41565-021-00898-0
Lange, J., Zhou, H., and McTague, A. (2022). Cerebral organoids and antisense oligonucleotide therapeutics: challenges and opportunities. Front. Mol. Neurosci. 15, 941528. doi:10.3389/fnmol.2022.941528
Lemke, G. (2013). Biology of the TAM receptors. Cold Spring Harb. Perspect. Biol. 5 (11), a009076. doi:10.1101/cshperspect.a009076
Liang, X. H., Sun, H., Nichols, J. G., and Crooke, S. T. (2017). RNase H1-dependent antisense oligonucleotides are robustly active in directing RNA cleavage in both the cytoplasm and the nucleus. Mol. Ther. J. Am. Soc. Gene Ther. 25 (9), 2075–2092. doi:10.1016/j.ymthe.2017.06.002
Noseworthy, J. H., Lucchinetti, C., Rodriguez, M., and Weinshenker, B. G. (2000). Multiple sclerosis. N. Engl. J. Med. 343 (13), 938–952. doi:10.1056/NEJM200009283431307
O’Bryan, J. P., Fridell, Y. W., Koski, R., Varnum, B., and Liu, E. T. (1995). The transforming receptor tyrosine kinase, Axl, is post-translationally regulated by proteolytic cleavage. J. Biol. Chem. 270 (2), 551–557. doi:10.1074/jbc.270.2.551
Pettersen, E. F., Goddard, T. D., Huang, C. C., Couch, G. S., Greenblatt, D. M., Meng, E. C., et al. (2004). UCSF Chimera--a visualization system for exploratory research and analysis. J. Comput. Chem. 25 (13), 1605–1612. doi:10.1002/jcc.20084
Quemener, A. M., Centomo, M. L., Sax, S. L., and Panella, R. (2022). Small drugs, huge impact: the extraordinary impact of antisense oligonucleotides in research and drug development. Mol. Basel, Switz. 27 (2), 536. doi:10.3390/molecules27020536
Raine, C. S. (1997). The Norton Lecture: a review of the oligodendrocyte in the multiple sclerosis lesion1Presented at the Albert Einstein College of Medicine, Bronx, NY, on February 3, 1997 in honor of the retirement of Dr. William T. Norton, a pioneer neurochemist in the myelin/oligodendrocyte arena.1. J. Neuroimmunol. 77 (2), 135–152. doi:10.1016/s0165-5728(97)00073-8
SantaLucia, J. (1998). A unified view of polymer, dumbbell, and oligonucleotide DNA nearest-neighbor thermodynamics. Proc. Natl. Acad. Sci. U. S. A. 95 (4), 1460–1465. doi:10.1073/pnas.95.4.1460
Scoles, D. R., Minikel, E. V., and Pulst, S. M. (2019). Antisense oligonucleotides: a primer. Genetics 5 (2), e323. doi:10.1212/NXG.0000000000000323
Shannon, P., Markiel, A., Ozier, O., Baliga, N. S., Wang, J. T., Ramage, D., et al. (2003). Cytoscape: a software environment for integrated models of biomolecular interaction networks. Genome Res. 13 (11), 2498–2504. doi:10.1101/gr.1239303
Shen, X., and Corey, D. R. (2018). Chemistry, mechanism and clinical status of antisense oligonucleotides and duplex RNAs. Nucleic acids Res. 46 (4), 1584–1600. doi:10.1093/nar/gkx1239
Stitt, T. N., Conn, G., Gore, M., Lai, C., Bruno, J., Radziejewski, C., et al. (1995). The anticoagulation factor protein S and its relative, Gas6, are ligands for the Tyro 3/Axl family of receptor tyrosine kinases. Cell 80 (4), 661–670. doi:10.1016/0092-8674(95)90520-0
Szklarczyk, D., Franceschini, A., Kuhn, M., Simonovic, M., Roth, A., Minguez, P., et al. (2011). The STRING database in 2011: functional interaction networks of proteins, globally integrated and scored. Nucleic acids Res. 39 (Database issue), D561–D568. doi:10.1093/nar/gkq973
Talanki Manjunatha, R., Habib, S., Sangaraju, S. L., Yepez, D., and Grandes, X. A. (2022). Multiple sclerosis: therapeutic strategies on the horizon. Cureus 14 (5), e24895. doi:10.7759/cureus.24895
Thorp, E., Vaisar, T., Subramanian, M., Mautner, L., Blobel, C., and Tabas, I. (2011). Shedding of the Mer tyrosine kinase receptor is mediated by ADAM17 protein through a pathway involving reactive oxygen species, protein kinase Cδ, and p38 mitogen-activated protein kinase (MAPK). J. Biol. Chem. 286 (38), 33335–33344. doi:10.1074/jbc.M111.263020
Torii, T., and Yamauchi, J. (2016). Gas6-Tyro3 signaling is required for Schwann cell myelination and possible remyelination. Neural Regen. Res. 11 (2), 215–216. doi:10.4103/1673-5374.177714
Wei, W., Ma, D., Li, L., and Zhang, L. (2021). Progress in the application of drugs for the treatment of multiple sclerosis. Front. Pharmacol. 12, 724718. doi:10.3389/fphar.2021.724718
Weinger, J. G., Omari, K. M., Marsden, K., Raine, C. S., and Shafit-Zagardo, B. (2009). Up-regulation of soluble Axl and Mer receptor tyrosine kinases negatively correlates with Gas6 in established multiple sclerosis lesions. Am. J. pathology 175 (1), 283–293. doi:10.2353/ajpath.2009.080807
Yin, W., and Rogge, M. (2019). Targeting RNA: a transformative therapeutic strategy. Clin. Transl. Sci. 12 (2), 98–112. doi:10.1111/cts.12624
Zahoor, I., and Haq, E. (2017). Multiple sclerosis in India: iceberg or volcano. J. Neuroimmunol. 307, 27–30. doi:10.1016/j.jneuroim.2017.03.015
Keywords: AXL gene, antisense oligonucleotides, multiple sclerosis, neurodegeneration inflammation, therapeutics
Citation: Shreevatsa B, Nagaraj A, Dharmashekar C, Jain A, Harendra B, Siddalingegowda SV, Al-Mazroua HA, Ahmad SF, Prasad SK, Srinivasa C, Shivamallu C and Kollur SP (2025) Exploring precision therapeutics: computational design of antisense oligonucleotides targeting AXL gene transcripts in multiple sclerosis treatment management. Front. Chem. 13:1548269. doi: 10.3389/fchem.2025.1548269
Received: 19 December 2024; Accepted: 06 January 2025;
Published: 05 February 2025.
Edited by:
Niyazi Bulut, Firat University, TürkiyeReviewed by:
Omer Kaygili, Firat University, TürkiyeCopyright © 2025 Shreevatsa, Nagaraj, Dharmashekar, Jain, Harendra, Siddalingegowda, Al-Mazroua, Ahmad, Prasad, Srinivasa, Shivamallu and Kollur. This is an open-access article distributed under the terms of the Creative Commons Attribution License (CC BY). The use, distribution or reproduction in other forums is permitted, provided the original author(s) and the copyright owner(s) are credited and that the original publication in this journal is cited, in accordance with accepted academic practice. No use, distribution or reproduction is permitted which does not comply with these terms.
*Correspondence: Chandan Shivamallu, Y2hhbmRhbnNAanNzdW5pLmVkdS5pbg==; Shiva Prasad Kollur, c2hpdmFjaGVtaXN0QGdtYWlsLmNvbQ==
Disclaimer: All claims expressed in this article are solely those of the authors and do not necessarily represent those of their affiliated organizations, or those of the publisher, the editors and the reviewers. Any product that may be evaluated in this article or claim that may be made by its manufacturer is not guaranteed or endorsed by the publisher.
Research integrity at Frontiers
Learn more about the work of our research integrity team to safeguard the quality of each article we publish.