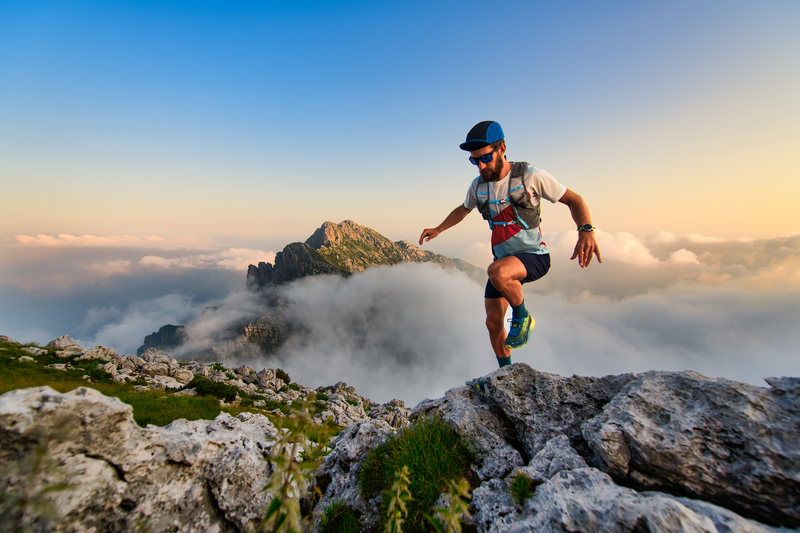
94% of researchers rate our articles as excellent or good
Learn more about the work of our research integrity team to safeguard the quality of each article we publish.
Find out more
ORIGINAL RESEARCH article
Front. Chem. , 09 January 2025
Sec. Medicinal and Pharmaceutical Chemistry
Volume 12 - 2024 | https://doi.org/10.3389/fchem.2024.1503593
Introduction: Treatment of type 2 diabetes (T2D) remains a significant challenge because of its multifactorial nature and complex metabolic pathways. There is growing interest in finding new therapeutic targets that could lead to safer and more effective treatment options. Takeda G protein-coupled receptor 5 (TGR5) is a promising antidiabetic target that plays a key role in metabolic regulation, especially in glucose homeostasis and energy expenditure. TGR5 agonists are attractive candidates for T2D therapy because of their ability to improve glycemic control. This study used machine learning-based models (ML), molecular docking (MD), and molecular dynamics simulations (MDS) to explore novel small molecules as potential TGR5 agonists.
Methods: Bioactivity data for known TGR5 agonists were obtained from the ChEMBL database. The dataset was cleaned and molecular descriptors based on Lipinski’s rule of five were selected as input features for the ML model, which was built using the Random Forest algorithm. The optimized ML model was used to screen the COCONUT database and predict potential TGR5 agonists based on their molecular features. 6,656 compounds predicted from the COCONUT database were docked within the active site of TGR5 to calculate their binding energies. The four top-scoring compounds with the lowest binding energies were selected and their activities were compared to those of the co-crystallized ligand. A 100 ns MDS was used to assess the binding stability of the compounds to TGR5.
Results: Molecular docking results showed that the lead compounds had a stronger affinity for TGR5 than the cocrystallized ligand. MDS revealed that the lead compounds were stable within the TGR5 binding pocket.
Discussion: The combination of ML, MD, and MDS provides a powerful approach for predicting new TGR5 agonists that can be optimised for T2D treatment.
Type 2 diabetes (T2D) is an escalating metabolic disorder of global health concern (Ong et al., 2023). This disease is characterised by persistent hyperglycaemia due to insulin resistance and an eventual decline in pancreatic β-cell function (Bhatti et al., 2022; Deol and Bashir, 2024). In 2017, approximately 462 million people worldwide were affected by T2DM (Abdul Basith Khan et al., 2020). Individuals with T2DM are susceptible to long-term complications, including cardiovascular disease, neuropathy, retinopathy, and kidney failure, which lead to significant morbidity and mortality (DeFronzo et al., 2015; Chatterjee et al., 2017; Sharma et al., 2024). Although genetic factors have been correlated with the pathogenesis of the disease, environmental factors (consumption of unhealthy diet, reduced physical activity, and obesity) enhance pathophysiological anomalies associated with defective glucose homeostasis (Abolo et al., 2024; Mansour et al., 2023; Ikwuka et al., 2023).
Takeda G protein-coupled receptor 5 (TGR5) is a member of the G protein-coupled receptor (GPCR), class A (Thomas et al., 2008; Guo et al., 2016). TGR5 has emerged as a promising target in the context of T2DM owing to its involvement in glucose homeostasis, energy expenditure, and anti-inflammatory pathways (Sato et al., 2007; Bhimanwar and Mittal, 2021). TGR5 is activated by bile acids and plays a crucial role in regulating metabolic processes in various tissues, including the liver, pancreas, and adipose tissue (Lun et al., 2023)
Despite its potential, development of a TGR5 agonist as a therapeutic agent has faced several challenges. Identifying selective and potent TGR5 agonists is complicated by the structural flexibility of the receptor and the need for compounds that can cross biological membranes and exhibit favourable pharmacokinetic properties. Moreover, many identified TGR5 agonists have off-target effects or are associated with safety concerns, particularly regarding their impact on the gastrointestinal system.
Recent studies have shown the use of multi-omics and transcriptomic data integration approaches to predict potential biomarkers for diseases (Alaya et al., 2024; Ben Aribi et al., 2024; Chikwambi et al., 2023; El Abed et al., 2023; Nzungize et al., 2022; Wesonga and Awe, 2022), as well as to understand disease susceptibility (Nyamari et al., 2023). Other studies have also provided intriguing insights into viral evolution, diversity, and variation using computational approaches (Awe et al., 2023; Mwanga et al., 2023; Obura et al., 2022; Oluwagbemi and Awe, 2018).
In the field of drug discovery, machine learning (ML), molecular docking (MD), and molecular dynamics simulations (MDS) have revolutionized the identification and optimization of novel drug candidates (Di Stefano et al., 2022; Sadybekov and Katritch, 2023). ML models can rapidly analyze vast chemical libraries and predict the bioactivity of compounds with high accuracy, thereby significantly reducing the time and cost associated with traditional drug discovery methods (Di Stefano et al., 2022; Bhimanwar et al., 2023). Molecular docking studies provide insight into the interactions between small molecules and their target receptors, enabling the identification of key binding interactions that contribute to receptor activation or inhibition (Mursal et al., 2024). MDS further refines these predictions by accounting for the dynamic nature of protein-ligand interactions, providing a more realistic assessment of a compound’s stability and efficacy (Ogbodo et al., 2023; Brueckner et al., 2024).
Recent advancements in machine learning have led to the development of sophisticated algorithms capable of learning complex patterns in chemical data, enabling the prediction of bioactive compounds from diverse chemical spaces (van Heerden et al., 2023). In the context of TGR5 agonist discovery, several studies have applied ML techniques to screen compound libraries and predict potential agonists (Qin et al., 2023). Furthermore, molecular docking has been used to explore the binding interactions of the predicted agonists with TGR5 (Sindhu and Srinivasan, 2015). This study aims to contribute to the growing field of TGR5-targeted therapies by providing a systematic and validated approach for the discovery of potential TGR5 agonists.
The workflow pipeline used in this study is summarised in Figure 1.
Figure 1. The workflow pipeline used in this study. It is divided into three parts: Machine learning, molecular docking, and molecular dynamics simulation.
Bioactivity data of compounds with biological activity for TGR5 (CHEMBL5409), which are expressed as EC50 values in nM (nanomolar), was downloaded from the ChEMBL database (https://www.ebi.ac.uk/chembl/) (Bento et al., 2014; Gaulton et al., 2017). ChEMBL is a comprehensive, curated bioactivity database containing information on molecule-target interactions extracted from published literature. The data were cleaned, which involved removing any compounds with missing EC50 values, those without smile notation and redundant bioactivity values. For the data preprocessing step, compounds were classified as active if their values were less than 1,000 nM, and inactive if they were more than 10,000 nM. Values between 1,000 and 10,000 nM were considered intermediate. The intermediate category was removed, leading to an exploratory data analysis that focused only on active and inactive compounds.
The dataset includes chemical names and corresponding SMILES notations, which provide information about the molecular structure used to calculate the molecular descriptors. The drug-likeness of the compounds was assessed based on the pharmacokinetic parameters of absorption, distribution, metabolism, and excretion (ADME). Lipinski’s Rule of Five (Ro5), which states that a compound should have a molecular weight of less than 500 Da, an octanol-water partition coefficient (LogP) of less than 5, fewer than 5 hydrogen bond donors, and fewer than 10 hydrogen bond acceptors, was used to compute the molecular descriptors (Lipinski et al., 2012). Ro5 provides insight into a compound’s potential for absorption in the body, distribution to the appropriate target tissue or organ, metabolism, and eventual excretion from the body. To ensure a more uniform distribution of EC50 data, EC50 values were converted to a negative logarithmic scale (i.e., -log10), resulting in the pEC50 metric.
Selected molecular descriptors were used as input features to build the model. The model was built using the Random Forest algorithm to distinguish between agonists and nonagonists. Fingerprint descriptors were generated using PaDEL (Yap, 2011), and data matrices were prepared accordingly. Features with low variance were removed from the dataset and divided in an 80:20 ratio for training purposes. To prevent potential bias arising from a single data split in constructing predictive models, the models were developed using 100 independent data splits (Puzyn et al., 2011). The optimised ML model was deployed in the form of an offline application using Streamlit to screen the COCONUT (COlleCtion of Open Natural prodUcTs) database (https://coconut.naturalproducts.net), predicting potential TGR5 agonists based on their molecular features (Sorokina et al., 2021). More than four hundred thousand natural compounds that have been sourced from open and free sources are stored in the COCONUT database (Capecchi and Reymond, 2021).
The SMILES of these compounds were obtained and converted to the 2D format using Datawarrior and prepared using the LigPrep module in Schrödinger. This tool was employed to generate the most probable protonation states at physiological pH (7.0 ± 2.0), ensure the correct stereochemistry, and minimise the energy of the ligand structures using the OPLS4 force field.
The crystal structure of the TGR5 receptor (PDBID:7CFN) (Yang et al., 2020) was downloaded from the Protein Data Bank (PDB) and imported into Maestro (Schrodinger, 2021). The Protein Preparation Wizard was used to prepare the downloaded protein, which involved removing water molecules beyond 5 Å from the binding site, adding missing hydrogen atoms, assigning proper bond orders, adjusting protonation states of ionisable residues, and minimising the receptor using the OPLS4 force field to relieve steric clashes and optimise geometry. A grid box was generated around the active site where the ligands were docked.
The prepared ligands were docked into the active site of TGR5 using the Glide tool in Schrödinger (Schrodinger, 2021). SP (standard precision) and XP (extra-precision) protocols were applied. The results were analysed by examining their binding energies to TGR5. 295 compounds had lower binding energies compared to the co-crystallised ligand. The top 4 ligands with the lowest docking scores were selected for succeeding molecular dynamics simulations.
The docking protocol was validated by re-docking the co-crystallised ligand into the active site of the TGR5 protein and calculating the RMSD of the two poses (Shivanika et al., 2020).
The simulation system was prepared using the Desmond System Setup tool. The TGR5-ligand complexes obtained from docking studies were embedded in a POPC (300k) membrane bilayer. Appropriate ions were added to neutralise the system. Energy minimisation was performed to remove any steric clashes, followed by equilibration to stabilise the temperature and pressure of the system.
The protein-ligand complex was solvated in an orthorhombic simulation box filled with explicit TIP3P water molecules. The buffer distance between the complex and edge of the simulation box was set to 10 Å to avoid boundary effects, and 0.15 M NaCl was added to neutralise the system and mimic physiological conditions. The OPLS4 force field was applied to describe the interactions between atoms in the system, including the protein, ligand, and solvent molecules. Before carrying out MD simulation, energy minimisation was performed to remove any steric clashes or bad contacts introduced during the system setup.
The simulations were conducted under a constant number of particles, pressure (1 atm), and temperature (300 K) using the Desmond module of the Schrodinger software. The model system was relaxed before simulation and equilibrated, after which a 100 ns production run was carried out, with coordinates recorded every 100 ps for subsequent analysis. The simulation trajectory was monitored to ensure system stability throughout the run. MDS was carried out on a GPU-enabled Linux operating system.
The trajectory was analysed to assess the binding stability, interaction energy, and conformational dynamics of the TGR5-ligand complexes. This analysis helps to identify the most promising TGR5 agonist for further experimental validation. The simulation trajectories were analysed using the simulation interaction diagram tool in Schrödinger. Key metrics included:
- Root Mean Square Deviation (RMSD): To evaluate the stability of the protein-ligand complex.
- Root Mean Square Fluctuation (RMSF): To analyse the flexibility of individual residues in the receptor.
- Radius of gyration (RoG): To measure the extendedness of a ligand, it is equivalent to its principal moment of inertia.
- Intramolecular hydrogen bonds (intraHB): the number of internal hydrogen bonds within a ligand molecule.
- Ligand-Protein interactions: To monitor the types of interactions (e.g., hydrogen bonds, hydrophobic contacts) between the ligand and receptor throughout the simulation.
A total of 518 active, 187 inactive, and 190 intermediate compounds were identified after the data preparation step (Figure 2A). The two bioactivity classes span similar chemical spaces, as shown by the scatter plot of MW vs. LogP (Figure 2B). Considering the pEC50 values (Figure 2C), the actives and inactives displayed statistically significant differences, which was to be expected since threshold values (EC50 < 1000 nM = Actives and EC50 > 10,000 nM = Inactives, corresponding to pEC50 > 6 = Actives and pEC50 < 5 = Inactives) were used to define actives and inactives.
Figure 2. (A) Frequency plot of the two bioactivity classes (B) scatter plot of MW versus LogP and (C) box plot showing the distribution of pEC50 values of the two bioactivity classes.
Figure 3 displays the box plots of Lipinski’s descriptors. Of the four Lipinski descriptors (MW, LogP, NumHDonors, and NumHAcceptors), only LogP exhibited no difference between the actives and inactives, while the other three descriptors (MW, NumHDonors, and NumHAcceptors) showed statistically significant differences between the active and inactive groups (Table 1).
Figure 3. Box plots of TGR5 agonists using Lipinski’s descriptors: LogP, molecular weight (MW), number of hydrogen acceptors (NumHAcceptors), and number of hydrogen donors (NumHDonors).
Figure 4A shows the resulting scatterplot of the regression model built using the random forest algorithm. The regression model score (r2) is given as 0.40. Figure 4B shows the predicted pEC50 values of the training data. The mean squared error (MSE) and coefficient of determination (R2) for model performance are 0.34 and 0.80, respectively. Figure 5 shows a visual representation of the model performance. This shows that the model had a high r2 and low root-mean-square error value.
Figure 4. Scatter plots of (A) regression model using random forest algorithm (B): experimental vs. predicted pEC50 for training data.
Figure 5. Comparison of the performance of machine learning algorithms against R-squared (A), RMSE (B), and time taken (C), respectively.
ML-based prediction identified 340,364 compounds with potential activity towards TGR5 having EC50 values ranging from 4.0–6.9. Only compounds with EC50 values between 4.0 and 4.9 were selected for docking, yielding 6,656 compounds in total. The bioactivity predictions of just the four top-scoring compounds selected in this study are displayed in Table 2.
Table 2. Predicted EC50 values of the four top-scoring compounds from the screening of the COCONUT database.
Figure 6 shows the 3D structure of TGR5 protein downloaded from the protein data bank (PDBID: 7CFN) in complex with its co-crystallized ligand. After re-docking the co-crystalized ligand into the TGR5 active site, the calculated RMSD value between the docked and re-docked pose was given as 1.42 Å (Figure 7A). Figure 7B shows the docked scores of the top four-scoring compounds (also referred to as lead compounds), represented by their COCONUT IDs, CNP0209363, CNP0424850, CNP0417335, CNP0224616, and co-crystalized ligand, given as −15.39, −14.87, −14.17, −14.01, and −9.01 kcal/mol, respectively. Figure 8 shows the 2D structure of the lead compounds. All the lead compounds contain an acetal/aminal-like group (X-CH(R)-Y, where X, Y are N, S, or O) that may be acid/base labile, releasing an aldehyde. CNP0417335 and CNP0224616 have an ester group and may undergo hydrolysis at high or low pH.
Figure 7. (A) Validation of docking protocol. The co-crystalized ligand (in grey) was redocked (in orange) into the active site of the TGR5 protein and superimposed. The calculated RMSD value between the native and re-docked pose was calulated as 1.42 Å. (B) Bar chart showing the docked scores of the lead compounds and the co-crystallized ligand.
Figure 8. 2D structures of the lead compounds from the COCONUT database (A) CNP0209363, (B) CNP0424850, (C) CNP0417335, (D) CNP0224616.
Figure 9 shows the molecular interactions of the compounds with the amino acid residues found within the TGR5 binding pocket. An overview of the interactions is provided in Table 3. We observed that all the lead compounds formed hydrogen bonding with residue Asn93 during molecular docking.
Figure 9. (A) 3D conformations of the compounds within the active site of TGR5, represented as coloured sticks: orange (INT-777), purple (CNP0209363), red (CNP0424850), green (CNP0417335) and blue (CNP0224616). (B) 2D molecular interaction diagrams of INT-777 (a), lead compounds (b. CNP0209363, c. CNP0424850, d. CNP0417335, e. CNP0224616) and TGR5. Hydrogen and hydrophobic bonds are shown. All the lead compounds show binding to residue Asn93.
Table 3. Overview of interacting amino acid residues and bond types of the compounds and TGR5 from molecular docking studies.
The kinetics of the TGR5-compound complex was investigated using molecular dynamics simulations to assess the bond configuration stability after the binding of lead compounds within the protein cavity. Simulations were conducted over a 100 ns period for the co-ligand (INT-777) and the four lead compounds. The thermodynamic stability of these complex systems was analysed using three key parameters: root mean square deviation (RMSD), root mean square fluctuation (RMSF), and radius of gyration (RoG), all of which were monitored throughout the molecular dynamics simulation.
As shown in Figure 10, the RMSD of the TGR5 protein in its apo state demonstrated instability throughout the 100 ns simulation but became more stable upon binding to the co-ligand. Moreover, when the TGR5 protein was bound to the lead compounds, stability was observed within the range of 1 Å to 3 Å after the 25 ns mark.
Figure 10. RMSD trajectories of TGR5 in the apo state and complex with INT-777, CNP0209363, CN 0424850, CNP0417335, and CNP0224616. Simulations were performed over a 100 ns (nanosecond) duration.
Among the lead compounds, CNP0224616 exhibited the highest stability, with an RMSD value of approximately 0.8 Å, compared to the co-ligand (INT-777), which showed an RMSD of 0.6 Å CNP0209363, however, displayed lower stability, with its RMSD fluctuating between 1 Å and 2.2 Å, throughout the 100 ns simulation. Meanwhile, CNP0417335 and CNP0424850 stabilised after 10 ns and 25 ns, with respective RMSD values of about 1.8 Å and 2.7 Å (Figure 10).
RMSF values provide insight into the magnitude of fluctuations for each residue in a protein; higher RMSF values indicate greater flexibility, and lower values suggest rigidity. Figure 11 shows that RMSF values between 2 Å and 5 Å were recorded during the 100 ns simulation, and the RMSF profiles of the co-ligand (INT-777) were comparable to those of the lead compounds.
Figure 11. RMSF trajectories of TGR5 in the apo state and complex with INT-777, CNP0209363, CNP0424850, CNP0417335 and CNP0224616.
Another parameter used to assess structural stability is the radius of gyration (RoG). Figure 12 illustrates the stability trends of the lead compounds and the co-ligand. CNP0417335 and CNP0224616 initially displayed slight fluctuations during the first 10 ns but stabilised for the remainder of the simulation, similar to the co-ligand, which remained stable around 4.6 Å. CNP0417335 and CNP0224616 stabilised at 4.7 Å and 4.6 Å, respectively. In contrast, CNP0424850 reached stability only after 50 ns, with a value close to 5 Å, while CNP0209363 showed little to no stability throughout the simulation.
Figure 13 shows the intramolecular hydrogen bonds within the compounds. Only the lead compounds showed intramolecular hydrogen bonding up to a magnitude of 4. INT-777 showed no intramolecular hydrogen bonding during the simulation run.
Figure 13. Only the lead compounds showed the presence of intramolecular hydrogen bonds present within the compounds. Only the lead compounds showed the presence of hydrogen bonds.
Figure 14 shows the distribution of hydrogen bonds, hydrophobic bonds, ionic bonds and water bridges formed between the compounds and TGR5 during MDS. All the compounds showed binding to TGR5 via hydrogen bonds, hydrophobic bonds, and water bridges. Only INT-777, CNP0424850 and CNP0224616 showed ionic bonding via Gln253 residue.
Figure 14. Protein-ligand contacts between TGR5 and the compounds during simulation run. The figure shows protein interactions with the ligand. The interactions are categorized into hydrogen bonds, hydrophobic bond, ionic bonds and water bridges. The bar charts show the amount of time a specific interaction is sustained.
Figure 15 shows the ligand-protein contacts made during simulation. An overview of the interactions is provided in Table 4. All the compounds showed binding with the Ser247 residue; this interaction was also observed from molecular docking studies. Only CNP0424850 and CNP0417335 showed pi-pi stacking.
Figure 15. Ligand-protein contacts between the compounds and TGR5 during simulation run (A) INT-777; (B) CNP0209363; (C) CNP0424850; (D) CNP0417335; (E) CNP0224616. The figure shows a schematic detail of the interactions that occur for more than 30% of the simulation time.
Table 4. Overview of the interacting amino acid residues and bond types of the compounds and TGR5 during MDS.
Type 2 diabetes is a leading cause of mortality (Abdul Basith Khan et al., 2020). Despite the therapeutic advancement in this disease management, imbalance in glucose homeostasis and energy expenditure associated with the progression of the diseases remains a challenge (Mirzadeh et al., 2022; Reed et al., 2021; Büsing et al., 2019; Stein et al., 2013). In this study, predictive machine learning-based models, molecular docking, and molecular dynamics simulation were used in the identification of TGR5 agonists for the management of type 2 diabetes.
Compounds with their corresponding EC50 values exhibiting biological activity towards TGR5 were downloaded from the ChEMBL database. Considering the biological activity of the compounds, they were characterised as either active or inactive. The ML evaluation of TGR5 agonists showed that the molecular weight (MW), number of hydrogen bond donors (nHDonors), and number of hydrogen bond acceptors (nHAcceptors) were the significant descriptors between active and inactive compounds (Sasaki et al., 2023). This observation can be further compared with drug-likeness principles such as the Lipinski’s Rule of Five, where smaller MW and ideal hydrogen bonding are efficient for pharmacokinetics and biopharmaceutical availability (Brueckner et al., 2024). Nevertheless, some bioactive natural compounds have higher molecular weight (Feher and Schmidt, 2003; Clardy and Walsh, 2004). Besides meeting the Ro5 criteria, natural products with high molecular masses have penetrated the pharmaceutical markets as approved oral drugs (Shultz, 2018; Price et al., 2024).
Active site residues of the crystal structure of TGR5 in complex with its co-crystallised ligand, INT-777, are Leu74, Tyr89, Phe161, Leu166, Tyr240, Thr 243, Leu244, Ser247, Tyr251, Leu262, Leu263, Leu266, and Ser270 as reported by Yang et al. (2020). The docking results show that all the compounds were positioned within the active site of TGR5. It also showed that hydrogen bonding and hydrophobic interactions are important in TGR5 receptor and agonist binding. Particularly, residues such as Tyr240 and Asp348 were predicted to be critical in stabilising the ligand-receptor complex, supporting previous findings on TGR5 activation (Guo et al., 2016). Nevertheless, the flexibility of that binding site remains a major problem for predicting the binding affinities, and to overcome that problem, dynamic studies are required in order to capture the conformational changes of the receptor upon the ligand binding (Mursal et al., 2024).
Molecular dynamics simulations further validated the stability of these interactions, showing that the identified lead compounds formed stable complexes with TGR5 throughout the simulation period. RMSD and RMSF are critical indicators of structural stability and flexibility for a simulation (Ahmad et al., 2020). According to Sindhu and Srinivasan (2015), smaller RMSD values for backbone atoms suggest that the predicted structural models closely match experimental data, indicating higher model accuracy. In contrast, larger RMSD values point to greater deviations and reduced accuracy. This is important in an effort to document the idea that potential drugs do not relinquish their efficiency when exposed to tangible physiology (Brueckner et al., 2024). Simulation studies show compound stability within the TGR5 binding pocket, especially for CNP0417335 and CNP0224616; however, in vivo and/or in vitro experimental validation is necessary to determining the pharmacokinetic and toxicity profiles of these compounds in biological systems. Intramolecular hydrogen bonds may have stabilised the bioactive conformation of the ligands, which might have led to stronger association observed between the lead compounds and TGR5. These bonds could have acted by lowering the translational and conformational entropy during binding (Davoren et al., 2016), resulting in lower binding energies. Water bridges are also an excellent way to manage protein-ligand complexes; these bridges exist where one or more water molecules are present between the protein and the ligand. Water bridges could have facilitated the formation of a water tunnel in TGR5 during the simulation, as mentioned by Olaposi et al. (2019), leading to the stability of the complexes.
The development of effective TGR5 agonists has been hampered due to gastrointestinal side effects (Zhuo et al., 2024). For instance, INT-777 was found to activate TGR5; however, when tested in the first phases, it was discovered that it poses negative effects on the gastrointestinal tract (Guo et al., 2016). For this reason, there is a need to find selective agonists that do not possess such undesired activity.
Machine learning has enhanced drug discovery and development by increasing efficiency and prediction accuracy. Incorporating big chemical data together with artificial neural network algorithms has enhanced the speed and accuracy of the predictions compared to conventional methods (van Heerden et al., 2023). However, as pointed out in this analysis, existing ML models are vastly dependent on the quality and size of data used in their development, and this has reduced the generality of models in practice. Molecular docking using TGR5 as a subject can also be enhanced by the help of special structural techniques such as cryo-electron microscopy or X-ray crystallography to gain more information regarding the active conformation of the receptor. The integration of these experimental methodologies with MD simulations may improve the reliability of the binding energy predictions and would be beneficial for the design of more selective TGR5 agonists (Brueckner et al., 2024).
According to this study, new TGR5 agonists for T2D could be developed via ML, MD and MDS techniques. Interestingly, the computational methods studied here illuminate new directions in the search for TGR5 agonists; the actual effectiveness of these approaches remains contingent on the experimental testing of predicted compounds. The integration of these techniques will provide a framework for designing novel TGR5 agonists, and improve the accuracy of identification of lead compounds for T2D treatment.
The dataset analyzed during the current study as a case study is publicly available at https://github.com/omicscodeathon/tgr5t2d/tree/main/data. The data supporting the results reported in this manuscript is included within the article and its additional files. The generated progress reports are in HTML format and can be viewed using any preferred browser such as Chrome, Safari, Internet Explorer and Firefox. The Project repository which also includes the entire code and other requirements can be downloaded from https://github.com/omicscodeathon/tgr5t2d. The guidelines for implementing this tool and related updates, are available at: https://github.com/omicscodeathon/tgr5t2d/blob/main/README.md.
OE: Conceptualization, Data curation, Formal Analysis, Investigation, Methodology, Project administration, Resources, Validation, Visualization, Writing–original draft, Writing–review and editing. CO: Writing–original draft, Writing–review and editing, Investigation, Project administration. HN: Data curation, Methodology, Resources, Writing–original draft, Writing–review and editing. OK: Writing–review and editing, Writing–original draft. AM: Writing–review and editing, Data curation, Formal Analysis, Software. FM: Writing–review and editing. AY: Writing–review and editing. OA: Project administration, Supervision, Writing–review and editing, Resources.
The author(s) declare that no financial support was received for the research, authorship, and/or publication of this article.
The authors thank the National Institutes of Health (NIH) Office of Data Science Strategy (ODSS) for their immense support before and during the October 2024 Omics codeathon organized by the African Society for Bioinformatics and Computational Biology (ASBCB).
The authors declare that the research was conducted in the absence of any commercial or financial relationships that could be construed as a potential conflict of interest.
The author(s) declare that no Generative AI was used in the creation of this manuscript.
All claims expressed in this article are solely those of the authors and do not necessarily represent those of their affiliated organizations, or those of the publisher, the editors and the reviewers. Any product that may be evaluated in this article, or claim that may be made by its manufacturer, is not guaranteed or endorsed by the publisher.
Abdul Basith Khan, M., Hashim, M. J., King, J. K., Govender, R. D., Mustafa, H., and Al Kaabi, J. (2020). Epidemiology of type 2 diabetes—global burden of disease and forecasted trends. J. Epidemiol. Glob. health 10 (1), 107–111. doi:10.2991/jegh.k.191028.001
Abolo, L., Ssenkaali, J., Mulumba, O., and Awe, O. I. (2024). Exploring the causal effect of omega-3 polyunsaturated fatty acid levels on the risk of type 1 diabetes: a Mendelian randomization study. Front. Genet. 15, 1353081. doi:10.3389/fgene.2024.1353081
Ahmad, S. S., Sinha, M., Ahmad, K., Khalid, M., and Choi, I. (2020). Study of caspase 8 inhibition for the management of Alzheimer’s disease: a molecular docking and dynamics simulation. Molecules 25 (9), 2071. doi:10.3390/molecules25092071
Alaya, F., Baraket, G., Adediran, D. A., Cuttler, K., Ajiboye, I., Kivumbi, M. T., et al. (2024). Multiple sclerosis stages and their differentially expressed genes: a bioinformatics analysis. bioRxiv. doi:10.1101/2024.01.20.576448
Awe, O. I., En Najih, N., Nyamari, M. N., and Mukanga, L. B. (2023). Comparative study between molecular and genetic evolutionary analysis tools using African SARS-CoV2 variants. Inf. Med. Unlocked 36, 101143. doi:10.1016/j.imu.2022.101143
Ben Aribi, H., Abassi, N., and Awe, O. I. (2024). NeuroVar: an open-source tool for gene expression and variation data visualization for biomarkers of neurological diseases. Gigabyte. doi:10.46471/gigabyte.143
Bento, A. P., Gaulton, A., Hersey, A., Bellis, L. J., Chambers, J., Davies, M., et al. (2014). The ChEMBL bioactivity database: an update. Nucleic acids Res. 42 (D1), D1083–D1090. doi:10.1093/nar/gkt1031
Bhatti, J. S., Sehrawat, A., Mishra, J., Sidhu, I. S., Navik, U., Khullar, N., et al. (2022). Oxidative stress in the pathophysiology of type 2 diabetes and related complications: current therapeutics strategies and future perspectives. Free Radic. Biol. Med. 184, 114–134. doi:10.1016/j.freeradbiomed.2022.03.019
Bhimanwar, R. S., Lokhande, K. B., Shrivastava, A., Singh, A., Chitlange, S. S., and Mittal, A. (2023). Identification of potential drug candidates as TGR5 agonist to combat type II diabetes using in silico docking and molecular dynamics simulation studies. J. Biomol. Struct. Dyn. 41 (22), 13314–13331. doi:10.1080/07391102.2023.2173654
Bhimanwar, R. S., and Mittal, A. (2021). TGR5 agonists for diabetes treatment: a patent review and clinical advancements (2012-present). Expert Opin. Ther. Pat. 32 (2), 191–209. doi:10.1080/13543776.2022.1994551
Brueckner, A., Shields, B., Kirubakaran, P., Suponya, A., Panda, M., Posy, S., et al. (2024). MDFit: automated molecular simulations workflow enables high throughput assessment of ligands-protein dynamics. ChemRxiv 38, 24. doi:10.1007/s10822-024-00564-2
Büsing, F., Hägele, F. A., Nas, A., Hasler, M., Müller, M. J., and Bosy-Westphal, A. (2019). Impact of energy turnover on the regulation of glucose homeostasis in healthy subjects. Nutr. Diabetes 9, 22. doi:10.1038/s41387-019-0089-6
Capecchi, A., and Reymond, J. L. (2021). Classifying natural products from plants, fungi or bacteria using the COCONUT database and machine learning. J. Cheminform 13, 82. doi:10.1186/s13321-021-00559-3
Chatterjee, S., Khunti, K., and Davies, M. J. (2017). Type 2 diabetes. lancet 389 (10085), 2239–2251. doi:10.1016/S0140-6736(17)30058-2
Chikwambi, Z., Hidjo, M., Chikondowa, P., Afolabi, L., Aketch, V., Jayeoba, G., et al. (2023). Multi-omics data integration approach identifies potential biomarkers for Prostate cancer. bioRxiv. doi:10.1101/2023.01.26.522643
Clardy, J., and Walsh, C. (2004). Lessons from natural molecules. Nature 432, 829–837. doi:10.1038/nature03194
Davoren, J. E., O’Neil, S. V., Anderson, D. P., Brodney, M. A., Chenard, L., Dlugolenski, K., et al. (2016). Design and optimization of selective azaindole amide M1 positive allosteric modulators. Bioorg. and Med. Chem. Lett. 26 (2), 650–655. doi:10.1016/j.bmcl.2015.11.053
DeFronzo, R., Ferrannini, E., Groop, L., Henry, R. R., Herman, W. H., Holst, J. J., et al. (2015). Type 2 diabetes mellitus. Nat. Rev. Dis. Prim. 1, 15019. doi:10.1038/nrdp.2015.19
Deol, R., and Bashir, S. (2024). Exploring the complications of type 2 diabetes mellitus: pathophysiology and management strategies. EPRA Int. J. Res. Dev. (IJRD) 9 (7), 173–182. doi:10.36713/epra17838
Di Stefano, M., Galati, S., Ortore, G., Caligiuri, I., Rizzolio, F., Ceni, C., et al. (2022). Machine learning-based virtual screening for the identification of CDK5 inhibitors. Int. J. Mol. Sci. 23 (18), 10653. doi:10.3390/ijms231810653
El Abed, F., Baraket, G., Nyamari, M. N., Naitore, C., and Awe, O. I. (2023). Differential expression analysis of miRNAs and mRNAs in epilepsy uncovers potential biomarkers. bioRxiv. doi:10.1101/2023.09.11.557132
Feher, M., and Schmidt, J. M. (2003). Property distributions: differences between drugs, natural products, and molecules from combinatorial chemistry. J. Chem. Inf. Comput. Sci. 43 (1), 218–227. doi:10.1021/ci0200467
Gaulton, A., Hersey, A., Nowotka, M., Bento, A. P., Chambers, J., Mendez, D., et al. (2017). The ChEMBL database in 2017. Nucleic acids Res. 45 (D1), D945–D954. doi:10.1093/nar/gkw1074
Guo, C., Chen, W. D., and Wang, Y. D. (2016). TGR5, not only a metabolic regulator. Front. physiology 7, 646. doi:10.3389/fphys.2016.00646
Ikwuka, A. O., Omoju, D. I., and Mahanera, O. K. (2023). Profiling of clinical dynamics of type 2 diabetes mellitus in patients: a perspective review. World J. Med. Pharm. Res. 5 (5), 210–218. doi:10.37022/wjcmpr.v5i5.294
Lipinski, C. A., Lombardo, F., Dominy, B. W., and Feeney, P. J. (2012). Experimental and computational approaches to estimate solubility and permeability in drug discovery and development settings. Adv. drug Deliv. Rev. 64, 4–17. doi:10.1016/j.addr.2012.09.019
Lun, W., Yan, Q., Guo, X., Zhou, M., Bai, Y., He, J., et al. (2024). Mechanism of action of the bile acid receptor TGR5 in obesity. Acta Pharm. Sin. B 14 (2), 468–491. doi:10.1016/j.apsb.2023.11.011
Mansour, A., Mousa, M., Abdelmannan, D., Tay, G., Hassoun, A., and Alsafar, H. (2023). Microvascular and macrovascular complications of type 2 diabetes mellitus: exome wide association analyses. Front. Endocrinol. 14, 1143067. doi:10.3389/fendo.2023.1143067
Mirzadeh, Z., Faber, C. L., and Schwartz, M. W. (2022). Central nervous system control of glucose homeostasis: a therapeutic target for type 2 diabetes? Annu. Rev. Pharmacol. Toxicol. 62, 55–84. doi:10.1146/annurev-pharmtox-052220-010446
Mursal, M., Ahmad, M., Hussain, S., and Khan, M. F. (2024). Navigating the computational seas: a comprehensive overview of molecular docking software in drug discovery. IntechOpen. doi:10.5772/intechopen.1004802
Mwanga, M. J., Obura, H. O., Evans, M., and Awe, O. I. (2023). Enhanced deep convolutional neural network for SARS-CoV-2 variants classification. bioRxiv. doi:10.1101/2023.08.09.552643
Nyamari, M. N., Omar, K. M., Fayehun, A. F., Dachi, O., Bwana, B. K., and Awe, O. I. (2023). Expression level analysis of ACE2 receptor gene in african-American and non-african-American COVID-19 patients. BioRxiv. doi:10.1101/2023.09.11.557129
Nzungize, L., Kengne-Ouafo, J. A., Wesonga, M. R., Umuhoza, D., Murithi, K., Kimani, P., et al. (2022). Transcriptional profiles analysis of COVID-19 and malaria patients reveals potential biomarkers in children. bioRxiv. doi:10.1101/2022.06.30.498338
Obura, H. O., Mlay, C. D., Moyo, L., Karumbo, B. M., Omar, K. M., Sinza, E. M., et al. (2022). Molecular phylogenetics of HIV-1 subtypes in african populations: a case study of sub-saharan african countries. bioRxiv. doi:10.1101/2022.05.18.492401
Ogbodo, U. C., Enejoh, O. A., Okonkwo, C. H., Gnanasekar, P., Gachanja, P. W., Osata, S., et al. (2023). Computational identification of potential inhibitors targeting cdk1 in colorectal cancer. Front. Chem. 11, 1264808. doi:10.3389/fchem.2023.1264808
Olaposi, O. I., Oyekanmi, N., Samuel, M. D., Enejoh, O. A., Victor, U. O., and Niyi, A. (2019). Takeda G-protein receptor (TGR)-5 evolves classical active-state conformational signatures in complex with chromolaena odorata-derived flavonoid-5, 7-dihydroxy-6-4-dimethoxyflavanone. Curr. Chem. Biol. 13 (3), 212–222. doi:10.2174/2212796813666190102102018
Oluwagbemi, O., and Awe, O. I. (2018). A comparative computational genomics of Ebola Virus Disease strains: in-silico Insight for Ebola control. Inf. Med. Unlocked 12, 106–119. doi:10.1016/j.imu.2018.07.004
Ong, K. L., Stafford, L. K., McLaughlin, S. A., Boyko, E. J., Vollset, S. E., Smith, A. E., et al. (2023). Global, regional, and national burden of diabetes from 1990 to 2021, with projections of prevalence to 2050: a systematic analysis for the Global Burden of Disease Study 2021. Lancet 402 (10397), 203–234. doi:10.1016/s0140-6736(23)01301-6
Price, E., Weinheimer, M., Rivkin, A., Jenkins, G., Nijsen, M., Cox, P. B., et al. (2024). Beyond rule of five and PROTACs in modern drug discovery: polarity reducers, chameleonicity, and the evolving physicochemical landscape. J. Med. Chem. 67 (7), 5683–5698. doi:10.1021/acs.jmedchem.3c02332
Puzyn, T., Mostrag-Szlichtyng, A., Gajewicz, A., Skrzyński, M., and Worth, A. P. (2011). Investigating the influence of data splitting on the predictive ability of QSAR/QSPR models. Struct. Chem. 22 (4), 795–804. doi:10.1007/s11224-011-9757-4
Qin, T., Gao, X., Lei, L., Feng, J., Zhang, W., Hu, Y., et al. (2023). Machine learning-and structure-based discovery of a novel chemotype as FXR agonists for potential treatment of nonalcoholic fatty liver disease. Eur. J. Med. Chem. 252, 115307. doi:10.1016/j.ejmech.2023.115307
Reed, J., Bain, S., and Kanamarlapudi, V. (2021). A review of current trends with type 2 diabetes epidemiology, aetiology, pathogenesis, treatments and future perspectives. Diabetes, Metabolic Syndrome Obes. 14, 3567–3602. doi:10.2147/DMSO.S319895
Sadybekov, A. V., and Katritch, V. (2023). Computational approaches streamlining drug discovery. Nature 616, 673–685. doi:10.1038/s41586-023-05905-z
Sasaki, T., Ikari, N., Hashimoto, S., and Sato, R. (2023). Identification of α-ionone, nootkatone, and their derivatives as TGR5 agonists. Biochem. Biophysical Res. Commun. 653, 147–152. doi:10.1016/j.bbrc.2023.02.070
Sato, H. C., Genet, A., Strehle, A., Thomas, C., Lobstein, A., Wagner, A., et al. (2007). Anti-hyperglycemic activity of a TGR5 agonist isolated from Olea europaea. Biochem. Biophysical Res. Commun. 362 (4), 793–798. doi:10.1016/j.bbrc.2007.06.130
Schrodinger (2021). Maestro version 12.8.117 release 2021-2: Glide. New York, NY, USA: Schrödinger LLC.
Sharma, U. K., Pujani, M., and Anuradha, J. (2024). Type-II-diabetes mellitus: etiology, epidemiology, risk factors and diagnosis and insight into demography (urban versus rural). Int. J. Health Sci. Res. 14 (1), 283–290. doi:10.52403/ijhsr.20240136
Shivanika, C., Kumar, D., Ragunathan, V., Tiwari, P., Sumitha, A., and P, B. D. (2020). Molecular docking, validation, dynamics simulations, and pharmacokinetic prediction of natural compounds against the SARS-CoV-2 main-protease. J. Biomol. Struct. and Dyn. 1, 1–27. doi:10.1080/07391102.2020.1815584
Shultz, M. D. (2018). Two decades under the influence of the rule of five and the changing properties of approved oral drugs: miniperspective. J. Med. Chem. 13 (4), 1701–1714. doi:10.1021/acs.jmedchem.8b00686
Sindhu, T., and Srinivasan, P. (2015). Exploring the binding properties of agonists interacting with human TGR5 using structural modeling, molecular docking and dynamics simulations. RSC Adv. 5 (19), 14202–14213. doi:10.1039/C4RA16617E
Sorokina, M., Merseburger, P., Rajan, K., Yirik, M. A., and Steinbeck, C. (2021). COCONUT online: collection of open natural products database. J. Cheminformatics 13 (1), 2. doi:10.1186/s13321-020-00478-9
Stein, S. A., Lamos, E. M., and Davis, S. N. (2013). A review of the efficacy and safety of oral antidiabetic drugs. Expert Opin. Drug Saf. 12 (2), 153–175. doi:10.1517/14740338.2013.752813
Thomas, C., Pellicciari, R., Pruzanski, M., Auwerx, J., and Schoonjans, K. (2008). Targeting bile-acid signalling for metabolic diseases. Nat. Rev. Drug Discov. 7 (8), 678–693. doi:10.1038/nrd2619
van Heerden, A., Turón, G., Duran-Frigola, M., Pillay, N., and Birkholtz, L.-M. (2023). Machine learning approaches identify chemical features for stage-specific antimalarial compounds. bioRxiv 8, 43813–43826. doi:10.1021/acsomega.3c05664
Wesonga, R. M., and Awe, O. I. (2022). An assessment of traditional and genomic screening in newborns and their applicability for africa. Inf. Med. Unlocked 32, 101050. doi:10.1016/j.imu.2022.101050
Yang, F., Mao, C., Guo, L., Lin, J., Ming, Q., Xiao, P., et al. (2020). Structural basis of GPBAR activation and bile acid recognition. Nature 587 (7834), 499–504. doi:10.1038/s41586-020-2569-1
Yap, C. W. (2011). PaDEL-descriptor: an open source software to calculate molecular descriptors and fingerprints. J. Comput. Chem. 32 (7), 1466–1474. doi:10.1002/jcc.21707
Keywords: TGR5, type 2 diabetes, machine learning, molecular docking, molecular dynamics simulation, COCONUT database
Citation: Enejoh OA, Okonkwo CH, Nortey H, Kemiki OA, Moses A, Mbaoji FN, Yusuf AS and Awe OI (2025) Machine learning and molecular dynamics simulations predict potential TGR5 agonists for type 2 diabetes treatment. Front. Chem. 12:1503593. doi: 10.3389/fchem.2024.1503593
Received: 29 September 2024; Accepted: 13 December 2024;
Published: 09 January 2025.
Edited by:
Marko Jukic, University of Maribor, SloveniaReviewed by:
Titilayo Omolara Johnson, University of Jos, NigeriaCopyright © 2025 Enejoh, Okonkwo, Nortey, Kemiki, Moses, Mbaoji, Yusuf and Awe. This is an open-access article distributed under the terms of the Creative Commons Attribution License (CC BY). The use, distribution or reproduction in other forums is permitted, provided the original author(s) and the copyright owner(s) are credited and that the original publication in this journal is cited, in accordance with accepted academic practice. No use, distribution or reproduction is permitted which does not comply with these terms.
*Correspondence: Ojochenemi A. Enejoh, Y2hlbmVtaWRhbGFAZ21haWwuY29t; Olaitan I. Awe, bGFpdGFuYXdlQGdtYWlsLmNvbQ==
†ORCID: Ojochenemi A. Enejoh, orcid.org/0000-0002-5684-2472; Chinelo H. Okonkwo, orcid.org/0000-0001-5421-8054; Florence N. Mbaoji, orcid.org/0000-0002-9705-3250; Abdulrazak S. Yusuf, orcid.org/0000-0002-7649-4022; Olaitan I. Awe, orcid.org/0000-0002-4257-3611
Disclaimer: All claims expressed in this article are solely those of the authors and do not necessarily represent those of their affiliated organizations, or those of the publisher, the editors and the reviewers. Any product that may be evaluated in this article or claim that may be made by its manufacturer is not guaranteed or endorsed by the publisher.
Research integrity at Frontiers
Learn more about the work of our research integrity team to safeguard the quality of each article we publish.