- 1College of Chemistry and Environmental Engineering, Shenzhen University, Shenzhen, China
- 2Additive Manufacturing Institute, Shenzhen University, Shenzhen, China
- 3Department of Mechanical Engineering, City University of Hong Kong, Kowloon, Hong Kong SAR, China
- 4A. J. Drexel Nanomaterials Institute and Department of Materials Science and Engineering, Drexel University, Philadelphia, PA, United States
- 5Department of Electrical Engineering, Sejong University, Seoul, Republic of Korea
- 6Shenzhen Children’s Hospital, Clinical Medical College of Southern University of Science and Technology, Shenzhen, China
- 7Department of Chemistry, School of Natural Sciences, National University of Sciences and Technology, Islamabad, Pakistan
- 8School of Mechanical Engineering, Dongguan University of Technology, Dongguan, China
Artificial intelligence (AI) has recently emerged as a unique developmental influence that is playing an important role in the development of medicine. The AI medium is showing the potential in unprecedented advancements in truth and efficiency. The intersection of AI has the potential to revolutionize drug discovery. However, AI also has limitations and experts should be aware of these data access and ethical issues. The use of AI techniques for drug discovery applications has increased considerably over the past few years, including combinatorial QSAR and QSPR, virtual screening, and denovo drug design. The purpose of this survey is to give a general overview of drug discovery based on artificial intelligence, and associated applications. We also highlighted the gaps present in the traditional method for drug designing. In addition, potential strategies and approaches to overcome current challenges are discussed to address the constraints of AI within this field. We hope that this survey plays a comprehensive role in understanding the potential of AI in drug discovery.
1 Introduction
It is estimated that 2.6 billion US dollars and over a decade of dedicated work are typically required in the field of drug discovery, which is notorious for its high costs, protracted timelines, and lack of success (Cohen et al., 2024). Several new drugs are approved, but many of these drug candidates subsequently fail. A significant precursor shift occurred in the context of drug discovery itself, enabling the rapid development of rapidly evolving artificial intelligence (AI) (Tripathi et al., 2024; Sarkar et al., 2023). Artificial intelligence has been successfully implemented into drug discovery, encompassing target protein structure identification (Hasselgren and Oprea, 2024), virtual screening (Turon et al., 2023), de novo drug design (Janet et al., 2023), retrosynthesis reaction prediction (Yan et al., 2023), bioactivity and toxicity prediction (Tran et al., 2023), all of which are categorized as predictive and generative processes (Figure 1A). Computer programs designed to emulate human cognitive processes constitute AI, a scientific discipline associated with intelligent machine learning. In this process, data is acquired, systems are constructed for using that data, conclusions are drawn, self-corrections are implemented, and adjustments are made where necessary (Buckner, 2023; Damiano and Stano, 2023; Prasad and Kalavakolanu, 2023; Ratten, 2024). It is generally used for the replication of cognitive tasks performed by humans through machine learning analysis. To conduct accurate analyses and provide meaningful interpretations, the technology relies on a variety of statistical models and computational intelligence (Klauschen et al., 2024). The application and integration of AI technology across diverse industries have become increasingly common in recent years (Ahmadi, 2024). Despite challenges such as shortages of pharmacists (Kilonzi et al., 2024), rising operating costs (Yaiprasert and Hidayanto, 2024), and diminished reimbursements (Pham et al., 2024), pharmacies have successfully met the rising demand for prescriptions during the past quarter-century. Pharmacy has made great strides in improving its workflow efficiency, reducing operating costs, and championing safety, accuracy, and efficiency through technology (Wilde et al., 2024). Besides giving pharmacists more time to direct their attention to a larger patient volume, automated dispensing systems improve health outcomes significantly. Intelligent automation is playing a pivotal role in improving both patient care and the pharmaceutical industry with this fusion of AI technology and pharmacy practices (Anthwal et al., 2024). The drug discovery market is expected to grow rapidly with advances in artificial intelligence technologies as well as their integration into the process as shown by Figure 1B.
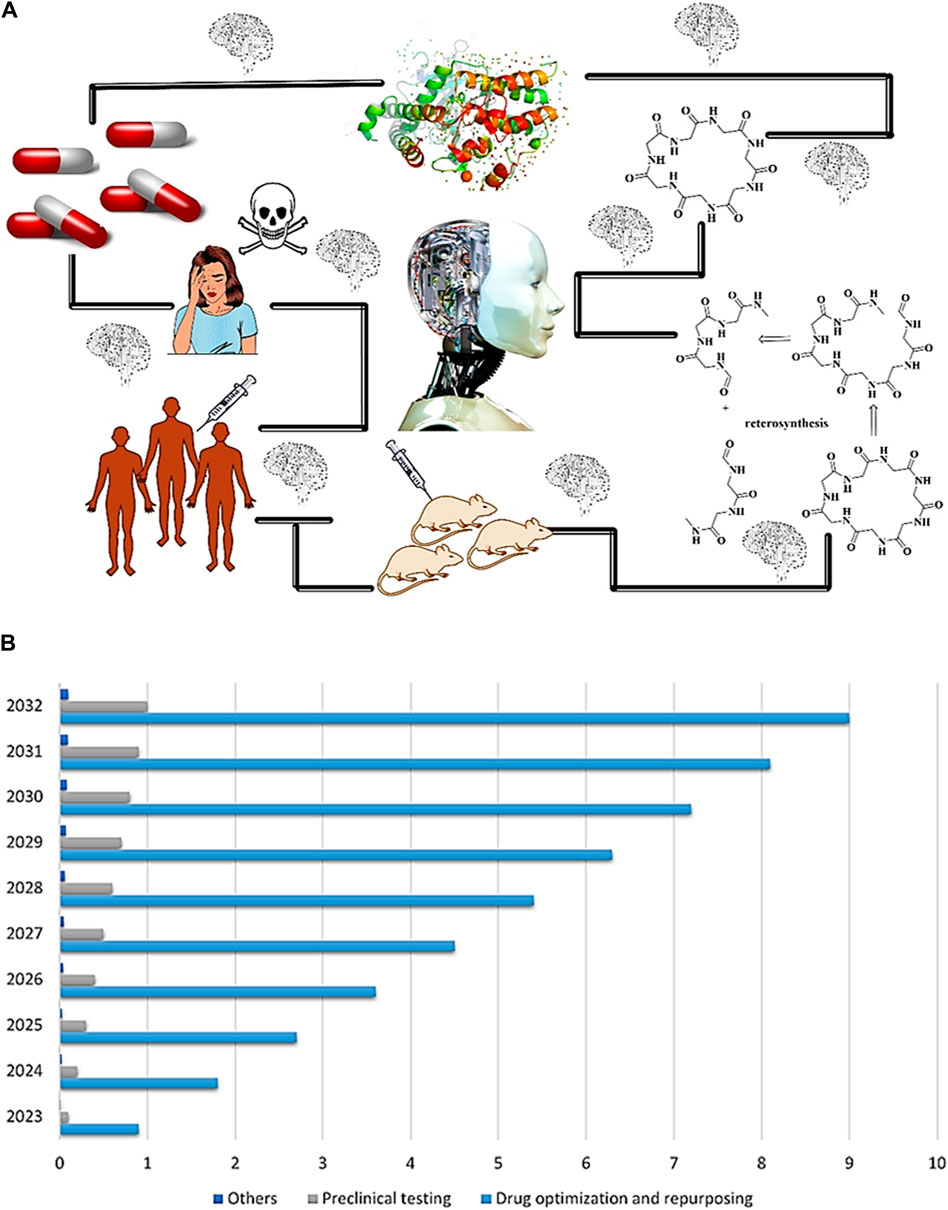
Figure 1. (A) Schematic diagram representing drug development through AI, (B) Significant growth in the US AI market in drug discovery is expected between 2023 and 2032.
2 Overview of artificial intelligence in drug discovery
Recent advances in artificial intelligence and machine learning have ushered in a new era of efficiency in drug discovery. By combining artificial intelligence with machine learning in drug discovery, new documents have been developed to address long-standing challenges associated with traditional drug discovery, and to accelerate the identification of promising drug candidates (Hasselgren and Oprea, 2024; Ramos et al., 2024). In computer science, artificial intelligence (AI) refers to the development of intelligent machines that can perform tasks usually requiring human intelligence. The role of machine learning in drug discovery involves analyzing vast datasets and deriving meaning from them using AI, a subset of machine learning (Kotkondawar et al., 2024).
2.1 Predicting drug efficacy and toxicity through machine learning (ML)
In medicinal chemistry, an important application of artificial intelligence is to predict the efficacy and toxicity of potential drug compounds. As a result, Artificial Intelligence (AI), especially Machine Learning (ML), has emerged as one of the most effective techniques for solving these problems (Alhatem et al., 2024). Analyzing large datasets allows ML algorithms to identify patterns and trends not readily evident to humans. This capability speeds up the identification of not only synthetic small molecules but also new bioactive compounds while minimizing side effects, outpacing the time constraints of traditional protocols (Thenuwara et al., 2023). For example, deep learning (DL) algorithms trained on a dataset of known drugs can predict the activity of new drugs with a high degree of success (Askr et al., 2023). The use of databases of known toxic and non-toxic compounds has enabled AI to make significant contributions to the prevention of the toxicity of potential drug compounds (Yang and Kar, 2023).
In addition to finding drug–drug interactions in patients with different diseases, AI is also essential to identifying altered or adverse reactions caused by multiple drugs being taken together for the same or different diseases (Creecy et al., 2024). The detection of drug interactions is based on AI methods that analyze patterns and trends in large datasets of known interactions. An ML algorithm, for instance, accurately predicts interactions of novel drug pairs (Atas Guvenilir and Doğan, 2023). As part of personalized medicine, AI can identify possible interactions between drugs. As a result, it is easier to develop tailor-made treatment plans based on the characteristics of individual patients, including genetic profiles and drug responses, aligned with personalized medicine, which tailor treatments based on individual characteristics (Blanco-Gonzalez et al., 2023).
2.2 Virtual screening: a lead identification approach
Virtual Screening (VS) serves as a potent methodology for lead identification within the domain of AI-driven drug discovery (Pun et al., 2023). By using this method, millions of compounds similar to drugs or leads are computationally screened against well-characterized proteins. Docking is used to filter ligands based on their affinities for binding (Chisholm et al., 2023; Shiota et al., 2023). These computational hits are then subjected to in vitro testing. Within the realm of AI drug discovery, virtual screening falls into two primary categories: ligand-based virtual screening (LBVS) (Oliveira et al., 2023) and structure-based virtual screening (SBVS) (Kumar and Acharya, 2023). LBVS entails the analysis of biological data to differentiate inactive compounds from active ones (Dragan et al., 2023). A consensus pharmacophore, similarity measure, or various descriptors are then used to identify highly active scaffolds. Conversely, SBVS requires knowledge of the 3D structure of the target protein (Rehman et al., 2023). By using computer algorithms, a target protein is docked with a large library of drug-like compounds available commercially. The docked complex is scored using a scoring function, followed by experimental validation assays (DiFrancesco et al., 2023). An important function of SBVS is scoring ligands. However, unlike ligand-based approaches, the structure-based approach does not rely on pre-existing experimental data (Stevenson et al., 2023).
3 Key technologies in AI–driven drug discovery
In the past decay, drug discovery was a labor-intensive process based on high-throughput screening and trial-and-error experimentation. ML and NLP techniques hold promise for improving the efficiency and effectiveness of analyzing large datasets. Improve accuracy, allowing for more precise and accurate entries through machine learning (ML) and natural language processing (NLP). (Sim et al., 2023). The recent achievements in applying deep learning to predict drug compound efficacy demonstrate AI’s transformative potential in this field. In addition, it has been proven that AI techniques are capable of projecting the criminal capabilities of an individual, showing the potential to interfere with the effectiveness of drug discovery and processing (Yang and Kar, 2023). Clearly, it is possible and research is needed on how AI can be used to create new bioactives, despite these advances and with challenges and limitations, including ethical ones. Medical advances in the future are driven in large part by artificial intelligence.
It refers to any computer or machine exhibiting responsiveness or intelligence, indicating human-like speed or intelligence, often called robotics or automation. Robotic systems are designed to perform complex repetitive tasks, while artificial intelligence is concerned with giving computers or machines the ability to think like humans (Wardat et al., 2024). As a branch of computer science, artificial intelligence (AI) aims to develop machines that can learn (Sanchez et al., 2024), organize (Nebreda et al., 2024), problem solve (Sanchez et al., 2024), sense like humans. (Akour et al., 2024), and language (Singh and Khatun, 2024) with similar success. In its current form, narrow AI, also known as weak AI, is designed for specialized tasks such as web search, face and voice recognition, and self-examination (Thangavel et al., 2024). Ultimately, the AI community wants to develop machines capable of performing all cognitive tasks better than humans, which would lead to the development of a strong or general AI.
3.1 A fusion of quantitative structure-activity relationship (QSAR), quantitative structure-property relationship (QSPR) and structure-based modeling
In the ever-evolving landscape of drug design, Artificial Intelligence (AI) combined with Quantitative Structure-Activity Relationship (QSAR), Quantitative Structure-Property Relationship (QSPR), and Structure-Based, has steadily gained ground in the 50 years. QSPR has proven its worth in guiding drug discovery, having proven its potential in predicting biological action and pharmacokinetic parameters (Zeng et al., 2024). As shown in Supplementary Figure S1. Traditionally reliant on simpler models, the field has progressively embraced universally applicable machine learning techniques such as support vector machines (Yin et al., 2024) and gradient boosting methods (Chellaswamy et al., 2024). Simultaneously, the resurgence of deep learning has brought forth advancements, with graph neural networks and recurrent neural networks offering automatic feature extraction capabilities (Philippe et al., 2024). This has made it possible to model complex molecular structures, including peptides (Jin and Wei, 2024) and macrocycles (Nguyen et al., 2024). Challenges, such as data scarcity and incomprehensibility, have sparked research into nature-inspired machine learning and active learning strategies. In structure-based modeling, the integration of deep learning architectures, inspired by computer vision, has revolutionized predictions for protein-ligand interactions (Xie et al., 2024). The marriage of AI with these well-established methodologies underscores a promising trajectory in drug design, with a focus on enhanced predictive accuracy and efficiency.
3.2 De novo drug design with artificial intelligence
The creation of novel molecular entities with desired pharmacological properties, known as De novo drug design, is a formidable challenge in computer-assisted drug discovery (Hasselgren and Oprea, 2024). The vast chemical space, estimated from 1060–10100 potential drug-like molecules, adds complexity. Traditional structure-based and ligand-based drug design methods, though pivotal in discovering small-molecule drug candidates, face limitations due to their reliance on specific templates derived from active sites or pharmacophores. The introduction of AI techniques has revolutionized de novo drug design, with models like ReLeaSE (Amilpur and Dasari, 2024), ChemVAE (Hasselgren and Oprea, 2024), Graph INVENT (Yao et al., 2023), and MolRNN (Tropsha et al., 2023) utilizing diverse molecular representations. These deep learning-based approaches accelerate the drug discovery process by exploring chemical space efficiently. Categorized as ligand-based or structure-based, these methods use rule-based or rule-free approaches (Tropsha et al., 2023). Rule-based methods involve construction rules, while rule-free approaches, often based on generative deep learning models, sample molecules from a learned latent molecular representation (Tropsha et al., 2023). These generative models, including recurrent neural networks and variation autoencoders, are praised for their efficacy in exploring chemical space. Evaluation metrics include validity, novelty, similarity to known compounds, and scaffold diversity. A promising approach combines both rule-based and rule-free methods for designing bioactive and synthesizable molecular entities (Sinha et al., 2023). While current studies predominantly focus on ligand-based approaches, there is growing interest in exploring structure-based generative design, especially for targeting orphan receptors and unexplored macromolecules.
3.3 Drug toxicity prediction
Prediction of drug toxicity is an essential aspect of the drug development process, with the aim of identifying and assessing the importance of potential adverse effects or adverse reactions associated with a drug in advance, when it grows further in the development pipeline. Predicting drug toxicity is important because it is critical to the safety and wellbeing of the patients who will ultimately use the drug. Predicting Drug Toxicity Traditional techniques have placed emphasis on experimental research and animal testing, which are time-consuming, expensive, and do not always accurately reflect human responses (Nasnodkar et al., 2023) and with advances in machine learning (ML), drug toxicity prediction is undergoing a paradigm shift. These techniques are based on large datasets, including chemical gradients (Nasnodkar et al., 2023), biological pathways (Guo et al., 2023), and includes information on known toxicity profiles (Dou et al., 2023). Machine learning algorithms, such as support vector machines (Khan et al., 2024), random forests (Daghighi, 2023), and neural networks (Noor et al., 2023), are trained on these data sets to learn patterns and relationships that identify potential toxicity.
The use of artificial intelligence in predicting drug toxicity offers several advantages. This enables the analysis of large data sets, allowing for a more complete understanding of the complex interactions between drugs and biological systems (Nasnodkar et al., 2023). Machine learning models can identify hidden patterns and consensual relationships that are not apparent through traditional techniques. In addition, these models can help to better and more quickly determine potential toxicities for new drug candidates, which helps in the drug development phase (Rasool et al., 2023). Yes, but challenges remain, such as the need for optimal quality, different training data, and evaluation of complex AI models. Ethical acceptance and regulatory standards also play an important role in the integration of AI-based toxicity prediction into the drug development process. Despite these challenges, there is great promise in artificial intelligence-driven drug toxicity prediction to aid the safety and success of novel pharmaceuticals (Vora et al., 2023). “Continued research and collaboration between researchers, data scientists, and regulatory agencies is essential to ensure the accuracy of the prediction of eye-driven toxicity and progress in this field.
3.4 Integration of AI in retrosynthesis and reaction prediction
Retrosynthesis and reaction prediction have long been crucial in organic chemistry, guiding the planning of synthetic routes. With the intersection of material science and bioscience at the bio-interface, the advent of Computer-Assisted Organic Synthesis (CAOS) (Sankaranarayanan and Jensen, 2023) has emerged as a powerful tool for synthetic planning. In recent years, the exponential growth in reaction datasets and computational power has paved the way for the development of advanced machine learning (ML) and artificial intelligence (AI) models specifically tailored for CAOS programs (Abbasi and Rahmani, 2023). These models exhibit the capability to accurately predict individual synthetic and retrosynthetic reactions, offering valuable insights for chemists in designing synthetic pathways. One notable advancement involves combining single-step predictions through the integration of proper graph search algorithms (Kassa et al., 2023). This innovative approach has allowed researchers to design CAOS programs that excel in making comprehensive synthetic pathway predictions. By leveraging the wealth of data and computational capabilities, these programs contribute to the efficiency of synthetic planning, especially in the intricate domains of material and bio-interface studies. The integration of AI and ML in CAOS not only accelerates the prediction of viable synthetic routes but also enables chemists to explore complex reaction landscapes efficiently (López, 2023). The success of these programs lies in their ability to navigate diverse chemical spaces, providing valuable guidance for designing novel compounds at the bio-interface. However, challenges persist in ensuring the reliability of predictions, addressing issues of interpretability, and refining the algorithms for diverse chemical contexts (Mittal and Ahuja, 2023). Continued collaboration between computational chemists, organic chemists, and data scientists remains essential for further advancing CAOS applications. The synergy of retrosynthesis, reaction prediction, and CAOS stands as a testament to the transformative potential of AI-driven tools in shaping the future of synthetic chemistry at the interface of materials and bioscience. Supplementary Table S1 provides a concise overview of different applications of AI in the field of drug discovery, making it easier to understand the breadth of impact.
4 Limitations of artificial intelligence
While artificial intelligence holds promise in drug discovery, there are significant challenges and limitations that demand careful consideration. One primary challenge is the availability of suitable data. AI-driven approaches typically rely on extensive datasets for effective training (Blanco-Gonzalez et al., 2023). However, in many instances, the accessible data may be limited, of suboptimal quality, or inconsistent, thereby compromising the accuracy and reliability of the results. Ethical considerations also present a challenge (Prem, 2023), as EI-based techniques have brought problems like fairness and biases, as discussed in the received section. For example, if the data used to train the machine learning (ML) algorithm is biased or does not properly represent the perspectives of different viewers, the unique predictions may be incorrect or invalid. Can be bent. Addressing and integrating the ethical implications of E-I is instrumental in the development of new therapeutic compounds. Different strategies can be used to meet these challenges within the scope of chemotherapy in this field. Data augmentation is a technique that involves the production of synthetic data to complement existing data sets. The amount and variety of data available for training these machine algorithms can be greatly increased, yielding and tolerating results. Other measures include the use of Explicit AI (XAI) methods, which aim to provide interpretability and transparency to the predictions of machine algorithms. Such methods contribute to addressing concerns about bias and fairness in AI-driven approaches, providing a clearer understanding of the underlying mechanisms and assumptions guiding predictions (Chen et al., 2023).
Contemporary AI-based methodologies should not be viewed as a substitute for conventional experimental approaches, and they cannot replace the valuable expertise and experience contributed by human researchers (Dwivedi et al., 2023). AI is limited to offering predictions based on available data, and the subsequent validation and interpretation of results still rely on human researchers. Nevertheless, the integration of AI alongside traditional experimental methods has the potential to enhance the drug discovery process. Through the synergistic combination of AI’s predictive capabilities and the insights derived from the expertise and experience of human researchers, there exists an opportunity to optimize the drug discovery process and expedite the development of new medications (Hasselgren and Oprea, 2024; Alharbi et al., 2024; Zhang et al., 2024; Shi et al., 2022; Kang et al., 2020; Bibbò et al., 2017; Khan et al., 2020a; Iqbal et al., 2019; Khan et al., 2018a; Khan et al., 2021a; Jamil et al., 2021; Khan et al., 2020b; Tareen et al., 2021a; Khan et al., 2023; Khan et al., 2020c; Khan et al., 2021b; Tareen et al., 2022a; Khan et al., 2019a; Cao et al., 2012; Zhang et al., 2019; Hu et al., 2020; Tareen et al., 2022b; Khan et al., 2019b; Khan et al., 2021c; Khan et al., 2021d; Khan et al., 2021e; Aslam et al., 2021; Ahmad et al., 2021a; Shaheen et al., 2023; Li et al., 2023; Tang et al., 2021; Khan et al., 2019c; Khan et al., 2019d; Khatoon et al., 2020; Khan et al., 2018b; Khan et al., 2020d; Khan et al., 2018c; Khan et al., 2018d; Ahmad et al., 2021b; Duan et al., 2023; Dai et al., 2018) (Figure 2A).
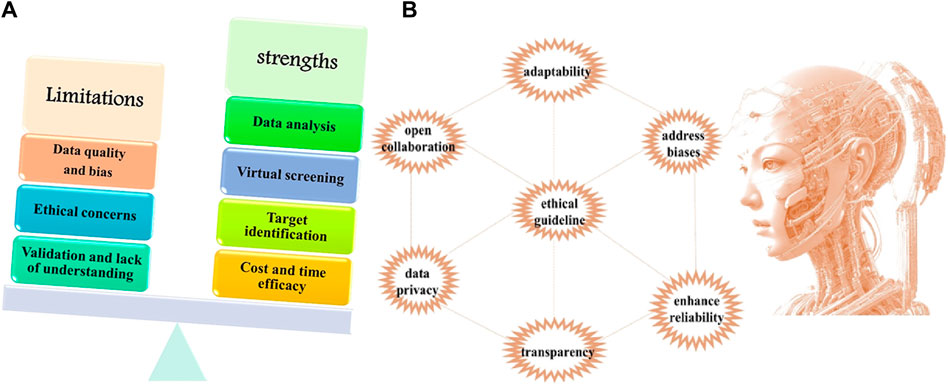
Figure 2. (A) Graphical representation of the comparison between strengths and limitations of AI, (B) Strategies and Approaches to Overcome Current Challenges.
5 Strategies and approaches to overcome current challenges
Incorporating artificial intelligence (AI) into drug discovery is a strategy of caution to overcome the current challenges. This consideration will aid in the continued development of AI in drug research. A foundational emphasis is placed on optimizing data inputs, prioritizing diverse and high-quality datasets as the bedrock for robust AI models. This addresses challenges related to data representativeness and accuracy (Figure 2B).
The establishment of ethical guidelines and governance frameworks is a critical imperative, making responsible and ethical AI use a guiding principle. This encompasses considerations such as data privacy and consent. Interdisciplinary collaboration emerges as an essential strategy, bridging the expertise of AI specialists with professionals in pharmacology, chemistry, and biology. This fosters a synergistic alliance, integrating computational capabilities with domain-specific knowledge. Transparency in AI decision-making gains significance, with the integration of Explainable AI (XAI) techniques instrumental in providing a clear understanding of AI-driven insights, particularly in the nuanced landscape of drug discovery. Adaptability is a key consideration, with the development of AI systems capable of continuous learning, ensuring sustained relevance in the dynamic field of drug discovery.
Holistic integration of computational predictions with traditional experimental methods is proposed, enhancing the reliability of drug discovery processes by capitalizing on the strengths inherent in both methodologies. Addressing biases within AI models becomes a central focus, with rigorous evaluations and mitigation strategies imperative to promote fairness and prevent disparities in drug discovery outcomes.
Engagements with regulatory bodies based on principles of quality and validation are supported to enable acceptance and regulation of AI-based tools in drug discovery. The driving force behind AI research is to promote open collaboration and data sharing that creates a culture of shared growth in the area of drug discovery.
Finally, the recommendation for investment in education and skill development programs serves to bridge the knowledge gap, ensuring a proficient workforce capable of navigating the intersection of AI and pharmaceutical sciences. In conclusion, these strategies collectively shape a comprehensive framework for overcoming existing challenges and optimizing the role of AI in advancing drug discovery methodologies (Chen et al., 2021; Sagar et al., 2021; Sagar et al., 2024).
6 Conclusion and summary of the potential of AI for revolutionizing drug discovery
A paradigm shift in pharmaceutical research and development is being brought about by the integration of AI into drug discovery processes. With the advent of AI, drug discovery pipelines have been significantly accelerated, offering novel solutions to longstanding challenges, such as identifying target protein structures, conducting virtual screenings, designing new drugs, predicting retrosynthesis reactions, bioactivity and toxicity. The scientific community and society overall must recognize the implications of AI-driven drug discovery moving forward. In the coming years, AI will have a significant impact on the drug development process, as it can streamline processes, reduce costs, and improve the efficiency and success rate of the identification of viable drug candidates. Furthermore, AI technologies could revolutionize patient care by improving medication management and improving healthcare delivery with the integration of AI technologies into pharmacy practices. In future, it is imperative to address several key issues. It is most important to develop new methods tailored to specific drug discovery challenges and optimize existing AI algorithms. It is also essential to integrate AI into existing drug discovery workflows seamlessly and foster collaboration among researchers, industry stakeholders, and regulatory bodies to ensure that AI is used in drug development in a responsible and ethical manner. As a result, the ongoing evolution of AI in drug discovery offers great promise for transforming the pharmaceutical sector and improving global health. It is possible to develop faster and more efficiently safer, more effective medications using AI-driven innovation and collaboration.
Author contributions
MaK: Writing–original draft. MR: Conceptualization, Writing–review and editing. MS: Data curation, Writing–review and editing. IH: Validation, Writing–review and editing. MuK: Formal Analysis, Writing–review and editing. ZX: Validation, Writing–review and editing. SS: Methodology, Writing–review and editing. AT: Methodology, Writing–review and editing. ZB: Formal analysis, riting–review and editing. KK: Supervision, Writing–review and editing.
Funding
The author(s) declare that no financial support was received for the research, authorship, and/or publication of this article.
Conflict of interest
The authors declare that the research was conducted in the absence of any commercial or financial relationships that could be construed as a potential conflict of interest.
Publisher’s note
All claims expressed in this article are solely those of the authors and do not necessarily represent those of their affiliated organizations, or those of the publisher, the editors and the reviewers. Any product that may be evaluated in this article, or claim that may be made by its manufacturer, is not guaranteed or endorsed by the publisher.
Supplementary material
The Supplementary Material for this article can be found online at: https://www.frontiersin.org/articles/10.3389/fchem.2024.1408740/full#supplementary-material
References
Abbasi, S., and Rahmani, A. M. (2023). Artificial intelligence and software modeling approaches in autonomous vehicles for safety management: a systematic review. Information 14 (10), 555. doi:10.3390/info14100555
Ahmad, S. O. A., Ashfaq, A., Akbar, M. U., Ikram, M., Khan, K., Wang, F., et al. (2021c). Application of two-dimensional materials in perovskite solar cells; recent progress, challenges and prospective solutions. J. Mater. Chem. C 9, 14065–14092. doi:10.1039/d1tc02407h
Ahmad, W., Gong, Y., Abbas, G., Khan, K., Khan, M., Ali, G., et al. (2021b). Evolution of low-dimensional material-based field-effect transistors. Nanoscale 13 (10), 5162–5186. doi:10.1039/d0nr07548e
Ahmad, W., Ullah, Z., Sonil, N. I., and Khan, K. (2021a). Introduction, production, characterization and applications of defects in graphene. J. Mater. Sci. Mater. Electron. 32, 19991–20030. doi:10.1007/s10854-021-06575-1
Ahmadi, S. (2024). A comprehensive study on integration of big data and AI in financial industry and its effect on present and future opportunities. Int. J. Curr. Sci. Res. Rev. 7 (01). doi:10.47191/ijcsrr/v7-i1-07
Akour, I., Alzyoud, M., Alquqa, E., Tariq, E., Alzboun, N., Al-Hawary, S., et al. (2024). Artificial intelligence and financial decisions: empirical evidence from developing economies. Int. J. Data Netw. Sci. 8 (1), 101–108. doi:10.5267/j.ijdns.2023.10.013
Alharbi, H. F., Bhupathyraaj, M., Mohandoss, K., Chacko, L., and Rani, K. R. V. (2024). An overview of artificial intelligence-driven pharmaceutical functionality. Artif. Intell. Pharm. Sci., 18–36. doi:10.1201/9781003343981-2
Alhatem, A., Wong, T., and Lambert, W. C. (2024). Revolutionizing diagnostic pathology: the emergence and impact of artificial intelligence-what doesn't kill you makes you stronger? Clin. Dermatology. doi:10.1016/j.clindermatol.2023.12.020
Amilpur, S., and Dasari, C. M. (2024). “20 DN-based to identify DTI model potential drug molecules against COVID-19,” in Handbook of AI-based Models in Healthcare and medicine: approaches, theories, and applications, 397.
Anthwal, A., Uniyal, A., Gairolla, J., Singh, R., Gehlot, A., Abbas, M., et al. (2024). Industry 4.0 technologies adoption for digital transition in drug discovery and development: a review. J. Industrial Inf. Integration 38, 100562. doi:10.1016/j.jii.2024.100562
Askr, H., Elgeldawi, E., Aboul Ella, H., Elshaier, Y. A., Gomaa, M. M., and Hassanien, A. E. (2023). Deep learning in drug discovery: an integrative review and future challenges. Artif. Intell. Rev. 56 (7), 5975–6037. doi:10.1007/s10462-022-10306-1
Aslam, S., Sagar, R. U. R., Ali, R., Gbadamasi, S., Khan, K., Butt, S., et al. (2021). Mixed-dimensional niobium disulfide-graphene foam heterostructures as an efficient catalyst for hydrogen production. Int. J. Hydrogen Energy 46 (68), 33679–33688. doi:10.1016/j.ijhydene.2021.07.170
Atas Guvenilir, H., and Doğan, T. (2023). How to approach machine learning-based prediction of drug/compound–target interactions. J. Cheminformatics 15 (1), 16–36. doi:10.1186/s13321-023-00689-w
Bibbò, L., Khan, K., Liu, Q., Lin, M., Wang, Q., and Ouyang, Z. (2017). Tunable narrowband antireflection optical filter with a metasurface. Photonics Res. 5 (5), 500–506. doi:10.1364/prj.5.000500
Blanco-Gonzalez, A., Cabezon, A., Seco-Gonzalez, A., Conde-Torres, D., Antelo-Riveiro, P., Pineiro, A., et al. (2023). The role of ai in drug discovery: challenges, opportunities, and strategies. Pharmaceuticals 16 (6), 891. doi:10.3390/ph16060891
Buckner, C. J. (2023) From deep learning to rational machines: what the history of philosophy can teach us about the future of artificial intelligence. Oxford University Press.
Cao, F., Zhang, Y., Wang, H., Khan, K., Tareen, A. K., Qian, W., et al. (2012). Recent advances in oxidation stable chemistry of two-dimensional MXenes. Adv. Mater. n/a, 2107554. doi:10.1002/adma.202107554
Chellaswamy, C., Ramasubramanian, B., and Sriram, A. (2024). FPGA-based remote target classification in hyperspectral imaging using multi-graph neural network. Microprocess. Microsystems 105, 105008. doi:10.1016/j.micpro.2024.105008
Chen, L., Sagar, R. U. R., Aslam, S., Deng, Y., Hussain, S., Ali, W., et al. (2021). Neodymium-decorated graphene as an efficient electrocatalyst for hydrogen production, Nanoscale 13, 15471–15480. doi:10.1039/d1nr03992j
Chen, P., Wu, L., and Wang, L. (2023). AI fairness in data management and analytics: a review on challenges, methodologies and applications. Appl. Sci. 13 (18), 10258. doi:10.3390/app131810258
Chisholm, T. S., Mackey, M., and Hunter, C. A. (2023). Discovery of high-affinity amyloid ligands using a ligand-based virtual screening pipeline. J. Am. Chem. Soc. 145 (29), 15936–15950. doi:10.1021/jacs.3c03749
Cohen, E. B., Patwardhan, M., Raheja, R., Alpers, D. H., Andrade, R. J., Avigan, M. I., et al. (2024). Drug-Induced liver injury in the elderly: consensus statements and recommendations from the IQ-DILI initiative. Drug Saf. 47, 301–319. doi:10.1007/s40264-023-01390-5
Creecy, A., Awosanya, O. D., Harris, A., Qiao, X., Ozanne, M., Toepp, A. J., et al. (2024). COVID-19 and bone loss: a review of risk factors, mechanisms, and future directions. Curr. Osteoporos. Rep. 22, 122–134. doi:10.1007/s11914-023-00842-2
Daghighi, A. (2023). in In silico toxicology: application of machine learning for predicting toxicity of organic compounds (Fargo, North Dakota : North Dakota State University of Agriculture and Applied Science).
Dai, M., Huo, C., Zhang, Q., Khan, K., Zhang, X., and Shen, C. (2018). Electrochemical mechanism and structure simulation of 2D lithium-ion battery. Adv. Theory Simulations 1 (10), 1800023. doi:10.1002/adts.201800023
Damiano, L., and Stano, P. (2023). Explorative synthetic biology in AI: criteria of relevance and a taxonomy for synthetic models of living and cognitive processes. Artif. Life 29 (3), 367–387. doi:10.1162/artl_a_00411
DiFrancesco, M., Hofer, J., Aradhya, A., Rufinus, J., Stoddart, J., Finocchiaro, S., et al. (2023). Discovery of small-molecule PD-1/PD-L1 antagonists through combined virtual screening and experimental validation. Comput. Biol. Chem. 102, 107804. doi:10.1016/j.compbiolchem.2022.107804
Dou, B., Zhu, Z., Merkurjev, E., Ke, L., Chen, L., Jiang, J., et al. (2023). Machine learning methods for small data challenges in molecular science. Chem. Rev. 123 (13), 8736–8780. doi:10.1021/acs.chemrev.3c00189
Dragan, P., Joshi, K., Atzei, A., and Latek, D. (2023). Keras/TensorFlow in drug design for immunity disorders. Int. J. Mol. Sci. 24 (19), 15009. doi:10.3390/ijms241915009
Duan, X., Liu, Z., Xie, Z., Tareen, A. K., Khan, K., Zhang, B., et al. (2023). Emerging monoelemental 2D materials (Xenes) for biosensor applications. Nano Res. 16 (5), 7030–7052. doi:10.1007/s12274-023-5418-3
Dwivedi, Y. K., Kshetri, N., Hughes, L., Slade, E. L., Jeyaraj, A., Kar, A. K., et al. (2023). “So what if ChatGPT wrote it?” Multidisciplinary perspectives on opportunities, challenges and implications of generative conversational AI for research, practice and policy. Int. J. Inf. Manag. 71, 102642. doi:10.1016/j.ijinfomgt.2023.102642
Guo, W., Liu, J., Dong, F., Song, M., Li, Z., Khan, M. K. H., et al. (2023). Review of machine learning and deep learning models for toxicity prediction. Exp. Biol. Med. 248, 1952–1973. doi:10.1177/15353702231209421
Hasselgren, C., and Oprea, T. I. (2024). Artificial intelligence for drug discovery: are we there yet? Annu. Rev. Pharmacol. Toxicol. 64, 527–550. doi:10.1146/annurev-pharmtox-040323-040828
Hu, H., Shi, Z., Khan, K., Cao, R., Liang, W., Tareen, A. K., et al. (2020). Recent advances in doping engineering of black phosphorus. J. Mater. Chem. A 8 (11), 5421–5441. doi:10.1039/d0ta00416b
Iqbal, M., Ali, A., Ahmad, K. S., Rana, F. M., Khan, J., Khan, K., et al. (2019). Synthesis and characterization of transition metals doped CuO nanostructure and their application in hybrid bulk heterojunction solar cells. SN Appl. Sci. 1 (6), 647. doi:10.1007/s42452-019-0663-5
Jamil, R., Ali, R., Loomba, S., Xian, J., Yousaf, M., Khan, K., et al. (2021) The role of nitrogen in transition-metal nitrides in electrochemical water splitting.
Janet, J. P., Mervin, L., and Engkvist, O. (2023). Artificial intelligence in molecular de novo design: integration with experiment. Curr. Opin. Struct. Biol. 80, 102575. doi:10.1016/j.sbi.2023.102575
Jin, Z., and Wei, Z. (2024). Molecular simulation for food protein–ligand interactions: a comprehensive review on principles, current applications, and emerging trends. Compr. Rev. Food Sci. Food Saf. 23 (1), 132800–e13329. doi:10.1111/1541-4337.13280
Kang, J., Zheng, C., Khan, K., Tareen, A. K., Aslam, M., and Wang, B. (2020). Two dimensional nanomaterials-enabled smart light regulation technologies: recent advances and developments. Optik 220, 165191. doi:10.1016/j.ijleo.2020.165191
Kassa, G., Liu, J., Hartman, T. W., Dhiman, S., Gadhamshetty, V., and Gnimpieba, E. (2023). “Artificial intelligence based organic synthesis planning for material and bio-interface discovery,” in Microbial stress response: mechanisms and data science (American Chemical Society), 93–111.
Khan, J., Ullah, H., Sajjad, M., Jatoi, W. B., Ali, A., Khan, K., et al. (2019e). Controlled synthesis of ammonium manganese tri-fluoride nanoparticles with enhanced electrochemical performance. Mater. Res. Express 6 (7), 075074. doi:10.1088/2053-1591/ab18bb
Khan, K., Khan Tareen, A., Li, J., Khan, U., Nairan, A., Yuan, Y., et al. (2018b). Facile synthesis of tin-doped mayenite electride composite as a non-noble metal durable electrocatalyst for oxygen reduction reaction (ORR). Dalton Trans. 47 (38), 13498–13506. doi:10.1039/c8dt02548g
Khan, K., Tareen, A. K., Aslam, M., Khan, M. F., Shi, Z., Ma, C., et al. (2020a). Synthesis, properties and novel electrocatalytic applications of the 2D-borophene Xenes. Prog. Solid State Chem. 59, 100283. doi:10.1016/j.progsolidstchem.2020.100283
Khan, K., Tareen, A. K., Aslam, M., Khan, Q., Khan, S. A., Khan, Q. U., et al. (2019b). Novel two-dimensional carbon–chromium nitride-based composite as an electrocatalyst for oxygen reduction reaction. Front. Chem. 7, 738. doi:10.3389/fchem.2019.00738
Khan, K., Tareen, A. K., Aslam, M., Khan, S. A., Khan, Q. U., Saeed, M., et al. (2019d). Fe-doped mayenite electride composite with 2D reduced graphene oxide: as a non-platinum based, highly durable electrocatalyst for Oxygen Reduction Reaction. Sci. Rep. 9 (1), 19809–19811. doi:10.1038/s41598-019-55207-6
Khan, K., Tareen, A. K., Aslam, M., Mahmood, A., Zhang, Y., Ouyang, Z., et al. (2019c). Going green with batteries and supercapacitor: two dimensional materials and their nanocomposites based energy storage applications. Prog. Solid State Chem. 58, 100254. doi:10.1016/j.progsolidstchem.2019.100254
Khan, K., Tareen, A. K., Aslam, M., Sagar, R. U. R., Zhang, B., Huang, W., et al. (2020b). Recent progress, challenges, and prospects in two-dimensional photo-catalyst materials and environmental remediation. Nano-Micro Lett. 12 (1), 167–177. doi:10.1007/s40820-020-00504-3
Khan, K., Tareen, A. K., Aslam, M., Thebo, K. H., Khan, U., Wang, R., et al. (2019f). A comprehensive review on synthesis of pristine and doped inorganic room temperature stable mayenite electride,[Ca24Al28O64] 4+(e−) 4 and its applications as a catalyst. Prog. Solid State Chem. 54, 1–19. doi:10.1016/j.progsolidstchem.2018.12.001
Khan, K., Tareen, A. K., Aslam, M., Wang, R., Zhang, Y., Mahmood, A., et al. (2020c). Recent developments in emerging two-dimensional materials and their applications. J. Mater. Chem. C 8 (2), 387–440. doi:10.1039/c9tc04187g
Khan, K., Tareen, A. K., Aslam, M., Zhang, Y., Wang, R., Khan, S. A., et al. (2020d). Facile synthesis of mayenite electride nanoparticles encapsulated in graphitic shells like carbon nano onions: non-noble-metal electrocatalysts for oxygen reduction reaction (ORR). Front. Chem. 7, 934. doi:10.3389/fchem.2019.00934
Khan, K., Tareen, A. K., Aslam, M., Zhang, Y., Wang, R., Ouyang, Z., et al. (2019a). Recent advances in two-dimensional materials and their nanocomposites in sustainable energy conversion applications. Nanoscale 11 (45), 21622–21678. doi:10.1039/c9nr05919a
Khan, K., Tareen, A. K., Elshahat, S., Muhammad, N., Li, J., Aboodd, I., et al. (2018d). Facile metal-free reduction-based synthesis of pristine and cation-doped conductive mayenite. Rsc Adv. 8 (43), 24276–24285. doi:10.1039/c8ra02790k
Khan, K., Tareen, A. K., Elshahat, S., Yadav, A., Khan, U., Yang, M., et al. (2018c). Facile synthesis of a cationic-doped [Ca24Al28O64] 4+(4e−) composite via a rapid citrate sol-gel method. Dalton Trans. 47 (11), 3819–3830. doi:10.1039/c7dt04543c
Khan, K., Tareen, A. K., Iqbal, M., Mahmood, A., Shi, Z., Yin, J., et al. (2021b). Recent development in graphdiyne and its derivative materials for novel biomedical applications. J. Mater. Chem. B 9, 9461–9484. doi:10.1039/d1tb01794b
Khan, K., Tareen, A. K., Iqbal, M., Shi, Z., Zhang, H., and Guo, Z. (2021d). Novel emerging graphdiyne based two dimensional materials: synthesis, properties and renewable energy applications. Nano Today 39, 101207. doi:10.1016/j.nantod.2021.101207
Khan, K., Tareen, A. K., Iqbal, M., Wang, L., Ma, C., Shi, Z., et al. (2021e). Navigating recent advances in monoelemental materials (Xenes)-fundamental to biomedical applications. Prog. Solid State Chem. 63, 100326. doi:10.1016/j.progsolidstchem.2021.100326
Khan, K., Tareen, A. K., Iqbal, M., Ye, Z., Xie, Z., Mahmood, A., et al. (2023). Recent progress in emerging novel MXenes based materials and their fascinating sensing applications. Small 19, 2206147. doi:10.1002/smll.202206147
Khan, K., Tareen, A. K., Khan, Q. U., Iqbal, M., Zhang, H., and Guo, Z. (2021c). Novel synthesis, properties and applications of emerging group VA two-dimensional monoelemental materials (2D-Xenes). Mater. Chem. Front. 5 (17), 6333–6391. doi:10.1039/d1qm00629k
Khan, K., tareen, A. k., Khan, U., Nairan, A., Elshahat, S., Muhammad, N., et al. (2018a). Single step synthesis of highly conductive room-temperature stable cation-substituted mayenite electride target and thin film. Sci. Rep. 9, 4967. SREP-18-33491B. doi:10.1038/s41598-019-41512-7
Khan, K., Tareen, A. K., Wang, L., Aslam, M., Ma, C., Mahmood, N., et al. (2021a). Sensing applications of atomically thin group iv carbon siblings xenes: progress, challenges, and prospects. Adv. Funct. Mater. 31 (3), 2005957. doi:10.1002/adfm.202005957
Khan, P. M., Jillella, G. K., and Roy, K. (2024). “Recent advancements in QSAR and machine learning approaches for risk assessment of organic chemicals,” in QSAR in safety evaluation and risk assessment (Academic Press), 167–185.
Khatoon, R., Guo, Y., Attique, S., Khan, K., Treen, A. K., Haq, M. U., et al. (2020). Facile synthesis of α-Fe2O3/Nb2O5 heterostructure for advanced Li-Ion batteries. J. Alloys Compd. 837, 155294. doi:10.1016/j.jallcom.2020.155294
Kilonzi, M., Mutagonda, R. F., Mwakawanga, D. L., Mlyuka, H. J., Mikomangwa, W. P., Kibanga, W. A., et al. (2024). Establishment of clinical pharmacy services: evidence-based information from stakeholders. Hum. Resour. Health 22 (1), 6–4. doi:10.1186/s12960-023-00887-5
Klauschen, F., Dippel, J., Keyl, P., Jurmeister, P., Bockmayr, M., Mock, A., et al. (2024). Toward explainable artificial intelligence for precision pathology. Annu. Rev. Pathology Mech. Dis. 19, 541–570. doi:10.1146/annurev-pathmechdis-051222-113147
Kotkondawar, R., Sutar, S., and Kiwelekar, A. (2024). Applications of deep learning in bioinformatics and drug discovery. Authorea Prepr. doi:10.22541/au.170433004.48304398/v1
Kumar, N., and Acharya, V. (2023). Advances in machine intelligence-driven virtual screening approaches for big-data. Med. Res. Rev. 44, 939–974. doi:10.1002/med.21995
Li, C., Tareen, A. K., Khan, K., Long, J., Hussain, I., Khan, M. F., et al. (2023). Highly efficient, remarkable sensor activity and energy storage properties of MXenes and borophene nanomaterials. Prog. Solid State Chem. 70, 100392. doi:10.1016/j.progsolidstchem.2023.100392
López, C. (2023). Artificial intelligence and advanced materials. Adv. Mater. 35 (23), 2208683. doi:10.1002/adma.202208683
Mittal, A., and Ahuja, G. (2023). Advancing chemical carcinogenicity prediction modeling: opportunities and challenges. Trends Pharmacol. Sci. 44, 400–410. doi:10.1016/j.tips.2023.04.002
Nasnodkar, S., Cinar, B., and Ness, S. (2023). Artificial intelligence in toxicology and pharmacology. J. Eng. Res. Rep. 25 (7), 192–206. doi:10.9734/jerr/2023/v25i7952
Nebreda, A., Shpakivska-Bilan, D., Camara, C., and Susi, G. (2024). “The social machine: artificial intelligence (AI) approaches to theory of mind,” in The theory of mind under scrutiny: psychopathology, neuroscience, philosophy of mind and artificial intelligence (Cham: Springer Nature Switzerland), 681–722.
Nguyen, N. Q., Park, S., Gim, M., and Kang, J. (2024). MulinforCPI: enhancing precision of compound–protein interaction prediction through novel perspectives on multi-level information integration. Briefings Bioinforma. 25 (1), bbad484. doi:10.1093/bib/bbad484
Noor, F., Asif, M., Ashfaq, U. A., Qasim, M., and Tahir ul Qamar, M. (2023). Machine learning for synergistic network pharmacology: a comprehensive overview. Briefings Bioinforma. 24, bbad120. doi:10.1093/bib/bbad120
Oliveira, T. A. D., Silva, M. P. D., Maia, E. H. B., Silva, A. M. D., and Taranto, A. G. (2023). Virtual screening algorithms in drug discovery: a review focused on machine and deep learning methods. Drugs Drug Candidates 2 (2), 311–334. doi:10.3390/ddc2020017
Pham, P., Zhang, H., Gao, W., and Zhu, X. (2024). Determinants and performance outcomes of artificial intelligence adoption: evidence from US Hospitals. J. Bus. Res. 172, 114402. doi:10.1016/j.jbusres.2023.114402
Philippe, G. J. B., Huang, Y. H., Mittermeier, A., Brown, C. J., Kaas, Q., Ramlan, S. R., et al. (2024). Delivery to, and reactivation of, the p53 pathway in cancer cells using a grafted cyclotide conjugated with a cell-penetrating peptide. J. Med. Chem. 67, 1197–1208. doi:10.1021/acs.jmedchem.3c01682
Prasad, K. D. V., and Kalavakolanu, S. (2023). The study of cognitive psychology in conjunction with artificial intelligence. Conhecimento Divers. 15 (36), 270–286. doi:10.18316/rcd.v15i36.10788
Pun, F. W., Ozerov, I. V., and Zhavoronkov, A. (2023). AI-powered therapeutic target discovery. Trends Pharmacol. Sci. 44, 561–572. doi:10.1016/j.tips.2023.06.010
Ramos, P. I. P., Marcilio, I., Bento, A. I., Penna, G. O., de Oliveira, J. F., Khouri, R., et al. (2024). Combining digital and molecular approaches using health and alternate data sources in a next-generation surveillance system for anticipating outbreaks of pandemic potential. JMIR Public Health Surveillance 10, e47673. doi:10.2196/47673
Rasool, S., Husnain, A., Saeed, A., Gill, A. Y., and Hussain, H. K. (2023). Harnessing predictive power: exploring the crucial role of machine learning in early disease detection. JURIHUM J. Inov. Dan. Hum. 1 (2), 302–315.
Ratten, V. (2024). Artificial intelligence, digital trends and globalization: future research trends. FIIB Bus. Rev., 23197145231222774. doi:10.1177/23197145231222774
Rehman, A. U., Khurshid, B., Ali, Y., Rasheed, S., Wadood, A., Ng, H. L., et al. (2023). Computational approaches for the design of modulators targeting protein-protein interactions. Expert Opin. drug Discov. 18 (3), 315–333. doi:10.1080/17460441.2023.2171396
Sagar, R. U. R., Zaiping, X., Iqbal, J., Rehman, S. U., Ashraf, H., Liu, C., et al. (2021) Extremely large, linear, and controllable positive magnetoresistance in neodymium-doped graphene foam for magnetic sensors, 100460.
Sagar, R. U. R., Rahman, M. M., Cai, Q., Liang, T., and Chen, Y. I. J. J. (2024). A comparative study on morphology dependent performance of neodymium–graphene as an anode material in lithium-ion batteries. J. Energy Storage 77, 109854. doi:10.1016/j.est.2023.109854
Sanchez, D., Slovacek, H., and Wang, R. (2024). Shaping the future of men's sexual health: how artificial intelligence can assist in the management and treatment of erectile dysfunction. UroPrecision. doi:10.1002/uro2.31
Sankaranarayanan, K., and Jensen, K. F. (2023). Computer-assisted multistep chemoenzymatic retrosynthesis using a chemical synthesis planner. Chem. Sci. 14 (23), 6467–6475. doi:10.1039/d3sc01355c
Sarkar, C., Das, B., Rawat, V. S., Wahlang, J. B., Nongpiur, A., Tiewsoh, I., et al. (2023). Artificial intelligence and machine learning technology driven modern drug discovery and development. Int. J. Mol. Sci. 24 (3), 2026. doi:10.3390/ijms24032026
Shaheen, I., Ali, I., Bibi, F., Hanan, A., Ahmad, M., Hussain, I., et al. (2023). Integrating 1D/2D nanostructure based on Ni–Co-oxalate for energy storage applications. Ceram. Int. 50, 10789–10796. doi:10.1016/j.ceramint.2023.12.394
Shi, Z., Zhang, H., Khan, K., Cao, R., Xu, K., and Zhang, H. (2022). Two-dimensional selenium and its composites for device applications. Nano Res. 15 (1), 104–122. doi:10.1007/s12274-021-3493-x
Shiota, K., Suma, A., Ogawa, H., Yamaguchi, T., Iida, A., Hata, T., et al. (2023). AQDnet: deep neural network for protein–ligand docking simulation. ACS Omega 8, 23925–23935. doi:10.1021/acsomega.3c02411
Sim, J. A., Huang, X., Horan, M. R., Stewart, C. M., Robison, L. L., Hudson, M. M., et al. (2023). Natural language processing with machine learning methods to analyze unstructured patient-reported outcomes derived from electronic health records: a systematic review. Artif. Intell. Med. 146, 102701. doi:10.1016/j.artmed.2023.102701
Singh, V., and Khatun, A. (2024). “Application of artificial intelligence in human resource management: a conceptual framework,” in The role of HR in the transforming workplace (Taylor & Francis Group, England : Productivity Press), 32–49.
Sinha, K., Ghosh, N., and Sil, P. C. (2023). A review on the recent applications of deep learning in predictive drug toxicological studies. Chem. Res. Toxicol. 36 (8), 1174–1205. doi:10.1021/acs.chemrestox.2c00375
Stevenson, G. A., Kirshner, D., Bennion, B. J., Yang, Y., Zhang, X., Zemla, A., et al. (2023). Clustering protein binding pockets and identifying potential drug interactions: a novel ligand-based featurization method. J. Chem. Inf. Model. 63 (21), 6655–6666. doi:10.1021/acs.jcim.3c00722
Tang, X., Sagar, R. U. R., Luo, M., Aslam, S., Liu, Y., Khan, K., et al. (2021). Graphene foam–polymer based electronic skin for flexible tactile sensor. Sensors Actuators A Phys. 327, 112697. doi:10.1016/j.sna.2021.112697
Tareen, A. K., Khan, K., Aslam, M., Liu, X., and Zhang, H. (2021b). Confinement in two-dimensional materials: major advances and challenges in the emerging renewable energy conversion and other applications. Prog. Solid State Chem. 61, 100294. doi:10.1016/j.progsolidstchem.2020.100294
Tareen, A. K., Khan, K., Aslam, M., Zhang, H., and Liu, X. (2021a). Recent progress, challenges, and prospects in emerging group-VIA Xenes: synthesis, properties and novel applications. Nanoscale 13 (2), 510–552. doi:10.1039/d0nr07444f
Tareen, A. K., Khan, K., Iqbal, M., Zhang, Y., Long, J., Mahmood, A., et al. (2022b). Recent advance in two-dimensional MXenes: new horizons in flexible batteries and supercapacitors technologies. Energy Storage Mater. 53, 783–826. doi:10.1016/j.ensm.2022.09.030
Tareen, A. K., Khan, K., Rehman, S., Iqbal, M., Yu, J., Zhou, Z., et al. (2022a). Recent development in emerging phosphorene based novel materials: progress, challenges, prospects and their fascinating sensing applications. Prog. Solid State Chem. 65, 100336. doi:10.1016/j.progsolidstchem.2021.100336
Thangavel, K., Sabatini, R., Gardi, A., Ranasinghe, K., Hilton, S., Servidia, P., et al. (2024). Artificial intelligence for trusted autonomous satellite operations. Prog. Aerosp. Sci. 144, 100960. doi:10.1016/j.paerosci.2023.100960
Thenuwara, G., Curtin, J., and Tian, F. (2023). Advances in diagnostic tools and therapeutic approaches for gliomas: a comprehensive review. Sensors 23 (24), 9842. doi:10.3390/s23249842
Tran, T. T. V., Surya Wibowo, A., Tayara, H., and Chong, K. T. (2023). Artificial intelligence in drug toxicity prediction: recent advances, challenges, and future perspectives. J. Chem. Inf. Model. 63 (9), 2628–2643. doi:10.1021/acs.jcim.3c00200
Tripathi, S. A., Rohokale, M. S., and Kumar, S. (2024). Advances in the treatment of cognitive diseases using IOT-based wearable devices. Cognitive Predict. Maintenance Tools Brain Dis., 138–157. doi:10.1201/9781003245346-9
Tropsha, A., Isayev, O., Varnek, A., Schneider, G., and Cherkasov, A. (2023). Integrating QSAR modelling and deep learning in drug discovery: the emergence of deep QSAR. Nat. Rev. Drug Discov. 23, 141–155. doi:10.1038/s41573-023-00832-0
Turon, G., Hlozek, J., Woodland, J. G., Kumar, A., Chibale, K., and Duran-Frigola, M. (2023). First fully-automated AI/ML virtual screening cascade implemented at a drug discovery centre in Africa. Nat. Commun. 14 (1), 5736. doi:10.1038/s41467-023-41512-2
Vora, L. K., Gholap, A. D., Jetha, K., Thakur, R. R. S., Solanki, H. K., and Chavda, V. P. (2023). Artificial intelligence in pharmaceutical technology and drug delivery design. Pharmaceutics 15 (7), 1916. doi:10.3390/pharmaceutics15071916
Wang, L., Dai, C., Jiang, L., Tong, G., Xiong, Y., Khan, K., et al. (2021). Advanced devices for tumor diagnosis and therapy. Small 17, 2100003. doi:10.1002/smll.202100003
Wardat, Y., Tashtoush, M., AlAli, R., and Saleh, S. (2024). Artificial intelligence in education: mathematics teachers’ perspectives, practices and challenges. Iraqi J. Comput. Sci. Math. 5 (1), 60–77. doi:10.52866/ijcsm.2024.05.01.004
Wilde, A. M., Song, M., Allen, W. P., Junkins, A. D., Frazier, J. M., Moore, S. E., et al. (2024). Implementation of a pharmacy-driven rapid bacteremia response program. Am. J. Health-System Pharm. 81 (2), 74–82. doi:10.1093/ajhp/zxad211
Xie, W., Zhang, J., Xie, Q., Gong, C., Xu, Y., Lai, L., et al. (2024) Accelerating discovery of novel and bioactive ligands with pharmacophore-informed generative models. arXiv preprint arXiv:2401.01059.
Yaiprasert, C., and Hidayanto, A. N. (2024). AI-powered ensemble machine learning to optimize cost strategies in logistics business. Int. J. Inf. Manag. Data Insights 4 (1), 100209. doi:10.1016/j.jjimei.2023.100209
Yan, Y., Zhao, Y., Yao, H., Feng, J., Liang, L., Han, W., et al. (2023). RPBP: deep retrosynthesis reaction prediction based on byproducts. J. Chem. Inf. Model. 63 (19), 5956–5970. doi:10.1021/acs.jcim.3c00274
Yang, S., and Kar, S. (2023). Application of artificial intelligence and machine learning in early detection of adverse drug reactions (ADRs) and drug-induced toxicity. Artif. Intell. Chem. 1, 100011. doi:10.1016/j.aichem.2023.100011
Yao, L., Wang, Z., Guo, W., Xiang, S., Liu, W., and Ke, G. (2023) Node-aligned graph-to-graph generation for retrosynthesis prediction. arXiv preprint arXiv:2309.15798.
Yin, H., Sharma, B., Hu, H., Liu, F., Kaur, M., Cohen, G., et al. (2024). Predicting the climate impact of healthcare facilities using gradient boosting machines. Clean. Environ. Syst. 12, 100155. doi:10.1016/j.cesys.2023.100155
Zeng, T., Jin, B., Glade, T., Xie, Y., Li, Y., Zhu, Y., et al. (2024). Assessing the imperative of conditioning factor grading in machine learning-based landslide susceptibility modeling: a critical inquiry. Catena 236, 107732. doi:10.1016/j.catena.2023.107732
Zhang, L., Khan, K., Zou, J., Zhang, H., and Li, Y. (2019). Recent advances in emerging 2D material-based gas sensors: potential in disease diagnosis. Adv. Mater. Interfaces 6 (22), 1901329. doi:10.1002/admi.201901329
Zhang, X., Khan, K., Tareen, A. K., and Zhang, Y. (2023). Application of 2D polyoxometalate clusterphene in a high-performance photoelectrochemical photodetector. Adv. Opt. Mater. 11 (20), 2300646. doi:10.1002/adom.202300646
Keywords: AI, drug discovery, machine learning, structure-activity relationship, artificial intelligence
Citation: Khan MK, Raza M, Shahbaz M, Hussain I, Khan MF, Xie Z, Shah SSA, Tareen AK, Bashir Z and Khan K (2024) The recent advances in the approach of artificial intelligence (AI) towards drug discovery. Front. Chem. 12:1408740. doi: 10.3389/fchem.2024.1408740
Received: 29 March 2024; Accepted: 26 April 2024;
Published: 31 May 2024.
Edited by:
Chenhui Yang, Northwestern Polytechnical University, ChinaReviewed by:
Rizwan Ur Rehman Sagar, Jiangxi University of Science and Technology, ChinaCopyright © 2024 Khan, Raza, Shahbaz, Hussain, Khan, Xie, Shah, Tareen, Bashir and Khan. This is an open-access article distributed under the terms of the Creative Commons Attribution License (CC BY). The use, distribution or reproduction in other forums is permitted, provided the original author(s) and the copyright owner(s) are credited and that the original publication in this journal is cited, in accordance with accepted academic practice. No use, distribution or reproduction is permitted which does not comply with these terms.
*Correspondence: Mohsin Raza, mohsinraza514@yahoo.com; Karim Khan, karim_khan_niazi@yahaoo.com