- 1Department of Biotechnology, School of Bioengineering, SRM Institute of Science and Technology, Chengalpattu, India
- 2Computational Biology Laboratory, Department of Genetic Engineering, School of Bioengineering, SRM Institute of Science and Technology, Chengalpattu, India
- 3Department of Chemistry and Department of Carbon Materials, Chosun University, Gwangju, South Korea
Coronavirus disease-2019 (COVID-19) has caused a severe impact on almost all aspects of human life and economic development. Numerous studies are being conducted to find novel therapeutic strategies to overcome COVID-19 pandemic in a much effective way. Ulva intestinalis L. (Ui), a marine microalga, known for its antiviral property, was considered for this study to determine the antiviral efficacy against severe acute respiratory syndrome-associated Coronavirus-2 (SARS-CoV-2). The algal sample was dried and subjected to ethanolic extraction, followed by purification and analysis using gas chromatography-coupled mass spectrometry (GC-MS). Forty-three known compounds were identified and docked against the S1 receptor binding domain (RBD) of the spike (S) glycoprotein. The compounds that exhibited high binding affinity to the RBD of S1 protein were further analyzed for their chemical behaviour using conceptual density-functional theory (C-DFT). Finally, pharmacokinetic properties and drug-likeliness studies were carried out to test if the compounds qualified as potential leads. The results indicated that mainly phenols, polyenes, phytosteroids, and aliphatic compounds from the extract, such as 2,4-di-tert-butylphenol (2,4-DtBP), doconexent, 4,8,13-duvatriene-1,3-diol (DTD), retinoyl-β-glucuronide 6′,3′-lactone (RBGUL), and retinal, showed better binding affinity to the target. Pharmacokinetic validation narrowed the list to 2,4-DtBP, retinal and RBGUL as the possible antiviral candidates that could inhibit the viral spike protein effectively.
Introduction
COVID-19, a contagious viral disease caused by SARS-CoV-2, was declared as a public health emergency of international concern by the World Health Organization (WHO) on 30 January 2020, and as a pandemic on March 11, 2020 (Ge et al., 2020). According to the recent pandemic situation report released by the WHO, SARS-CoV-2 has infected nearly 180 million individuals, causing about four million deaths. Being a positive, single-stranded RNA virus of size 50–200 nm and genome size of 29.9 k ribonucleotides, it is the most recent member included in the Betacoronavirus genus of the Orthocoranavirinae subfamily of coronaviruses (Lu et al., 2020). The viral genome was found to encode twelve main proteins, of which two, the spike glycoprotein and the main protease (Mpro) have gained attention as potential COVID-19 drug targets (Pavlova et al., 2021). The availability of structural details of these two proteins has accelerated computational studies. The thermodynamically favoured irreversible inhibition of Mpro by Michael acceptors has been studied by computational methods such as molecular dynamics and density functional theory (Poater 2020; Ramos-Guzmán et al., 2021; Zanetti-Polzi et al., 2021). The covalent and non-covalent binding free energies of Mproinhibitors have been studied to aid in rational drug discovery and design for targeted antiviral therapy (Awoonor-Williams and Abu-Saleh, 2021). Several experimentations suggest that SARS-CoV and SARS-CoV–2 have a sequence identity of approximately 79 percent, and both variants use angiotensin converting enzyme 2 (ACE2) as their cellular receptor. Similarly, some studies suggest that the infectivity rate varies with amino acid change in the spike protein, and the adsorption of S protein on gold nanoparticles was completely dependant on the size of the core nano-gold (Bette et al., 2021; Yokoyama and Ichiki, 2021). The spike glycoprotein is comprised of two subunits, the S1, which has the receptor binding domain, and the S2, which facilitates membrane fusion and endocytosis of the virus (Walls et al., 2020). Several studies have shown that SARS-CoV-2 utilizes the S1 protein to bind to the functional receptor human ACE2 (hACE2) at the RBD. The same mechanism was used for viral entry by SARS-CoV too. Eventually S2 protein aids in fusion of viral particles in the host. The receptor-binding motif (RBM) in RBD is the main functional motif and is composed of two regions (region 1 and region 2) that form the interface between the S protein and hACE2. The region outside the RBM in RBD also plays an important role in maintaining the structural stability of the RBD (Li et al., 2003; Yi et al., 2020; Zhou et al., 2020).
The current challenge faced by the health sector is the resistance and insensitivity of the virus to existing drugs, and those drugs that have an edge over the virus were found to have some detrimental side effects. Drugs such as hydroxychloroquine and chloroquine (FDA-approved drugs that are effective against malaria, lupus, and rheumatoid arthritis) were found to hamper this viral infection, but the risks of developing cardiovascular and renal disorders were found in many of its consumers (FDA, 2020). Also, the recovery rate fluctuated from region to region, in fact, from person to person, with varying degrees of side-effects, forcing the WHO to halt the solidarity trial of hydroxychloroquine a few months after the COVID-19 outbreak.
In silico techniques play an important role in accelerating research to identify potential leads against SARS-CoV-2. Molecular docking, molecular dynamic simulation and drug repurposing are the strategies currently practiced for drug development against COVID-19 (Acharya et al., 2020). Molecular dynamic simulation studies futher help to substantiate the reciprocity between the protein and the ligand. Such tools can be exploited for drug developmental studies which further aid in lead optimization with increased specificity and selectivity (Raudah et al., 2020). Various herbs and plant-based compounds are being tested for possible antiviral activity against SARS-CoV-2 (Anand et al., 2021). Ui, also called gutweed or grass kelp, a common but often unnoticed macro alga, was mainly studied for its anti-microbial and anti-cancer properties in vitro, however, few studies were published on its anti-viral activity (Morán-Santibañez et al., 2016; Klongklaew et al., 2020). It is a member of the Ulvaceae family, which belongs to the Chlorophyta (green seaweed) division (Class: Ulvophyceae, Order: Ulvales). It is found to be a euryhaline and thus can grow even in freshwaters, exclusively in nutrient-rich niches such as in water bodies that receive industrial and farm discharges, and low tidal zones. These tubular algae can reach up to 0.3 m in length, with a thickness of about 0.02 m, and exhibit a perennial isomorphic biphasic reproductive cycle. Considering its abundance in the Coromandel coastline of South India, and its possible action against viruses such as the measles Morbillivirusin Vero cell lines (Morán-Santibañez et al., 2016), Ui was considered as the source of phytochemicals that can serve as possible lead compounds against the S protein RBD of SARS-CoV-2.
Materials and Methods
Sample Collection and Preparation
The alga Ui were collected from the Olaikuda area (Gulf of Mannar) situated near North Mandapam, Rameswaram, Tamil Nadu, India, with the help of the Central Marine Fisheries Research Institute, Mandapam, and Rajendra Kumar Algae Project Center, Mandapam. The algal sample was washed thoroughly with water to remove dirt and debris and packed safely in polythene zip-lock bags. Upon reaching the laboratory it was dried using a tray drier (Figures 1A,C), mainly to concentrate the extract, preserve the hydrolabile compounds, and prevent the growth of bacteria and mold.
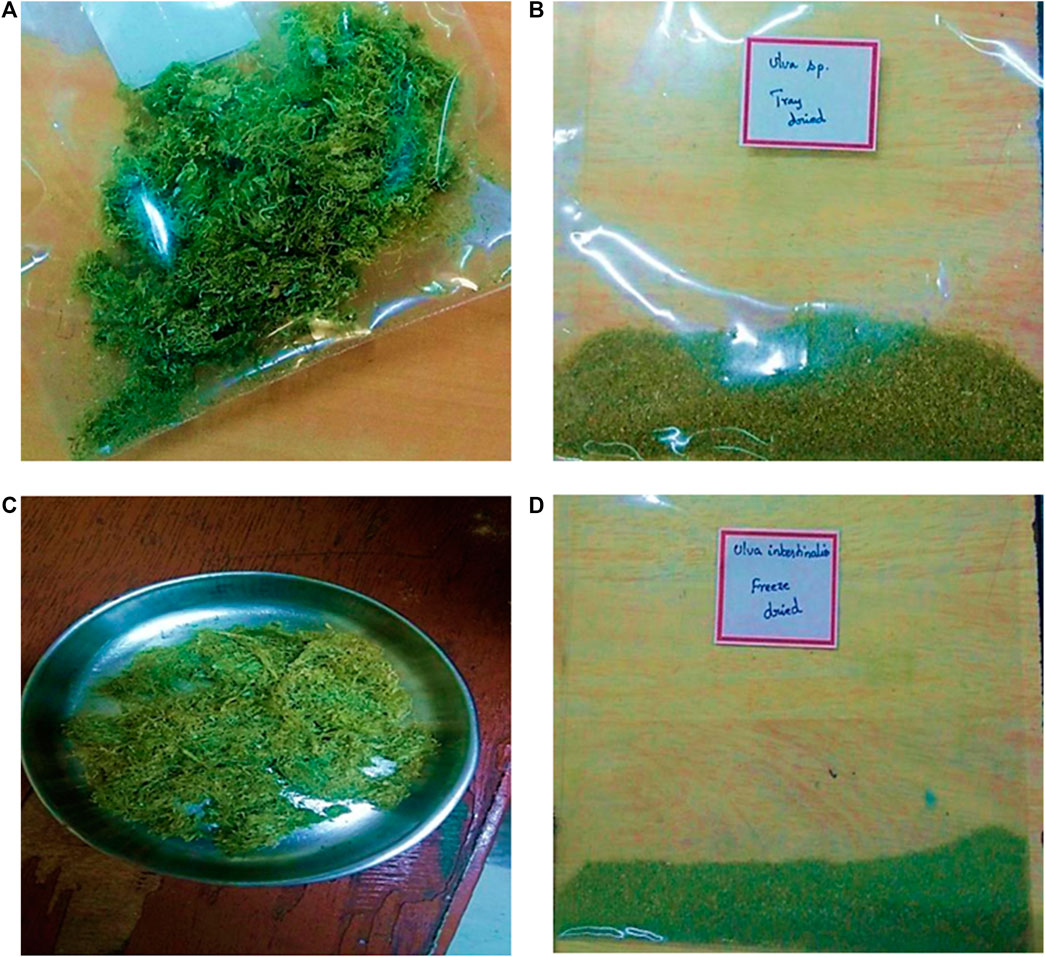
FIGURE 1. Samples of U. intestinalisused for Soxhlet extraction using ethanol. (A) Tray-dried sample (B) powdered form of tray-dried sample. (C) Freeze-dried sample (D) powdered form of freeze-dried sample.
Isolation and Identification of Phytochemicals
Phytochemical extraction was performed by Soxhlet extraction. The dried sample (∼60 g) was pulverized using a mortar and pestle (Figures 1B,D), and transferred into a thimble in the extraction tube. The extraction solvent used was 95% ethanol (100 ml). The all-glass Soxhlet apparatus was set up according to the standard protocol and was run for 6 h at 78°C using an isomantle. The extract was analyzed for the phytochemicals using a 7890B GC coupled with a 5977A mass selective detector (MSD). The chromatographic column used for GC was HP-5MS of dimensions 30 m × 250 μm × 0.25 μm (length, inner diameter, and film thickness, respectively). It is a bonded, cross-linked, and solvent-rinsable non-polar column made of (5%-phenyl)-methylpolysiloxane, with a capillary tubing made of fused silica (Agilent Technologies, Santa Clara, CA). The volume of the sample injected was 1 μl and the flow rate of the carrier gas (helium) was 1.0 ml.min−1 with a split ratio of 1:1. The injection port temperature was 250°C. The system started with a 2 min-hold at 50°C, then ramped 3°C per minute until the temperature reached 270°C. The system was on hold at this temperature for 20 min. Simultaneously, the separated samples were fed automatically to the MSD at an interface temperature of 280°C. The electron ionization was performed at 70 eV, and the scan range of the system was 40–700 m/z. The total run time of the process was 95 min. The retention indices of the compounds were determined relative to trichloromethane, the standard compound selected for data analysis. Further, the compounds were identified by comparing their mass spectra with the data in NIST-14 Mass Spectral Data Library.
Preparation of Ligands and Target
The three-dimensional chemical structures of the identified phytochemicals were obtained from PubChem (https://pubchem.ncbi.nlm.nih.gov/). These were then saved as SDF files. The energy minimization and format conversion of these structures were performed in PyRx software (Dallakyan and Olson 2015). The default energy minimization parameters were the universal force field and the conjugate gradient algorithm. Once energy minimization was completed, the structures were rewritten as PDBQT files. The target protein used in this study was S1 receptor binding domain of the spike (S) glycoprotein. The three-dimensional structure of RBD was retrieved from a complex of ACE2 and RBD (PDB ID: 6M0J) from the Protein Data Bank (RCSB-PDB; https://www.rcsb.org/). As the first step, the optimization of protein structures was performed using AutoDock Tools by deleting chain A, water molecules, and co-crystal ligands. The missing atoms were then repaired, and polar hydrogens were added. Charges were distributed and minimized over the protein structure. The structure was then saved in PDBQT format.
Active Site Prediction and Grid Box Parameters
An active site is defined as a groove or pocket of an enzymatic or non-enzymatic protein which facilitates ligand binding or biochemical reactions (Pravda et al., 2014). The characteristics of the active site are mainly determined by the active site residues (Srinivasan, 2020), and various studies have characterized the possible active site residues of RBD of S1 subunit of spike protein (Figure 2). Tyr449, Tyr453, Arg454, Lys458, Ser459, Ser469, Glu471, Phe486, Asn487, Tyr489, Leu492, Gln493, Gly496, Gln498, Thr500, Asn501, Gly502, and Tyr505 were the reported active site residues (Lan et al., 2020; Kulkarni et al., 2020; Prajapat et al., 2020). These residues were further validated using the ‘Zone’ function in UCSF Chimera software (https://www.cgl.ucsf.edu/chimera/). The zone parameter was set to “<5.0 Å from currently selected atoms” (Ashraf et al., 2014), where the currently selected atoms were the atoms of chain A. The mean of the X, Y, and Z coordinates of the final atom of each interacting residue highlighted by UCSF Chimera was calculated and applied as the dimension of the grid-box center. The grid size was manually adjusted to cover the interacting residues. Further, the values of these coordinates were saved as a configuration text file which was later used for docking.
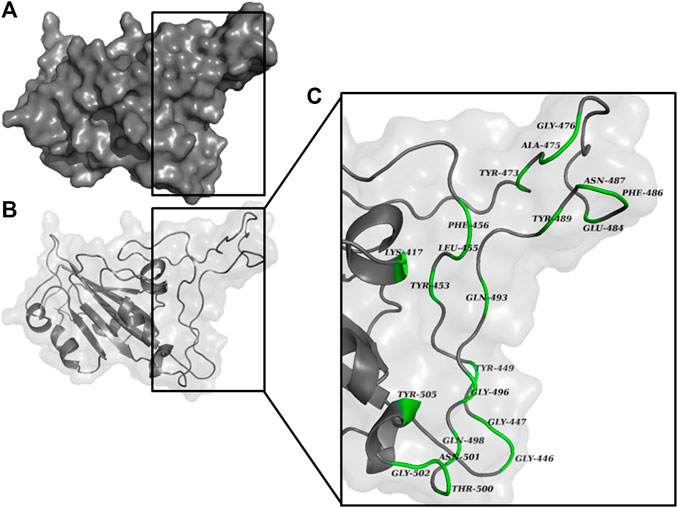
FIGURE 2. RBD of S1 protein represented as (A) surface, and (B) chain. The magnified view of the RBD (C) shows the possible interacting residues (green) in <5.0 Å vicinity with ACE2.
Molecular Docking and Target-Ligand Visualization
Molecular docking is an in silico approach which is used to predict the conformational binding energy of ligands to a preferred target using matching and scoring algorithms (Leach et al., 2006). In this experiment, we have used AutoDockVina (Trott and Olson, 2010) in PyRx software as the docking tool, The optimal binding energy of the ligands was obtained based on least root mean square deviation (RMSD) for each conformers of a particular ligand, and arranged in ascending order to select the best ligand(s) for further calculating the chemical behaviour using C-DFT and pharmacokinetic analyses. PyMOL (https://pymol.org/), an open-source molecular visualization software was used to identify the polar contacts (H-bonds) between the ligand and the interacting active site residue, and develop printable figures of this interaction. To analyze hydrophobic interactions between the ligand and residues, another visualization software, BIOVIA Discovery Studio Client 2020 (https://discover.3ds.com/discovery-studio-visualizer-download) was used.
Conceptual DFT Analysis
Conceptual Density-functional theory (C-DFT) is a computational method to predict chemical behaviour of the compounds (Poater et al., 2010; Domingo et al., 2016). Density-functional theory(DFT) has been developed from Hohenberg-Kohn theorem, which is an in-silico quantum mechanical modeling strategy used to determine the properties of a many-electron systems, using spatially-dependent electron density functionals (Hohenberg and Kohn, 1964; Kohn and Sham, 1965). C-DFT, a sub-field of DFT, helps to analyze the molecular orbital energies of conformers and can give rise to cues for understanding the structure-activity relationship of the molecule (Parr and Yang, 1989; Geerlings et al., 2003; Sarkar and Chattaraj, 2021a; Sarkar and Chattaraj, 2021b). To describe the orbital properties of a molecule, ten different molecular descriptors, known as the global reactivity descriptors and its derivatives, were considered viz. total energy (Eγ; in eV), molecular dipole moment (Dp; in Debye units), the energy of the lowest unoccupied molecular orbit (LUMO) (ELUMO; in eV), the energy of the highest occupied molecular orbit (HOMO) (EHOMO; in eV), energy gap (ΔE; in eV), absolute hardness (η; in eV), global softness (σ; in eV−1), electronegativity (χ), chemical potential (μ; in eV), and global electrophilicity index (ψ; in eV−1) (Chattaraj et al., 2003; Chattaraj et al., 2006). These molecular descriptors are calculated based on the electron density of molecules using Fukui’s molecular orbital theory (Fukui 1982; Ayers and Parr, 2000). ELUMO and EHOMO are the primary and the most important descriptors which determine the ability of a molecule to accept or donate electrons. Dp is the measure of the total polarity of a system. It is also a positive indicator of the reactivity of the molecule. It was found that the higher the Dp, the greater the reactivity of the molecule (Roy et al., 2006; Mert et al., 2011). The derived descriptors of ELUMO and EHOMO are ΔE, η, σ, χ, μ, and ψ, which also account for the ability of the molecule to interact and contribute to electron sharing or transfer with the target by transiting from HOMO to LUMO. For example, if ΔE is found to be less, the molecule can easily transit from HOMO to LUMO (Chattaraj and Roy, 2007; Bostan et al., 2012). It represents the chemical reactivity and kinetic stability of the molecule; if χ is found to be less, the inhibitory effect of the ligand is higher (Zhan et al., 2003). As the first step in determining these descriptors, the selected ligands were optimized using the Becke-3-parameter, Lee-Yang-Parr (B3LYP) function (Becke 1988; Lee et al., 1988) with 6-311G(2d, p) basis set in Gaussian-16 software (http://gaussian.com/gaussian16/) (Frisch et al., 2016). B3LYP is the most popular functional used in molecular quantum mechanical modeling and is derived from a defined set of atomic/molecular energies and potentials.
Pharmacokinetic and Drug-Likeliness Analyses
The drug-likeliness and pharmacokinetic properties such as Absorption, Distribution, Metabolism, Excretion, and Toxicity (ADMET) of the selected ligands were predicted. The Drug Likeliness Tool (DruLiTo; http://www.niper.gov.in/pi_dev_tools/DruLiToWeb/DruLiTo_index.html), an open-source drug-likeness software developed by the Department of Pharmacoinformatics, National Institute of Pharmaceutical Education and Research (NIPER), Punjab, India was used to analyze drug likeliness by checking whether the ligands violate any of Lipinski’s Rule of Five (RO5), or would pass the Ghose and Veber filters. A reliable online tool for pharmacokinetic predictions of small molecules, pkCSM (http://biosig.unimelb.edu.au/pkcsm/), was used to predict the ADMET properties of the ligands (Pires et al., 2015), in which the canonical or isomeric SMILES of the ligands from Pub Chem were given as input.
Results
Chemical Composition of Extract
The GC-MS data of the Ui ethanolic extract showed 55 peaks (Figure 3), and on comparison with NIST-14 library, 43 known phytochemicals were identified (Table 1). The phytochemical class analysis revealed that 18 phytochemicals were simple carboxylic acids, fatty acids, or their derivatives (palmitic acid, HIP, methylpalmitate, ethylpalmitate, butanoic acid, paullinic acid, doconexent, allyl stearate, ethyllinolelaidate, ethyllinolenate, ethylelaidate, icosapent, MHDTE, BOD4E, BOD3E, BTES, DPPP, and propyllinoleate), seven belonged to terpenoid class (damascene, cyclosativene, dihydroactinolide, 3-DOCH, phytol, CMBA, and oxymesterone), three (6.98%) each were aldehydes and its derivatives (methylglyoxal, furfural, and retinal), alcohols and its derivatives (TAA, 1-heptatriacotanol, and DTD), alkene hydrocarbons and its derivatives (cetene, 8-heptadecene, and 9-octadecene), and alkane hydrocarbons and its derivatives (myristyl chloride, TMHA, and EEBOD), two were monoglycerides (2-monopalmitin and 1-monolinolein), and one each were an organosulfur compound (DMSO), an aromatic hydrocarbon (azulene), a phenol (2,4-DtBP), and a glycoside (RBGUL). The peak corresponding to HIP showed the highest signal abundance of >2.8×107, however, the mean relative peak area of phytol (21.404%) was found to be the widest, followed by 2-monopalmitin, 9-octadecene, palmitic acid, and other compounds. The details of the GC-MS analysis such as peak number(s), retention time(s), and mean relative peak area are presented in Table 1.
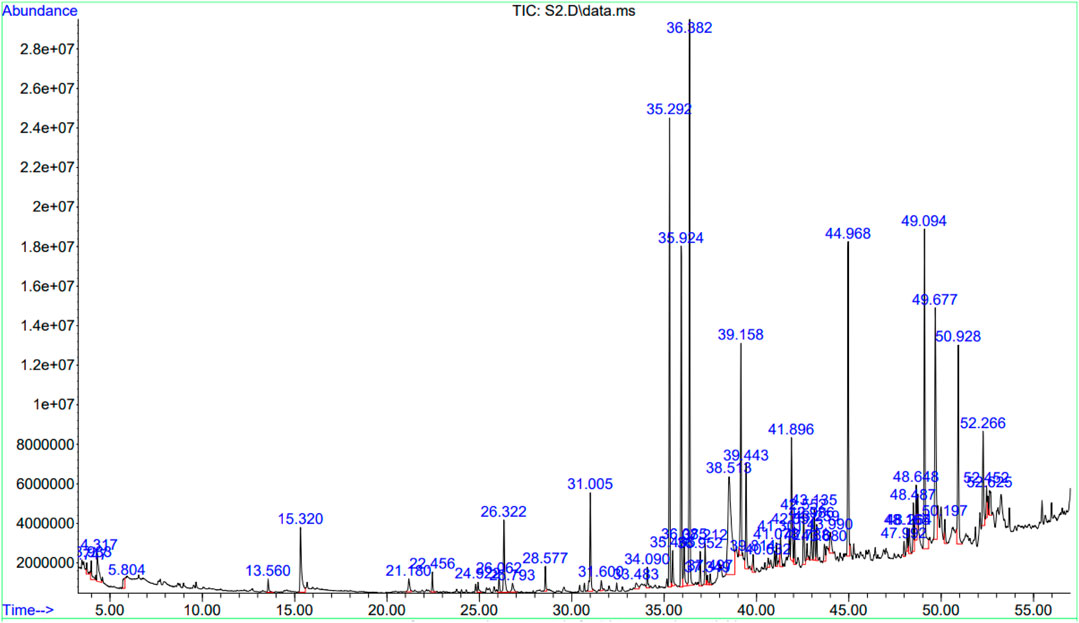
FIGURE 3. Chromatogram showing the results of GC-MS. The chromatogram was plotted against retention time in minutes (X-axis), and signal abundance (Y-axis). The collected fractions were fed automatically into an MS.
Molecular Docking
Hydroxychloroquine, the control ligand, showed a binding affinity of −5.7 kcal.mol−1 with the optimized structure of RBD. Twenty-one (48.84%) compounds had binding energies ranging from −4.0 kcal.mol−1 to −4.8 kcal.mol−1. Out of the 43 compounds, only 16 were considered for studying their molecular interaction (Tables 2,3). Interaction analysis revealed that furfural had three hydrogen bonds interacting with Arg454, Ser469, and Glu471, but its binding energy was -3.8 kcal mol−1. Considering hydrophobic interactions, icosapent interacted with Arg403, Tyr453, Tyr495, Phe497, and Tyr505. The binding energy of this molecule was −4.8 kcal.mol−1. Out of these 16 compounds, only the best five compounds (2,4-DtBP, doconexent, DTD, RBGUL, and retinal) were considered for C-DFT, drug-likeliness studies using DruLiTo, and ADMET properties using pkCSM. The criteria used for this selection was mainly their relative lower binding energy. The conformations were visualized using PyMOL software and depicted in Figures 4–9.
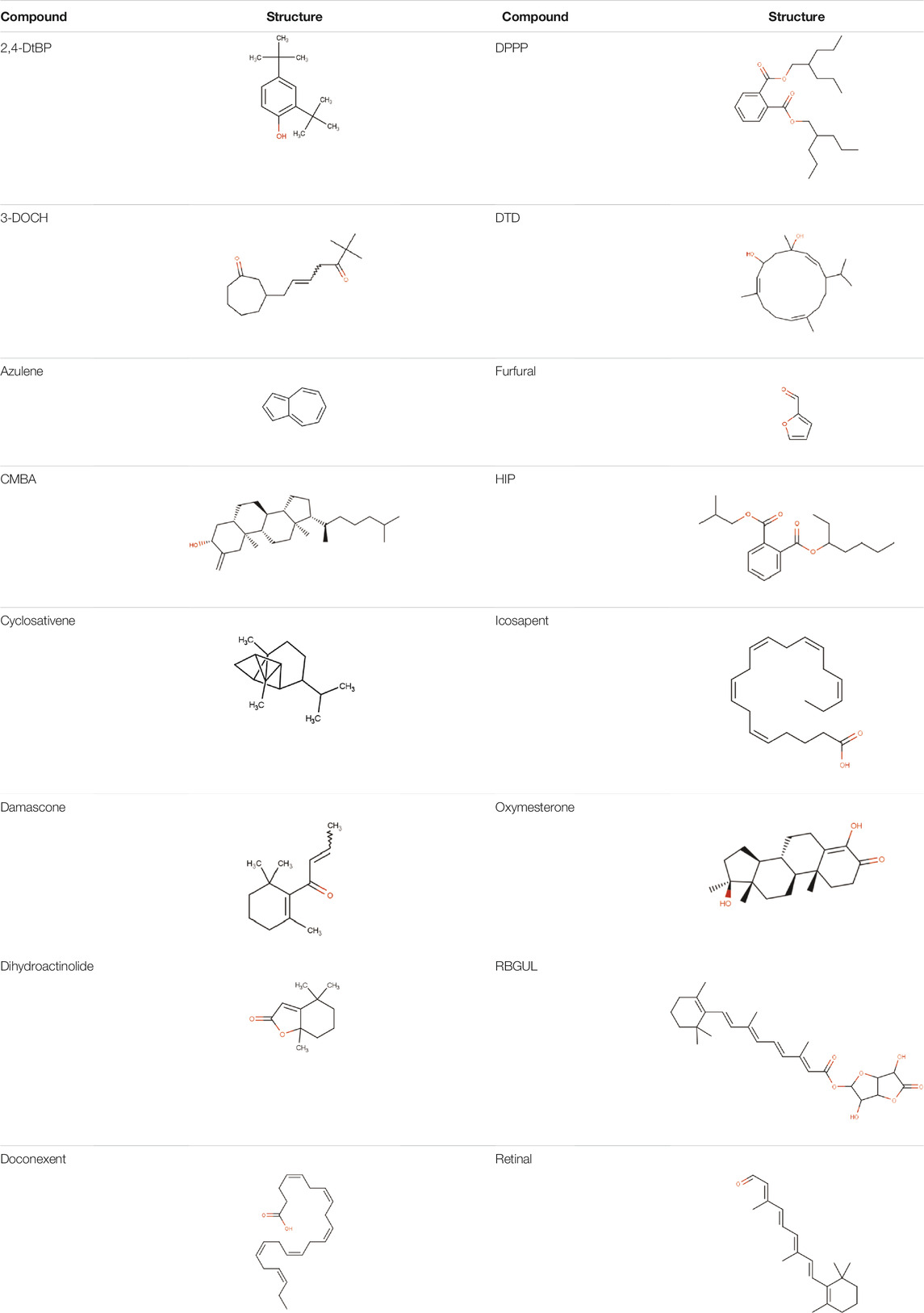
TABLE 2. List of selected compounds identified from Ui extract with their two-dimensional chemical structures.
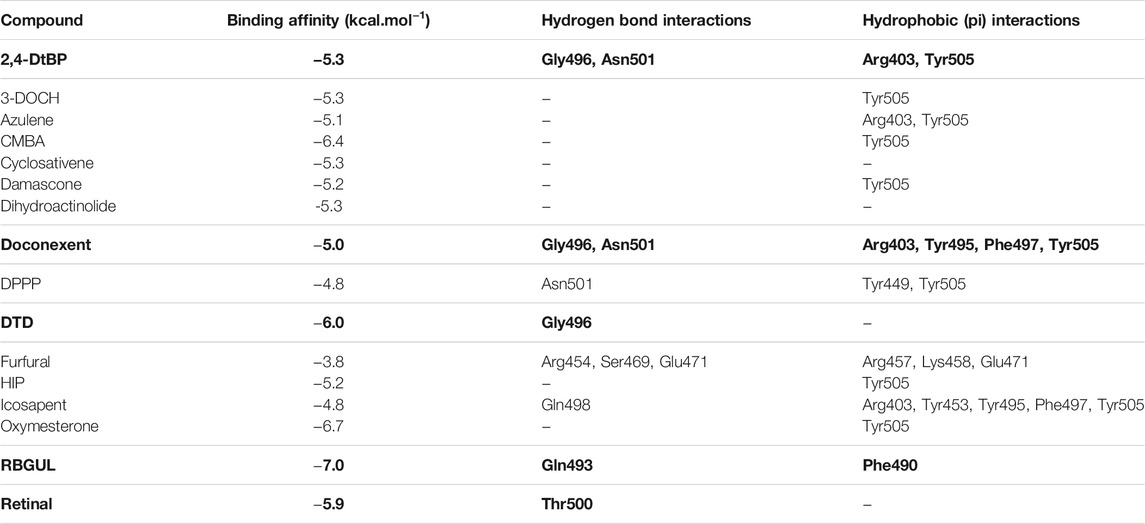
TABLE 3. | The binding affinities of selected phytochemicals from Ui extract on SARS-CoV-2 spike RBD with the interacting amino acid residues contributing towards hydrogen bonds and hydrophobic interactions. The top five high scoring compounds have been highlighted (bold).
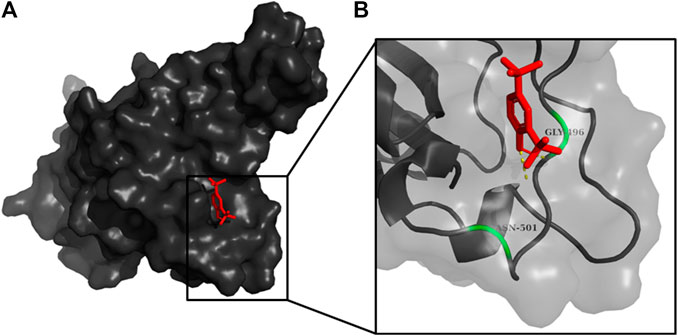
FIGURE 4. (A) The binding pose of 2,4-DtBP (red) in RBD of S1 protein. (B) The hydrogen bonds (yellow) formed between 2,4-DtBP and the interacting residues, Gly496 and Asn501 are also shown.
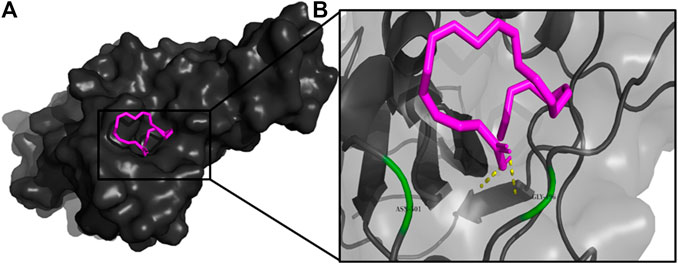
FIGURE 5. (A) The binding pose of doconexent (magenta) in RBD of S1 protein. (B) The hydrogen bonds (yellow) formed between doconexent and the interacting residues, Gly496 and Asn501 are also shown.
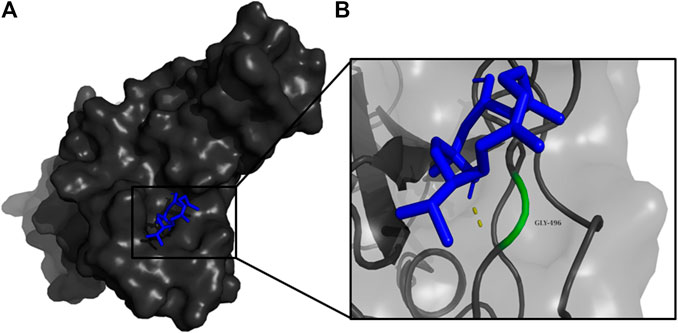
FIGURE 6. (A) The binding pose of DTD (blue) in RBD of S1 protein. (B) The hydrogen bond (yellow) formed between DTD and the interacting residue, Gly496, is also shown.
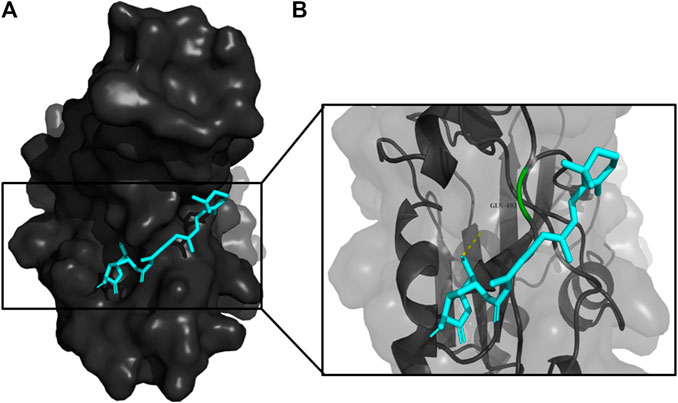
FIGURE 7. (A) The binding pose of RBGUL (cyan) in RBD of S1 protein. (B) The hydrogen bond (yellow) formed between RBGUL and the interacting residue, Gly493, is also shown.
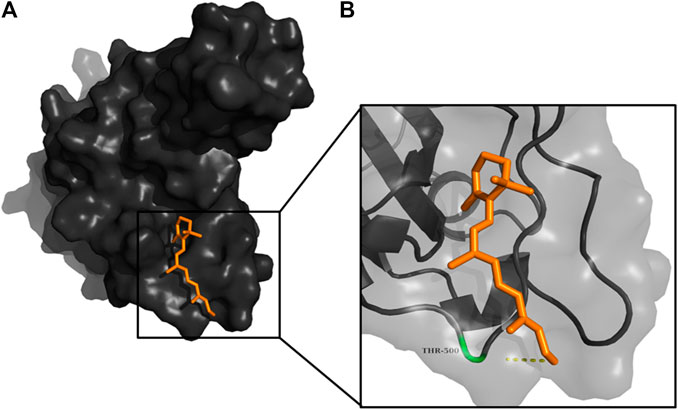
FIGURE 8. (A) The binding pose of retinal (orange) in RBD of S1 protein. (B) The hydrogen bond (yellow) formed between retinal and the interacting residue, Thr500, is also shown.
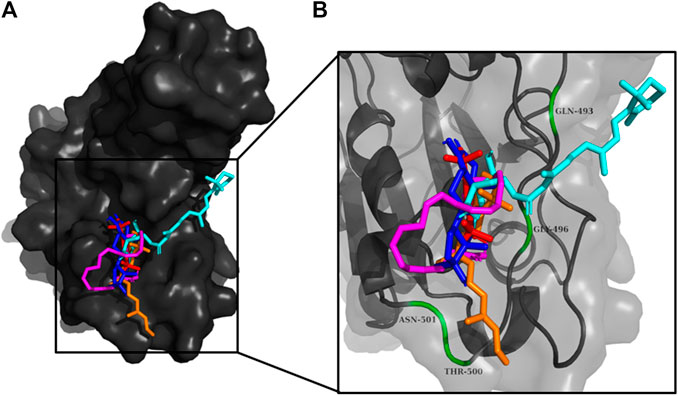
FIGURE 9. (A) The binding poses of the selected compounds from U. intestinalisextract in one of the active binding pockets of RBD of S1 protein. (B) The pocket residues interacting with the compounds are highlighted in green.
Estimated Descriptors of Conceptual DFT
The molecular descriptors were calculated after optimization, based on the FMO theory (Table 4). The total energy of the compounds is the total electron energy of the ground state. Lower the total energy, higher is their stability. RBGUL displayed the lowest total energy with value −41.84 × 103 eV. Molecular orbital energies such as HOMO energy (EHOMO) and LUMO energy (ELUMO) were calculated and analyzed (Table 5). Retinal showed the least energy gap with an energy difference of 3.04 eV. The energy gap of RBGUL (ΔE = 3.20 eV) was also found to be close enough to that of retinal. The maximum Dp was also shown by retinal (Dp = 6.33 Debye units). Considering derived descriptors, the most electronegative compound in the selected list was retinal (χ = 3.82). The electronegativity of RBGUL (χ = 3.67) was found to be highly similar to that of retinal. Absolute hardness and Global softness are criterions of overall stability of the system and also they are supporting parameters of electronegativity. In our study Retinal and RBGUL showed acceptable values of absolute hardness, 1.52 and 1.60 and softness, 0.33 and 0.31, respectively. Chemical potential of compounds is the negative value of electronegativity values, which is also an indication of high chemical activity. Therefore in this case too, retinal and RGBUL exhibited high chemical potential. High electrophilicity of retinal (4.80) and RBGUL (4.21) suggests their elevated likeliness to accept electrons. According to the above findings, RBGUL, and retinal were considered good inhibitors of S1 RBD of SARS-CoV-2.

TABLE 4. | Statistics of the conceptual DFT-global reactivity descriptors and their derivatives of the best phytochemicals.
Prediction of Pharmacokinetic Properties and Drug-Likeliness
The drug-likeliness prediction from DruLiTo and ADMET results from pkCSM are presented in Table 6. Evaluation of drug-likeliness showed that 2,4-DtBP satisfied and passed through the Lipinski’s RO5, Ghose, and Veber filters, whereas other ligands violated atleast one of the three parameters. Absorption properties revealed that all ligands were readily absorbed intestinally. 2,4-DtBP, doconexent, DTD, and retinal showed no interference with the P-glycoprotein system, however, RBGUL was found to be both a substrate and an inhibitor in the system. Skin permeability prediction showed that 2,4-DtBP was slightly permeable. Distribution properties showed that these compounds have tendencies to cross the blood-brain barrier (BBB) and central nervous system (CNS). Metabolic properties revealed that no ligand escaped the cytochrome P450 (CYP) system of the liver completely. Amongst the five selected ligands, DTD and RBGUL showed minimum interference with the system (acted as CYP2C19 inhibitor and CYP3A4 substrate, respectively). Considering excretion and toxicity properties, no ligand acted as renal OCT2 substrate, and human ether-à-go-go-related gene (hERG)-I protein inhibitors. The compounds passed the Ames toxicity test, indicating their inability to be a mutagen and thus a carcinogen. However, hepatotoxicity was predicted with doconexent, RBGUL, and retinal. Except for RBGUL, all other selected ligands showed skin sensitization too.
Discussion
Medicine has started to change from completely “synthetic” to “semi-herbal” in the last couple of decades. Due to the lack of effective treatment and management strategies to treat COVID-19, alternative therapies are being explored. Conventional drug development process involves elaborate and time-consuming protocols, and they seldom produce drugs on demand. To increase the complexity, the causative agent, SARS-CoV-2, is a virus with high mutability and variable reproduction number (Rahman et al., 2020) that is slightly greater than its pathological cousins, SARS-CoV and MERS-CoV (Liu et al., 2020). Due to these facts, it is challenging to develop drugs against this virus presently. However, drugs could be developed against conserved regions of its genome or proteins encoded from these regions, such as spike glycoprotein or main protease, and intense research is being conducted world-wide, for the same. Drug repurposing is the most accepted strategy considered in this approach. Using in silico techniques, commercially available drugs are docked with a target protein, and the screened drug could be made available for patients within a much shorter period because the clinical profile of the drug has been already established. Some drugs repurposed against SARS-CoV-2 were Remdesivir, Favipiravir, Ribavirin, Lopinavir, Ritonavir, Darunavir, Tocilizumab, type I and type II interferons, chloroquine, hydroxychloroquine, arbidiol and statins (Singh et al., 2020). Though it is a fast-paced approach, in vitro and in vivo studies are required to fully understand its mechanism in the human body, especially when the stakes of comorbid symptoms are high with this disease.
The undesirable side-effects of synthetic drugs has attracted researchers, and scientists towards developing plant-based medicines. Various compounds obtained fromt extracts of plants that belong to families such as Lamiaceae, Fabaceae, Geraniaceae, Rosaceae, Asteraceae, Rutaceae and Malvaceaehave been reported to exhibit antiviral activity against SARS-CoV-2 and certain other viruses too (Drevinskas et al., 2018; Denaro et al., 2020; Siddiqui et al., 2020). The top compounds identified as potent antivirals in our study have been previously reported to have exhibited a wide array of functions. 2,4-DtBP is a lipophilic phenol found mostly in higher plants. The phenol and its analogs were reported to have anti-oxidant, anti-inflammatory, anti-cancer, and anti-microbial properties. Considering their anti-viral activities, they reduced the growth of Coxsackievirus B-3 and Herpes Virus type-2 (Zhao et al., 2020). Our study revealed that 2,4-DtBP binds to S1 RBD of SARS-CoV-2 with a binding energy of −5.3 kcal.mol−1, and interacted with Gly496 and Asn501 by hydrogen bonds and Arg403 and Tyr505, hydrophobically. Doconexent is a fatty acid which is rich in docosahexaenoic acid (DHA), is a compound with high anti inflammatory properties which is commercially produced from certain microalgae (Milledge, 2011). It has been repurposed to treat cancer and COVID-19 (Li et al., 2020; Singhal et al., 2020; Stanly et al., 2020). Retinal is a vitamin A aldehyde in the most absorbable form. Many studies have pointed the role of vitamins which include retinal, in managing COVID-19 (Michele et al., 2020; Morais et al., 2020; Gröber and Holick, 2021). DTD is a macrocyclicditerpene, primarily isolated from the Tobacco plant (Nicotianatabacum). It was found to be a major constituent in the oil extract from the aerial parts of Hercules’ all-heal (Opopanaxchironium) (Maggio et al., 2013) and has a structural similarity with cembrene (Roberts and Rowland, 1962). Though DTD was not studied for its clinical properties, it was found that cembrenoid derivatives showed anti-cancer properties in vitro (Jassbi et al., 2017). With a binding affinity of −6.0 kcal.mol−1 against SARS-CoV-2, it proved to be a good inhibitor of the virus. RBGUL has similar properties to retinoic acid, and retinol. It was proposed to be a valuable therapeutic compound for the treatment of dermatological conditions and certain cancers, and also a dose-dependent teratogen (Barua, 1997). In our study, RBGUL was found to be the best inhibitor of SARS-CoV-2, compared to the other compounds with good binding affinity to the virus (−7.0 kcal.mol−1).
In silico techniques occupy a prominent role in early drug discovery process. A quantitative computational study of the interaction between a particular protein target and a set of ligands, provides a fair idea as to which of the ligands may have an effect on the protein in vitro. Screening a large number of compounds against a particular target to narrow down the number of compounds to be tested in vitro is easily achievable by bioinformatics techniques. Molecular docking aids in assessing and visualizing the interactions between the ligands and protein. Similarly, the C-DFT study performed by calculating global molecular descriptors based on DFT provides a quantum level understanding of the ligands and helps to construct the relationship between their electronic properties and biological activity. It can also be used to understand the quantitative structure-activity relationship and perform pharmacophore modeling to design effective drugs out of the existing, according to the target. RBGUL and retinal show similar electron density in the orbitals except that the structures look inverted, suggesting that the inhibitory action of both compounds may be similar. They were also considered as highly active compounds as they showed low ΔE, which helps in an easy transition from HOMO to LUMO. Comparing the results of docking and C-DFT, the compounds with higher electronegativity showed better activity. Thus it can be comprehended that smaller ΔE, high Dp, and low electronegativity are essential for the inhibitory effect of a molecule. However, compared to RBGUL, retinal had more disadvantages based on the pharmacokinetic predictions. Besides RBGUL, 2,4-DtBP is also a potential candidate against RBD of SARS-CoV-2, considering its less adverse effects. That being said, the most recommended inhibitors against RBD would be 2,4-DtBP and RBGUL. More studies on these phytochemicals can reveal their efficacy, thus validating the results of this experiment.
Conclusion
Phytochemicals obtained from Ui extract were docked with the SARS-CoV-2 RBD to ascertain if it exhibited antiviral activity, and also to screen for the compounds that are responsible for the activity. Through this study, we conclude that RBGUL, 2,4-DtBP and Retinal could be used as potent inhibitors against the RBD of coronavirus based on the molecular docking, C-DFT and ADMET studies. However, further studies involving in vitro and in vivo testing is essential to confirm the antiviral efficiency of the compounds against SARS-CoV-2.
Data Availability Statement
The raw data supporting the conclusions of this article will be made available by the authors, without undue reservation.
Author Contributions
TM and HS contributed to the conception, design, and data acquisition. SK and SB drafted the manuscript. SK, BC, and KB contributed to data analysis and have critically revised the manuscript. All authors have given final approval and have agreed to be accountable for all aspects of the work.
Funding
This study was supported by the Computational Biology Lab, funded by SERB Young Scientist grant (SB/YS/LS-128/2013). This work was supported by the National Research Foundation of Korea (NRF) grant funded by the Korean government (MSIT) (No. 2021R1F1A1062300). This study was also supported by a research fund from Chosun University, 2021. Authors also express gratitude to StemOnc R and D Private Ltd. and Mr. M. Mahesh Kumar (Assistant Professor, Food and Process Engineering Department, SRMIST, Chennai) for helping through the compound extraction.
Conflict of Interest
The authors declare that the research was conducted in the absence of any commercial or financial relationships that could be construed as a potential conflict of interest.
Publisher’s Note
All claims expressed in this article are solely those of the authors and do not necessarily represent those of their affiliated organizations, or those of the publisher, the editors and the reviewers. Any product that may be evaluated in this article, or claim that may be made by its manufacturer, is not guaranteed or endorsed by the publisher.
References
Acharya, A., Agarwal, R., Baker, M. B., Baudry, J., Bhowmik, D., Boehm, S., et al. (2020). Supercomputer-Based Ensemble Docking Drug Discovery Pipeline with Application to COVID-19. J. Chem. Inf. Model. 60 (12), 5832–5852. doi:10.1021/acs.jcim.0c01010
Anand, A. V., Balamuralikrishnan, B., Kaviya, M., Bharathi, K., Parithathvi, A., Arun, M., et al. (2021). Medicinal Plants, Phytochemicals, and Herbs to Combat Viral Pathogens Including SARS-CoV-2. Molecules 26 (6), 1775. doi:10.3390/molecules26061775
Ashraf, Z., Saeed, A., and Nadeem, H. (2014). Design, Synthesis and Docking Studies of Some Novel Isocoumarin Analogues as Antimicrobial Agents. RSC Adv. 4 (96), 53842–53853. doi:10.1039/C4RA07223E
Awoonor-Williams, E., and Abu-Saleh, A. (2021). Covalent and Non-Covalent Binding Free Energy Calculations for Peptidomimetic Inhibitors of SARS-CoV-2 Main Protease. Phys. Chem. Chem. Phys. 23 (11), 6746–6757. doi:10.1039/d1cp00266j
Ayers, P. W., and Parr, R. G. (2000). Variational Principles for Describing Chemical Reactions: The Fukui Function and Chemical Hardness Revisited. J. Am. Chem. Soc. 122 (9), 2010–2018. doi:10.1021/ja9924039
Barua, A. B. (1997). Retinoyl β-glucuronide: A Biologically Active Form of Vitamin A. Nutr. Rev. 55 (7), 259–267. doi:10.1111/j.1753-4887.1997.tb01615.x
Becke, A. D. (1988). Density-Functional Exchange-Energy Approximation with Correct Asymptotic Behavior. Phys. Rev. A. Gen. Phys. 38 (6), 3098. doi:10.1103/PhysRevA.38.3098
Bette, K., Will, M. F., Sandrasegaram, G., Hyejin, Y., and James, T. (2021). Tracking Changes in SARS-CoV-2 Spike : Evidence that D614G Increase Infectivity of the COVID-19 Virus. Cell 182 (4), 812–827. doi:10.1016/j.cell.2020.06.043
Bostan, R., Varvara, S., Găină, L., and Mureşan, L. M. (2012). Evaluation of Some Phenothiazine Derivatives as Corrosion Inhibitors for Bronze in Weakly Acidic Solution. Corrosion Sci. 63, 275–286. doi:10.1016/j.corsci.2012.06.010
Chattaraj, P. K., Maiti, B., and Sarkar, U. (2003). Philicity: a Unified Treatment of Chemical Reactivity and Selectivity. The J. Phys. Chem. A 107 (25), 4973–4975. doi:10.1021/jp034707u
Chattaraj, P. K., and Roy, D. R. (2007). Update 1 of: Electrophilicity Index. Chem. Rev. 107 (9), PR46–PR74. doi:10.1021/cr078014b
Chattaraj, P. K., Sarkar, U., Roy, D. R., Elango, M., Parthasarathi, R., and Subramanian, V. (2006). Is Electrophilicity a Kinetic or a Thermodynamic Concept? Indian J. Chem. 45, 1099–1112.
Dallakyan, S., and Olson, A. J. (2015). “Small-Molecule Library Screening by Docking with PyRx,” in Chemical Biology (New York, NY: Humana Press), 243–250. doi:10.1007/978-1-4939-2269-7_19
Denaro, M., Smeriglio, A., Barreca, D., De Francesco, C., Occhiuto, C., Milano, G., et al. (2020). Antiviral Activity of Plants and Their Isolated Bioactive Compounds: An Update. Phytotherapy Res. 34 (4), 742–768. doi:10.1002/ptr.6575
Domingo, L. R., Ríos-Gutiérrez, M., and Pérez, P. (2016). Applications of the Conceptual Density Functional Theory Indices to Organic Chemistry Reactivity. Molecules 21 (6), 748. doi:10.3390/molecules21060748
Drevinskas, T., Mickienė, R., Maruška, A., Stankevičius, M., Tiso, N., Šalomskas, A., et al. (2018). Confirmation of the Antiviral Properties of Medicinal Plants via Chemical Analysis, Machine Learning Methods and Antiviral Tests: A Methodological Approach. Anal. Methods 10 (16), 1875–1885. doi:10.1039/C8AY00318A
FDA (2020). FDA Cautions against Use of Hydroxychloroquine or Chloroquine for COVID-19 outside of the Hospital Setting or a Clinical Trial Due to Risk of Heart Rhythm Problems. Available at: https://www.fda.gov/drugs/drug-safety-and-availability/fda-cautions-against-use-hydroxychloroquine-or-chloroquine-covid-19-outside-hospital-setting-or (Accessed November 23, 2020).
Frisch, M., Trucks, G., Schlegel, H., Scuseria, G., Robb, M., Cheeseman, J., et al. (2016). Gaussian 16. (Version Revision B. 01)[Linux]. Wallingford CT: Gaussian.
Fukui, K. (1982). The Role of Frontier Orbitals in Chemical Reactions (Nobel Lecture). Angew. Chem. Int. Edition English 21 (11), 801–809. doi:10.1002/anie.198208013
Ge, H., Wang, X., Yuan, X., Xiao, G., Wang, C., Deng, T., et al. (2020). The Epidemiology and Clinical Information about COVID-19. Eur. J. Clin. Microbiol. Infect. Dis. 39 (6), 1011–1019. doi:10.1007/s10096-020-03874-z
Geerlings, P., DeProft, F., and Langenaeker, W. (2003). Conceptual Density Functional Theory. Chem. Rev. 103 (5), 1793–1874. doi:10.1021/cr990029p
Gröber, U., and Holick, M. F. (2021). The Coronavirus Disease (COVID-19)A Supportive Approach with Selected Micronutrients. Int. J. Vitamin Nutr. Res., 1–12. doi:10.1024/0300-9831/a000693
Hohenberg, P., and Kohn, W. (1964). Density Functional Theory (DFT). W.J.P.R. Phys. Rev. 136, B864. doi:10.1103/physrev.136.b864
Jassbi, A. R., Vafapour, M., Shokrollahi, A., Firuzi, O., Zare, M., Chandran, J. N., et al. (2017). Cytotoxic Activity of Two Cembranoidditerpenes from Nicotianasylvestris against Three Human Cancer Cell Lines. Open Bioactive Compd. J. 5 (1), 1–8. doi:10.2174/1874847301705010001
Klongklaew, N., Praiboon, J., Tamtin, M., and Srisapoome, P. (2020). Antibacterial and Antiviral Activities of Local Thai green Macroalgae Crude Extracts in pacific white Shrimp (Litopenaeusvannamei). Mar. Drugs 18 (3), 140. doi:10.3390/md18030140
Kohn, W., and Sham, L. J. (1965). Self-consistent Equations Including Exchange and Correlation Effects. Phys. Rev. 140 (4A), A1133. doi:10.110310.1103/physrev.140.a1133
Kulkarni, S. A., Nagarajan, S. K., Ramesh, V., Palaniyandi, V., Selvam, S. P., and Madhavan, T. (2020). Computational Evaluation of Major Components from Plant Essential Oils as Potent Inhibitors of SARS-CoV-2 Spike Protein. J. Mol. Struct. 1221, 128823. doi:10.1016/j.molstruc.2020.128823
Lan, J., Ge, J., Yu, J., Shan, S., Zhou, H., Fan, S., et al. (2020). Structure of the SARS-CoV-2 Spike Receptor-Binding Domain Bound to the ACE2 Receptor. Nature 581 (7807), 215–220. doi:10.1038/s41586-020-2180-5
Leach, A. R., Shoichet, B. K., and Peishoff, C. E. (2006). Prediction of Protein− Ligand Interactions. Docking and Scoring: Successes and Gaps. J. Med. Chem. 49 (20), 5851–5855. doi:10.1021/jm060999m
Lee, C., Yang, W., and Parr, R. G. (1988). Development of the Colle-Salvetti Correlation-Energy Formula into a Functional of the Electron Density. Phys. Rev. B Condens Matter 37 (2), 785. doi:10.1103/PhysRevB.37.785
Li, W., Moore, M. J., Vasilieva, N., Sui, J., Wong, S. K., Berne, M. A., et al. (2003). Angiotensin-Converting Enzyme 2 Is a Functional Receptor for the SARS Coronavirus. Nature 426, 450–454. doi:10.1038/nature02145
Liu, Y., Gayle, A. A., Wilder-Smith, A., and Rocklöv, J. (2020). The Reproductive Number of COVID-19 Is Higher Compared to SARS Coronavirus. J. Trav. Med. 27 (2), taaa021. doi:10.1093/jtm/taaa021
Lu, R., Zhao, X., Li, J., Niu, P., Yang, B., Wu, H., et al. (2020). Genomic Characterisation and Epidemiology of 2019 Novel Coronavirus: Implications for Virus Origins and Receptor Binding. The lancet 395 (10224), 565–574. doi:10.1016/S0140-6736(20)30251-8
Maggio, A., Bruno, M., Formisano, C., Rigano, D., and Senatore, F. (2013). Chemical Composition of the Essential Oils of Three Species of Apiaceae Growing Wild in Sicily: Bonanniagraeca, Eryngiummaritimum and Opopanaxchironium. Nat. Product. Commun. 8 (6), 1934578X1300800640. doi:10.1177/1934578X1300800640
Mert, B. D., Mert, M. E., Kardaş, G., and Yazıcı, B. (2011). Experimental and Theoretical Investigation of 3-amino-1, 2, 4-Triazole-5-Thiol as a Corrosion Inhibitor for Carbon Steel in HCl Medium. Corrosion Sci. 53 (12), 4265–4272. doi:10.1016/j.corsci.2011.08.038
Michele, C. A., Angel, B., Valeria, L., Teresa, M., Giuseppe, C., Giovanni, M., et al. (2020). Vitamin Supplements in the Era of SARS-Cov2 Pandemic. GSC Biol. Pharm. Sci. 11 (2), 007–019. doi:10.30574/gscbps.2020.11.2.0114
Milledge, J. J. (2011). Commercial Application of Microalgae Other Than as Biofuels: A Brief Review. Rev. Environ. Sci. Bio/Technology 10 (1), 31–41. doi:10.1007/s11157-010-9214-7
Morais, A. H., Passos, T. S., Maciel, B. L., and da Silva-Maia, J. K. (2020). Can Probiotics and Diet Promote Beneficial Immune Modulation and Purine Control in Coronavirus Infection? Nutrients 12 (6), 1737. doi:10.3390/nu12061737
Morán-Santibañez, K., Cruz-Suárez, L. E., Ricque-Marie, D., Robledo, D., Freile-Pelegrín, Y., Peña-Hernández, M. A., et al. (2016). Synergistic Effects of Sulfated Polysaccharides from Mexican Seaweeds against Measles Virus. Biomed. Research International 2016. doi:10.1155/2016/8502123
Parr, R. G., and Yang, W. (1989). Density-functional Theory of Atoms and Molecules. New York: Oxford University Press. doi:10.1007/978-94-009-9027-2_2
Pavlova, A., Lynch, D. L., Daidone, I., Zanetti-Polzi, L., Smith, M. D., Chipot, C., et al. (2021). Inhibitor Binding Influences the Protonation States of Histidines in SARS-CoV-2 Main Protease. Chem. Sci. 12 (4), 1513–1527. doi:10.1039/D0SC04942E
Pires, D. E., Blundell, T. L., and Ascher, D. B. (2015). pkCSM: Predicting Small-Molecule Pharmacokinetic and Toxicity Properties Using Graph-Based Signatures. J. Med. Chem. 58 (9), 4066–4072. doi:10.1021/acs.jmedchem.5b00104
Poater, A. (2020). Michael Acceptors Tuned by the Pivotal Aromaticity of Histidine to Block COVID-19 Activity. J. Phys. Chem. Lett. 11 (15), 6262–6265. doi:10.1021/acs.jpclett.0c01828
Poater, A., Saliner, A. G., Solà, M., Cavallo, L., and Worth, A. P. (2010). Computational Methods to Predict the Reactivity of Nanoparticles through Structure–Property Relationships. Expert Opin. Drug Deliv. 7 (3), 295–305. doi:10.1517/17425240903508756
Prajapat, M., Shekhar, N., Sarma, P., Avti, P., Singh, S., Kaur, H., et al. (2020). Virtual Screening and Molecular Dynamics Study of Approved Drugs as Inhibitors of Spike Protein S1 Domain and ACE2 Interaction in SARS-CoV-2. J. Mol. Graphics Model. 101, 107716. doi:10.1016/j.jmgm.2020.107716
Pravda, L., Berka, K., Vařeková, R. S., Sehnal, D., Banáš, P., Laskowski, R. A., et al. (2014). Anatomy of Enzyme Channels. BMC bioinformatics 15 (1), 1–8. doi:10.1186/s12859-014-0379-x
Rahman, B., Sadraddin, E., and Porreca, A. (2020). The Basic Reproduction Number of SARS CoV2 in Wuhan is About to die out, How About the Rest of the World?. Rev. Med. Virol. 30 (4), e2111. doi:10.1002/rmv.2111
Ramos-Guzmán, C. A., Ruiz-Pernía, J. J., and Tuñón, I. (2021). A Microscopic Description of SARS-CoV-2 Main Protease Inhibition with Michael Acceptors. Strategies for Improving Inhibitor Design. Chem. Sci. 12 (10), 3489–3496. doi:10.1039/D0SC04978F
Raudah, L., Donghyuk, S., and Sun, C. (2020). Advances in Molecular Dynamics Simulations and Enhanced Sampling Methods for the Study of Protein Systems. Int. J. Mol. Sci. 21 (17), 6339. doi:10.3390/ijms21176339
Roberts, D. L., and Rowland, R. L. (1962). MacrocyclicDiterpenes. α-and β-4, 8, 13-Duvatriene-1, 3-diols from Tobacco. J. Org. Chem. 27 (11), 3989–3995. doi:10.1021/jo01058a056
Roy, D. R., Parthasarathi, R., Padmanabhan, J., Sarkar, U., Subramanian, V., and Chattaraj, P. K. (2006). Careful Scrutiny of the Philicity Concept. J. Phys. Chem. A 110 (3), 1084–1093. doi:10.1021/jp053641v
Sarkar, U., and Chattaraj, P. K. (2021a). Conceptual DFT Based Electronic Structure Principles in a Dynamical Context. J. Indian Chem. Soc. 98 (7), 100098. doi:10.1039/D0SC07017C10.1016/j.jics.2021.100098
Sarkar, U., and Chattaraj, P. K. (2021b). Reactivity Dynamics. J. Phys. Chem. A 125 (10), 2051–2060. doi:10.1021/acs.jpca.0c10788
Siddiqui, A. J., Danciu, C., Ashraf, S. A., Moin, A., Singh, R., Alreshidi, M., et al. (2020). Plants-Derived Biomolecules as Potent Antiviralphytomedicines: New Insights on Ethnobotanical Evidences against Coronaviruses. Plants 9 (9), 1244. doi:10.3390/plants9091244
Singh, T. U., Parida, S., Lingaraju, M. C., Kesavan, M., Kumar, D., and Singh, R. K. (2020). Drug Repurposing Approach to Fight COVID-19. Pharmacol. Rep. 72 (6), 1479–1508. doi:10.1007/s43440-020-00155-6
Singhal, T., Chansoria, A., and Kothari, S. (2020). Evaluation of Anti-Inflammatory Activity of Docosahexanoic Acid on Carrageenan Induced Paw Oedema in Rats. Int. J. Basic Clin. Pharmacol. 9 (5), 718–721. doi:10.18203/2319-2003.ijbcp20201745
Srinivasan, B. (2020). Words of Advice: Teaching Enzyme Kinetics. FEBS J. 288, 2068–2083. doi:10.1111/febs.15537
Stanly, C., Alfieri, M., Ambrosone, A., Leone, A., Fiume, I., and Pocsfalvi, G. (2020). Grapefruit-Derived Micro and Nanovesicles Show Distinct Metabolome Profiles and Anticancer Activities in the A375 Human Melanoma Cell Line. Cells 9 (12), 2722. doi:10.3390/cells9122722
Trott, O., and Olson, A. J. (2010). AutoDockVina: Improving the Speed and Accuracy of Docking with a New Scoring Function, Efficient Optimization, and Multithreading. J. Comput. Chem. 31 (2), 455–461. doi:10.1002/jcc.21334
Walls, A. C., Park, Y. J., Tortorici, M. A., Wall, A., McGuire, A. T., and Veesler, D. (2020). Structure, Function, and Antigenicity of the SARS-CoV-2 Spike Glycoprotein. Cell 181 (2), 281–292. doi:10.1016/j.cell.2020.02.058
Yi, C., Sun, X., Ye, J., Ding, L., Liu, M., Yang, Z., et al. (2020). Key Residues of the Receptor Binding Motif in the Spike Protein of SARS-CoV-2 that Interact with ACE2 and Neutralizing Antibodies. Cell Mol. Immunol. 17 (6), 621–630. doi:10.1038/s41423-020-0458-z
Yokoyama, K., and Ichiki, A. (2021). Nano-Size Dependence in the Adsorption by the SARS-CoV-2 Spike Protein Over Gold Colloid. Colloids Surf. A: Physicochemical Eng. Aspects 615, 126275. doi:10.1016/j.colsurfa.2021.126275
Zanetti-Polzi, L., Smith, M. D., Chipot, C., Gumbart, J. C., Lynch, D. L., Pavlova, A., et al. (2021). Tuning Proton Transfer Thermodynamics in SARS-Cov-2 Main Protease: Implications for Catalysis and Inhibitor Design. J. Phys. Chem. Lett. 12 (17), 4195–4202. doi:10.1021/acs.jpclett.1c00425
Zhan, C. G., Nichols, J. A., and Dixon, D. A. (2003). Ionization Potential, Electron Affinity, Electronegativity, Hardness, and Electron Excitation Energy: Molecular Properties from Density Functional Theory Orbital Energies. J. Phys. Chem. A 107 (20), 4184–4195. doi:10.1021/jp0225774
Zhao, F., Wang, P., Lucardi, R. D., Su, Z., and Li, S. (2020). Natural Sources and Bioactivities of 2, 4-Di-Tert-Butylphenol and its Analogs. Toxins 12 (1), 35. doi:10.3390/toxins12010035
Keywords: SARS-CoV-2 spike S1 subunit, Ulva intestinalis L., phytochemicals, GC-MS, COVID-19, molecular docking, ADMET studies, conceptual DFT
Citation: Kulkarni SA, Krishnan SB, Chandrasekhar B, Banerjee K, Sohn H and Madhavan T (2021) Characterization of Phytochemicals in Ulva intestinalis L. and Their Action Against SARS-CoV-2 Spike Glycoprotein Receptor-Binding Domain. Front. Chem. 9:735768. doi: 10.3389/fchem.2021.735768
Received: 03 July 2021; Accepted: 10 September 2021;
Published: 27 September 2021.
Edited by:
Sugunadevi Sakkiah, National Center for Toxicological Research (FDA), United StatesCopyright © 2021 Kulkarni, Krishnan, Chandrasekhar, Banerjee, Sohn and Madhavan. This is an open-access article distributed under the terms of the Creative Commons Attribution License (CC BY). The use, distribution or reproduction in other forums is permitted, provided the original author(s) and the copyright owner(s) are credited and that the original publication in this journal is cited, in accordance with accepted academic practice. No use, distribution or reproduction is permitted which does not comply with these terms.
*Correspondence: Honglae Sohn, aHNvaG5AY2hvc3VuLmFjLmty; Thirumurthy Madhavan, dGhpcnUubXVydGh5dW5vbUBnbWFpbC5jb20=
†This author have contributed equally to this work