- 1State Key Laboratory of Microbial Metabolism, Joint International Research Laboratory of Metabolic and Developmental Sciences, School of Life Sciences and Biotechnology, Shanghai Jiao Tong University, Shanghai, China
- 2Shanghai Laiyi Center for Biopharmaceutical R&D, Shanghai, China
The recent pandemic caused by SARS-CoV-2 has spread to over 100 countries, infected more than 47 million people and resulted in more than 1.2 million deaths worldwide until October. It is well known that, the SARS-CoV-2 starts an infection by binding its Receptor Binding Domain (RBD) of spike protein to human Angiotensin converting enzyme 2 (ACE2) receptor, and strenuous efforts had been made to prevent the infection. However, no successful drugs or vaccines have appeared. Herein, molecular docking and molecular simulations were carried out to study the interaction between RBD and two glycopeptide antibiotics (Vancomycin and Teicoplanin). Key residues in binding pocket were highlighted and the binding free energies were calculated. Our results suggested that Vancomycin and Teicoplanin, as natural and accepted antibiotics, could block the interaction between RBD of spike protein and human ACE2 receptor, which might be developed to potential drugs against the SARS-CoV-2.
Introduction
Since the end of 2019, the epidemic disease caused by Severe Acute Respiratory Syndrome Coronavirus 2 (SARS-CoV-2) has spread in more than 100 countries, causing over 47 million infections with more than 1.2 million deaths, which severely threatens the global public health, the economic development, and the social stability (Jiang et al., 2020). SARS-CoV-2 is a kind of novel enveloped positive-stranded RNA viruses. The SARS-CoV-2 genome can encode four major structural proteins (Figure 1): the spike (S) protein, the membrane (M) protein, the envelope (E) protein and the nucleocapsid (N) protein (Dutta et al. 2020). Among them, the S protein has attracted much attention, because it is a critical determinant of viral host range and tissue tropism, as well as a major inducer of host immune response (Walls et al. 2020).
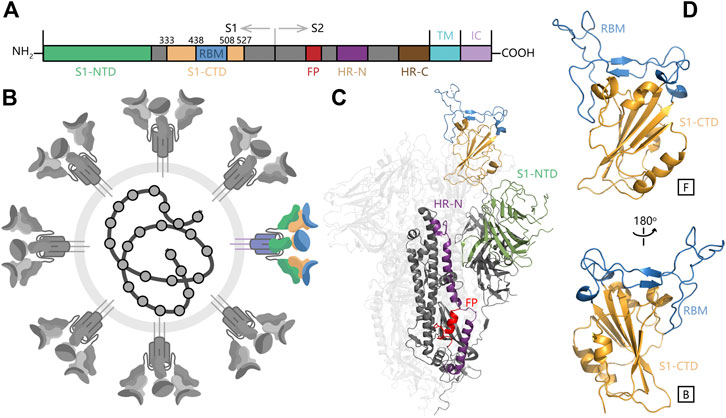
FIGURE 1. The overall structure of SARS-CoV-2 S protein and RBM domain. (A) Important monomers in SARS-CoV-2 S protein. (B) Schematic of SARS-CoV-2 and S protein in color. (C) The overall structure of SARS-CoV-2 S protein (D) The structure of S1-NTD (RBD) in SARS-CoV-2 S protein.
The SARS-CoV-2 S protein consists of three segments: a large ectodomain (ED), a single-pass transmembrane anchor (TM) and a short intracellular (IC) tail (Figure 1C). The ED is a clove-shaped trimer, comprising three receptor-binding subunits (S1) heads and a trimeric membrane-fusion subunits (S2) stalk. The C-terminal domain of S1 (S1-CTD), also named as receptor binding domain (RBD), is responsible to recognize some protein receptors like ACE2, APN, and DPP4. Furthermore, the SARS-CoV-2 RBD contains a core structure and a receptor-binding motif (RBM) as two subdomains. The core structure is formed by a group of five-stranded antiparallel β-sheets and RBM contains another two short β-sheets and long loop areas (Figure 1D). The RBM presents a bowl-like concave surface to accommodate the conformation of N-terminal α-helix in ACE2 for better binding (Li, 2016).
During the invasion process of SARS-CoV-2, S1 binds to the receptor on the host cell surface firstly and S2 fuses with the host cell membrane, allowing viral genomes to enter into the host cell (Walls et al., 2017). After that, viral genomes can encode N protein to promote RNA replication intracellularly. S protein is involved in receptor binding and membranes fusion that are indispensable steps in the coronavirus infectious cycle, so S protein has been considered to be the primary drug target for recent pandemic caused by SARS-CoV-2.
Although antibiotics become the effective therapy method of microbial infections after the introduction of sulfonamides and the discovery of penicillin in 1928, the overuse of them has resulted in the subsequent emergence of antibiotic-resistant bacteria, which dramatically reduces the therapeutic effect (Hutchings et al., 2019). Under this serious circumstance, antimicrobial peptides (AMP) has been quickly used as an alternative to antibiotics to treat bacterial and viral infections because of its natural source and high efficacy. The mechanism of AMPs against pathogens includes membrane permeabilization, membrane destabilization, inhibition of macromolecular synthesis, intracellular translocation of the peptide and inhibition of DNA/RNA/protein synthesis (Yeaman and Yount, 2003). A previous study has also shown that the peptide compounds Mo-CBP3-PepII and PepKAA can interact with the S1 and S2 domains of the S protein through molecular docking respectively (Souza et al., 2020). Peptides have been reported as the potential chemical compound to prevent SARS-CoV-2 from infecting the cell for the first time, which inspires us to study the role of glycopeptides in inhibiting the invasion of SARS-CoV-2 further.
Vancomycin (V) is a tricyclic glycopeptide antibiotic originally derived from the organism Streptococcus Orientalis. As the first member of Vancomycin family, Vancomycin was soon approved by the Food and Drug Administration in 1978 as a therapy of penicillin-resistant Staphylococcus aureus infection (Levine, 2006). After introduced into the clinical use, it was soon replaced by the β-lactam antibiotics including methicillin, ethoxylpenicillin and cloxacillin due to their higher efficiency and lower cytotoxicity. Since 2000, Vancomycin has returned as the clinical therapy of MRSA after the cytotoxic problem was solved. Nowadays, Vancomycin is still used to treat severe infections that cannot respond to other antibiotics (Pais et al., 2020).
Teicoplanin (T), another member of the Vancomycin family glycopeptide antibiotics, was first discovered in 1975 (Boger, 2001). The antibacterial spectrum and antibacterial activity of Teicoplanin were similar to those of Vancomycin (Figure 2), which is attributed to the same backbone structure. Compared with the structure of Vancomycin, the additional fat acid side chain in Teicoplanin is beneficial for penetrating cells more easily (Costa et al., 2011).
Considering the glycopeptide structure and the pre-existing therapeutic effect for pneumonia (Pais et al., 2020), Vancomycin and Teicoplanin were chosen as the potential compounds against SARS-CoV2. Their feasibility was investigated by exploring the interaction with SARS-CoV2-RBD and antiviral experiments in this study. We constructed the systems of SARS-CoV2-RBD with Vancomycin (RBD-V) and Teicoplanin (RBD-T) by molecular docking experiments. For each system, the most stable conformations were selected from 200 structures to perform 3 × 50 ns molecular dynamics (MD) simulations. Then the binding energy were calculated via MMPBSA method. The conformational characteristics were analyzed during MD simulations and key residues that play a critical role in different binding modes were highlighted. The strong and stable interaction between SARS-CoV2-RBD and Vancomycin/Teicoplanin may be beneficial to prevent receptor binding and membranes fusion process of SARS-CoV-2 infectious cycle.
Methods
System Preparation
The crystal structure of SARS-CoV2-RBD was derived from the Protein Data Bank database (PDB ID: 6M0J) (Lan et al., 2020). We used H++ website (http://biophysics.cs.vt.edu/) to compute the pKa values of ionizable residues in RBD and determine their protonation states at pH = 7.0 (Anandakrishnan et al., 2012). The chemical structures of two glycopeptides, Vancomycin and Teicoplanin, were obtained from PubChem. Their three-dimensional structures were optimized by Gaussian16 (Steen et al., 2015) at m062x (Zhao and Truhlar, 2008)-D3 (Grimme et al., 2011)/6-31G(d)(Krishnan et al., 1980) level. Then the electrostatic surface potential (ESP) charges were calculated for force field preparation. Afterward, a two-step restrained electrostatic potential (RESP) (Wang et al., 2000) model was applied to determine the charges distribution on the substrates.
Docking
In this work, the AutoDock4.2 software (Goodsell et al., 1996) was utilized to build SARS-CoV-2 RBD-V complex and RBD-T complex. According to the binding position of RBD and ACE2, the map of 126,126,126 grid points in the point interval of 0.375 Å was set and calculated. The Lamarckian Genetic Algorithm (LGA) (Fuhrmann et al., 2010) was adopted to search for stable complexes. The number of runs and maximum energy evaluations were fixed at 200 and 2,500,000. Other parameters were set as default values. Finally, the results were ranked by docking energy. The top five for each system with the low binding energy were extracted to carry out classical MD simulations later.
Classical Molecular Dynamics Simulation
MD simulations were performed on RBD-V and RBD-T complexes using AMBER16 accelerated by GPU(Gotz et al., 2012; Le Grand et al., 2013; Salomon-Ferrer et al., 2013) under ff14SB force field (Maier et al., 2015). The complexes were solvated in an octahedral box of TIP3P(Mark and Nilsson, 2001) water molecules with the thickness of the external water layer exceeding 10 Å, totally 10,190 water molecules in RBD-V system and 10,120 water molecules in RBD-T system. To achieve the charge neutralization, two chloride ions were added in both systems.
Both solvated systems were firstly subjected to 10,000 steps of minimization followed by heating and equilibration cycles. In the heating cycle, the systems were gradually heated from 0 to 300 K through 25,000 iterations. After equilibration for 50 ps in the NPT ensemble, three 50 ns molecular dynamics simulation (300 K, 1 atm) were conducted for each pose of each system with different random seeds. The Particle Mesh Ewald (PME) method was employed to account for long-range electrostatic interactions, and the SHAKE algorithm in its matrix form was used to fix bonds and angles involving hydrogen atoms (Ryckaert et al. 1977). The cutoff of Van der Waals interactions was set to 10.0 Å. Each system included top five docking results and three parallel trajectories for each mode, 15 × 50 ns trajectories in total (Table 1). Then trajectories analyses were carried out using Cpptraj (Roe and Cheatham, 2013) in Ambertools18.
Trajectories Analysis
Root mean square deviation (RMSD) was widely used to measure the variation between two structures. To judge the change of protein structures, the coordinate difference of alpha carbon, carbon atom of carbonyl groups and nitrogen atom in the system relative to the initial structure was calculated and averaged during the MD simulation. In the Eq. 1, di was the distance between the original and the present coordinates of atom i, and N was the atom number of the system to calculate the distance.
Root mean square fluctuation (RMSF) represented the average variation of single alpha atom over time. In the Eq. 2, xi means the coordinate of atom i and N means the number of frames to be calculated.
Binding Free Energy Calculation
Molecular Mechanics Poisson-Boltzmann Surface Area (MMPBSA) and Molecular Mechanics Generalized Born Surface Area (MMGBSA) were two efficient methods to calculate the binding ability between ligands and receptors (Genheden and Ryde, 2015). The total binding energy was calculated on the basis of the difference of complex free energy and sum of the free energy of receptor plus ligand. The module anti-MMPBSA.py in AMBER18 was used to create three topology files (complex, receptor and ligand) for binding energy calculation (Miller et al., 2012). Each term on the right-hand side of Eq. 3 was estimated according to Eq. 4.
In this study, one 50 ns MD simulation trajectory of each mode of each system was used to calculate the binding free energy. Each trajectory consists of 25,000 frames.
Results
Docking Results of SARS-Cov-2 RBD and Vancomycin/Teicoplanin
According to the binding mode of SARS-CoV-2 RBD and human ACE2, a docking box was set at the top of RBD. After 200 times molecular docking in each system, docking modes were ranked by binding energy in Supplementary Figure S1. The sampling of the docking structures was sufficient according to the distribution of binding energy and normal distribution of each three-dimensional coordinate vector (Figure 3). The flexible binding positions of T and V to ACE2 implied that they could efficiently block the interactions of RBD with human ACE2 receptor by occupying their interface.
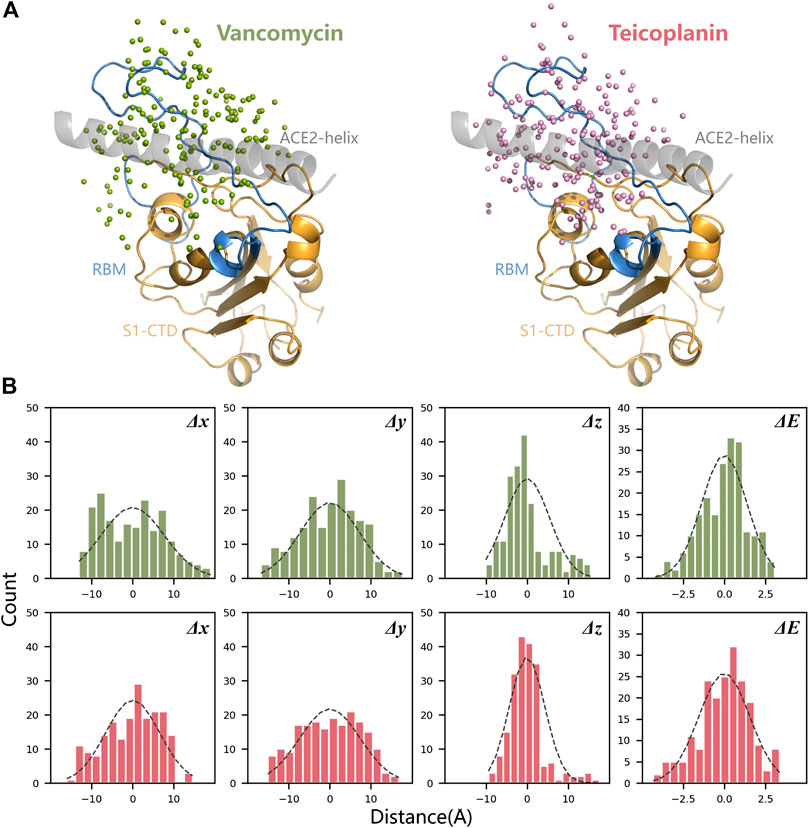
FIGURE 3. Spatial position analysis of RBD-V and RBD-T complexes. (A) Centroids distribution of V and T in all docking modes were marked with green dots and pink dots respectively. N-terminal α-helix of ACE2 was displayed in grey, the core area of RBD (S1-CTD) was displayed in orange and RBM was displayed in blue. (B) The mean and variance of each three-dimensional coordinate vector (Δx, Δy, and Δz) and docking energy score (ΔE) were calculated and represented by histograms.
Next, top five structures with the low energy were collected for each system and shown in Figure 4A. It can be seen that the receptor-binding motif (RBM) can easily accommodated V and T because of its bowl-like concave surface, except for T3, which seems escaped from the bowl. After counting up all polar interaction between ligands and RBD in those docking modes, five important residues were found and shown in Figure 4B. The hydrogen bonds formed by K417, Y449, E484, Q493 and S494 and existed in more than three docking modes. These residues primarily concentrated on RBM region and had been reported widely to play a key role in RBM binding. Also, other residues listed in Figure 4B might be involved in the recognition process between two ligands and RBD. These results suggested that our docking results are reliable.
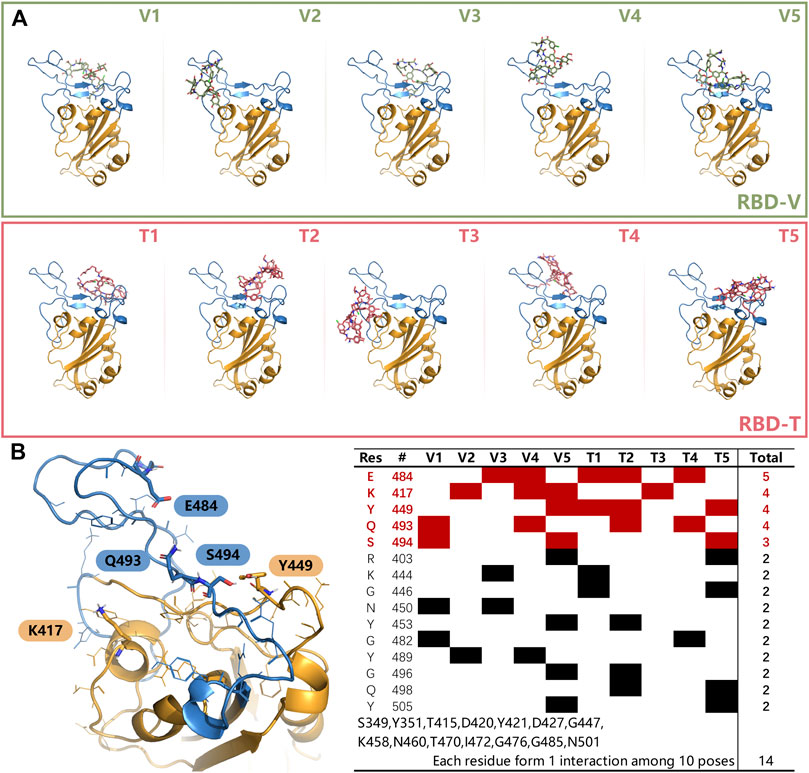
FIGURE 4. (A) The top 5 modes obtained from docking experiments of RBD-V and RBD-T system. (B) Residues that could form interactions more than twice in top five modes with V and T were listed in the table. The residues, appeared once, were merged together on the bottom. Key residues K417, Y449, E484, Q493, and S494 were shown in sticks in left. The color of RBD is as the same as Figure 3.
Structural Analysis of Receptor-Binding Motif
To study the structural characteristic of RBD-V and RBD-T complexes, the top five structures mentioned above were utilized to perform MD simulations in each system (Supplementary Figure S2). The RMSD of the RBD-V system was shown in Supplementary Figure S3. In both systems, the RMSD values with slight fluctuations (mean is 1.63 ± 0.31 Å in RBD-V system and 1.55 ± 0.25 Å in RBD-T system) suggested that the initial structures of RBD-V system and RBD-T system obtained by docking experiments were suitable and the binding states reached their respective equilibriums during 50 ns MD simulations.
The structural fluctuations of RBD in two systems were depicted in Supplementary Figure S3. Based on the RMSF analysis, residues located on the top and the bottom regions of RBD including loop1, loop2, and α1 were highlighted (Figure 5A). According to the structure of the whole S protein (Figure 1C), loop1 and α2 played important roles in the interaction of RBD with S1-NTD. The loss of S1-NTD during MD simulations might lead to the instability of these areas. Compared with the structure of RBD protein without ligands, the same RMSF variations in two systems meant the structure flexibilities of loop1 and α1-3 were not caused by the existence of ligands. And loop2 exhibited more remarkable structural flexibilities in RBD-V and RBD-T systems (Figure 5B), which suggested the existence of ligands could influence the conformational change of RBD protein. Besides loop2, V445, G446 and T500 resides at RBM also showed flexible structure movements in both RBD-V and RBD-T systems. Furthermore, two systems exhibited the similar structural movement tendency, suggesting RBD consisted of a stable core structure and the flexible RBM region responsible for binding with V and T.
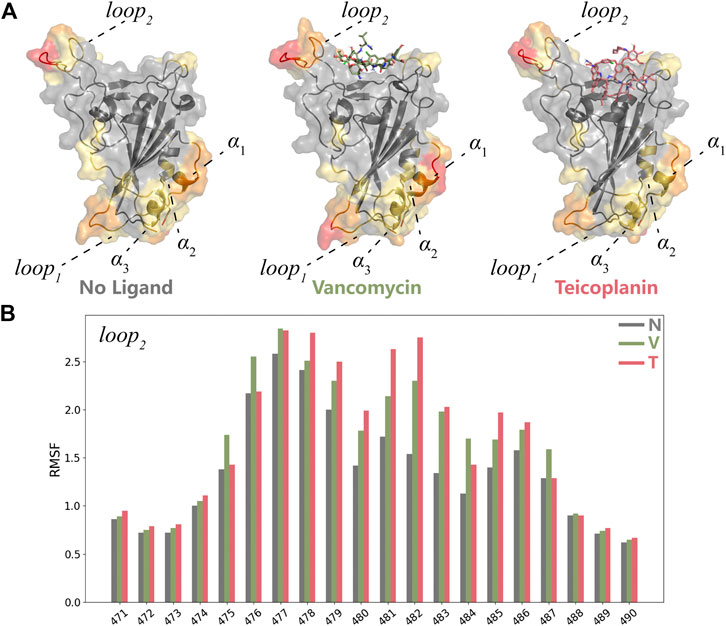
FIGURE 5. MD simulation analyses of RBD-V and RBD-T (A) The representative conformations of the system without ligand, RBD-V system and RBD-T system according to the cluster analysis. The structures were colored by RMSF value. (B) The histogram of RMSF distribution. The RMSF value of each residue in the system without ligand (N), RBD-V (V) system and RBD-T (T) system was colored by grey, green and red respectively.
In a word, V and T could bind with RBD steadily and influence the key residues in RBM involved in the recognition of ACE2. Therefore, more attention would be paid to RBM in the subsequent studies.
Linear Regression Analysis between MMPBSA Binding Free Energy and Interactions
Considering that RBM was a wide binding surface, which was much different from the traditional binding site. Also, V and T were large amphiphilic ligands. The binding modes of RBD-V and RBD-T systems were not only a single stable configuration, so conformers obtained by MD simulations every 1 ns were collected to study their structural characteristics. Hydrogen bond (HB) and hydrophobic (HP) interactions were studied. The number of the residues that interacted with V and T was counted for each system (Supplementary Table S3) and the mean was shown in Figure 6A. Compared with the chemical structure of V, T had more glycosylated modifications and a saturated nine-membered carbon chain which could increase the possibility of forming HB and HP interactions with RBD. During 50 ns MD simulations, T could form HP interaction with 3.25 residues in average, while 2.95 residues in RBD-V system. Similarly, more glycosylated modifications and polar functional group were beneficial for T to form nearly 0.5 HB interactions more than V. In a conclusion, T could bind with RBD more closely than V. What’s more, five residues in average could interact with these glycopeptide ligands for each system, indicating the existence of effective binding.
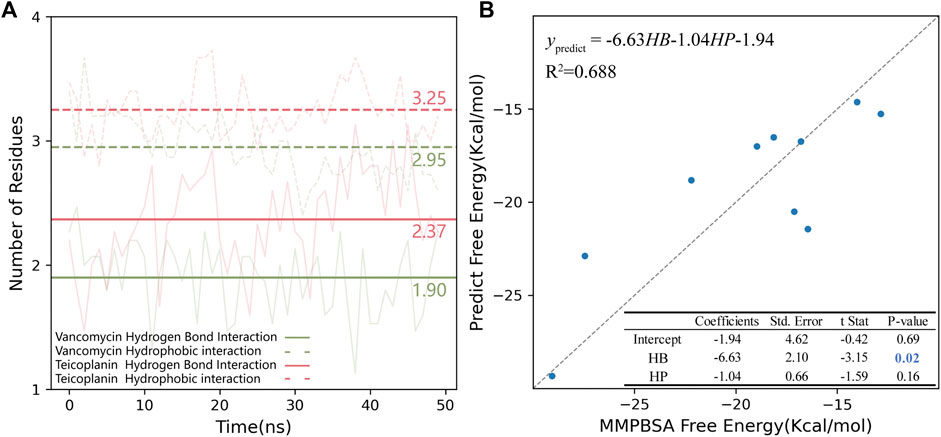
FIGURE 6. (A) Hydrogen bond and hydrophobic interaction in RBD-V and RBD-T systems. The number of residues interacting with V (green) and T (pink) in RBD region were counted every 1 ns during each 50 ns MD simulation. The average of 15 trajectories for each system was shown as the transparent line and the mean was shown as the opaque horizontal line. In the plot, hydrogen bond interaction was shown as solid lines and hydrophobic interaction was shown as dotted line. (B) The multiple linear regression model of MMPBSA binding free energy and HB/HP interactions. The standard line representing the free energy calculated by MMPBSA was equaled to the value predicted by the model was showed as a grey dotted line.
The binding energy was calculated via MMPBSA method on the top five complexes and the results were all shown in Table 2. Comparing the average binding energy of two systems, we found RBD-T system was lower than RBD-V by 3.0 kcal/mol, which validated the aforementioned conclusion that T could bind with RBD more closely than V. Then a line regression analysis was performed to investigate the correlation between MMPBSA binding free energies and HB as well as HP interactions (Figure 6B). The squared correlation coefficient (R2), also known as the coefficient of determination, was 0.688 that means the positive relationship between the binding free energy and the interactions. The coefficient between the free energy and the number of residues forming HB interaction was equaled to −6.63 with the p-value below 0.05. The binding energy contributed by HB interaction lay within the reasonable range (Emamian et al., 2019). Although the P-value of the coefficient between the free energy and the number of residues forming HP interaction was larger than 0.05, which was possibly attributed to the obscure definition of HP interaction. Besides, the tendency that the binding free energy was lower with more HP interaction was in accordance with our knowledge.
Diverse binding modes led to different binding energies. The regression analysis above showed that lower energy values were caused by better conformations with more HB and HP interactions. The average binding energies of RBD-V5 complex and RBD-T1 complex are the lowest in each system because of more HB and HP interactions, so the key residues of the two modes were explored subsequently.
Key Residues Found through Dynamic Hydrogen Bond Analysis and MMPBSA Decomposition
Basing on the results mentioned above, HB interaction showed the positive correlation with the binding free energy. Therefore, we counted up all HBs in RBD-V and RBD-T systems and the survival time was used to define the strength of these HBs to search important residues.
The HB whose survival time was longer than 1% MD simulation time was listed as dots in Figure 7. As we can see, most dots were concentrated at RBM. The HB distribution in different modes was complicated and dissimilar, but Y449, E484, S494, and Y501 interacted with glycopeptide ligands in most modes. Combined with the results of MMPBSA decomposition (Supplementary Table S3), the key residues were found out.
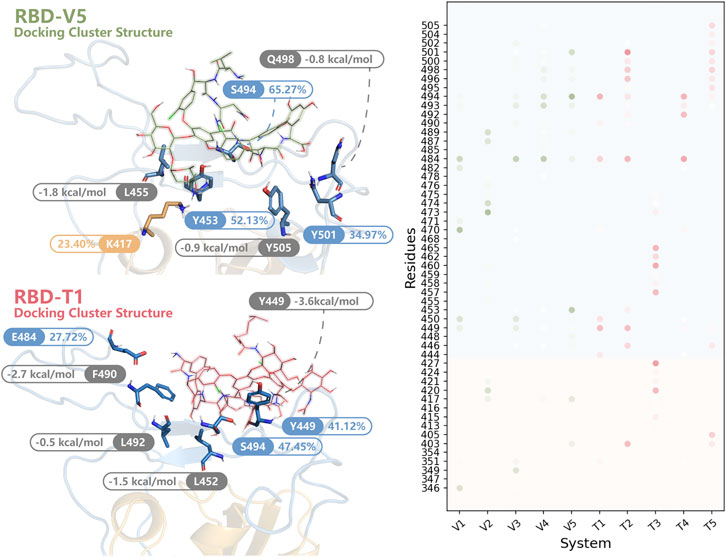
FIGURE 7. The key residues in RBD-V and RBD-T systems. In the structures of RBD-V5 and RBD-T1, residues forming HBs for more than 20% MD simulation time (blue and orange) were highlighted. Besides, residues listed in the top 10 of the MMPBSA decomposition (grey) were shown. All residues forming HBs in more than 1% modes were summarized in a dot matrix where the transparency was proportional to the survival time. The dots in RBD-V system were colored in green and the dots of RBD-T system were colored in pink.
In RBD-V5 complex, K417, Y453, S494 and Y501 stabilized V by long-time HB interaction. These HBs formed by K417, Y453, S494, and Y501 maintained 23.40, 52.13, 65.27, and 34.97% of MD simulation time respectively. Meanwhile, L455, Q498, and Y505 could be helpful to lower the binding free energy by interacting with V.
Except S494, the interaction mode in RBD-T1 was significantly different from that of RBD-V5 complex. The HBs formed by Y449, E484 and S494 could retain 41.12, 27.72, and 47.45% of MD simulation time respectively. Furthermore, L452 donated −1.5 kcal/mol, F490 donated −2.7 kcal/mol and L492 donated −0.5 kcal/mol to stabilize the binding mode between T and RBD.
The importance also showed through alanine scanning base on the wild type trajectories (Liu et al., 2018). The difference of the MMGBSA energies were shown in Supplementary Table S4. Except L492, the mutation of the rest residues showed energy difference more than 0.5 kcal/mol in at least one system, indicating their important roles in stabilizing the ligands with RBD.
Aforementioned K417, Y449, Y453, L455, Q498, Y501, and Y505 had been reported to play a crucial part in the binding with ACE2 (Hussain et al., 2020; Lan et al., 2020; Veeramachaneni et al., 2020). The remaining key residues we found including L452, E484, F490, L492, and S494 were adjacent to these important residues associated with ACE2. The binding site of V and T was similar to that of ACE2, so V and T were thought to be able to prevent the infection of SARS-CoV2 by competitive binding.
The Antiviral Potential of Vancomycin and Teicoplanin
Besides all the computational analyses mentioned above, antiviral experiments were performed to confirm our proposal. We used pseudovirus to simulate the SARS-CoV-2 under 50 μM Vancomycin or Teicoplanin. The antiviral potential of V and T were tested through an antiviral sever. The results revealed that V and T respectively with 83 and 87% being antiviral against SARS-CoV-2. (Supplementary Table S2).
Conclusion
In conclusion, two glycopeptides, Vancomycin and Teicoplanin, were investigated as potential antiviral molecules by analyzing the interactions with SARS-CoV-2 RBD of spike protein. Firstly, 200 times docking experiments were used to choose the proper binding modes and K417, Y449, E484, Q493, and S494 were found out as key residues to stabilize the ligands with RBD. After classical MD simulations of top five structures with low energy, the RMSD analysis showed the binding states reached their equilibriums and the RMSF analysis showed both ligands could cause structural change of loop2 by binding with RBD. Afterwards, the number of hydrogen bonds and hydrophobic interactions was counted as independent variable and the binding free energy was calculated as dependent variable. The linear regression analysis between binding free energy and interactions suggested that hydrogen bonds played important roles in maintaining the stability of the conformation. Also compared two systems, More glycosylated modifications and the fat acid side chain in T increases the possibility of forming more hydrogen bonds and hydrophobic interactions with RBD. Furthermore, dynamic hydrogen bond analysis and MMPBSA decomposition helped us to find out more important residues. With the help of alanine scanning analysis of those important residues, we more convinced that they played an important role of enhancing the combination of two ligands and RBD. Part of those key residues were also found to bind with ACE2 reported in other studies (Hussain et al., 2020; Lan et al., 2020; Veeramachaneni et al., 2020), such as K417, Y449, Y453, L455, Q498, Y501 and Y505. Although the remaining key residues such as L452, E484, F490, L492, and S494 did not directly forming interactions, they were adjacent to these important residues associated with ACE2. Based on those results mentioned above, we implied that Vancomycin and Teicoplanin could be the potential competitive inhibitor to block the binding of SARS-CoV-2 RBD and ACE2, and further prevent the SARS-CoV-2 infectious cycle.
Data Availability Statement
The original contributions presented in the study are included in the article/Supplementary Material, further inquiries can be directed to the corresponding author.
Author Contributions
Conceptualization, TS, Y-LZ, and MG; formal analysis, W-TT; experiment, Y-LL and MG; funding acquisition, TS, Y-LZ, and MG; investigation, W-TT; methodology, TS, Y-LZ, and MG; writing-original draft, W-TT, QY, and TS; writing-review and editing, W-TT and TS.
Funding
This research was supported by the National Key R&D Program of China (No. 2019YFA0905400), the National Natural Science Foundation of China (Nos. 32070041, 31770070, and 31970041) and the Natural Science Foundation of Shanghai (No. 19ZR1427300 and 20S11900900).
Conflict of Interest
The authors declare that the research was conducted in the absence of any commercial or financial relationships that could be construed as a potential conflict of interest.
Acknowledgments
The authors thank SJTU-ZH2018ZDA26 for financial supports and SJTU-HPC computing facility award for computational hours.
Supplementary Material
The Supplementary Material for this article can be found online at: https://www.frontiersin.org/articles/10.3389/fchem.2020.639918/full#supplementary-material.
References
Anandakrishnan, R., Aguilar, B., and Onufriev, A. V. (2012). H++ 3.0: automating pK prediction and the preparation of biomolecular structures for atomistic molecular modeling and simulations. Nucleic Acids Res. 40, W537–W541. doi:10.1093/nar/gks375
Boger, D. L. (2001). Vancomycin, teicoplanin, and ramoplanin: synthetic and mechanistic studies. Med. Res. Rev. 21, 356–381. doi:10.1002/med.1014
Costa, F., Carvalho, I. F., Montelaro, R. C., Gomes, P., and Martins, M. C. (2011). Covalent immobilization of antimicrobial peptides (AMPs) onto biomaterial surfaces. Acta Biomater. 7, 1431–1440. doi:10.1016/j.actbio.2010.11.005
Dutta, N. K., Mazumdar, K., and Gordy, J. T. (2020). The nucleocapsid protein of SARS-CoV-2: a target for vaccine development. J. Virol. 94, e00647-20. doi:10.1128/JVI.00647-20
Emamian, S., Lu, T., Kruse, H., and Emamian, H. (2019). Exploring nature and predicting strength of hydrogen bonds: a correlation analysis between atoms-in-molecules descriptors, binding energies, and energy components of symmetry-adapted perturbation theory. J. Comput. Chem. 40 (40), 2868–2881. doi:10.1002/jcc.26068
Fuhrmann, J., Rurainski, A., Lenhof, H. P., and Neumann, D. (2010). A new Lamarckian genetic algorithm for flexible ligand-receptor docking. J. Comput. Chem. 31, 1911–1918. doi:10.1002/jcc.21478
Genheden, S., and Ryde, U. (2015). The MM/PBSA and MM/GBSA methods to estimate ligand-binding affinities. Expet. Opin. Drug Discov. 10, 449–461. doi:10.1517/17460441.2015.1032936
Goodsell, D. S., Morris, G. M., and Olson, A. J. (1996). Automated docking of flexible ligands: applications of AutoDock. J. Mol. Recogn. 9, 1–5. doi:10.1002/(SICI)1099-1352(199601)9:1<1::AID-JMR241>3.0.CO;2-6
Götz, A. W., Williamson, M. J., Xu, D., Poole, D., Le Grand, S., and Walker, R. C. (2012). Routine microsecond molecular dynamics simulations with AMBER on GPUs. 1. Generalized Born. J. Chem. Theor. Comput. 8, 1542–1555. doi:10.1021/ct200909j
Grimme, S., Ehrlich, S., and Goerigk, L. (2011). Effect of the damping function in dispersion corrected density functional theory. J. Comput. Chem. 32, 1456–1465. doi:10.1002/jcc.21759
Hussain, M., Jabeen, N., Raza, F., Shabbir, S., Baig, A. A., Amanullah, A., et al. (2020). Structural variations in human ACE2 may influence its binding with SARS-CoV-2 spike protein. J. Med. Virol. 92, 1580–1586. doi:10.1002/jmv.25832
Hutchings, M. I., Truman, A. W., and Wilkinson, B. (2019). Antibiotics: past, present and future. Curr. Opin. Microbiol. 51, 72–80. doi:10.1016/j.mib.2019.10.008
Jiang, S., Hillyer, C., and Du, L. (2020). Neutralizing antibodies against SARS-CoV-2 and other human coronaviruses: (trends in immunology 41, 355–359; 2020). Trends Immunol. 41, 545–359. doi:10.1016/j.it.2020.04.008
Krishnan, R., Binkley, J. S., Seeger, R., and Pople, J. A. (1980). Self‐consistent molecular orbital methods. XX. A basis set for correlated wave functions. J. Chem. Phys. 72, 650–654. doi:10.1063/1.438955
Lan, J., Ge, J., Yu, J., Shan, S., Zhou, H., Fan, S., et al. (2020). Structure of the SARS-CoV-2 spike receptor-binding domain bound to the ACE2 receptor. Nature 581, 215–220. doi:10.1038/s41586-020-2180-5
Le Grand, S., Götz, A. W., and Walker, R. C. (2013). SPFP: speed without compromise-A mixed precision model for GPU accelerated molecular dynamics simulations. Comput. Phys. Commun. 184, 374–380. doi:10.1016/j.cpc.2012.09.022
Levine, D. P. (2006). Vancomycin: a history. Clin. Infect Dis. (42 Suppl. 1), S5–S12. doi:10.1086/491709
Li, F. (2016). Structure, function, and evolution of coronavirus spike proteins. Annu. Rev. Virol. 3 (3), 237–261. doi:10.1146/annurev-virology-110615-042301
Liu, X., Peng, L., Zhou, Y., Zhang, Y., and Zhang, J. Z. H. (2018). Computational alanine scanning with interaction entropy for protein-ligand binding free energies. J. Chem. Theory Comput. Mar. 13 (14), 1772–1780. doi:10.1021/acs.jctc.7b01295
Maier, J. A., Martinez, C., Kasavajhala, K., Wickstrom, L., Hauser, K. E., and Simmerling, C. (2015). ff14SB: improving the accuracy of protein side chain and backbone parameters from ff99SB. J. Chem. Theor. Comput. 11 (11), 3696–3713. doi:10.1021/acs.jctc.5b00255
Mark, P., and Nilsson, L. (2001). Structure and dynamics of the TIP3P, SPC, and SPC/E water models at 298 K. J. Phys. Chem. B. 105, 24a. doi:10.1021/jp003020w
Miller, B. R., McGee, T. D., Swails, J. M., Homeyer, N., Gohlke, H., and Roitberg, A. E. (2012). MMPBSA.py: an efficient Program for end-state free energy calculations. J. Chem. Theor. Comput. 8 (8), 3314–3321. doi:10.1021/ct300418h
Pais, G. M., Liu, J., Zepcan, S., Avedissian, S. N., Rhodes, N. J., Downes, K. J., et al. (2020). Vancomycin-Induced kidney injury: animal models of toxicodynamics, mechanisms of injury, human translation, and potential strategies for prevention. Pharmacotherapy 40, 438–454. doi:10.1002/phar.2388
Roe, D. R., and Cheatham, T. E. (2013). PTRAJ and CPPTRAJ: software for processing and analysis of molecular dynamics trajectory Data. J. Chem. Theor. Comput. 9 (9), 3084–3095. doi:10.1021/ct400341p
Ryckaert, J.-P., Ciccotti, G., and Berendsen, H. J. C. (1977). Numerical integration of the cartesian equations of motion of a system with constraints: molecular dynamics of n-alkanes. J. Comput. Phys. 23, 327–341. doi:10.1016/0021-9991(77)90098-5
Salomon-Ferrer, R., Götz, A. W., Poole, D., Le Grand, S., and Walker, R. C. (2013). Routine microsecond molecular dynamics simulations with AMBER on GPUs. 2. Explicit solvent particle Mesh Ewald. J. Chem. Theor. Comput. 9 (9), 3878–3888. doi:10.1021/ct400314y
Souza, P. F. N., Lopes, F. E. S., Amaral, J. L., Freitas, C. D. T., and Oliveira, J. T. A. (2020). A molecular docking study revealed that synthetic peptides induced conformational changes in the structure of SARS-CoV-2 spike glycoprotein, disrupting the interaction with human ACE2 receptor. Int. J. Biol. Macromol. 164, 66–76. doi:10.1016/j.ijbiomac.2020.07.174
Steen, G. W., Fuchs, E. C., Wexler, A. D., and Offerhaus, H. L. (2015). Identification and quantification of 16 inorganic ions in water by Gaussian curve fitting of near-infrared difference absorbance spectra. Appl. Optic. 54, 5937–5942. doi:10.1364/ao.54.005937
Veeramachaneni, G. K., Thunuguntla, V. B. S. C., Bobbillapati, J., and Bondili, J. S. (2020). Structural and simulation analysis of hotspot residues interactions of SARS-CoV 2 with human ACE2 receptor. J. Biomol. Struct. Dyn. 4, 1–11. doi:10.1080/07391102.2020.1773318
Walls, A. C., Park, Y. J., Tortorici, M. A., Wall, A., McGuire, A. T., and Veesler, D. (2020). Structure, function, and antigenicity of the SARS-CoV-2 spike glycoprotein. Cell 181, 281–e6. doi:10.1016/j.cell.2020.02.058
Walls, A. C., Tortorici, M. A., Snijder, J., Xiong, X., Bosch, B. J., Rey, F. A., et al. (2017). Tectonic conformational changes of a coronavirus spike glycoprotein promote membrane fusion. Proc. Natl. Acad. Sci. U.S.A. 114, 11157–11162. doi:10.1073/pnas.1708727114
Wang, J., Cieplak, P., and Kollman, P. A. (2000). How well does a restrained electrostatic potential (RESP) model perform in calculating conformational energies of organic and biological molecules?. J. Comput. Chem. 21, 1049–1074. doi:10.1002/1096-987x(200009)21:12<1049::aid-jcc3>3.0.co;2-f
Yeaman, M. R., and Yount, N. Y. (2003). Mechanisms of antimicrobial peptide action and resistance. Pharmacol. Rev. 55, 27–55. doi:10.1124/pr.55.1.2
Zhao, Y., and Truhlar, D. G. (2008). The M06 suite of density functionals for main group thermochemistry, thermochemical kinetics, noncovalent interactions, excited states, and transition elements: two new functionals and systematic testing of four M06-class functionals and 12 other functionals. Theor. Chem. Account. 120, 215–241. doi:10.1007/s00214-007-0310-x
Keywords: SARS-CoV-2, RBD, ACE2, molecular docking, MD simulation
Citation: Tao W-T, Yu Q, Li Y-L, Ge M, Zhao Y-L and Shi T (2021) Exploring the Interaction between Vancomycin/Teicoplanin and Receptor Binding Domain (RBD) of SARS-CoV-2. Front. Chem. 8:639918. doi: 10.3389/fchem.2020.639918
Received: 10 December 2020; Accepted: 31 December 2020;
Published: 19 January 2021.
Edited by:
Fei Ye, Zhejiang Sci-Tech University, ChinaReviewed by:
Fei Xia, East China Normal University, ChinaMin Huang, Northwestern Polytechnical University, China
Copyright © 2021 Tao, Yu, Li, Ge, Zhao and Shi. This is an open-access article distributed under the terms of the Creative Commons Attribution License (CC BY). The use, distribution or reproduction in other forums is permitted, provided the original author(s) and the copyright owner(s) are credited and that the original publication in this journal is cited, in accordance with accepted academic practice. No use, distribution or reproduction is permitted which does not comply with these terms.
*Correspondence: Ting Shi, tshi@sjtu.edu.cn