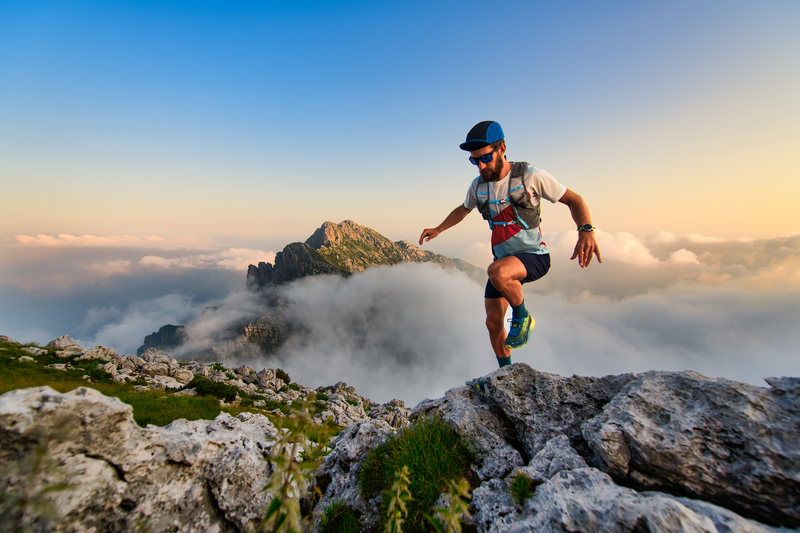
94% of researchers rate our articles as excellent or good
Learn more about the work of our research integrity team to safeguard the quality of each article we publish.
Find out more
ORIGINAL RESEARCH article
Front. Chem. , 08 February 2021
Sec. Medicinal and Pharmaceutical Chemistry
Volume 8 - 2020 | https://doi.org/10.3389/fchem.2020.595097
This article is part of the Research Topic Molecular Studies of COVID-19 Chemistry View all 19 articles
The pandemic that started in Wuhan (China) in 2019 has caused a large number of deaths, and infected people around the world due to the absence of effective therapy against coronavirus 2 of the severe acute respiratory syndrome (SARS-CoV-2). Viral maturation requires the activity of the main viral protease (Mpro), so its inhibition stops the progress of the disease. To evaluate possible inhibitors, a computational model of the SARS-CoV-2 enzyme Mpro was constructed in complex with 26 synthetic ligands derived from coumarins and quinolines. Analysis of simulations of molecular dynamics and molecular docking of the models show a high affinity for the enzyme (∆Ebinding between −5.1 and 7.1 kcal mol−1). The six compounds with the highest affinity show Kd between 6.26 × 10–6 and 17.2 × 10–6, with binding affinity between −20 and −25 kcal mol−1, with ligand efficiency less than 0.3 associated with possible inhibitory candidates. In addition to the high affinity of these compounds for SARS-CoV-2 Mpro, low toxicity is expected considering the Lipinski, Veber and Pfizer rules. Therefore, this novel study provides candidate inhibitors that would allow experimental studies which can lead to the development of new treatments for SARS-CoV-2.
In recent years, different viruses have emerged in around of the world. These diseases are a generation of respiratory diseases in infected patients, also due to the rapid dissemination of the diseases. These kinds of viruses include the severe acute respiratory syndrome coronavirus (SARS-CoV), Middle East respiratory syndrome coronavirus (MERS-CoV), avian influenza A/H7N9 and H5N1 viruses, and Nipah virus (Yuen et al., 1998; Peiris et al., 2003; MacNeil and Rollin, 2012; Marsh and Wang, 2012; To et al., 2012; To et al., 2013; Zaki et al., 2012; Chan et al., 2015). The capacity of these viruses to evolve and infect humans has been associated with the close interaction occurring between human populations and different animal species in markets of densely populated areas (Chan et al., 2015). In December 2019, cases of atypical pneumonia began to be observed in the city of Wuhan (China) (Lu et al., 2020; Zhu et al., 2020). By January 2020, the etiological agent was classified as a new member the of family Coronaviridae and genus β-coronavirus (2019-nCoV) that differ from SARS-CoV and MERS-CoV. The genome of 2019-nCoV shares an 82% sequence identify to SARS-CoV (Elfiky, 2020; Hui et al., 2020; Rothan and Byrareddy, 2020; World Health Organization, 2020). As a matter of fact, its genome has high similarity with the genome of a bat coronavirus (96.2% identity), which has allowed the virus to be associated with a zoonotic origin (Lu et al., 2020; Wu et al., 2020; Zhou et al., 2020). According to the International Committee Virus Taxonomy the new β-coronavirus was called severe acute respiratory syndrome coronavirus 2 (SARS-CoV-2) and on February 11 the set of symptoms associated with this new virus was designated as COVID-19 by the World Health Organization (WHO) (Zhang et al., 2020b). The evolution of infections at a global level increased as the number of cases and deaths, with the most affected countries being the USA, India, Brazil, Russia, France and United Kingdom (COVID-19 Map - Johns Hopkins Coronavirus Resource Center, 2020), which together have presented more than 50% of the global cases in more than 180 countries, declaring it a pandemic on March 11 (WHO Director-General’s opening remarks at the media briefing on COVID-19–20 March, 2020).
Consequently, developed and developing countries are working on the generation of vaccines or antivirals find a solution in the short or medium term. In this context, many governments, medical institutions, and scientists have tried various treatments used for other diseases with promising but so far inconclusive results. These treatments include Chloroquine, Hydroxychloroquine, Camostat, Nafamostat, Umifenovir, Tenofovir, Ramdesidir, Sofosbuvir, Galidesivir, Lopinavir, and indinavir, which are used to treat other diseases but have shown a degree of inhibitory activity of SARS-CoV-2 (Chu et al., 2004; Sissoko et al., 2016; Yamamoto et al., 2016; Mulangu et al., 2019; Cortegiani et al., 2020; Deng et al., 2020; Elfiky, 2020; Grein et al., 2020; Hirota et al., 2020; Hoffmann et al., 2020). Each of these compounds has different modes of action and targets such as antiviral drug (RNA-dependent RNA polymerase (RdRp), viral proteases and membrane fusion clathrin-mediated endocytosis (CME), antimalarial drug (elevation of the endosomal pH and ACE2) and serine protease inhibitor (TMPRSS2) (Chu et al., 2004; Sissoko et al., 2016; Yamamoto et al., 2016; Mulangu et al., 2019; Cortegiani et al., 2020; Deng et al., 2020; Grein et al., 2020; Hirota et al., 2020; Hoffmann et al., 2020; McKee et al., 2020).
Like all other coronaviruses, SARS-CoV-2 is composed by single-stranded RNA as their genetic material with an approximate length of 29,891 nucleotides and a 5′-cap structure and 3′-poly-A tail, encoding 9,860 amino acids (Chan et al., 2020). This RNA encodes both the structural and non-structural proteins of the virus. Among the structural proteins, there is the Spike (S) (present in all coronaviruses), Nucleocapsid (N), Matrix (M), and Envelope (E) (Chan et al., 2020; Walls et al., 2020; Wrapp et al., 2020). Proteases and RNA-dependent RNA polymerase constitute the non-structural proteins of the virus. The genome contains at least six open reading frames (ORFs), the first of these ORF occupies about 60% of the length of the genome and translates two polyproteins known as pp1a and pp1ab, which are processed by the main protease (Mpro, also called 3CLpro) and papain-like proteases (PLPs) (Jin et al., 2020a; Zhang et al., 2020b; Chen et al., 2020). Consequently, inhibiting Mpro activity blocks virus replication and thus affects the life cycle of SARS-CoV-2. Compounds derived from coumarins and quinoline have been tested against various viruses (McKee et al., 2020; Mishra et al., 2020). Quinolines have recently been used in experimental treatments for SARS-CoV-2 infected persons in several countries (Arshad et al., 2020; Lopez et al., 2020; Mori et al., 2020) and it has been proposed that coumarins inhibit the replication of several viruses including influenza (Pavurala et al., 2018), HIV (Jesumoroti et al., 2019), Dengue (Coulerie et al., 2013), Chikungunya (Hwu et al., 2019), hepatitis (Hwu et al., 2008) and filoviruses (EBOLA, Marburgvirus (MARV) and Cuevavirus) (Liu et al., 2019).
In this study, we evaluated twenty-sixth molecules derived from coumarins and quinolines as promising SARS-CoV-2 Mpro inhibitors, and so, by using computational biochemistry protocols we tried to find the most appropriate molecules that can act as potential anti-SARS-CoV-2 activity drugs. Six of the compounds evaluated are highlighted, which are CTR9, 7HC6, CTR6, 7HC5, 7HC3 and 8HQ6. We performed molecular docking (rigid), efficiency calculations of ligands, pharmacological and toxicological property predictions (ADMET), and molecular dynamics simulations (MD) simulations, together with MM-GBSA binding free energy predictions to identify the binding characteristics for identifying the inhibitors of SARS-CoV-2 Mpro.
In this work, we use twenty-sixth ligands selected for their possible capability to inhibit SARS-CoV-2 protease Mpro. The coumarins and quinolines derivatives were extracted from two compound series that have been synthesized by the laboratories of applied chemistry of the Universidad de Ibagué, in Ibague-Colombia. The work was based on strategies that the authors have reported in the literature (García-Beltrán et al., 2013; Mena et al., 2015; Aguirre et al., 2017; Garćia-Beltran et al., 2017). 13b is our reference molecule and was obtained from the Protein Data Bank (PDB) (Zhang et al., 2020b) (PDB id: 6Y2F). These molecules were designed in silico and evaluated using docking methodologies and physicochemical and pharmacokinetic descriptors, and to predict ADME parameters. Their chemical structures are shown in Figure 1. Their molecular conformations were optimized using PM6-D3H4 semi-empirical method (Stewart, 2007; Řezáč and Hobza, 2012) as implemented in MOPAC2016 (Stewart, 2016) software. The optimized molecules were used for molecular docking simulations in order to study the interactions established by these compounds in the SARS-CoV-2 Mpro pocket.
We made molecular docking analyses to examine the potential binding modes of ligands to the main protease Mpro of SARS-CoV-2, as potential inhibitors. Then, based on structural information obtained from the crystal structures of Mpro in complex with other ligands we established the binding site of the proposed inhibitors for SARS-CoV-2 Mpro. In addition to delimit the binding region of possible inhibitors to Mpro (Dai et al., 2020; Jin et al., 2020a; ul Qamar, 2020; Xu et al., 2020; Zhang et al., 2020a, Zhang et al., 2020b). We established the key residues for catalysis according to experimental and theoretical data. Finally, we used AutoDock (v 4.2.1) and AutoDock Vina (Trott and Olson, 2010) for all dockings in this study. The initial 3D inhibitors structures were drawn using Discovery Studio (Dassault Systèmes BIOVIA, 2017) 3.1 (Accelrys, CA) which were optimized (considering the RMS gradient of 0.001 kcal/mol) using the PM6-D3H4 semi-empirical method (Stewart, 2007; Řezáč and Hobza, 2012) implemented in the MOPAC2016 (Stewart, 2016) software. PM6-D3H4 introduces dispersion and hydrogen-bonded corrections to the PM6 method. The ligand files were prepared using the AutoDockTools package (Sanner, 1999) provided by AutoDock through accepting all rotatable bonds; moreover, the atomic charges are computed toward the PM6-D3H4 procedure, and non-polar hydrogen atoms are merged. The semi-empirical method has shown to increase significantly docking accuracy and cluster population of the most accurate docking (Bikadi and Hazai, 2009; Fanfrlík et al., 2010; Hou et al., 2013). The crystal structure of SARS-CoV-2 Mpro (PDB Code: 6YB7, https://www.rcsb.org/structure/6YB7), was downloaded from the PDB (Berman et al., 2000). The resolution of the retrieved structure was 1.25 Å. The SARS-CoV-2 Mpro was treated with the Schrödinger's (Schrödinger, 2020) Protein Preparation Wizard (Madhavi Sastry et al., 2013); polar hydrogen atoms were added, non-polar hydrogen atoms were merged, and charges were assigned. Docking was treated as rigid and carried out using the empirical free energy function and the Lamarckian Genetic Algorithm provided by AutoDock Vina (Morris et al., 1998). The docking grid dimensions were 30.75 × 30.75 × 30.75 Å, making the binding pocket of SARS-CoV-2 Mpro the center of mass between amino-acid residues (Cys145 and His41) of the catalytic site. All other parameters were set as defined by default through AutoDock Vina. Dockings were repeated 50 times with space search exhaustiveness set to 100. The best interaction binding energy (kcal·mol−1) was selected for evaluation and analyzed according to the potential intermolecular interactions (protein/ligand), such as hydrogen bonding, hydrophobic interactions and the cation–π, π–π stacking. Docking results 3D representations were used. VMD molecular graphics system (Humphrey et al., 1996).
Ligand efficiency (LE) calculations were performed using one parameter
where
where
The purpose of calculating ADMET profiles is to supply, with reasonable accuracy, a preliminary prediction of the in vivo behavior of a compound to assess its potential to become a drug (Yu and Adedoyin, 2003). The molecules used in this study were submitted to the calculation of their absorption, distribution, metabolism, excretion and toxicological properties (ADMET). Also, the physicochemical properties such as molecular hydrogen bond acceptor (HBA), hydrogen bond donor (HBD), molecular weight (MW), topological polar surface area (TPSA), rotatable bond count (RB) and octanol/water partition coefficient (LogP) were calculated using SwissADME webserver (Daina et al., 2017). Compound toxicological properties were analyzed taking into account the Lipinski, Veber and Pfizer toxicity empirical rules, see Table 1. (MacLeod-Carey et al., 2020).
MDs calculations were performed for the lowest six binding energy docking and the compound 13b, which is our reference ligand. These calculations were also obtained from Protein Data Bank (PDB id: 6Y2E). The ligands were bound to SARS-CoV-2 Mpro protein (PDB ID:6YB7) in aqueous solutions with an explicit solvent TIP3P water model (Neria et al., 1996) (≈16.000 water molecules). Protonation states of ionizable residues corresponding to pH 7.0 were determined by H++ web interface for computes pK values of ionizable groups in macromolecules and adds missing hydrogen atoms according to the specified pH of the environment (Anandakrishnan et al., 2012). Besides, NaCl ions were modeled to neutralize the systems and maintain an ionic concentration of 0.15 mol/L. The compounds were parameterized by GAFF Force Field for organic molecules. (Wang et al., 2004; ÖzpInar et al., 2010), using the Antechamber module in AmberTools18 with AM1-BCC charges, (Jakalian et al., 2000). The protein structures were modeled with the force field ff14SB. (Salomon-Ferrer et al., 2013). The simulations were carried out using a standard MD protocol: (I) Minimization and structural relaxation of water molecules with 2000 steps of minimization (downward step) and MD simulation with an NPT (300 K) assembly by 1,000 ps using harmonic restrictions of 10 kcal molÅ−2 for protein and ligand; (II) minimization of the complete structure considering 2000 downstream minimization steps and 6,500 steps of conjugate gradient minimization; (III) the minimized systems were progressively heated to 300 K, with harmonic restrictions of 10 kcal mol Å−2 in the carbon skeleton and ligand during 0.5 ns; (IV) the system was then balanced by 0.5 ns maintaining the restrictions and then by 5 ns without restrictions to 300 K in a canonical assembly (NVT); and (5) finally, a production dynamic was carried out with an isothermal isobaric assembly (NPT) without restrictions for 200 ns at 310 K and 1 atm with a temporary passage of 2 fs. In the MD simulation, the temperature was controlled by the Langevin dynamics with a collision frequency of 1 ps−1 (NVT) and the pressure with the Berendsen barostat (NPT). Besides, the Particle Mesh Ewald (PME) method with a cut-off value of 10 Å was used to treat nonbonding and long-range electrostatic interactions. All MD simulation calculations were performed using the AMBER-GPU Implementations18 (Mermelstein et al., 2018). Molecular visualization of the systems and MD trajectory analysis was carried out with the VMD software package (Humphrey et al., 1996).
The molecular MM/GBSA method was employed to estimate the binding free energy of the protease-ligand complexes. For calculations from a total of 200 ns of MD, the last 50 ns were extracted for analysis, and the explicit water molecules and ions were removed. The MM/GBSA analysis was performed on three subsets of each system: the protein alone, the ligand alone, and the complex (protein-ligand). For each of these subsets, the total free energy (
where
The principal cluster of main component analysis of trajectory were analyzed with the non-covalent interaction index (NCI) (Johnson et al., 2010; Contreras-García et al., 2011) using NCIPLOT program (Contreras-García et al., 2011) to identify and map non-covalent interactions, such hydrogen bonds, steric repulsion, and van der Waals interactions, using the promolecular densities (
These interactions are local and manifest in real space as low-gradient isosurfaces with low densities which are interpreted and colored according to the corresponding values of sign(λ2)ρ. The surfaces are colored on a blue-green-red scale according to the strength and type of interaction. Blue indicates strong attractive interactions, green indicates weak van der Waals interactions, and red indicates a strong non bonded overlap.
The docking results, which were conducted to estimate the possible binding sites of potential inhibitors on SARS-CoV-2 Mpro. The genetic material of SARS-CoV-2 expresses multiple proteins (more than 20 proteins), among these proteins the main protease (Mpro) is identified, a molecule similar to 3 chymotrypsin (3CLpro) that shows a similarity of 96.1% with the 3CLpro of SARS-CoV. 3CLpro plays a very important role in replication and transcription processes of the virus genome (Hui et al., 2020). Therefore, 3CLpro is a strategic drug target in the inhibition of the SARS-CoV cycle. The protease is active as a homodimer, structured by the dimerization of two protomers designated as monomer A and monomer B, and the catalytic dyad in each protomer is defined by Cys145 and its residues (Zhang et al., 2020b). This has led to the development of multiple studies with experimental and computational approaches in search of possible inhibitors that can effectively block the activity of this protease (Balaramnavar et al., 2020; Dai et al., 2020; Gao et al., 2020; Jin et al., 2020a; Jin et al., 2020b; Ngo et al., 2020; Zhang et al., 2020b). Work with the 3C-like proteinase from SARS coronavirus revealed that the Cys145 residue is key at the active site of 3CLpro (Huang et al., 2004), this advance allowed the mentioned residue to be an attractive target for covalent ligands to bind acting as inhibitors of 3CLpro. This amino acidic residue is also a popular target for covalent inhibitors because of its intrinsic reactivity at physiological pH (Cuesta et al., 2020). Tung Ngo et al. report in recent studies that additionally Glu166 residue has a prominent and important role in binding ligands to SARS-CoV-2 Mpro (Ngo et al., 2020).
Twenty-seven inhibitors, including the reference ligand 13b were evaluated in silico anti-SARS-CoV-2 activity. The results of this study of molecular docking calculations indicate the strong interactions of molecules derived from coumarins and quinolones targets the Cys-His catalytic dyad (Cys145 and His41) in the binding pocket of SARS-CoV-2 Mpro. The results of the binding of these molecules are presented in Table 2. Meanwhile, the binding to various amino acid residues due to their presence in the conserved region of the active site in all compounds is seen and presents a very important role in enzymatic catalysis.
TABLE 2. Molecular docking study between selected ligands and SARS-CoV-2 Mpro. Intermolecular docking values, presented with their interaction energy (∆Ebinding), H-bond residues, interacting residues are shown and Ligand efficiency calculation for SARS-CoV-2 Mpro complexes.
Docking results with SARS-CoV-2 Mpro, indicated that all ligands present binding energies between −5.3 and −7.2 kcal mol−1 (Table 2), with a difference of 0.1 kcal mol−1 between 13b and CTR6, which means that the level of stability is very similar between these two protease-complex ligands. AutoDock Vina, presents that the ligand 13b attached to the co-crystal, and the re-coupled ligand 13b presents an RMSD value of 3.1 Å, suggesting a partially acceptable value of the coupling method. Furthermore, it shows that the compound CTR6 gives the lowest energy (−7.1 kcal-mol−1) in complex with the protease, which is the best score when compared to other docked compounds used in this study. CTR6 gives better score than 7HC6 (−6.7 kcal mol−1), 7HC5 (−6.6 kcal mol−1), CTR9 (−6.6 kcal mol−1), 7HC3 (−6.6 kcal mol−1), 8HQ6 (−6.5 kcal mol−1) and the other compounds. The coumarins and quinolines derivatives are located inside the protein pocket, by means of electrostatic and hydrophobic interactions with the residues Arg188, Asn142, Asp187, Cys145, Gln189, Glu166, Gly143, His164, His163, His41, Leu167, Leu27, Met165, Met49, Phe140, Pro168, Ser144, Thr25 and Thr26. In relation to 13b, it shows two hydrogen bonding interactions with Leu167 and Glu166 residues. In the case of CTR6, 7HC6 and CTR9 in complex with SARS-CoV-2 Mpro (Figure 2 and Table 2) show possible hydrogen-bonding interactions with the residues Ser46, Met49, Asn142, Met165, Glu166, Gln189 and Gln192 (H-donor). This allows us to conclude that the hydrogen bonds and hydrophobic forces, are the majority interactions that dominate these complexes. Interactions between the rest of the compounds and SARS-CoV-2 Mpro are reported in Table 2.
FIGURE 2. The best seven docking poses of different ligands in SARS-CoV-2 Mpro binding pocket. Snapshots of (A) CTR6, (B) 7HC6, (C) 7HC5, (D) CTR9, (E) 7HC3, (F) 8HQ6 and (G) 13b during docking simulations. The yellow dotted line usually represents intermolecular interactions, like hydrogen bonds.
The parameters dissociation constant (Kd) and ligand efficiency (LE) were used to compare the affinity of the molecules studied and SARS-CoV-2 Mpro. The Kd of a ligand-protease complex, the values shown indicate the strength of the protein-ligand interaction. Very low values are an indicator that the ligand has a very close bond to the protein. LE represents the average bonding energy per non-hydrogen atom, giving standardized values allowing to compare the molecules derived from coumarins and quinolines of different sizes, see Table 2. The six best ligands obtained from the docking exhibit low Kd values, which leads to the conclusion that these complexes are the most stable of the series presented, these ligands are CTR6, 7HC6, 7HC5, CTR9, 7HC3 and 8HQ6, including the reference ligand 13b. The results are coherent with those obtained in the molecular docking, where these complexes according to the values of ∆Ebinding showed greater stability. Default tolerable values of LE of inhibitor candidate compounds should show LE values >0.3 kcal-mol−1. According to the values, the compounds 7HC6, 7HC5, CTR9, 7HC3 and 8HQ6 are excellent prospects to be used as SARS-CoV-2 Mpro inhibitors.
Molecular dynamics simulations were performed in 200 ns to analyze the steady nature and conformations stability of ligand-SARS-CoV-2 Mpro complexes (ligands: CTR9, 7HC6, CTR6, 7HC3, 8HQ6 and 7HC5).
The RMSD was used to estimate the stability of protein-ligand systems. RMSD trajectories of SARS-CoV-2 Mpro-ligand complexes during 200 ns simulation indicated that the complexes formed with the ligands during the DM simulations have a high stability during the simulation time (Figure 3). The structure does not show significant changes, in this case there is an increase of the RMSD until reaching a point in which the values fluctuate around values of 0.5 and 1.8 Å of RMSD. After a Molecular dynamics of 200 ns the structures remain within the parameter that considers the system to be in equilibrium, therefore, no complex suffered structural destabilization during the simulation. Deviations with a maximum difference of 3.0 Å of RMSD (Carugo, 2003) indicate that the system is in equilibrium, situation that is fulfilled for the simulation of the possible SARS-CoV-2 Mpro indicating equilibrium states of the ligands within the active site of protease. Also, RMSD curves for 7HC5 and CTR6 are remarkably more stable than those of CTR9, 7HC6, 7HC3 and 8HQ6. To complement the analysis carried out from the RMSD, the study of the Radius of Gyration (RGyr) was carried out for the same runs. From RGyr analysis Figure 4, we can conclude that the RGyr of ligands 7HC5 and 7HC3 have values that oscillate in an interval close to 3.0 Å, for the case of CTR9, 8HQ6 and 7HC6 have values fluctuating in an interval close to 3.5 Å and for the CTR6 ligand it has values higher than 4.0 Å. The stable values during the 200 ns simulation for RGyr indicate that ligand binding at the active site of the protein does not induce major conformational changes in the protein structure.
FIGURE 3. Root Mean Square Deviation (RMSD) as a function of simulated times for the complexes formed between SARS-CoV-2 Mpro and (A) CTR9, (B) 7HC6, (C) CTR6, (D) 7HC3, (E) 8HQ6 and (F) 7HC5 molecules.
FIGURE 4. Radius of gyration for the SARS-CoV-2 Mpro in complex with CTR9, 7HC6, CTR6, 7HC3, 8HQ6 and 7HC5, during 200 ns simulation time.
With the purpose of identifying the deviation of the ligand from respect to its initial position and the movement of proteins residues, the Normal Mode Analysis (NMA) and Root Mean Square Fluctuations (RMSF) values were calculated averaging over all the conformations sampled during the last 100 ns simulation. The NMA and RMSF were calculated using the Cα atom of each amino acid residue as a reference and the graph was used to represent the fluctuations in the residue level. NMA plot in Figure 5 shows a similar trend of residue fluctuation profile for the complex with an average NMA of 1.0 × 10–6 fluctuations. The two CTR6-protease and 7HC3-protease complexes showed a comparatively higher fluctuation in some residues. This trend in the quadratic displacement figure of the complex suggests that the binding of the six compounds to the protein showed stability and no effect on the flexibility of the protein was observed in the whole range of the simulations. The N-terminus to C-terminus of the proteins normally present great fluctuations and in most cases their movement does not represent importance. As shown in Figure 5, the RMSF graph, all the compounds made the residues present the same fluctuation, except for the two 8HQ6-protease and 7HC5-protease complexes showed a comparatively higher fluctuation in some residues. We consider and from the theoretical point of view, that these ligands present movements in the active site to settle in the best orientation, reason why part of the amino acidic residues fluctuate more than normal. However, other ligands exhibit good behavior and their RMSF values show that they can handle the fluctuations of the residues. On the active site of the protease, the fluctuation values of the main residues (Zhang et al., 2020a) (His41, His163, His164, Phe140 and Cys145) of the six selected molecules were similar among them. The results of the MD simulation indicate that two of the ligands obtained from the coupling analysis (CTR9 and 7HC6) remain close to their initial locations even in uncontrolled simulations, which points to the constitution of stable complexes. From these results it can be clearly deduced that it is likely that the molecules CTR9 and 7HC6 play the same role in inhibiting SARS-CoV-2 Mpro as 13b.
FIGURE 5. Normal Mode Analysis and RMSF of the α-carbon. A main component analysis was carried out using the last 100 ns trajectories, and the main normal mode of movement was obtained. The displacement was plotted for each residue of SARS-CoV-2 Mpro in complex with CTR9, 7HC6, CTR6, 7HC3, 8HQ6 and 7HC5. In grey boxes represented pocket site residues. His41 and Cys145 residues of the catalytic site are highlighted with bold font.
The analyses of trajectories indicate that during most of the simulation the ligands CTR9, 7HC6, CTR6, 7HC5, 7HC3 and 8HQ6 maintain hydrogen bonds with residues of the active site of SARS-CoV-2 Mpro. However, the number of hydrogen bridges formed was different for each ligand (Figure 6). CTR9 formed three hydrogen bridges between the residues Cys44, His41 and Thr26, highlighting the participation of the residues Glu166, Cys145, Asn142 and Asp187. 7HC6 formed two hydrogen bridges between the residues Thr24 and Thr25, highlighting the participation of the residues Leu27, Ser46, Met49 and Glu166. In the case of CTR6, three hydrogen bridges between the residues Glu166, Gln189 and Thr190 were determined, highlighting the participation of the residues Arg188 and Ser46. 7HC3 formed one hydrogen bridge with the residue Glu166, highlighting the participation of the residues His163, Met49 and Phe140. Three hydrogen bridges are formed between 8HQ6 and the residues Asp187, Glu166 and His163, highlighting the participation of the residues Gln189, Phe140, Ser144 and Val186. Finally, 7HC5 formed one hydrogen bridge with the Glu166 residue, highlighting the participation of the residues Leu50, Asn142 and Phe140. These residues, see Figures 6 and 7, are consistent with previous theoretical-experimental studies carried out by Dai et al. (Dai et al., 2020), where they detail the interaction that some of the synthesized compounds have with the active site of the protease.
FIGURE 6. Fraction of intermolecular hydrogen bonds for SARS-CoV-2 Mpro interacting with (A) CTR9, (B) 7HC6, (C) CTR6, (D) 7HC3, (E) 8HQ6 and (F) 7HC5. The graph bar shows the most common hydrogen bonds formed between the residues on the pocket and the inhibitors. Values obtained from CPPTRAJ script in AMBER.
FIGURE 7. Schematic representations of main component analysis of their respective production run for ligands (A) CTR9, (B) 7HC6, (C) CTR6, (D) 7HC3, (E) 8HQ6 and (F) 7HC5 bound to SARS-CoV-2 Mpro. The surrounding amino acid residues in the binding pocket of SARS-CoV-2 Mpro within 3Å from ligands. The yellow dotted line usually represents intermolecular interactions, like hydrogen bonds.
The noncovalent interactions analysis labeled all the hydrogen-bonding interactions in total agreement with the molecular dynamics simulations, providing a qualitative confirmation of these interactions, using a topological and visual analysis of a scalar field related to the electron density (Figure 8). These results suggest that the better inhibitors character is due to direct mechanisms.
FIGURE 8. Schematic representations of main component analysis of their respective production run for NCIPLOT isosurface gradient (0.6 au) of ligands (A) CTR9, (B) 7HC6, (C) CTR6, (D) 7HC3, (E) 8HQ6 and (F) 7HC5 on the structure of SARS-CoV-2 Mpro. The color scale is −2.0 < ρ < 2.0 au.
Finally, the binding free energy (MM-GBSA) was estimated subsequent to the MD simulation; the last 50 ns for all the complexes and the results are given in Table 3. CTR9 and 7HC6 compounds depicted the lowest binding free energy (−24.8 and −24.7 kcal mol−1) with SARS-CoV-2 Mpro, while the compounds CTR6, 7HC5, 7HC3 and 8HQ6 showed relatively higher binding energy (−24.7, −22.8, −22.6, −20.5 and −20.5 kcal mol−1). On the other hand, the reference compound 13b showed the lowest binding free energy (−29.1 kcal mol−1) with the SARS-CoV-2 Mpro in comparison to compound CTR9, with a slight difference of 4.3 kcal mol−1. Although compound 13b has a lowest binding free energy, it presents the problem of breaking the rules of Lipinski and Veber rules, however compound CTR9 does not.
TABLE 3. Predicted binding free energies (∆Gbinding) calculated from molecular dynamics simulation through the MM/GBSA protocol for SARS-CoV-2 Mpro complexes.
In the search for new drugs, safety is very important and the regulations related to ADMET (Absorption, Distribution, Metabolism, Excretion and Toxicity), most of the time are the cause for a drug to fail. Therefore, it is of utmost importance to identify aspects such as the toxicity of compounds in early stages of development and thus avoid the loss of resources and time. (Sivamani et al., 2012). To evaluate the best ligands as potential anti-SARS-CoV-2 activity drugs; we have calculated some pharmacokinetic properties (Table 4). These results were compared to Veber’s (Veber et al., 2002), Pfizer’s (Hughes et al., 2008) and Lipinski’s rule (Lipinski et al., 2001). in the development of new drugs if a molecule complies only with one of the Lipinski's rule is not an appropriate candidate and it is not relevant to continue with the study, however, by presenting a greater number of rules the probabilities of being a candidate begin to increase and deepen their study. In accordance with Veber's rule, if a compound does not satisfy at least two parameters, it is not a candidate for further development. In addition, Pfizer 3/75 toxicity rules have also been taken into account in this study, concluding that if any of the proposed ligands do not meet the established parameters, then it is not a suitable candidate.
ADME prediction showed that in most cases, all the compounds proposed in this study satisfy with the Veber’s, Pfizer’s and Lipinski’s rule. This suggests that these ligands could be safe molecules for use as anti-SARS-CoV-2 activity drugs. In the case of reference ligand 13b presents a violation of Lipinski’s and Veber’s rule, due to their molecular weight, topological polar surface area and rotatable bond count. The values of these properties are higher than the admissible limit, making this substance fat-soluble which indicates a tendency to be more toxic and less selective to their target. In the case of the six best compounds found in the docking simulations (CTR6, 7HC6, 7HC5, CTR9, 7HC3 and 8HQ6), all of them satisfactorily meet the Veber’s, Pfizer’s and Lipinski’s rule. These compounds represent most promising compounds to molecular dynamics simulation and MM-GBSA.
This paper predicts that compounds derived from coumarins and quinolines that can be successfully potential drugs to treat viral diseases such as COVID-19. Herein, we used a computational chemistry protocol to identify the ligands most promising candidates that may inhibit main protease of SARS-CoV-2 activity determined by means of this protocol involves of molecular dockings, molecular dynamics simulations, MM-GBSA, NCI and ADMET properties to predict whether these compounds are appropriate to be utilized in an anti-COVID-19 therapy. We identified six compounds (CTR9, 7HC6, CTR6, 7HC5, 7HC3, 8HQ6) that are already synthesized (García-Beltrán et al., 2013; Mena et al., 2015; Aguirre et al., 2017; Garćia-Beltran et al., 2017) with a potential inhibition of main protease of SARS-CoV-2. These compounds might be repurposed against COVID-19. These hits were described as drug-like compounds and showed harmless ADMET properties and may aid in developing and optimizing more efficient and potent COVID-19 inhibitors. Trajectory analysis showed that the studied complexes display structural stability during the MD runs. These results encourage further in vitro and in vivo investigations and also preventively boost the traditional use of coumarins and quinolines derivatives preventively. We anticipate that the insights obtained in the present study may prove valuable for researching and developing novel anti-COVID-19 therapeutic agents in the future.
The original contributions presented in the study are included in the article/Supplementary Material, further inquiries can be directed to the corresponding author.
OY, OG-B, CA, WT and FG-N contributed to the conception and design of the study; OY, MIO and JPD preformed the theoretical calculations; OY, EU, MO and JP-D organized the database; OY, MO, JP-D, CA and OG-B wrote the first draft of the manuscript; OY, OG-B, and FG-N wrote sections of the manuscript. All authors contributed to manuscript revision, read and approved the submitted version.
The authors declare that the research was conducted in the absence of any commercial or financial relationships that could be construed as a potential conflict of interest.
The authors express their thanks to Grant RC-FP44842-212-2018 Colombia científica and Universidad de Ibagué and for the financial support of the ANID/PIA/ACT192144. OG-B Thank funding from the Ministry of Science, Technology and Innovation, the Ministry of Education, the Ministry of Industry, Commerce and Tourism, and ICETEX, Programme Ecosistema Científico-Colombia Científica, from the Francisco José de Caldas Fund, Grant RC-FP44842–212-2018.
Abad-Zapatero, C. (2013). Ligand efficiency indices for drug Discovery, in Editor C. Abad-Zapatero (San Diego: Academic Press). doi:10.1016/B978-0-12-404635-1.00009-8
Abad-Zapatero, C. (2007). Ligand efficiency indices for effective drug discovery. Expet Opin. Drug Discov. 2, 469–488. doi:10.1517/17460441.2.4.469
Abad-Zapatero, C., Perišić, O., Wass, J., Bento, A. P., Overington, J., Al-Lazikani, B., et al. (2010). Ligand efficiency indices for an effective mapping of chemico-biological space: the concept of an atlas-like representation. Drug Discov. Today 15, 804–811. doi:10.1016/J.DRUDIS.2010.08.004
Abroshan, H., Akbarzadeh, H., and Parsafar, G. A. (2010). Molecular dynamics simulation and MM-PBSA calculations of sickle cell hemoglobin in dimer form with Val, Trp, or Phe at the lateral contact. J. Phys. Org. Chem. 23, 866–877. doi:10.1002/poc.1679
Aguirre, P., García-Beltrán, O., Tapia, V., Muñoz, Y., Cassels, B. K., and Núñez, M. T. (2017). Neuroprotective effect of a new 7,8-Dihydroxycoumarin-based Fe2+/Cu2+ chelator in cell and animal models of Parkinson’s disease. ACS Chem. Neurosci. 8, 178–185. doi:10.1021/acschemneuro.6b00309
Anandakrishnan, R., Aguilar, B., and Onufriev, A. V. (2012). H++ 3.0: automating pK prediction and the preparation of biomolecular structures for atomistic molecular modeling and simulations. Nucleic Acids Res. 40, W537–W541. doi:10.1093/nar/gks375
Arshad, S., Kilgore, P., Chaudhry, Z. S., Jacobsen, G., Wang, D. D., Huitsing, K., et al. (2020). Treatment with hydroxychloroquine, azithromycin, and combination in patients hospitalized with COVID-19. Int. J. Infect. Dis. 97, 396–403. doi:10.1016/j.ijid.2020.06.099
Balaramnavar, V. M., Ahmad, K., Saeed, M., Ahmad, I., Kamal, M., and Jawed, T. (2020). Pharmacophore-based approaches in the rational repurposing technique for FDA approved drugs targeting SARS-CoV-2 Mpro. RSC Adv. 10, 40264–40275. doi:10.1039/D0RA06038K
Berman, H. M., Westbrook, J., Feng, Z., Gilliland, G., Bhat, T. N., Weissig, H., et al. (2000). The protein Data Bank. Nucleic Acids Res. 28, 235–242. doi:10.1093/nar/28.1.235
Bikadi, Z., and Hazai, E. (2009). Application of the PM6 semi-empirical method to modeling proteins enhances docking accuracy of AutoDock. J. Cheminf. 1, 1–16. doi:10.1186/1758-2946-1-15
Carugo, O. (2003). How root-mean-square distance (r.m.s.d.) values depend on the resolution of protein structures that are compared. J. Appl. Crystallogr. 36, 125–128. doi:10.1107/S0021889802020502
Cavalluzzi, M. M., Mangiatordi, G. F., Nicolotti, O., and Lentini, G. (2017). Ligand efficiency metrics in drug discovery: the pros and cons from a practical perspective. Expet Opin. Drug Discov. 12, 1087–1104. doi:10.1080/17460441.2017.1365056
Chan, J. F.-W., Kok, K.-H., Zhu, Z., Chu, H., To, K. K.-W., Yuan, S., et al. (2020). Genomic characterization of the 2019 novel human-pathogenic coronavirus isolated from a patient with atypical pneumonia after visiting Wuhan. Emerg. Microb. Infect. 9, 221–236. doi:10.1080/22221751.2020.1719902
Chan, J. F. W., Lau, S. K. P., To, K. K. W., Cheng, V. C. C., Woo, P. C. Y., and Yuen, K.-Y. (2015). Middle East respiratory syndrome coronavirus: another zoonotic betacoronavirus causing SARS-like disease. Clin. Microbiol. Rev. 28, 465–522. doi:10.1128/CMR.00102-14
Chen, Y., Liu, Q., and Guo, D. (2020). Emerging coronaviruses: genome structure, replication, and pathogenesis. J. Med. Virol. 92, 418–423. doi:10.1002/jmv.25681
Chu, C. M., Cheng, V. C. C., Hung, I. F. N., Wong, M. M. L., Chan, K. H., Chan, K. S., et al. (2004). Role of lopinavir/ritonavir in the treatment of SARS: initial virological and clinical findings. Thorax. 59, 252–256. doi:10.1136/thorax.2003.012658
Contreras-García, J., Johnson, E. R., Keinan, S., Chaudret, R., Piquemal, J.-P., Beratan, D. N., et al. (2011). NCIPLOT: a program for plotting noncovalent interaction regions. J. Chem. Theor. Comput. 7, 625–632. doi:10.1021/ct100641a
Cortegiani, A., Ingoglia, G., Ippolito, M., Giarratano, A., and Einav, S. (2020). A systematic review on the efficacy and safety of chloroquine for the treatment of COVID-19. J. Crit. Care 21, 684. doi:10.1016/j.jcrc.2020.03.005
Coulerie, P., Maciuk, A., Lebouvier, N., Hnawia, E., Guillemot, J. C., Canard, B., et al. (2013). Phytochemical study of myrtopsis corymbosa, perspectives for anti-dengue natural compound research. Record Nat. Prod. 7, 250–253.
COVID-19 Map - Johns Hopkins Coronavirus Resource Center (2020). https://coronavirus.jhu.edu/map.html.[Accessed]
Cuesta, A., Wan, X., Burlingame, A. L., and Taunton, J. (2020). Ligand conformational bias Drives enantioselective modification of a surface-exposed lysine on Hsp90. J. Am. Chem. Soc. 142, 3392–3400. doi:10.1021/jacs.9b09684
Dai, W., Zhang, B., Su, H., Li, J., Zhao, Y., Xie, X., et al. (2020). Structure-based design of antiviral drug candidates targeting the SARS-CoV-2 main protease. Science (80-.). 4489, 1–10. doi:10.1126/science.abb4489
Daina, A., Michielin, O., and Zoete, V. (2017). SwissADME: a free web tool to evaluate pharmacokinetics, drug-likeness and medicinal chemistry friendliness of small molecules. Sci. Rep. 7, 1–13. doi:10.1038/srep42717
Deng, L., Li, C., Zeng, Q., Liu, X., Li, X., Zhang, H., et al. (2020). Arbidol combined with LPV/r versus LPV/r alone against Corona Virus Disease 2019: a retrospective cohort study. J. Infect. 17, 592. doi:10.1016/j.jinf.2020.03.002
Elfiky, A. A. (2020). Ribavirin, Remdesivir, Sofosbuvir, Galidesivir, and Tenofovir against SARS-CoV-2 RNA dependent RNA polymerase (RdRp): a molecular docking study. Life Sci. 253, 117592. doi:10.1016/j.lfs.2020.117592
Fanfrlík, J., Bronowska, A. K., Řezáč, J., Přenosil, O., Konvalinka, J., and Hobza, P. (2010). A reliable docking/scoring scheme based on the semiempirical quantum mechanical PM6-DH2 method accurately covering dispersion and H-bonding: HIV-1 protease with 22 ligands. J. Phys. Chem. B. 114, 12666–12678. doi:10.1021/jp1032965
Gao, K., Nguyen, D. D., Chen, J., Wang, R., and Wei, G.-W. (2020). Repositioning of 8565 existing drugs for COVID-19. J. Phys. Chem. Lett. 11, 5373–5382. doi:10.1021/acs.jpclett.0c01579
Garćia-Beltran, O., Mena, N. P., Aguirre, P., Barriga-Gonzalez, G., Galdamez, A., Nagles, E., et al. (2017). Development of an iron-selective antioxidant probe with protective effects on neuronal function. PLoS One 12, 1–18. doi:10.1371/journal.pone.0189043
García-Beltrán, O., Mena, N., Yañez, O., Caballero, J., Vargas, V., Nuñez, M. T., et al. (2013). Design, synthesis and cellular dynamics studies in membranes of a new coumarin-based “turn-off” fluorescent probe selective for Fe 2+. Eur. J. Med. Chem. 67, 60–63. doi:10.1016/j.ejmech.2013.06.022
Götz, A. W., Williamson, M. J., Xu, D., Poole, D., Le Grand, S., and Walker, R. C. (2012). Routine microsecond molecular dynamics simulations with AMBER on GPUs. 1. Generalized Born. J. Chem. Theor. Comput. 8, 1542–1555. doi:10.1021/ct200909j
Grein, J., Ohmagari, N., Shin, D., Diaz, G., Asperges, E., Castagna, A., et al. (2020). Compassionate use of Remdesivir for patients with severe covid-19. N. Engl. J. Med. 382, 2327–2336. doi:10.1056/NEJMoa2007016
Hayes, J. M., Archontis, G., and Wang, L. (2012). “MM-GB(PB)SA calculations of protein-ligand binding free energies” in Molecular dynamics. (Rijeka: IntechOpen). doi:10.5772/37107
Hirota, M., Shimosegawa, T., Kitamura, K., Takeda, K., Takeyama, Y., Mayumi, T., et al. (2020). Continuous regional arterial infusion versus intravenous administration of the protease inhibitor nafamostat mesilate for predicted severe acute pancreatitis: a multicenter, randomized, open-label, phase 2 trial. J. Gastroenterol. 55, 342–352. doi:10.1007/s00535-019-01644-z
Hoffmann, M., Kleine-Weber, H., Schroeder, S., Krüger, N., Herrler, T., Erichsen, S., et al. (2020). SARS-CoV-2 cell entry Depends on ACE2 and TMPRSS2 and is blocked by a clinically proven protease inhibitor. Cell 181, 271–280. doi:10.1016/j.cell.2020.02.052
Hou, X., Du, J., Zhang, J., Du, L., Fang, H., and Li, M. (2013). How to improve docking accuracy of AutoDock4.2: a case study using different electrostatic potentials. J. Chem. Inf. Model. 53, 188–200. doi:10.1021/ci300417y
Huang, C., Wei, P., Fan, K., Liu, Y., and Lai, L. (2004). 3C-like proteinase from SARS coronavirus catalyzes substrate hydrolysis by a general base mechanism. Biochemistry 43, 4568–4574. doi:10.1021/bi036022q
Hughes, J. D., Blagg, J., Price, D. A., Bailey, S., DeCrescenzo, G. A., Devraj, R. V., et al. (2008). Physiochemical drug properties associated with in vivo toxicological outcomes. Bioorg. Med. Chem. Lett. 18, 4872–4875. doi:10.1016/j.bmcl.2008.07.071
Hui, D. S., Azhar, I.E., Madani, T. A., Ntoumi, F., Kock, R., et al. (2020). The continuing 2019-nCoV epidemic threat of novel coronaviruses to global health—the latest 2019 novel coronavirus outbreak in Wuhan, China. Int. J. Infect. Dis. 91, 264–266. doi:10.1016/j.ijid.2020.01.009
Humphrey, W., Dalke, A., and Schulten, K. (1996). VMD: visual molecular dynamics. J. Mol. Graph. 14, 33–38. doi:10.1016/0263-7855(96)00018-5
Hwu, J. R., Huang, W.-C., Lin, S.-Y., Tan, K.-T., Hu, Y.-C., Shieh, F.-K., et al. (2019). Chikungunya virus inhibition by synthetic coumarin–guanosine conjugates. Eur. J. Med. Chem. 166, 136–143. doi:10.1016/j.ejmech.2019.01.037
Hwu, J. R., Singha, R., Hong, S. C., Chang, Y. H., Das, A. R., Vliegen, I., et al. (2008). Synthesis of new benzimidazole–coumarin conjugates as anti-hepatitis C virus agents. Antiviral Res. 77, 157–162. doi:10.1016/j.antiviral.2007.09.003
Jakalian, A., Bush, B. L., Jack, D. B., and Bayly, C. I. (2000). Fast, efficient generation of high-quality atomic charges. AM1-BCC model: I. Method. J. Comput. Chem. 21, 132–146. doi:10.1002/(SICI)1096-987X(20000130)21:2<132::AID-JCC5>3.0.CO;2-P
Jesumoroti, O. J., Faridoon, M. D., Isaacs, M., Hoppe, H. C., and Klein, R. (2019). Evaluation of novel N′-(3-hydroxybenzoyl)-2-oxo-2H-chromene-3-carbohydrazide derivatives as potential HIV-1 integrase inhibitors. Med. Chem. Commun. 10, 80–88. doi:10.1039/C8MD00328A
Jin, Z., Du, X., Xu, Y., Deng, Y., Liu, M., Zhao, Y., et al. (2020a). Structure of Mpro from SARS‐CoV‐2 and discovery of its inhibitors. Nature 582, 289. doi:10.1038/s41586-020-2223-y
Jin, Z., Du, X., Xu, Y., Deng, Y., Liu, M., Zhao, Y., et al. (2020a). Structure of Mpro from SARS-CoV-2 and discovery of its inhibitors. Nature 582, 289–293. doi:10.1038/s41586-020-2223-y
Jin, Z., Zhao, Y., Sun, Y., Zhang, B., Wang, H., Wu, Y., et al. (2020b). Structural basis for the inhibition of SARS-CoV-2 main protease by antineoplastic drug carmofur. Nat. Struct. Mol. Biol. 27, 529–532. doi:10.1038/s41594-020-0440-6
Johnson, E. R., Keinan, S., Mori-Sánchez, P., Contreras-García, J., Cohen, A. J., and Yang, W. (2010). Revealing noncovalent interactions. J. Am. Chem. Soc. 132, 6498–6506. doi:10.1021/ja100936w
Lipinski, C. A., Lombardo, F., Dominy, B. W., and Feeney, P. J. (2001). Experimental and computational approaches to estimate solubility and permeability in drug discovery and development settings. Adv. Drug Deliv. Rev. 46, 3–26. doi:10.1016/s0169-409x(00)00129-0
Liu, G., Wang, C., Wang, H., Zhu, L., Zhang, H., Wang, Y., et al. (2019). Antiviral efficiency of a coumarin derivative on spring viremia of carp virus in vivo. Virus Res. 268, 11–17. doi:10.1016/j.virusres.2019.05.007
Lopez, A., Duclos, G., Pastene, B., Bezulier, K., Guilhaumou, R., Solas, C., et al. (2020). Effects of hydroxychloroquine on covid-19 in intensive care unit patients: preliminary results. Int. J. Antimicrob. Agents 56, 106136. doi:10.1016/j.ijantimicag.2020.106136
Lu, R., Zhao, X., Li, J., Niu, P., Yang, B., Wu, H., et al. (2020). Genomic characterisation and epidemiology of 2019 novel coronavirus: implications for virus origins and receptor binding. Lancet 395, 565–574. doi:10.1016/S0140-6736(20)30251-8
MacLeod-Carey, D., Solis-Céspedes, E., Lamazares, E., and Mena-Ulecia, K. (2020). Evaluation of new antihypertensive drugs designed in silico using Thermolysin as a target. Saudi Pharm. J. 28, 582–592. doi:10.1016/j.jsps.2020.03.010
MacNeil, A., and Rollin, P. E. (2012). Ebola and marburg hemorrhagic fevers: neglected tropical diseases?. PLoS Negl. Trop. Dis. 6, e1546. doi:10.1371/journal.pntd.0001546
Madhavi Sastry, G., Adzhigirey, M., Day, T., Annabhimoju, R., and Sherman, W. (2013). Protein and ligand preparation: parameters, protocols, and influence on virtual screening enrichments. J. Comput. Aided Mol. Des. 27, 221–234. doi:10.1007/s10822-013-9644-8
Marsh, G. A., and Wang, L.-F. (2012). Hendra and Nipah viruses: why are they so deadly?. Curr. Opin. Virol. 2, 242–247. doi:10.1016/j.coviro.2012.03.006
McKee, D. L., Sternberg, A., Stange, U., Laufer, S., and Naujokat, C. (2020). Candidate drugs against SARS-CoV-2 and COVID-19. Pharmacol. Res. 157, 104859. doi:10.1016/j.phrs.2020.104859
Mena, N. P., García-Beltrán, O., Lourido, F., Urrutia, P. J., Mena, R., Castro-Castillo, V., et al. (2015). The novel mitochondrial iron chelator 5-((methylamino)methyl)-8-hydroxyquinoline protects against mitochondrial-induced oxidative damage and neuronal death. Biochem. Biophys. Res. Commun. 463, 787–792. doi:10.1016/j.bbrc.2015.06.014
Mermelstein, D. J., Lin, C., Nelson, G., Kretsch, R., McCammon, J. A., and Walker, R. C. (2018). Fast and flexible gpu accelerated binding free energy calculations within the amber molecular dynamics package. J. Comput. Chem. 39, 1354–1358. doi:10.1002/jcc.25187
Mishra, S., Pandey, A., and Manvati, S. (2020). An emerging antiviral agent. Heliyon. 6, e03217. doi:10.1016/j.heliyon.2020.e03217
Mori, N., Katayama, M., and Nukaga, S. (2020). Triple therapy with hydroxychloroquine, azithromycin, and ciclesonide for COVID-19 pneumonia. J. Microbiol. Immunol. Infect. 93, 5187. doi:10.1016/j.jmii.2020.09.003
Morris, G. M., Goodsell, D. S., Halliday, R. S., Huey, R., Hart, W. E., Belew, R. K., et al. (1998). Automated docking using a Lamarckian genetic algorithm and an empirical binding free energy function. J. Comput. Chem. 19, 1639–1662. doi:10.1002/(SICI)1096-987X(19981115)19:14<1639::AID-JCC10>3.0.CO;2-B
Mulangu, S., Dodd, L. E., Davey, R. T., Tshiani Mbaya, O., Proschan, M., Mukadi, D., et al. (2019). A randomized, controlled trial of ebola virus disease therapeutics. N. Engl. J. Med. 381, 2293–2303. doi:10.1056/NEJMoa1910993
Neria, E., Fisher, S., and Karplus, M. (1996). Simulation of activation free energies in molecular systems. J. Chem. Phys. 105, 1902–1921. doi:10.1063/1.472061
Ngo, S. T., Quynh Anh Pham, N., Thi Le, L., Pham, D.-H., and Vu, V. (2020). VComputational Determination of potential inhibitors of SARS-CoV-2 main protease. J. Chem. Inf. Model. 4, 91. doi:10.1021/acs.jcim.0c00491
ÖzpInar, G. A., Peukert, W., and Clark, T. (2010). An improved generalized AMBER force field (GAFF) for urea. J. Mol. Model. 16, 1427–1440. doi:10.1007/s00894-010-0650-7
Pavurala, S., Vaarla, K., Kesharwani, R., Naesens, L., Liekens, S., and Vedula, R. R. (2018). Bis coumarinyl bis triazolothiadiazinyl ethane derivatives: synthesis, antiviral activity evaluation, and molecular docking studies. Synth. Commun. 48, 1494–1503. doi:10.1080/00397911.2018.1455871
Peiris, J. S. M., Lai, S. T., Poon, L. L. M., Guan, Y., Yam, L. Y. C., Lim, W., et al. (2003). Coronavirus as a possible cause of severe acute respiratory syndrome. Lancet (London, England). 361, 1319–1325. doi:10.1016/s0140-6736(03)13077-2
Reynolds, C. H., Tounge, B. A., and Bembenek, S. D. (2008). Ligand binding efficiency: trends, physical basis, and implications. J. Med. Chem. 51, 2432–2438. doi:10.1021/jm701255b
Řezáč, J., and Hobza, P. (2012). Advanced corrections of hydrogen bonding and dispersion for semiempirical quantum mechanical methods. J. Chem. Theor. Comput. 8, 141–151. doi:10.1021/ct200751e
Rothan, H. A., and Byrareddy, S. N. (2020). The epidemiology and pathogenesis of coronavirus disease (COVID-19) outbreak. J. Autoimmun. 109, 102433. doi:10.1016/j.jaut.2020.102433
Salomon-Ferrer, R., Case, D. A., and Walker, R. C. (2013). An overview of the Amber biomolecular simulation package. Wiley Interdiscip. Rev. Comput. Mol. Sci. 3, 198–210. doi:10.1002/wcms.1121
Sanner, M. F. (1999). Python: a programming language for software integration and development. J. Mol. Graph. Model. 17, 55–84. doi:10.1016/S1093-3263(99)99999-0
Sissoko, D., Laouenan, C., Folkesson, E., M’Lebing, A. B., Beavogui, A. H., Baize, S., et al. (2016). Experimental treatment with favipiravir for ebola virus disease (the JIKI trial): a historically controlled, single-arm proof-of-concept trial in Guinea. PLoS Med. 13, 1–36. doi:10.1371/journal.pmed.1001967
Sivamani, P., Singaravelu, G., Thiagarajan, V., Jayalakshmi, T., and Kumar, G. R. (2012). Comparative molecular docking analysis of essential oil constituents as elastase inhibitors. Bioinformation 8, 457–460. doi:10.6026/97320630008457
Song, L., Lee, T.-S., Zhu, C., York, D. M., and Kajid, M. M. (2019). Validation of AMBER/GAFF for relative free energy calculations. ChemrXiv. doi:10.26434/chemrxiv.7653434.v1
Stewart, J. J. P. (2007). Optimization of parameters for semiempirical methods V: modification of NDDO approximations and application to 70 elements. J. Mol. Model. 13, 1173–1213. doi:10.1007/s00894-007-0233-4
To, K. K., Ng, K. H., Que, T.-L., Chan, J. M., Tsang, K.-Y., Tsang, A. K., et al. (2012). Avian influenza A H5N1 virus: a continuous threat to humans. Emerg. Microb. Infect. 1, 1–12. doi:10.1038/emi.2012.24
To, K. K. W., Chan, J. F. W., Chen, H., Li, L., and Yuen, K.-Y. (2013). The emergence of influenza A H7N9 in human beings 16 years after influenza A H5N1: a tale of two cities. Lancet Infect. Dis. 13, 809–821. doi:10.1016/S1473-3099(13)70167-1
Trott, O., and Olson, A. (2010). AutoDock Vina: improving the speed and accuracy of docking with a new scoring function, efficient optimization and multithreading. J. Comput. Chem. 31, 455–461. doi:10.1002/jcc.21334
ul Qamar, M. T., Alqahtani, S. M., Alamri, M. A., and Chen, L.-L (2020). Structural basis of SARS-CoV-2 3CLpro and anti-COVID-19 drug discovery from medicinal plants. J. Pharm. Anal. 10, 313–319. doi:10.1016/j.jpha.2020.03.009
Veber, D. F., Johnson, S. R., Cheng, H.-Y., Smith, B. R., Ward, K. W., and Kopple, K. D. (2002). Molecular properties that influence the oral bioavailability of drug candidates. J. Med. Chem. 45, 2615–2623. doi:10.1021/jm020017n
Walls, A. C., Park, Y.-J., Tortorici, M. A., Wall, A., McGuire, A. T., and Veesler, D. (2020). Structure, function, and antigenicity of the SARS-CoV-2 spike glycoprotein. Cell 181, 281–292. doi:10.1016/j.cell.2020.02.058
Wang, J., Wolf, R. M., Caldwell, J. W., Kollman, P. A., and Case, D. A. (2004). Development and testing of a general amber force field. J. Comput. Chem. 25, 1157–1174. doi:10.1002/jcc.20035
WHO Director-General’s opening remarks at the media briefing on COVID-19–20 March (2020). https://www.who.int/dg/speeches/detail/who-director-general-s-opening-remarks-at-the-media-briefing-on-covid-19–-20-march-2020.[Accessed]
World Health Organization (2020). Surveillance case definitions for human infection with novel coronavirus (nCoV). Geneva: World Health Organization https://apps.who.int/iris/handle/10665/330376
Wrapp, D., Wang, N., Corbett, K. S., Goldsmith, J. A., Hsieh, C. L., Abiona, O., et al. (2020). Cryo-EM structure of the 2019-nCoV spike in the prefusion conformation. Science (80-.). 367, 1260–1263. doi:10.1126/science.aax0902
Wu, F., Zhao, S., Yu, B., Chen, Y.-M., Wang, W., Song, Z.-G., et al. (2020). A new coronavirus associated with human respiratory disease in China. Nature 579, 265–269. doi:10.1038/s41586-020-2008-3
Xu, Z., Peng, C., Shi, Y., Zhu, Z., Mu, K., Wang, X., et al. (2020). Nelfinavir was predicted to be a potential inhibitor of 2019-nCov main protease by an integrative approach combining homology modelling, molecular docking and binding free energy calculation. bioRxiv. 13, 59. doi:10.1101/2020.01.27.921627.2020.01.27.921627
Yamamoto, M., Matsuyama, S., Li, X., Takeda, M., Kawaguchi, Y., Inoue, J., et al. (2016). Identification of nafamostat as a potent inhibitor of Middle East respiratory syndrome coronavirus S protein-mediated membrane fusion using the split-protein-based cell-cell fusion assay. Antimicrob. Agents Chemother. 60, 6532–6539. doi:10.1128/AAC.01043-16
Yu, H., and Adedoyin, A. (2003). ADME-Tox in drug discovery: integration of experimental and computational technologies. Drug Discov. Today 8, 852–861. doi:10.1016/S1359-6446(03)02828-9
Yuen, K. Y., Chan, P. K., Peiris, M., Tsang, D. N., Que, T. L., Shortridge, K. F., et al. (1998). Clinical features and rapid viral diagnosis of human disease associated with avian influenza A H5N1 virus. Lancet (London, England). 351, 467–471. doi:10.1016/s0140-6736(98)01182-9
Zaki, A. M., van Boheemen, S., Bestebroer, T. M., Osterhaus, A. D. M. E., and Fouchier, R. A. M. (2012). Isolation of a novel coronavirus from a man with pneumonia in Saudi arabia. N. Engl. J. Med. 367, 1814–1820. doi:10.1056/NEJMoa1211721
Zhang, L., Lin, D., Kusov, Y., Nian, Y., Ma, Q., Wang, J., et al. (2020a). α-Ketoamides as broad-spectrum inhibitors of coronavirus and enterovirus replication: structure-based design, synthesis, and activity assessment. J. Med. Chem. 18, 28. doi:10.1021/acs.jmedchem.9b01828
Zhang, L., Lin, D., Sun, X., Curth, U., Drosten, C., Sauerhering, L., et al. (2020b). Crystal structure of SARS-CoV-2 main protease provides a basis for design of improved α-ketoamide inhibitors. Science (80-.). 3405, eabb3405. doi:10.1126/science.abb3405
Zhou, P., Yang, X.-L., Wang, X.-G., Hu, B., Zhang, L., Zhang, W., et al. (2020). A pneumonia outbreak associated with a new coronavirus of probable bat origin. Nature 579, 270–273. doi:10.1038/s41586-020-2012-7
Keywords: SARS-CoV-2, coumarins, quinolines, protease, molecular dynamics
Citation: Yañez O, Osorio MI, Uriarte E, Areche C, Tiznado W, Pérez-Donoso JM, García-Beltrán O and González-Nilo F (2021) In Silico Study of Coumarins and Quinolines Derivatives as Potent Inhibitors of SARS-CoV-2 Main Protease. Front. Chem. 8:595097. doi: 10.3389/fchem.2020.595097
Received: 15 August 2020; Accepted: 16 December 2020;
Published: 08 February 2021.
Edited by:
Sonia Di Gaetano, Italian National Research Council, ItalyReviewed by:
Gabriella Gabriella, University of Florence, ItalyCopyright © 2021 Yañez, Osorio, Uriarte, Areche, Tiznado, Perez-Donoso, García-Beltrán and González-Nilo. This is an open-access article distributed under the terms of the Creative Commons Attribution License (CC BY). The use, distribution or reproduction in other forums is permitted, provided the original author(s) and the copyright owner(s) are credited and that the original publication in this journal is cited, in accordance with accepted academic practice. No use, distribution or reproduction is permitted which does not comply with these terms.
*Correspondence: Olimpo García-Beltrán, am9zZS5nYXJjaWFAdW5pYmFndWUuZWR1LmNv; Fernando González-Nilo, ZmVybmFuZG8uZ29uemFsZXpAdW5hYi5jbA==
Disclaimer: All claims expressed in this article are solely those of the authors and do not necessarily represent those of their affiliated organizations, or those of the publisher, the editors and the reviewers. Any product that may be evaluated in this article or claim that may be made by its manufacturer is not guaranteed or endorsed by the publisher.
Research integrity at Frontiers
Learn more about the work of our research integrity team to safeguard the quality of each article we publish.