- 1Department of Biochemistry, Indian Institute of Science, Bangalore, India
- 2Center for Infectious Disease Research, Indian Institute of Science, Bangalore, India
- 3Center for Biosystems Science and Engineering, Indian Institute of Science, Bangalore, India
Tuberculosis is one of the deadliest infectious diseases worldwide and the prevalence of latent tuberculosis acts as a huge roadblock in the global effort to eradicate tuberculosis. Most of the currently available anti-tubercular drugs act against the actively replicating form of Mycobacterium tuberculosis (Mtb), and are not effective against the non-replicating dormant form present in latent tuberculosis. With about 30% of the global population harboring latent tuberculosis and the requirement for prolonged treatment duration with the available drugs in such cases, the rate of adherence and successful completion of therapy is low. This necessitates the discovery of new drugs effective against latent tuberculosis. In this work, we have employed a combination of bioinformatics and chemoinformatics approaches to identify potential targets and lead candidates against latent tuberculosis. Our pipeline adopts transcriptome-integrated metabolic flux analysis combined with an analysis of a transcriptome-integrated protein-protein interaction network to identify perturbations in dormant Mtb which leads to a shortlist of 6 potential drug targets. We perform a further selection of the candidate targets and identify potential leads for 3 targets using a range of bioinformatics methods including structural modeling, binding site association and ligand fingerprint similarities. Put together, we identify potential new strategies for targeting latent tuberculosis, new candidate drug targets as well as important lead clues for drug design.
1. Introduction
Tuberculosis, despite being one of the oldest infectious diseases known to humankind with a history dating back to 70,000 years, continues to affect millions of people, causing over 0.1 million deaths globally every year (Barberis et al., 2017; WHO, 2018). A major roadblock in TB eradication is the capacity of the causative organism Mycobacterium tuberculosis (Mtb) to remain in a non-replicative state inside an infected person for decades without causing any symptoms. An approximate 1.7 billion of the world's population is thought to harbor latent tuberculosis (Ai et al., 2016; WHO, 2018). These individuals carry about a 10% lifetime risk of developing active TB, a risk that increases in the presence of factors such as malnutrition or immunocompromised conditions. The treatment of latent TB, therefore, becomes an important requirement for successful control and management of TB around the world.
Clinically, latent TB signifies a state of persistent immune response to M.tuberculosis infection, where the host remains asymptomatic and the bacilli are in a non-replicating dormant state but have the potential to reactivate when the host gets immunocompromised. Mtb owes its ability to reside for long periods inside the host in a dormant condition to its capacity of rewiring its own metabolism to a viable yet non-replicative state which allows its maintenance and evasion of host immune mechanisms (Gengenbacher et al., 2010). It gets engulfed by alveolar macrophages at the site of infection, but prevents phagosomal maturation and thus stops its own subsequent destruction by the host immune system, forming granuloma (Russell, 2001; Pethe et al., 2004). In most of the cases, the granuloma becomes solid and fibrous, successfully containing the infection inside and establishing latent TB infection (Gengenbacher and Kaufmann, 2012). In order to survive inside the host cells, the bacteria must adapt to their hostile environment where they are challenged with various stresses such as low nutrients, hypoxia, low pH etc. (Schnappinger et al., 2003). Multiple in vitro models have elucidated different aspects of the biochemical and molecular correlates of Mtb dormancy. A metabolic downshift induced by the granuloma environment is a predominant change that the pathogen goes through in order to achieve the dormant state (Betts et al., 2002). The glyoxylate shunt has been proven to be essential for bacterial survival during dormancy (McKinney et al., 2000; Gengenbacher et al., 2010). Hypoxia has been one of the most leveraged stress conditions in induction of dormancy in vitro and it has led to the identification of the Dos regulon that controls about 50 genes and regulates bacterial adaptation into non-replicative state in hypoxia (Park et al., 2003; Voskuil et al., 2004). Utilization of lipids as an alternative carbon source has also been suggested as an important adaptation in dormancy (Santucci et al., 2016).
Most of the anti-tubercular drugs target cellular processes in actively replicating cells such as the transcription, cell wall synthesis and the generation of ATP (Campbell et al., 2001; Timmins and Deretic, 2006; Nagabushan and Roopadevi, 2014). These drugs lose their potency against latent tuberculosis due to the metabolic alterations seen in the dormant bacteria. At present, for lack of better options, the existing anti-tubercular drugs are administered for prolonged periods to latent TB individuals. Although, both monotherapy and combination therapy have been reported to have similar efficacy, the longer duration monotherapy has been in common practice due to the possibility of drug interaction between rifampicin/ rifapentine and other drugs like the ones used for treatment of HIV (Fox et al., 2017; Saha et al., 2020). This prolonged antibiotic course is often perceived as unnecessary for an asymptomatic condition by both the patient and the clinician, leading to lack of adherence and an average of 20–30% treatment completion rate in most cases (Horsburgh, 2004). Therefore to successfully eradicate TB when about 2 billion of the world's population could be acting as the pathogen reservoir, new shorter drug regimens are urgently required.
Although drug discovery for tuberculosis has gained momentum with the emergence of MDR and XDR Mtb strains, the number of new drugs has not increased significantly. An ideal new TB drug should lead to a shorter treatment duration than those in practice, have minimal toxicity and interaction with other drugs and kill different subpopulations observed in clinical TB with different replication rates (Lechartier et al., 2014). Metronidazole, pretomanid and certain hydrazone molecules have shown promise against non-replicating Mtb (Wayne and Sramek, 1994; Stover et al., 2000; Bonnett et al., 2018). High-throughput compound screening and bioinformatics analysis have also identified potential drug candidates against dormant tuberculosis (Cho et al., 2007; Raman et al., 2008; Defelipe et al., 2016). Yet most of the proposed drugs in different phases of clinical trial target processes like DNA replication and cell wall synthesis which have limited efficacy against dormant bacteria (Dietze et al., 2008; Gler et al., 2012; Naidoo et al., 2017). Since the complete sequencing of Mtb genome (Cole et al., 1998), different omics-based studies have provided insights into the biology of tuberculosis in active and latent conditions and have been utilized for drug target identification (Lew et al., 2011; Goff et al., 2020). Although a drug-to-target pipeline has been traditionally more successful in discovering new TB drugs than a target-to-drug pipeline, it has not been able to identify potential drugs for specifically killing the non-replicating pathogen (Lechartier et al., 2014). On the other hand, drugs like Bedaquiline, that target the ATP homeostasis are shown to have high efficacy in killing dormant bacteria as they target processes necessary for bacterial survival in dormancy (Koul et al., 2008; Rao et al., 2008; Kaushik et al., 2019). It shows that in the case of latent TB, a target-based drug approach might be more efficient in identifying new drugs targeted toward processes crucial for bacterial dormancy.
In this work, we have used a systems biology based computational pipeline to strategically identify six different potential drug targets for tackling latent TB. We further select three of these as candidate targets and use multiple bioinformatics and chemoinformatics approaches to identify lead clues for these targets that may aid in the process of drug discovery. Our approach utilizes a combination of transcriptome analysis, genome-scale metabolic modeling, condition-specific genome-wide protein-protein interaction networks and bioinformatics based on protein sequences, protein structure, binding sites and chemoinformatics that analyse the chemical structures and topologies of the ligand molecules.
2. Methods
2.1. Curation of Publicly Available Transcriptome Data
The objective of this study was to identify potential drug targets for latent TB. Transcriptome data was not publicly available from dormant pathogens residing in infected host tissues. But, a significant number of studies have been successful in establishing dormancy of Mtb in vitro with the application of different stress conditions, which partially replicates the multitude of stresses faced by the pathogen inside the host granuloma environment. We searched in the public repository Gene Expression Omnibus (GEO) (Edgar et al., 2002) for transcriptome data of dormant Mtb and selected the datasets listed in Table 1 based on the following criteria: (a) presence of well-defined timepoints of Mtb dormancy, i.e., establishment of bacteriostasis and non-culturability induced by external stress, (b) samples from exponential/ logarithmic growth phase in absence of an external stress, (c) data generated with microarray or RNAseq techniques, (d) ≥ 3 samples per condition and time points, from which we selected one dataset each (that has data for the maximum number of time points) representing different stress conditions.
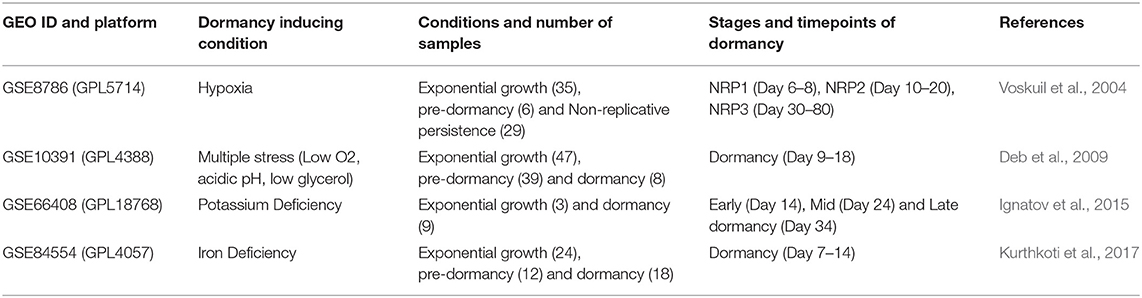
Table 1. Transcriptome datasets from GEO database considered in this study for in vitro Mtb for 4 different dormancy models.
2.2. Gene Expression Analysis
Raw data from the selected datasets listed in Table 1 were downloaded from GEO. Each dataset was pre-processed and normalized separately using EdgeR and limma package from Bioconductor in R statistical environment (Robinson et al., 2010; Huber et al., 2015; Ritchie et al., 2015). In brief, the two-channel microarray datasets were background corrected, followed by within and between array normalization with loess and quantile methods, respectively. The RNAseq dataset, GSE66408, was subjected to the TMM scaling normalization method (Robinson and Oshlack, 2010). Normalized gene expression values were used for differential gene expression calculation between dormancy and exponential growth conditions with moderated t-statistics and Benjamini–Hochberg's method to control for false discovery rate (FDR). Genes with ≥ 1.5-fold change in expression, with FDR ≤ 0.05 were considered to be statistically significant differentially expressed genes (DEGs).
2.3. Contextualization of Mtb Genome-Scale Metabolic Model and Analysis of Reaction Fluxes
Genome-scale metabolic models (GEM) are large-scale in silico reconstructions of metabolic networks of an organism that describes the association between genes, proteins and reactions. We selected the most updated GEM of Mtb, iEK1011, which combined the previous GEMs and is manually curated for recent literature, for this study (Kavvas et al., 2018). This model contains 1228 metabolic reactions, 998 metabolites and 1011 genes.
Flux balance analysis (FBA) is a constraint-based modeling approach that analyzes the flow of metabolites through a metabolic network, i.e., fluxes of metabolic reactions. The principle and procedure for FBA have been thoroughly described elsewhere (Raman and Chandra, 2009; Orth et al., 2010). To identify the metabolic changes occurring in Mtb when it enters a dormant state, we integrated gene expression data from different growth states into the metabolic model iEK1011, using the algorithm E-Flux (Colijn et al., 2009). E-Flux utilizes continuous gene expression data to set the upper and lower bounds of the reaction fluxes using gene-protein-reaction (GPR) rule from the model. Therefore, a reaction associated with highly expressed genes in a given condition will allow higher flux through it while maximizing the defined objective function. We have used E-Flux (Colijn et al., 2009) to contextualize the iEK1011 model by integrating the corresponding transcriptome data. Next, we have limited the exchange reactions of the respective stress condition to replicate the experimental environment. For example, in hypoxia-induced dormancy model, the oxygen exchange reaction, that allows entry of oxygen into the cell, is limited to reproduce the hypoxia state. We then computed the fluxes through all the reactions for each growth and dormancy condition and time point with FBA using the biomass objective. The reactions with no flux or flux <1E-06 in both exponential and dormancy conditions were removed from further study. A reaction was considered to be significantly perturbed in dormancy if flux fold-change ≥ 1.5, as compared to corresponding exponential phase flux. The analysis was performed using COBRA toolbox in MATLAB R2018b (Becker et al., 2007; Heirendt et al., 2019).
2.4. Updation of Knowledge-Based Mtb Protein-Protein Interaction Network (MtbPPIN)
A high-confidence knowledge-based protein-protein interaction network, MtbPPIN, was updated from the previously constructed in-house Mtb network (Ghosh et al., 2013) using information from databases as well as primary literature. First, all interaction information available in the database STRING (Szklarczyk et al., 2015) for Mtb H37Rv strain was downloaded. Each interaction in the database is associated with a confidence score on a scale of 0 to 1000, where a higher score corresponds to higher confidence interactions. Only the interactions with a confidence score ≥ 800 were retained for MtbPPIN. Next, functional interactions were manually curated from KEGG database (Kanehisa and Goto, 2000; Kanehisa, 2019; Kanehisa et al., 2019) Mtb pathways. Further physical associations were added to the network from the high-throughput screening studies (Wang et al., 2010; Wu et al., 2017). High confident transcriptional regulatory interactions were added to the network from multiple primary studies (Balázsi et al., 2008; Zeng et al., 2012; Galagan et al., 2013; Minch et al., 2015). Only the interactions between protein-coding genes were retained. Directionality information was incorporated into the network wherever available. Interactions representing transcriptional regulation were directed from regulator to target, signaling or metabolic interactions were directed from upstream to downstream of the pathways. Interactions signifying physical binding were considered as bidirectional. Unknown functionality interactions were undirected. After a final round of manual curation, the high-confidence genome-scale network, MtbPPIN consists of 3,980 nodes (proteins) and 53,208 edges (interactions) with 62% directionality. About 96% of the known Mtb H37Rv proteome is included in MtbPPIN. MtbPPIN interactions are provided in Supplementary File 1.
2.5. Response Network Analysis
Response network analysis is an in-house computational pipeline that integrates transcriptome data in protein-protein interaction networks to capture the important perturbations in a cell or organism in a certain condition (Sambarey et al., 2013, 2017; Padiadpu et al., 2016; Bhosle et al., 2020). We generated response networks for each dormancy stage of each condition by weighing the nodes and edges with transcriptome information using the following Equations (1), (2), and (3). The feasibility of the flow of information through the nodes can be calculated as the path cost through those nodes (Equation 4).
Since the weights of the edges are inversely proportional to the node weights, the lower the path cost the higher the flow of information. The paths with the least cost capture the highest perturbations in a given condition. The top 0.001 percentile paths from both the active and repressed response networks were used to obtain the top perturbed response networks of dormancy stages (DormancyTPN). For cases where transcriptome data from multiple timepoints are available for one dormancy stage, a union of the DormancyTPN of the corresponding timepoints were considered. All networks and pathways were visualized using Cytoscape 3.7 (Shannon et al., 2003).
2.6. Structural Studies of Shortlisted Mtb Proteins
For the shortlisted candidate target molecules, we studied their protein sequences, structures and binding sites using well-established bioinformatics methods. The input sequences of all regulated proteins were retrieved from the Mycobrowser in FASTA format (Kapopoulou et al., 2011). The structure template appropriate for query sequence was identified using the popular fold recognition software called MUSTER (Wu and Zhang, 2008). MUSTER is based on an extension of the traditional sequence profile-profile alignment algorithms (PPA) with additional structural features such as prediction of secondary structure elements, solvent accessibility, backbone dependent dihedral angle and hydrophobic scoring matrix. In addition to sequence derived information, used by other programs, new features incorporated by MUSTER aid in deriving structural relationships thereby increasing the sensitivity of template being chosen. The quality of template that is aligned with the query sequence was verified based on the Z-score cutoff scheme as reported in the original MUSTER paper. Z-score is obtained either by dividing raw alignment score (Rscore) by full length alignment (Lfull) or by dividing Rscore by partial length alignment (Lpartial). Z-score value greater than 7.5 indicates a significant alignment of query sequence over template structures. Finally, an all-atom structure reconstruction was built using MODELER, a software for comparative protein structure modeling (Webb and Sali, 2016). The quality and robustness of models were investigated using ERRAT (Colovos and Yeates, 1993).
2.7. Consensus Binding Site Detection Pipeline
Numerous site prediction algorithms are available online each exploiting distinct ways to find binding pockets in the proteins. Consensus identification of sites from multiple methods yields high confidence binding sites essential for this work. Three independent pocket detection methods used for this study were (i) PocketDepth, a geometry-based method (Kalidas and Chandra, 2008), (ii) SiteHound, energy-based (Ghersi and Sanchez, 2009) and (iii) FPocket, based on Voronoi tessellations (Le Guilloux et al., 2009). PocketDepth, an inhouse method, uses the notion of a depth factor to identify putative binding pockets for a given protein structure. It is a geometry-based method which encompasses the protein as a volume of grid cells each of size 1Å. All grid cells are then classified either as internal (if the cell is within 2Å from atom occupied grids) or external (everything else). A depth-first graph traversal search along all six faces of the cube gives a list of connected cells defining the pockets. The second algorithm, SiteHound, which is an energy-based method, predicts a site by finding energetically favorable regions on the protein surface using Molecular Interaction Fields (MIF). Lastly, FPocket relies on Voronoi tessellation by constructing a set of small alpha spheres in proteins that connect four atoms on its boundary and all connected atoms are equidistant to the alpha sphere center. All three methods run on different principles and hence served as independent methods from which only those sites predicted by all three methods were considered as a consensus pocket. The minimum residue overlap of 4 residues was considered for consensus pocket identification and by default pocket definition from FPocket was used for further analysis.
2.8. Multiple Approaches of Associating Targets With Lead Molecules
Three methods were used for associating ligand molecules to the selected targets, based on (i) binding site sub-structures, (ii) whole protein structures level and (iii) protein sequences. The site-based association uses the structural similarity between sites as a basis to transfer function from one protein to another. It broadly comprises the following steps—comparing binding sites against known ligand sites from Protein Data Bank (PDB) (Berman et al., 2000) and transferring ligands, measuring similarity between PDB ligands and drugs in DrugBank (Wishart et al., 2008), and associating drugs to the query protein. On the contrary, the second approach uses whole fold similarity for making a ligand association transfer. For each drug entry available in the DrugBank database, UniProt Ids of protein to which it gets recognized were taken. Following that, the primary sequence data of all drug binding proteins were downloaded from the UniProt database (UniProt Consortium, 2019). A large-scale protein structural modeling exercise was then set up using the MUSTER program. Structural templates suitable for modeling each sequence were taken based on Z-score 7.5. Proteins whose Z-score value is less than 7.5 were not considered further in this work as they do not have a reliable template for predicting their structures. Finally, sequence-based annotation involves identification of putative drug-like molecules based on similarity with sequences in the ChEMBL database (Gaulton et al., 2012). The binding energy of each drug molecule with the target proteins was calculated using AutoDock (Forli et al., 2016).
3. Results
3.1. Identification of Potential Drug Targets for Latent TB With Systems-Level Analysis
With an aim of identifying targetable cellular mechanisms that have increased activity in non-dividing Mtb, we configure a multi-step computational pipeline that performs genome-scale metabolic modeling followed by a contextualized genome-wide protein-protein interaction network analysis (Figure 1). Different steps in the pipeline serve as filters to select only those genes that satisfy the criteria defined at that step. The filters correspond to gene expression variations or alterations in metabolic fluxes in associated reactions, as described below.
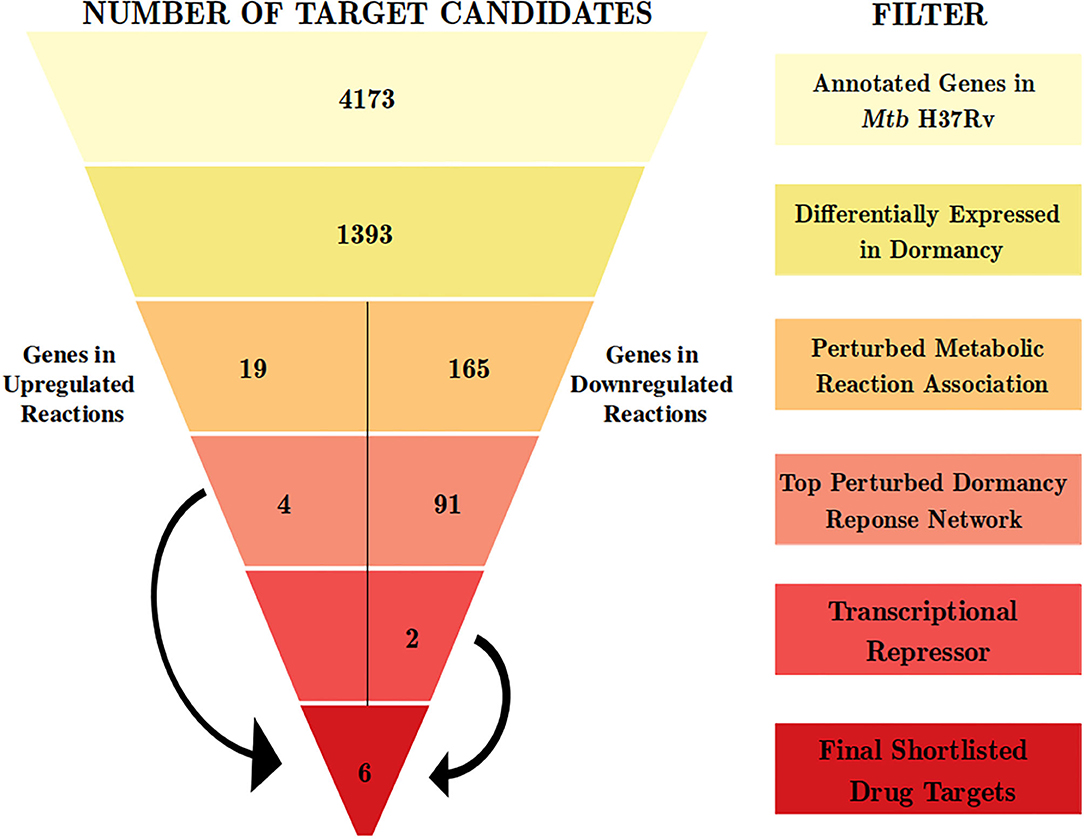
Figure 1. The computational pipeline used for drug target candidate identification for latent tuberculosis. The pipeline involved stepwise filters based on gene expression, metabolic modeling, and protein-protein interaction network analysis to shortlist proteins crucial for Mtb dormancy. Six proteins were shortlisted as potential drug target candidates from this pipeline.
3.1.1. Genome-Wide Profiling of Alterations in Mtb During Dormancy in Different Models Identify Most Likely Perturbations Inside Latent Host Granuloma
First, we obtain an unbiased view of the alterations in the dormant Mtb cells as compared to their actively replicating counterparts. We selected publicly available transcriptome data from various in vitro dormancy models of Mtb that employed different kinds of stress factors, as described in the section 2, to induce a non-replicating stage in the bacterial culture (Voskuil et al., 2004; Deb et al., 2009; Ignatov et al., 2015; Kurthkoti et al., 2017). We selected one dataset per condition, which included hypoxia, multiple stress, potassium deficiency and iron deficiency, to represent the different kinds of stresses faced by Mtb in the granuloma environment. In each of the dormancy inducing conditions, dormant cells showed a significant number of genes to be differentially regulated (FC≥ ±1.5, FDR ≤ 0.05) when compared to cells growing in exponential phase (Figure 2A). In the datasets where different stages of dormancy were classified (GSE8786 and GSE66408), the transcriptome showed a gradual change in the perturbation from early to later time points. Although a large number of genes were perturbed in each condition, the overall change in the transcriptome varied between different conditions as could be observed from the clustering pattern that clearly separates the dormancy conditions from each other (Figure 2A). Each of the stress conditions individually caused an up or downregulation in a large portion of the Mtb transcriptome, but only 350 genes were differentially regulated in all four of the conditions and 1393 genes were DEGs in any three of the conditions studied (Figure 2B). Interestingly, the commonly downregulated genes are enriched in protein synthesis, metabolism and growth, indicative of a non-replicative state whereas the commonly upregulated genes were enriched in different biological processes such as “response to host immune response,” “response to oxidative stress,” and “response to copper ion” which are indicative of responses similar to those observed in dormant Mtb surviving in latent TB granuloma (Figures 2C,D). This shows that although individual stress-inducing conditions trigger responses in Mtb that are mostly specific to that particular stress, the transcriptome perturbation that occurs in multiple conditions does indeed replicate the state of the pathogen inside latent host granuloma, where it stays metabolically dormant as a survival strategy against the host immune response.
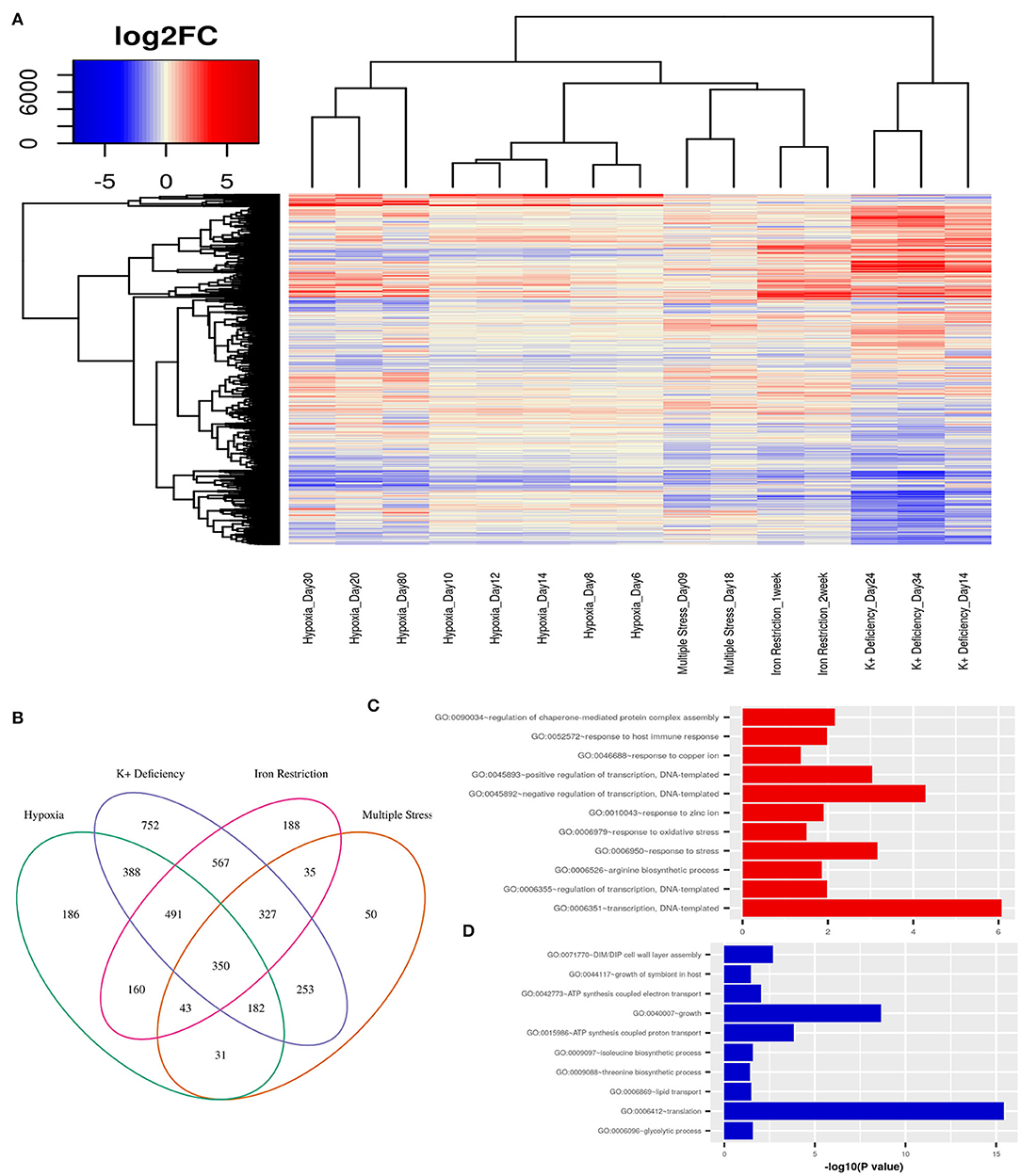
Figure 2. (A) Variations in gene expression profiles of Mtb in different in-vitro dormancy models with respect to actively replicating Mtb. Gene expression alterations are depicted as log2(fold changes) over an exponential growth condition taken as the reference in each dataset. Red signifies upregulation and blue signifies downregulation of gene expression. Each column in the heatmap shows the gene expression in the time point and model indicated in the figure. (B) A Venn diagram showing the overlap between DEGs (fold change ≥ ±1.5, FDR ≤ 0.05) between different dormancy models w.r.t. exponential growth. 350 genes were differentially regulated in all four dormancy models at some time point and 1393 genes were differentially regulated in at least 3 models. (C) GO: biological processes overrepresented in the commonly upregulated genes amongst dormancy conditions and (D) GO: biological processes overrepresented amongst the genes commonly downreguated across multiple dormancy conditions as analyzed using the enrichment tool in DAVID database (Huang et al., 2009).
We used the transcriptome analysis of dormancy conditions not only to ascertain the applicability of in vitro dormancy models in studying latent TB in the host, but also as our first step of the filter in the drug target identification pipeline for dormant Mtb. Mtb H37Rv has 3994 known proteins annotated in Uniprot (UniProt Consortium, 2019) and 4,173 genes annotated in Mycobrowser (Kapopoulou et al., 2011). The first filter applied in the drug target identification pipeline was that of a gene being a DEG in 3 or more of the in vitro dormancy conditions studied. This resulted in 1,393 genes from Mtb passing the first filter (Figure 1 and Supplementary File 2).
3.1.2. Contextualized Genome-Scale Metabolic Modeling Elucidates Most Perturbed Metabolic Reactions in Dormant Mtb
Mtb goes through extensive metabolic remodeling to achieve the non-replicative yet viable state during latency (Boshoff and Barry, 2005; Schubert et al., 2015; Baker and Abramovitch, 2018). We utilized the most updated Genome Scale Metabolic Model of Mtb, iEK1011, integrated with gene expression data, to generate a contextualized metabolic model for each dormancy condition (as described in section 2) (Colijn et al., 2009; Kavvas et al., 2018). A consequent FBA on the exponential growth as well as dormant Mtb specific iEK1011 provided the fluxes through the reactions in the respective condition. Contextualization of the GEM with transcriptome data showed a 50-100% reduction in the generalized biomass production reaction in the dormancy conditions from the exponential growth phase (Figure 3A), showing the capability of the method to replicate the dormancy phenotype. Fluxes through 421 reactions were found to be altered in three or the more dormancy conditions (Supplementary Files 3, 4). Of these, 407 reactions were downregulated in all the four dormancy conditions compared to exponential growth phase whereas 14 of the reactions showed significant upregulation across dormancy condition (Figure 3B and Supplementary Figures 1A–C). The downregulated reactions belonged to different amino acid synthesis pathways, cell wall and mycolic acid biosynthesis, fatty acid metabolism, aerobic respiration etc. (Figure 3C and Supplementary File 5). Among the reactions with significant upregulation across dormancy models were key reactions in the fatty acid metabolism, glyoxylate cycle and glycine cleavage system (Figure 3C and Table 2).
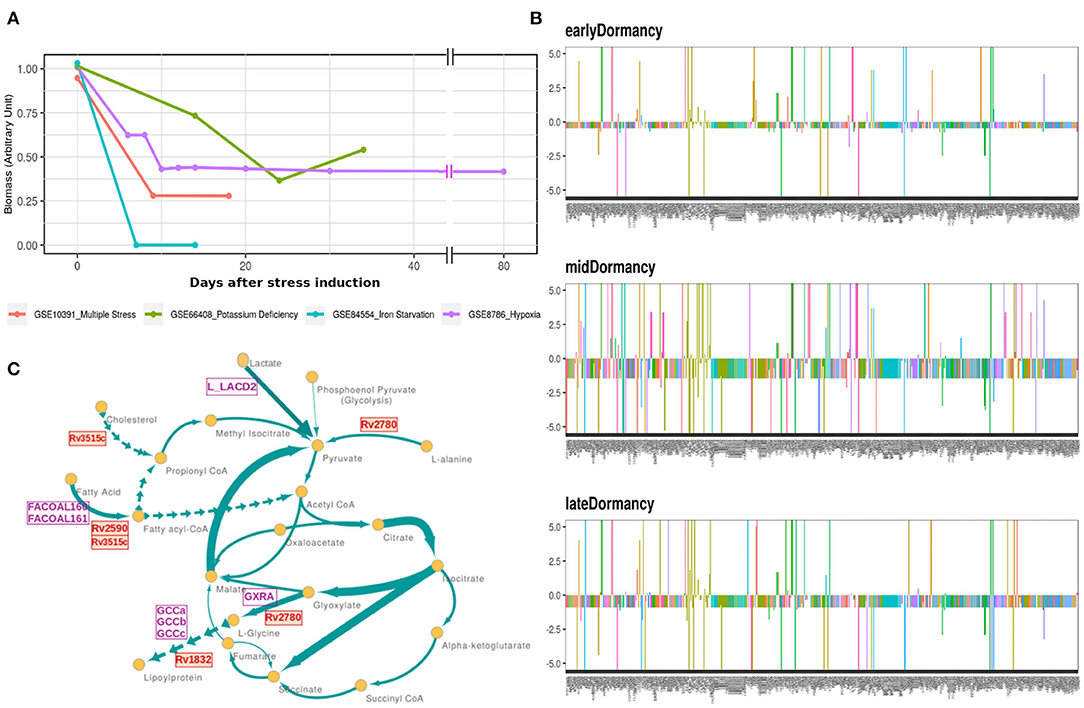
Figure 3. (A) Optimal flux through the hypothetical biomass reaction from iEK1011 in different timepoints of all the models showing a significant reduction in biomass generation in dormancy compared to exponential growth. (B) Flux fold change of all non-zero reactions in Potassium deficiency model of dormancy at 3 different stages in comparison to the exponential growth phase. X axis in each contains the reactions with non-zero fluxes and Y axis shows the fold change value. Most of the reactions are downregulated during dormancy. (C) A representative subgraph of metabolic pathways focused on the TCA-glyoxylate cycle of central carbon metabolism in dormant state Mtb in multiple stress induced model. The thickness of the edges is directly proportional to flux through the reactions. Yellow circles signify metabolites. Purple squares indicate reactions from the 14 commonly upregulated reactions across dormancy models. Red squares highlight the genes eventually identified as drug target candidate further down the pipeline.
From this analysis, the differentially regulated reactions were selected for further steps in the target identification pipeline. The up and downregulated reactions without GPR information were filtered out, leaving 12 and 356 reactions into the pipeline, respectively. The 12 upregulated reactions were associated with 45 genes, whereas the 356 downregulated reactions were associated with 390 genes. Of these, 19 and 165 genes, respectively, overlapped with the list of 1393 DEGs from the previous step (Figure 1 and Supplementary File 2). These DEGs are involved in perturbed metabolic reactions in the dormant state of Mtb and were taken forward to the next step of the pipeline.
3.1.3. Response Network Analysis Identifies Top-Ranked Hubs Associated With Perturbations in Dormant Mtb
Response network analysis has been proven to be an elegant tool in strategic identification of drug targets in pathogens due to its capacity of delineating the most perturbed cellular processes and cross-talk in a condition of interest Padiadpu et al. (2016); Bhosle et al. (2020). Here, we have constructed a knowledge-based functional interactome MtbPPIN and generated dormancy response networks (DormancyTPN) for each of the in vitro models and stages (see section 2). The DormancyTPN for a condition captured the top active and repressed interactions based on a stringent path-cost based filter. This not only included metabolic enzymes but also other important proteins, e.g., transcriptional regulators, structural components etc. In our previous step of the target identification pipeline, 19 upregulated and 165 downregulated reaction-associated genes were shortlisted. Proteins with higher expression or activity level are more viable drug targets since inhibiting those with a chemical or biological compound is more practicable than increasing the activity of any protein with external intervention. Thus, for the next stage of the pipeline, we treated the up and downregulated candidates as two separate groups (Figure 1). First, we shortlisted the proteins captured in the DormancyTPNs of 3 or more in vitro dormancy models. 4 of the 19 upregulated candidates were found to pass the response network filter. These were Rv2590, Rv3515c, Rv1832, and Rv2780 (Table 3). These four genes were selected as final shortlisted targets for dormant Mtb (Figure 1 and Table 3). Since the DormancyTPNs also provided crucial information on transcriptional regulation of different genes, we aimed to find repressors of the genes associated with downregulated reactions from the previous step. Repressors of such genes could be expected to be upregulated themselves, thereby acting as potential drug targets. With this aim, we first filtered those of the 165 genes that were captured in DormancyTPNs of 3 or more in vitro dormancy models, retaining 91. Next, we obtained all known and predicted transcriptional regulators in Mtb from the Mycobrower and other resources (Balázsi et al., 2008; Zeng et al., 2012; Galagan et al., 2013; Minch et al., 2015). Edges capturing regulatory interactions were selected from the DormancyTPNs. These interactions were further pruned to retain only the edges occurring in at least 50% of the dormancy conditions, where any of the 91 genes were the targets of the regulators. This exercise provided a list of 25 transcriptional regulators. Two of these regulators were found to be upregulated themselves and connected to genes significantly downregulated in all the dormancy conditions, confirming transcriptional repression (Figure 4). These two transcriptional repressors of metabolic genes, Rv1994c and Rv0324, were added to the final list of shortlisted candidate targets (Figure 1 and Table 3).
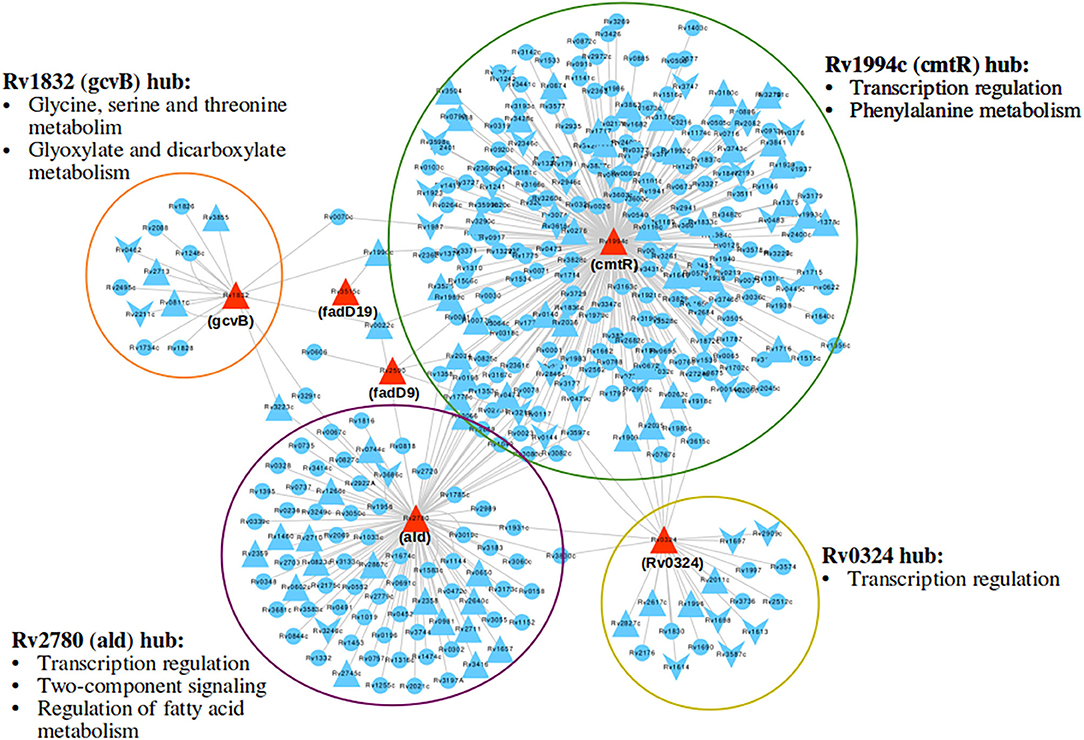
Figure 4. A subnetwork of union dormancy response network for the 6 shortlisted drug target candidates and their first neighbors. The target candidates are colored in red. Upward and downward triangular nodes signify up and downregulation of gene expression, respectively. cmtR (Rv1994c) is the biggest hub in the network, followed by ald (Rv2780). Most over-represented pathways among the first neighbors of each of the hubs are mentioned.
The list of 91 downregulated genes associated with repressed metabolic reactions also provide us with insights into potentially poor targets for latent TB. A number of drug target candidates in actively growing Mtb have been reported in literature (Jamshidi and Palsson, 2007; Raman et al., 2008; Kaur et al., 2017). From the present analysis, we observe that 27 of those predicted active TB targets are not likely to be effective against dormant TB (Supplementary File 6). We tested if known targets for clinically used first and second-line drugs featured in our lists and found that the model predicts most of the currently used anti-TB drugs to show low efficacy against dormant bacilli (Supplementary Table 1).
3.1.4. Supporting Evidence of Functional Importance of Shortlisted Drug Target Candidates From Experimentally Derived Literature
Multiple studies have used omics-based and individual molecular techniques to study genes and proteins playing crucial roles in Mtb in different in vivo infection models in cells or animals, and in vitro conditions such as stress-inducing conditions or dormancy. We used this vastly available knowledge as a fold of validation for the potential of success of our shortlisted drug candidates against latent tuberculosis. Five of our six shortlisted targets [fadD9 (Rv2590), fadD19 (Rv3515c), ald (Rv2780), cmtR (Rv1994c), Rv0324] have been reported to be transcriptionally upregulated in Mtb once they infect macrophages and encounter the intra-phagosomal stress (Schnappinger et al., 2003). Another proteomics quantification study showed that fadD9 (Rv2590), gcvB (Rv1832), and ald (Rv2780) levels significantly increase in Mtb in in vitro hypoxia-induced dormancy model (Schubert et al., 2015). Upregulation of genes involved in lipid degradation, such as fadD9 (Rv2590) and fadD19 (Rv3515c), in a high lipid environment or hypoxia is intriguing because these conditions closely simulate the environment inside a granuloma (Karakousis et al., 2004; Salina et al., 2009). gcvB (Rv1832) and ald (Rv2780), encoding a glycine cleavage complex component and L-alanine dehydrogenase, respectively, have also been observed to be present in high amount in hypoxia-induced dormancy models at transcript and protein levels (Wayne and Lin, 1982; Betts et al., 2002; Schnappinger et al., 2003; Rustad et al., 2008). Glyoxylate shunt and the associated enzymes are critical to non-replicating Mtb inside host cells and its survival in a nutrient-limited condition (McKinney et al., 2000; Puckett et al., 2017). Fatty acid degradation can be directly linked to increased flux through glyoxylate shunt as an alternative source of energy. Increased fatty acid oxidation also increases cellular demand for NAD+ since it is utilized in the process. Higher activity of L-alanine dehydrogenase (ald/Rv2780), can help the bacterial cell to meet this demand of NAD+ in the reversible pyruvate deamination reaction (Schnappinger et al., 2003). It may also support the maintenance of NAD pool in dormant Mtb in anaerobic condition when terminal electron acceptor oxygen becomes limiting (Starck et al., 2004; Gopinath et al., 2015). ald (Rv2780) has been suggested as a druggable target for dormant Mtb in previous in silico analysis as well (Murphy and Brown, 2007; Defelipe et al., 2016). The two transcriptional regulators, cmtR (Rv1994c) and Rv0324, identified as drug target candidates, have also been implicated to be crucial in various dormancy inducing models. cmtR (Rv1994c) is reported to be strongly induced by copper-mediated toxicity in replicating as well as dormant mycobacteria (Salina et al., 2018), whereas Rv0324 plays a major role in Mtb enduring hypoxic response and in the tolerance against Bedaquiline (Peterson et al., 2016, 2020). Overall, our shortlisted target candidates have been linked to Mtb dormancy is various models of dormancy induction and it provides additional confidence in our prediction of their potential in treating latent tuberculosis.
3.1.5. Pruning the Target Shortlist as Candidates for Lead Identification
With our systems biology pipeline we have identified 6 drug target candidates for latent tuberculosis treatment. Further, we pruned the list to 3 targets most suitable for lead identification with structural modeling and chemoinformatics analysis. cmtR (Rv1994c) and ald (Rv2780) were linked to the highest number of DEGs (103 and 39, respectively) amongst the 6 shortlisted targets in the DormancyTPN subnetwork (Figure 4). As mentioned in the previous section, ald (Rv2780) is reported to be important in dormancy (Wayne and Lin, 1982; Betts et al., 2002; Schnappinger et al., 2003; Rustad et al., 2008). On the other hand, although cmtR (Rv1994c) has been mostly reported in the context of metal toxicity, it is upregulated in all the dormancy conditions studied and is highly connected in the top perturbed network. Also, of the 6 shortlisted targets, experimentally solved structures are available only for cmtR (Rv1994c) and ald (Rv2780) (Banci et al., 2007; Tripathi and Ramachandran, 2008). The third target candidate fadD19 (Rv3515c) was selected based on functional relevance. Its function is well-elucidated, unlike some of the target candidates in our list. It catalyzes the addition of CoA group to long-chain fatty acids, which can get transferred to the polyketide synthases (Trivedi et al., 2004). It is also reported to catalyze thioesterification of C8 alkanoate side chain of cholestenoate, which is an intermediate of cholesterol degradation (Casabon et al., 2014) and is essential for cholesterol degradation in Mtb (Griffin et al., 2011). Accumulation of lipids, especially cholesterol, inside host granuloma and its utilization by Mtb plays a critical role in the survival and dormancy of the bacteria (VanderVen et al., 2015). All the three selected targets were found to have no close homologue in the human proteome. ald (Rv2780) and fadD19 (Rv3515c) were suggested as druggable targets in the tuberculosis drugome (Kinnings et al., 2010). In summary, cmtR (Rv1994c), ald (Rv2780), and fadD19 (Rv3515c) were selected as the most relevant targets to pursue in the next phase of our study.
3.2. Associating Potential Lead Molecules With Drug Target Candidates
We aimed to identify drug molecules that can be potentially repurposed for latent TB or would provide clues for designing new ligands for the selected targets. We utilized a combined structural modeling and chemoinformatics approach for this purpose as schematically represented in Figure 5.
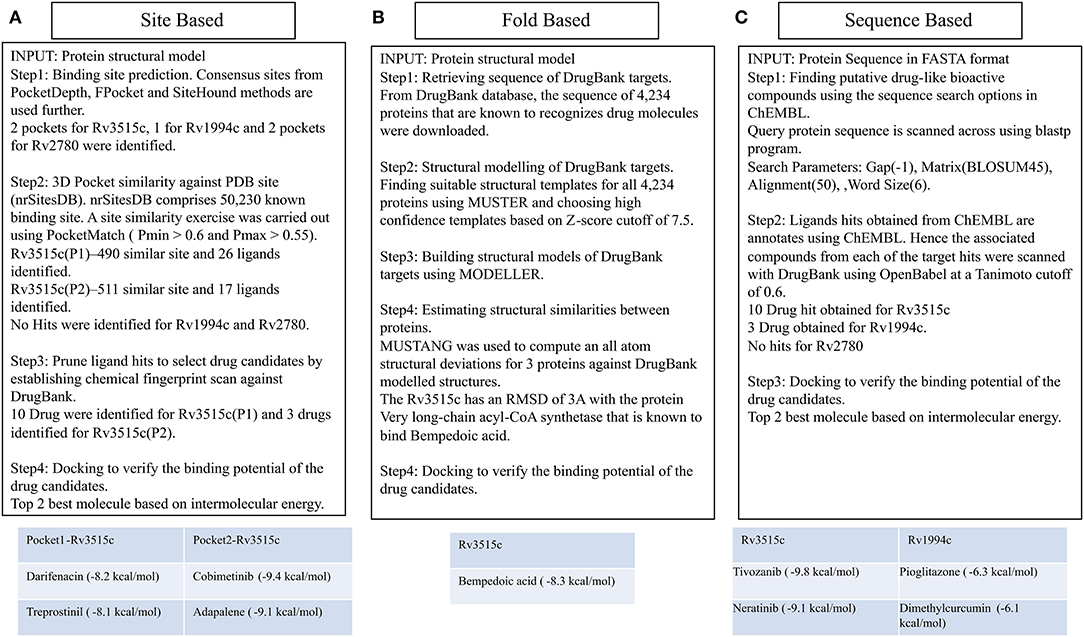
Figure 5. Different ways of associating targets with DrugBank drugs. The site-based association uses binding site similarity as a metric for assigning function to a protein. Consensus pocket prediction exercise yields two pockets for the protein fadD19 (Rv3515c), 1 pocket for cmtR (Rv1994c), and two pockets for ald (Rv2780). Site matching exercise on PDB site library gave 43 ligands that could bind with fadD19. Ligand hits were then pruned by establishing chemical fingerprints on DrugBank molecules. Cobimetinib and darifenacin came out as the best repurposable drug candidate for fadD19. No hits were identified for ald and cmtR. Fold based annotation involves mapping drugs to the targets from the known drug binding proteins. The 3D structure of 4,234 proteins was built using MUSTER and MODELER, of which one hit, very long-chain acyl-CoA synthetase, binding to bempedoic acid share a fold level similarity with fadD19. No hits were identified for ald and cmtR. Finally, sequence derived analysis uses the sequence search option in ChEMBL to identify bioactive compounds. 10 drugs were identified for fadD19, 3 for cmtR. No hit was obtained for ald.
3.2.1. Structural Characterization of Shortlisted Drug Target Candidates
Three proteins (fadD19, ald, and cmtR) that were identified as target candidates in the steps described earlier were analyzed with a goal of identifying possible lead candidates. Structural insights from these proteins were derived based on available in silico structure prediction methods and high-resolution templates available from PDB. MUSTER, a threading algorithm, was used to find the optimal template based on query coverage with the target structure and the Z-score (Wu and Zhang, 2008). With a Z-score threshold greater than 7.5, all three proteins were found to have high confidence templates, which were then modeled using Modeler software (Webb and Sali, 2016). cmtR (Rv1994c) is a winged helical DNA binding transcriptional repressor of 118 residues which senses lead and cadmium. CmtR exists both as monomer and dimer with Cys-102 from one subunit and Cys57, Cys-61 from another subunit forming cadmium binding site. The far UV CD spectra reveal the major portion of this protein is made of α helical segments (Banci et al., 2007).
ald (Rv2780) is an L-Alanine dehydrogenase catalyzing the conversion of ammonia and pyruvate to L-alanine. It constitutes two domains, substrate binding and NAD binding connected by two alpha helices (Jackson et al., 2016). Both, cmtR (Rv1994c) and ald (Rv2780) had a crystal structure already deposited in PDB and hence the same protein was used for structural modeling (Banci et al., 2007; Tripathi and Ramachandran, 2008). For fadD19 (Rv3515c), structural information was not available. Nevertheless, all three proteins have good query coverage and hence considered them for further analysis. Supplementary Table 2 shows the result of structural modeling exercise along with the template taken from MUSTER fold library and the extent of structural deviation in Å.
3.2.2. Characterizing the Drug Binding Nature of Target Candidates
Using the modeled structures as input, the drug binding capabilities of each of them against DrugBank molecules were studied comprehensively via three independent approaches: (i) Site-level study which involves binding site prediction, site similarity against known PDB ligand binding site and ligand similarity between PDB ligands and DrugBank molecules, (ii) Measuring structural similarity against known DrugBank targets by doing a pairwise structural alignment of six proteins onto known drug binding proteins and (iii) By measuring the sequence similarity of the query sequence with known DrugBank target sequences.
(i) Binding site prediction: Consensus binding site prediction yields a total of 5 high confidence pockets for three proteins that can recognize small molecules (Figure 6). The nature and type of ligand binding to individual sites could be analyzed based on pre-existing resources. PDB currently holds unique 50,230 structural complexes excluding buffer ligands, thus serving as a knowledge base of protein-ligand interaction information (Rose et al., 2017). A fast site comparison study was used to assign different PDB ligands to consensus pockets using the in-house PocketMatch algorithm (Yeturu and Chandra, 2008). All 5 high confidence pockets were scanned against 50,230 known PDB binding sites in an all-vs-all manner. The output of PocketMatch results was pruned based on two scoring schemes, Pmax (to encompass global similarities between sites) and Pmin (to capture local similarities between sites). From our previous study, it was shown that Pmax > 0.5 and Pmin > 0.6 reflect meaningful similarities between a given pair of sites, hence the same cutoff was employed here (Anand et al., 2012). The binding sites of all three proteins have similarities with the sites of proteins recognizing natural ligands. For example sites of ald (Rv2780) were predominantly mapped to NAD and FAD liganded sites. Associations made with natural ligand sites were not considered as we were only interested in finding candidate drugs. To accomplish that, we used open-babel and compared the 149 ligand hits obtained from PocketMatch against ligands deposited in DrugBank. DrugBank stores ligand molecules based on one of these groups, Approved, Nutraceutical and Investigational and we screened only those in the Approved category as most of the commercial drugs belong to this class. Of 11,414 entries being deposited in DrugBank, 10 drugs were identified as hits for fadD19 (Rv3515c) at a given Tanimoto cutoff of 0.70 (Table 4 and Supplementary Figure 4). No hits were obtained for cmtR (Rv1994c) and ald (Rv2780). For fadD19 (Rv3515c), the binding energy of each of the drugs was calculated using Autodock. Full results describing the name of each drug along with the predicted binding affinity with the respective target is provided in Supplementary File 7.
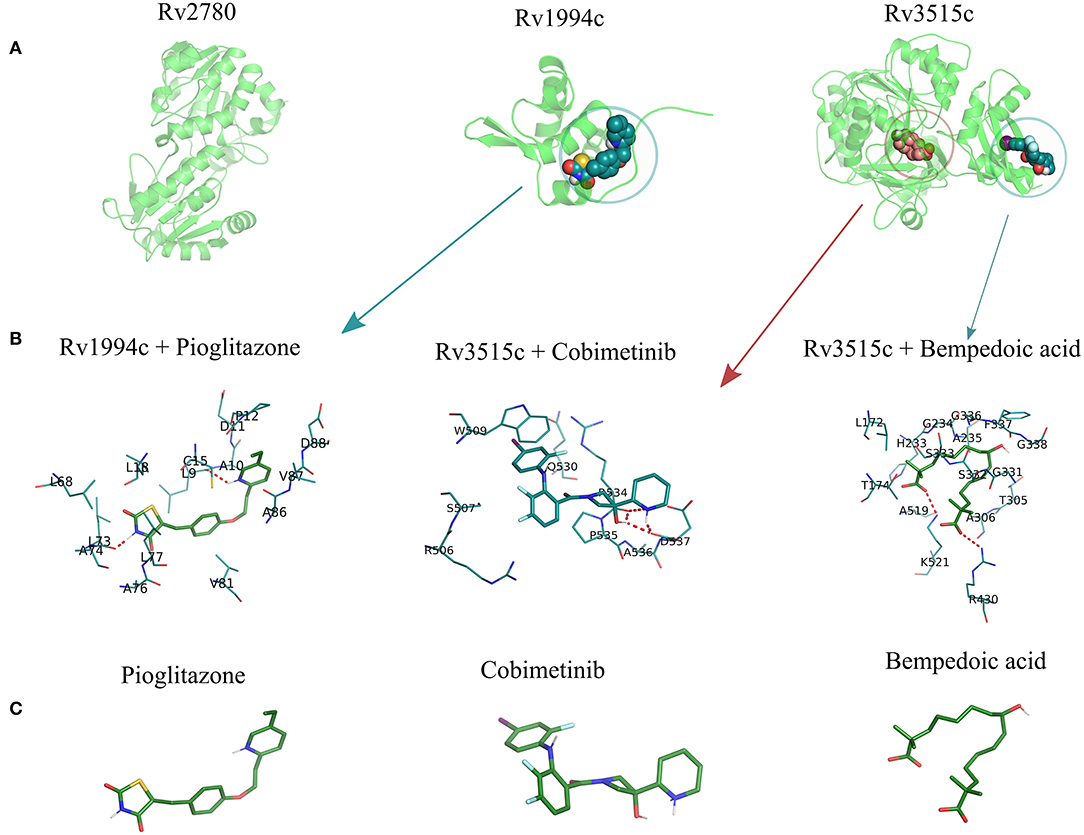
Figure 6. The structural model of the three shortlisted target candidates predicted along with the pocket identified from the consensus approach. (A) The structures predicted for each of the 3 proteins, i.e., ald (Rv2780), cmtR (Rv1994c), and fadD19 (Rv3515c), are represented in cartoon format. (B) The docking pose of the best drugs for the targets. fadD19 (Rv3515c) is docked against Bempedoic acid and Cobimetinib, while cmtR (Rv1994c) is docked with Pioglitazone. No drug hits are identified for ald (Rv2780). (C) Three-dimensional structures of the 3 docked ligands.
(ii) Prediction of drug binding from structures: Next we mapped drugs to our identified targets based on the existing structural data available in databases such as DrugBank. For all drug molecules available in DrugBank, the UniProt IDs of the proteins that were known to recognize them were retrieved. The sequence information of these proteins was then fetched from the UniProt website amounting to a total of 4,555 sequences. A structural library for DrugBank targets was created for all 4,555 proteins using the MUSTER programme. High confidence templates were taken based on reported Z-score cutoff of 7.5 resulting in a total of 4,234 proteins that are suitable for structural modeling. The final structure was built using MODELER. To transfer functional annotation from one structure to another, we employed a protein structure alignment approach to computing the extent of deviations between structures using a well-known structure alignment software called MUSTANG (Konagurthu et al., 2006). All 3 proteins were aligned onto 4,234 structures in a pairwise manner resulting in 12,702 combinations, out of which only 1 pair aligned with an RMSD less than 3Å. The protein Very long-chain acyl-CoA synthetase (UniProt ID: O14975) was found to be structurally similar to fadD19 (Rv3515c) and was known to bind Bempedoic acid, a drug for the treatment of hypercholesterolemia. As both fadD19 (Rv3515c) and O14975 possess global fold level similarity, we believe Bempedoic acid can be used as a repurposable drug candidate.
(iii) Deriving drug binding from sequence analysis: We explored associating drugs to our targets from proteins that are known to bind drug molecules. Sequence-based search option in ChEMBL was used to get the list of related proteins along with their ChEMBL compounds in 2D sdf format. Conversion from 2D to 3D coordinates was made using Open Babel software (O'Boyle et al., 2011). Many of the compounds available in ChEMBL are not annotated and are completely new, but we were only interested in drugs that were approved. To do this, the structure of all ChEMBL molecules was scanned against approved drugs in DrugBank. At a Tanimoto cutoff of 0.70, we obtained 14 drug molecules for fadD19 (Rv3515c) and 3 for cmtR (Rv1994c) (Table 4, Supplementary Figure 4, and Supplementary File 7). No drug hits were obtained for ald (Rv2780).
4. Discussion
Large-scale systems-level modeling approaches are becoming increasingly popular to understand biological systems due to its capacity of providing insights into their complex molecular networks, pathways and crosstalk. High-throughput methods are of particular importance in the field of drug discovery due to the urgent need for new and improved drugs for most of the diseases. Although chemical screening methods have been successfully utilized for drug identification for many diseases (Ananthan et al., 2009; Siqueira-Neto et al., 2010; Debnath et al., 2012), the large scale experiments incur a high cost and time, and in many cases, the mechanisms of action of the drugs remain a black-box. Systems-level modeling provides an efficient top-down approach for predicting potential drug targets in biological systems based on functionality and to associate lead molecules with them.
Latent TB is a widespread disease and is difficult to tackle due to our limited understanding of the condition. Majority of the anti-TB drugs target cellular processes critical to replicating pathogens (Campbell et al., 2001; Timmins and Deretic, 2006; Nagabushan and Roopadevi, 2014). Two of the most well-established phenomena associated with latent tuberculosis is the non-replicative state of the bacteria and its significant metabolic rewiring (McKinney et al., 2000; Betts et al., 2002; Voskuil et al., 2004; Gengenbacher et al., 2010). Therefore an understanding of the differences in the metabolic processes and their regulations between actively growing Mtb and dormant ones holds the potential of providing critical clues for tackling latent TB. In this study, we have utilized a genome-scale metabolic model of Mtb (iEK1011) integrated with transcriptome information to identify the key differences between the replicating and dormant pathogen. One major limitation in studying latent TB remains the lack of a model system that correctly simulates the conditions of the dormant bacteria inside human granuloma. Different in vitro models have been established to achieve the dormant state of Mtb but each of these has been limited in the use of any one or few of the stress conditions that the bacteria face inside the host, thereby mimicking the in vivo condition only partially. In order to overcome this limitation, we have considered different models of dormancy and identified the metabolic alterations that occur across different stress conditions. Such alterations are most likely to be occurring in dormant Mtb inside the host and be critical for their survival, making the associated enzymes interesting targets for drug discovery. We further filtered the targets using transcriptome integrated genome-wide protein-protein interaction network (response network) analysis. This step provided a broader perspective into the perturbations occurring in dormant Mtb as it considers not only the metabolic network but all the other molecular and functional interactions as well. The metabolic enzymes captured in the top-perturbed response networks for dormancy along with being associated with reactions with the highest flux changes across dormancy conditions could be considered as high confidence drug targets. fadD9 (Rv2590), fadD19 (Rv3515c), gcvB (Rv1832), and ald (Rv2780) were shortlisted from this pipeline as drug target candidates. Since overexpressed proteins are easier to inhibit with a drug than targeting any gene or protein that is downregulated, we intended to focus on upregulated proteins as drug target candidates. Predictably, most of the metabolic reactions were downregulated in dormant Mtb. This led us to use the regulatory interactions from dormancy response networks to find transcriptional regulators that might be associated with such downregulated metabolic reactions. We found two transcriptional regulators, cmtR (Rv1994c) and Rv0324, to be regulating multiple metabolic reactions while being upregulated themselves. All of these 6 target candidates were closely connected with each other in the response network, suggesting a functional interaction between them. The metabolic enzymes in the shortlist are involved in lipid metabolism and the glyoxylate cycle. These processes are critical in the maintenance of dormancy in Mtb and survival inside nutrient-limited host granuloma environment (McKinney et al., 2000; VanderVen et al., 2015; Puckett et al., 2017), showing that the functional relevance of the identified drug target candidates.
In the next step we further pruned the target shortlist to 3 candidates, fadD19 (Rv3515c), cmtR (Rv1994c), and ald (Rv2780), based on their biological significance and network properties, for lead association. The structures of the target proteins were obtained from experimentally solved structures or were generated with homology modeling. To identify potential lead molecule associations three independent approaches were taken. In the first approach, we predicted the binding sites for each of the target candidates using a consensus between three orthogonal methods, PocketDepth, SiteHound, and FPocket (Kalidas and Chandra, 2008; Ghersi and Sanchez, 2009; Le Guilloux et al., 2009). Each of these methods has its own strengths and limitations and employs a different strategy for binding site identification and deploying a consensus approach provides higher confidence in the predicted binding sites. Multiple resources were then scanned to find ligands and drugs that can bind to the high-confidence binding sites in the target proteins. In the second approach, structural information of known drug-target interactions from DrugBank was used to associate drugs with our three candidate proteins by finding similarity between structural folds. In the third approach, lead-target associations were obtained using a sequence level similarity analysis in the ChEMBL database. The utilization of three different approaches enables us to derive the strengths of each of the methods and explore the available resources to the fullest. We have successfully associated multiple known natural ligands such as NAD and FAD as well as 28 drug molecules with our shortlisted targets. These associated drug leads are already used as approved drugs for other disease and could therefore be readily explored for the possibility of repurposing.
In summary, this study provides a ready shortlist of potential drug targets for latent tuberculosis to be further studied. Multiple known drug molecules have been associated with the shortlisted targets which can either be explored as possible repurposable drugs or at the very least will provide significant clues for chemically designing new drug molecules for the target proteins.
5. Equations
For active response network,
For repressed response network,
Where NW means node weight, FC stands for fold change in gene expression and ‘i' indicates the gene.
The edge weight (EW) between nodes ‘i' and ‘j' is calculated based on the node weights as,
Data Availability Statement
Publicly available datasets were analyzed in this study. This data can be found here: GEO repository (https://www.ncbi.nlm.nih.gov/geo/) under IDs GSE8786, GSE10391, GSE66408, and GSE84554.
Author Contributions
NC conceptualized, designed, and supervised the study. UB performed the data curation and analysis and interpretation of target identification. SS performed the data curation and analysis and interpretation of lead identification. AS provided critical insights and discussions. UB and SS wrote the first draft of the manuscript. NC revised the manuscript. All authors read and approved the submitted version.
Funding
Authors thank DBT-IISc Partnership Program phase II at IISc, Government of India for providing support for this study.
Conflict of Interest
NC is associated with qBiome Research Pvt Ltd and HealthSeq Precision Medicine Pvt Ltd, it has no role in this manuscript.
The remaining authors declare that the research was conducted in the absence of any commercial or financial relationships that could be construed as a potential conflict of interest.
Acknowledgments
UB is grateful to Department of Biotechnology, India for supporting her with Senior Research Fellowship (DBT-SRF).
Supplementary Material
The Supplementary Material for this article can be found online at: https://www.frontiersin.org/articles/10.3389/fchem.2020.593497/full#supplementary-material
Abbreviations
FAD, Flavin Adenine Dinucleotide; FBA, Flux Balance Analysis; GEM, Genome-scale Metabolic Model; GEO, Gene Expression Omnibus; GPR, Gene Protein Rule; MDR, Multidrug Resistant; NAD, Nicotinamide Adenine Dinucleotide; PDB, Protein Data Bank; TB, Tuberculosis; XDR, Extremely Drug Resistant.
References
Ai, J.-W., Ruan, Q.-L., Liu, Q.-H., and Zhang, W.-H. (2016). Updates on the risk factors for latent tuberculosis reactivation and their managements. Emerg. Microbes Infect. 5:e10. doi: 10.1038/emi.2016.10
Anand, P., Yeturu, K., and Chandra, N. (2012). PocketAnnotate: towards site-based function annotation. Nucleic Acids Res. 40, W400–W408. doi: 10.1093/nar/gks421
Ananthan, S., Faaleolea, E. R., Goldman, R. C., Hobrath, J. V., Kwong, C. D., Laughon, B. E., et al. (2009). High-throughput screening for inhibitors of Mycobacterium tuberculosis H37Rv. Tuberculosis 89, 334–353. doi: 10.1016/j.tube.2009.05.008
Baker, J. J., and Abramovitch, R. B. (2018). Genetic and metabolic regulation of Mycobacterium tuberculosis acid growth arrest. Sci. Rep. 8:4168. doi: 10.1038/s41598-018-22343-4
Balazsi, G., Heath, A. P., Shi, L., and Gennaro, M. L. (2008). The temporal response of the Mycobacterium tuberculosis gene regulatory network during growth arrest. Mol. Syst. Biol. 4:225. doi: 10.1038/msb.2008.63
Banci, L., Bertini, I., Cantini, F., Ciofi-Baffoni, S., Cavet, J. S., Dennison, C., et al. (2007). NMR structural analysis of cadmium sensing by Winged Helix Repressor CmtR. J. Biol. Chem. 282, 30181–30188. doi: 10.1074/jbc.M701119200
Barberis, I., Bragazzi, N., Galluzzo, L., and Martini, M. (2017). The history of tuberculosis: from the first historical records to the isolation of Koch's bacillus. J. Prevent. Med. Hyg. 58:E9–E12. doi: 10.15167/2421-4248/jpmh2017.58.1.728
Becker, S. A., Feist, A. M., Mo, M. L., Hannum, G., Palsson, B., and Herrgard, M. J. (2007). Quantitative prediction of cellular metabolism with constraint-based models: the COBRA Toolbox. Nat. Protoc. 2, 727–738. doi: 10.1038/nprot.2007.99
Berman, H. M., Westbrook, J., Feng, Z., Gilliland, G., Bhat, T. N., Weissig, H., et al. (2000). The protein data bank. Nucleic Acids Res. 28, 235–242. doi: 10.1093/nar/28.1.235
Betts, J. C., Lukey, P. T., Robb, L. C., McAdam, R. A., and Duncan, K. (2002). Evaluation of a nutrient starvation model of Mycobacterium tuberculosis persistence by gene and protein expression profiling. Mol. Microbiol. 43, 717–731. doi: 10.1046/j.1365-2958.2002.02779.x
Bhosle, A., Datey, A., Chandrasekharan, G., Singh, D., Chakravortty, D., and Chandra, N. (2020). A Strategic target rescues trimethoprim sensitivity in Escherichia coli. iScience 23:100986. doi: 10.1016/j.isci.2020.100986
Bonnett, S. A., Dennison, D., Files, M., Bajpai, A., and Parish, T. (2018). A class of hydrazones are active against non-replicating Mycobacterium tuberculosis. PLoS ONE 13:e0198059. doi: 10.1371/journal.pone.0198059
Boshoff, H. I. M., and Barry, C. E. (2005). Tuberculosis-metabolism and respiration in the absence of growth. Nat. Rev. Microbiol. 3, 70–80. doi: 10.1038/nrmicro1065
Campbell, E. A., Korzheva, N., Mustaev, A., Murakami, K., Nair, S., Goldfarb, A., et al. (2001). Structural mechanism for rifampicin inhibition of bacterial RNA polymerase. Cell 104, 901–912. doi: 10.1016/S0092-8674(01)00286-0
Casabon, I., Swain, K., Crowe, A. M., Eltis, L. D., and Mohn, W. W. (2014). Actinobacterial acyl coenzyme a synthetases involved in steroid side-chain catabolism. J. Bacteriol. 196, 579–587. doi: 10.1128/JB.01012-13
Cho, S. H., Warit, S., Wan, B., Hwang, C. H., Pauli, G. F., and Franzblau, S. G. (2007). Low-oxygen-recovery assay for high-throughput screening of compounds against nonreplicating Mycobacterium tuberculosis. Antimicrob. Agents Chemother. 51, 1380–1385. doi: 10.1128/AAC.00055-06
Cole, S. T., Brosch, R., Parkhill, J., Garnier, T., Churcher, C., Harris, D., et al. (1998). Deciphering the biology of Mycobacterium tuberculosis from the complete genome sequence. Nature 393, 537–544. doi: 10.1038/31159
Colijn, C., Brandes, A., Zucker, J., Lun, D. S., Weiner, B., Farhat, M. R., et al. (2009). Interpreting expression data with metabolic flux models: predicting Mycobacterium tuberculosis mycolic acid production. PLoS Comput. Biol. 5:e1000489. doi: 10.1371/journal.pcbi.1000489
Colovos, C., and Yeates, T. O. (1993). Verification of protein structures: patterns of nonbonded atomic interactions. Protein Sci. 2, 1511–1519. doi: 10.1002/pro.5560020916
Deb, C., Lee, C.-M., Dubey, V. S., Daniel, J., Abomoelak, B., Sirakova, T. D., et al. (2009). A novel in vitro multiple-stress dormancy model for Mycobacterium tuberculosis generates a lipid-loaded, drug-tolerant, dormant pathogen. PLoS ONE 4:e6077. doi: 10.1371/journal.pone.0006077
Debnath, A., Parsonage, D., Andrade, R. M., He, C., Cobo, E. R., Hirata, K., et al. (2012). A high-throughput drug screen for Entamoeba histolytica identifies a new lead and target. Nat. Med. 18, 956–960. doi: 10.1038/nm.2758
Defelipe, L. A., Do Porto, D. F., Pereira Ramos, P. I., Nicolas, M. F., Sosa, E., Radusky, L., et al. (2016). A whole genome bioinformatic approach to determine potential latent phase specific targets in Mycobacterium tuberculosis. Tuberculosis 97, 181–192. doi: 10.1016/j.tube.2015.11.009
Dietze, R., Hadad, D. J., McGee, B., Molino, L. P. D., Maciel, E. L. N., Peloquin, C. A., et al. (2008). Early and extended early bactericidal activity of linezolid in pulmonary tuberculosis. Am. J. Respirat. Crit. Care Med. 178, 1180–1185. doi: 10.1164/rccm.200806-892OC
Edgar, R., Domrachev, M., and Lash, A. E. (2002). Gene Expression Omnibus: NCBI gene expression and hybridization array data repository. Nucleic Acids Res. 30, 207–210. doi: 10.1093/nar/30.1.207
Forli, S., Huey, R., Pique, M. E., Sanner, M. F., Goodsell, D. S., and Olson, A. J. (2016). Computational protein-ligand docking and virtual drug screening with the AutoDock suite. Nat. Protoc. 11, 905–919. doi: 10.1038/nprot.2016.051
Fox, G. J., Dobler, C. C., Marais, B. J., and Denholm, J. T. (2017). Preventive therapy for latent tuberculosis infection–The promise and the challenges. Int. J. Infect. Dis. 56, 68–76. doi: 10.1016/j.ijid.2016.11.006
Galagan, J. E., Minch, K., Peterson, M., Lyubetskaya, A., Azizi, E., Sweet, L., et al. (2013). The Mycobacterium tuberculosis regulatory network and hypoxia. Nature 499, 178–183. doi: 10.1038/nature12337
Gaulton, A., Bellis, L. J., Bento, A. P., Chambers, J., Davies, M., Hersey, A., et al. (2012). ChEMBL: a large-scale bioactivity database for drug discovery. Nucleic Acids Res. 40, D1100–D1107. doi: 10.1093/nar/gkr777
Gengenbacher, M., and Kaufmann, S. H. E. (2012). Mycobacterium tuberculosis: success through dormancy. FEMS Microbiol. Rev. 36, 514–532. doi: 10.1111/j.1574-6976.2012.00331.x
Gengenbacher, M., Rao, S. P. S., Pethe, K., and Dick, T. (2010). Nutrient-starved, non-replicating Mycobacterium tuberculosis requires respiration, ATP synthase and isocitrate lyase for maintenance of ATP homeostasis and viability. Microbiology 156, 81–87. doi: 10.1099/mic.0.033084-0
Ghersi, D., and Sanchez, R. (2009). EasyMIFs and SiteHound: a toolkit for the identification of ligand-binding sites in protein structures. Bioinformatics 25, 3185–3186. doi: 10.1093/bioinformatics/btp562
Ghosh, S., Baloni, P., Mukherjee, S., Anand, P., and Chandra, N. (2013). A multi-level multi-scale approach to study essential genes in Mycobacterium tuberculosis. BMC Syst. Biol. 7:132. doi: 10.1186/1752-0509-7-132
Gler, M. T., Skripconoka, V., Sanchez-Garavito, E., Xiao, H., Cabrera-Rivero, J. L., Vargas-Vasquez, D. E., et al. (2012). Delamanid for multidrug-resistant pulmonary tuberculosis. N. Engl. J. Med. 366, 2151–2160. doi: 10.1056/NEJMoa1112433
Goff, A., Cantillon, D., Muraro Wildner, L., and Waddell, S. J. (2020). Multi-omics technologies applied to tuberculosis drug discovery. Appl. Sci. 10:4629. doi: 10.3390/app10134629
Gopinath, V., Raghunandanan, S., Gomez, R. L., Jose, L., Surendran, A., Ramachandran, R., et al. (2015). Profiling the proteome of Mycobacterium tuberculosis during dormancy and reactivation. Mol. Cell. Proteom. 14, 2160–2176. doi: 10.1074/mcp.M115.051151
Griffin, J. E., Gawronski, J. D., DeJesus, M. A., Ioerger, T. R., Akerley, B. J., and Sassetti, C. M. (2011). High-resolution phenotypic profiling defines genes essential for mycobacterial growth and cholesterol catabolism. PLoS Pathog. 7:e1002251. doi: 10.1371/journal.ppat.1002251
Heirendt, L., Arreckx, S., Pfau, T., Mendoza, S. N., Richelle, A., Heinken, A., et al. (2019). Creation and analysis of biochemical constraint-based models using the COBRA Toolbox v.3.0. Nat. Protoc. 14, 639–702. doi: 10.1038/s41596-018-0098-2
Horsburgh, C. R. (2004). Priorities for the treatment of latent tuberculosis infection in the United States. N. Engl. J. Med. 350, 2060–2067. doi: 10.1056/NEJMsa031667
Huang, D. W., Sherman, B. T., and Lempicki, R. A. (2009). Bioinformatics enrichment tools: paths toward the comprehensive functional analysis of large gene lists. Nucleic Acids Res. 37, 1–13. doi: 10.1093/nar/gkn923
Huber, W., Carey, V. J., Gentleman, R., Anders, S., Carlson, M., Carvalho, B. S., et al. (2015). Orchestrating high-throughput genomic analysis with Bioconductor. Nat. Methods 12, 115–121. doi: 10.1038/nmeth.3252
Ignatov, D. V., Salina, E. G., Fursov, M. V., Skvortsov, T. A., Azhikina, T. L., and Kaprelyants, A. S. (2015). Dormant non-culturable Mycobacterium tuberculosis retains stable low-abundant mRNA. BMC Genomics 16:954. doi: 10.1186/s12864-015-2197-6
Jackson, D. R., Tu, S. S., Nguyen, M., Barajas, J. F., Schaub, A. J., Krug, D., et al. (2016). Structural insights into anthranilate priming during type II polyketide biosynthesis. ACS Chem. Biol. 11, 95–103. doi: 10.1021/acschembio.5b00500
Jamshidi, N., and Palsson, B. (2007). Investigating the metabolic capabilities of Mycobacterium tuberculosis H37Rv using the in silico strain iNJ661 and proposing alternative drug targets. BMC Syst. Biol. 1:26. doi: 10.1186/1752-0509-1-26
Kalidas, Y., and Chandra, N. (2008). PocketDepth: a new depth based algorithm for identification of ligand binding sites in proteins. J. Struct. Biol. 161, 31–42. doi: 10.1016/j.jsb.2007.09.005
Kanehisa, M. (2019). Toward understanding the origin and evolution of cellular organisms. Protein Sci. 28, 1947–1951. doi: 10.1002/pro.3715
Kanehisa, M., and Goto, S. (2000). KEGG: kyoto encyclopedia of genes and genomes. Nucleic Acids Res. 28, 27–30. doi: 10.1093/nar/28.1.27
Kanehisa, M., Sato, Y., Furumichi, M., Morishima, K., and Tanabe, M. (2019). New approach for understanding genome variations in KEGG. Nucleic Acids Res. 47, D590–D595. doi: 10.1093/nar/gky962
Kapopoulou, A., Lew, J. M., and Cole, S. T. (2011). The MycoBrowser portal: a comprehensive and manually annotated resource for mycobacterial genomes. Tuberculosis 91, 8–13. doi: 10.1016/j.tube.2010.09.006
Karakousis, P. C., Yoshimatsu, T., Lamichhane, G., Woolwine, S. C., Nuermberger, E. L., Grosset, J., et al. (2004). Dormancy phenotype displayed by extracellular Mycobacterium tuberculosis within artificial granulomas in mice. J. Exp. Med. 200, 647–657. doi: 10.1084/jem.20040646
Kaur, D., Kutum, R., Dash, D., and Brahmachari, S. K. (2017). Data intensive genome level analysis for identifying novel, non-toxic drug targets for multi drug resistant Mycobacterium tuberculosis. Sci. Rep. 7:46595. doi: 10.1038/srep46825
Kaushik, A., Ammerman, N. C., Tyagi, S., Saini, V., Vervoort, I., Lachau-Durand, S., et al. (2019). Activity of a long-acting injectable bedaquiline formulation in a paucibacillary mouse model of latent tuberculosis infection. Antimicrob. Agents Chemother. 63, 1–10. doi: 10.1128/AAC.00007-19
Kavvas, E. S., Seif, Y., Yurkovich, J. T., Norsigian, C., Poudel, S., Greenwald, W. W., et al. (2018). Updated and standardized genome-scale reconstruction of Mycobacterium tuberculosis H37Rv, iEK1011, simulates flux states indicative of physiological conditions. BMC Syst. Biol. 12:25. doi: 10.1186/s12918-018-0557-y
Kinnings, S. L., Xie, L., Fung, K. H., Jackson, R. M., Xie, L., and Bourne, P. E. (2010). The Mycobacterium tuberculosis drugome and its polypharmacological implications. PLoS Comput. Biol. 6:e1000976. doi: 10.1371/journal.pcbi.1000976
Konagurthu, A. S., Whisstock, J. C., Stuckey, P. J., and Lesk, A. M. (2006). MUSTANG: a multiple structural alignment algorithm. Prot. Struct. Funct. Bioinform. 64, 559–574. doi: 10.1002/prot.20921
Koul, A., Vranckx, L., Dendouga, N., Balemans, W., Wyngaert, I. V. D., Vergauwen, K., et al. (2008). Diarylquinolines are bactericidal for dormant mycobacteria as a result of disturbed ATP homeostasis. J. Biol. Chem. 283, 25273–25280. doi: 10.1074/jbc.M803899200
Kurthkoti, K., Amin, H., Marakalala, M. J., Ghanny, S., Subbian, S., Sakatos, A., et al. (2017). The capacity of Mycobacterium tuberculosis to survive iron starvation might enable it to persist in iron-deprived microenvironments of human granulomas. mBio 8, 1–17. doi: 10.1128/mBio.01092-17
Le Guilloux, V., Schmidtke, P., and Tuffery, P. (2009). Fpocket: an open source platform for ligand pocket detection. BMC Bioinform. 10:168. doi: 10.1186/1471-2105-10-168
Lechartier, B., Rybniker, J., Zumla, A., and Cole, S. T. (2014). Tuberculosis drug discovery in the post-post-genomic era. EMBO Mol. Med. 6, 158–168. doi: 10.1002/emmm.201201772
Lew, J. M., Kapopoulou, A., Jones, L. M., and Cole, S. T. (2011). TubercuList-10 years after. Tuberculosis 91, 1–7. doi: 10.1016/j.tube.2010.09.008
McKinney, J. D., zu Bentrup, K. H., Munoz-Elias, E. J., Miczak, A., Chen, B., Chan, W.-T., et al. (2000). Persistence of Mycobacterium tuberculosis in macrophages and mice requires the glyoxylate shunt enzyme isocitrate lyase. Nature 406, 735–738. doi: 10.1038/35021074
Minch, K. J., Rustad, T. R., Peterson, E. J. R., Winkler, J., Reiss, D. J., Ma, S., et al. (2015). The DNA-binding network of Mycobacterium tuberculosis. Nat. Commun. 6:5829. doi: 10.1038/ncomms6829
Murphy, D. J., and Brown, J. R. (2007). Identification of gene targets against dormant phase Mycobacterium tuberculosis infections. BMC Infect. Dis. 7:84. doi: 10.1186/1471-2334-7-84
Nagabushan, H., and Roopadevi, H. S. (2014). Bedaquiline: a novel antitubercular drug for multidrug-resistant tuberculosis. J. Postgrad. Med. 60:300. doi: 10.4103/0022-3859.138772
Naidoo, A., Naidoo, K., McIlleron, H., Essack, S., and Padayatchi, N. (2017). A review of moxifloxacin for the treatment of drug-susceptible tuberculosis. J. Clin. Pharmacol. 57, 1369–1386. doi: 10.1002/jcph.968
O'Boyle, N. M., Banck, M., James, C. A., Morley, C., Vandermeersch, T., and Hutchison, G. R. (2011). Open Babel: an open chemical toolbox. J. Cheminform. 3:33. doi: 10.1186/1758-2946-3-33
Orth, J. D., Thiele, I., and Palsson, B. (2010). What is flux balance analysis? Nat. Biotechnol. 28, 245–248. doi: 10.1038/nbt.1614
Padiadpu, J., Baloni, P., Anand, K., Munshi, M., Thakur, C., Mohan, A., et al. (2016). Identifying and tackling emergent vulnerability in drug-resistant mycobacteria. ACS Infect. Dis. 2, 592–607. doi: 10.1021/acsinfecdis.6b00004
Park, H.-D., Guinn, K. M., Harrell, M. I., Liao, R., Voskuil, M. I., Tompa, M., et al. (2003). Rv3133c/dosR is a transcription factor that mediates the hypoxic response of Mycobacterium tuberculosis. Mol. Microbiol. 48, 833–843. doi: 10.1046/j.1365-2958.2003.03474.x
Peterson, E. J. R., Abidi, A. A., Arrieta-Ortiz, M. L., Aguilar, B., Yurkovich, J. T., Kaur, A., et al. (2020). Intricate genetic programs controlling dormancy in Mycobacterium tuberculosis. Cell Rep. 31:107577. doi: 10.1016/j.celrep.2020.107577
Peterson, E. J. R., Ma, S., Sherman, D. R., and Baliga, N. S. (2016). Network analysis identifies Rv0324 and Rv0880 as regulators of bedaquiline tolerance in Mycobacterium tuberculosis. Nat. Microbiol. 1, 1–7. doi: 10.1038/nmicrobiol.2016.78
Pethe, K., Swenson, D. L., Alonso, S., Anderson, J., Wang, C., and Russell, D. G. (2004). Isolation of Mycobacterium tuberculosis mutants defective in the arrest of phagosome maturation. Proc. Natl. Acad. Sci. U.S.A. 101, 13642–13647. doi: 10.1073/pnas.0401657101
Puckett, S., Trujillo, C., Wang, Z., Eoh, H., Ioerger, T. R., Krieger, I., et al. (2017). Glyoxylate detoxification is an essential function of malate synthase required for carbon assimilation in Mycobacterium tuberculosis. Proc. Natl. Acad. Sci. U.S.A. 114, E2225–E2232. doi: 10.1073/pnas.1617655114
Raman, K., and Chandra, N. (2009). Flux balance analysis of biological systems: applications and challenges. Brief. Bioinform. 10, 435–449. doi: 10.1093/bib/bbp011
Raman, K., Yeturu, K., and Chandra, N. (2008). targetTB: a target identification pipeline for Mycobacterium tuberculosis through an interactome, reactome and genome-scale structural analysis. BMC Syst. Biol. 2:109. doi: 10.1186/1752-0509-2-109
Rao, S. P. S., Alonso, S., Rand, L., Dick, T., and Pethe, K. (2008). The protonmotive force is required for maintaining ATP homeostasis and viability of hypoxic, nonreplicating Mycobacterium tuberculosis. Proc. Natl. Acad. Sci. U.S.A. 105, 11945–11950. doi: 10.1073/pnas.0711697105
Ritchie, M. E., Phipson, B., Wu, D., Hu, Y., Law, C. W., Shi, W., et al. (2015). limma powers differential expression analyses for RNA-sequencing and microarray studies. Nucleic Acids Res. 43:e47. doi: 10.1093/nar/gkv007
Robinson, M. D., McCarthy, D. J., and Smyth, G. K. (2010). edgeR: a Bioconductor package for differential expression analysis of digital gene expression data. Bioinformatics 26, 139–140. doi: 10.1093/bioinformatics/btp616
Robinson, M. D., and Oshlack, A. (2010). A scaling normalization method for differential expression analysis of RNA-seq data. Genome Biol. 11:R25. doi: 10.1186/gb-2010-11-3-r25
Rose, P. W., Prlic, A., Altunkaya, A., Bi, C., Bradley, A. R., Christie, C. H., et al. (2017). The RCSB protein data bank: integrative view of protein, gene and 3D structural information. Nucleic Acids Res. 45, D271–D281. doi: 10.1093/nar/gkw1000
Russell, D. G. (2001). Mycobacterium tuberculosis: here today, and here tomorrow. Nat. Rev. Mol. Cell Biol. 2, 569–577. doi: 10.1038/35085034
Rustad, T. R., Harrell, M. I., Liao, R., and Sherman, D. R. (2008). The enduring hypoxic response of Mycobacterium tuberculosis. PLoS ONE 3:e1502. doi: 10.1371/journal.pone.0001502
Saha, S., Kumar, A., Saurabh, K., Shankar, S. H., Kashyap, A., Nischal, N., et al. (2020). Current status of treatment of latent tuberculosis infection in India. Indian J. Med. Sci. 71, 54–59. doi: 10.25259/IJMS_18_2019
Salina, E., Mollenkopf, H., Kaufmann, S., and Kaprelyants, A. (2009). M. tuberculosis gene expression during transition to the “non-culturable” state. Acta Nat. 1, 73–77. doi: 10.32607/20758251-2009-1-2-73-77
Salina, E. G., Huszar, S., Zemanova, J., Keruchenko, J., Riabova, O., Kazakova, E., et al. (2018). Copper-related toxicity in replicating and dormant Mycobacterium tuberculosis caused by 1-hydroxy-5- R -pyridine-2(1 H)-thiones. Metallomics 10, 992–1002. doi: 10.1039/C8MT00067K
Sambarey, A., Devaprasad, A., Baloni, P., Mishra, M., Mohan, A., Tyagi, P., et al. (2017). Meta-analysis of host response networks identifies a common core in tuberculosis. NPJ Syst. Biol. Appl. 3, 1–12. doi: 10.1038/s41540-017-0005-4
Sambarey, A., Prashanthi, K., and Chandra, N. (2013). Mining large-scale response networks reveals ‘topmost activities' in Mycobacterium tuberculosis infection. Sci. Rep. 3:2302. doi: 10.1038/srep02302
Santucci, P., Bouzid, F., Smichi, N., Poncin, I., Kremer, L., De Chastellier, C., et al. (2016). Experimental models of foamy macrophages and approaches for dissecting the mechanisms of lipid accumulation and consumption during dormancy and reactivation of tuberculosis. Front. Cell. Infect. Microbiol. 6:122. doi: 10.3389/fcimb.2016.00122
Schnappinger, D., Ehrt, S., Voskuil, M. I., Liu, Y., Mangan, J. A., Monahan, I. M., et al. (2003). Transcriptional adaptation of Mycobacterium tuberculosis within macrophages. J. Exp. Med. 198, 693–704. doi: 10.1084/jem.20030846
Schubert, O., Ludwig, C., Kogadeeva, M., Zimmermann, M., Rosenberger, G., Gengenbacher, M., et al. (2015). Absolute proteome composition and dynamics during dormancy and resuscitation of Mycobacterium tuberculosis. Cell Host Microbe 18, 96–108. doi: 10.1016/j.chom.2015.06.001
Shannon, P., Markiel, A., Ozier, O., Baliga, N. S., Wang, J. T., Ramage, D., et al. (2003). Cytoscape: a software environment for integrated models of biomolecular interaction networks. Genome Res. 13, 2498–2504. doi: 10.1101/gr.1239303
Siqueira-Neto, J. L., Song, O.-R., Oh, H., Sohn, J.-H., Yang, G., Nam, J., et al. (2010). Antileishmanial high-throughput drug screening reveals drug candidates with new scaffolds. PLoS Negl. Trop. Dis. 4:e675. doi: 10.1371/journal.pntd.0000675
Starck, J., Kallenius, G., Marklund, B.-I., Andersson, D. I., and Akerlund, T. (2004). Comparative proteome analysis of Mycobacterium tuberculosis grown under aerobic and anaerobic conditions. Microbiology 150, 3821–3829. doi: 10.1099/mic.0.27284-0
Stover, C. K., Warrener, P., VanDevanter, D. R., Sherman, D. R., Arain, T. M., Langhorne, M. H., et al. (2000). A small-molecule nitroimidazopyran drug candidate for the treatment of tuberculosis. Nature 405, 962–966. doi: 10.1038/35016103
Szklarczyk, D., Franceschini, A., Wyder, S., Forslund, K., Heller, D., Huerta-Cepas, J., et al. (2015). STRING v10: protein-protein interaction networks, integrated over the tree of life. Nucleic Acids Res. 43, D447–D452. doi: 10.1093/nar/gku1003
Timmins, G. S., and Deretic, V. (2006). Mechanisms of action of isoniazid. Mol. Microbiol. 62, 1220–1227. doi: 10.1111/j.1365-2958.2006.05467.x
Tripathi, S. M., and Ramachandran, R. (2008). Crystal structures of the Mycobacterium tuberculosis secretory antigen alanine dehydrogenase (Rv2780) in apo and ternary complex forms captures “open” and “closed” enzyme conformations. Proteins Struct. Funct. Bioinform. 72, 1089–1095. doi: 10.1002/prot.22101
Trivedi, O. A., Arora, P., Sridharan, V., Tickoo, R., Mohanty, D., and Gokhale, R. S. (2004). Enzymic activation and transfer of fatty acids as acyl-adenylates in mycobacteria. Nature 428, 441–445. doi: 10.1038/nature02384
UniProt Consortium (2019). UniProt: a worldwide hub of protein knowledge. Nucleic Acids Res. 47, D506–D515. doi: 10.1093/nar/gky1049
VanderVen, B. C., Fahey, R. J., Lee, W., Liu, Y., Abramovitch, R. B., Memmott, C., et al. (2015). Novel Inhibitors of cholesterol degradation in Mycobacterium tuberculosis reveal how the bacterium's metabolism is constrained by the intracellular environment. PLoS Pathog. 11:e1004679. doi: 10.1371/journal.ppat.1004679
Voskuil, M. I., Visconti, K. C., and Schoolnik, G. K. (2004). Mycobacterium tuberculosis gene expression during adaptation to stationary phase and low-oxygen dormancy. Tuberculosis 84, 218–227. doi: 10.1016/j.tube.2004.02.003
Wang, Y., Cui, T., Zhang, C., Yang, M., Huang, Y., Li, W., et al. (2010). Global protein-protein interaction network in the human pathogen Mycobacterium tuberculosis H37Rv. J. Proteome Res. 9, 6665–6677. doi: 10.1021/pr100808n
Wayne, L. G., and Lin, K. Y. (1982). Glyoxylate metabolism and adaptation of Mycobacterium tuberculosis to survival under anaerobic conditions. Infect. Immun. 37, 1042–1049. doi: 10.1128/IAI.37.3.1042-1049.1982
Wayne, L. G., and Sramek, H. A. (1994). Metronidazole is bactericidal to dormant cells of Mycobacterium tuberculosis. Antimicrob. Agents Chemother. 38, 2054–2058. doi: 10.1128/AAC.38.9.2054
Webb, B., and Sali, A. (2016). Comparative protein structure modeling using MODELER. Curr. Protoc. Protein Sci. 86, 2.9.1–2.9.37. doi: 10.1002/cpps.20
Wishart, D. S., Knox, C., Guo, A. C., Cheng, D., Shrivastava, S., Tzur, D., et al. (2008). DrugBank: a knowledgebase for drugs, drug actions and drug targets. Nucleic Acids Res. 36(Suppl_1), D901–D906. doi: 10.1093/nar/gkm958
Wu, F.-L., Liu, Y., Jiang, H.-W., Luan, Y.-Z., Zhang, H.-N., He, X., et al. (2017). The Ser/Thr protein kinase protein-protein interaction map of M. tuberculosis. Mol. Cell. Proteomics 16, 1491–1506. doi: 10.1074/mcp.M116.065771
Wu, S., and Zhang, Y. (2008). MUSTER: improving protein sequence profile-profile alignments by using multiple sources of structure information. Proteins Struct. Funct. Bioinform. 72, 547–556. doi: 10.1002/prot.21945
Yeturu, K., and Chandra, N. (2008). PocketMatch: a new algorithm to compare binding sites in protein structures. BMC Bioinform. 9:543. doi: 10.1186/1471-2105-9-543
Keywords: latent tuberculosis, target identification, systems biology, chemoinformatics, lead identification, metabolic modeling, response network
Citation: Banerjee U, Sankar S, Singh A and Chandra N (2020) A Multi-Pronged Computational Pipeline for Prioritizing Drug Target Strategies for Latent Tuberculosis. Front. Chem. 8:593497. doi: 10.3389/fchem.2020.593497
Received: 10 August 2020; Accepted: 24 November 2020;
Published: 14 December 2020.
Edited by:
Ricardo Daniel Enriz, National University of San Luis, ArgentinaReviewed by:
Simon Waddell, Brighton and Sussex Medical School, United KingdomGuillermo R. Labadie, National University of Rosario, Argentina
Copyright © 2020 Banerjee, Sankar, Singh and Chandra. This is an open-access article distributed under the terms of the Creative Commons Attribution License (CC BY). The use, distribution or reproduction in other forums is permitted, provided the original author(s) and the copyright owner(s) are credited and that the original publication in this journal is cited, in accordance with accepted academic practice. No use, distribution or reproduction is permitted which does not comply with these terms.
*Correspondence: Nagasuma Chandra, bmNoYW5kcmEmI3gwMDA0MDtpaXNjLmFjLmlu
†These authors have contributed equally to this work