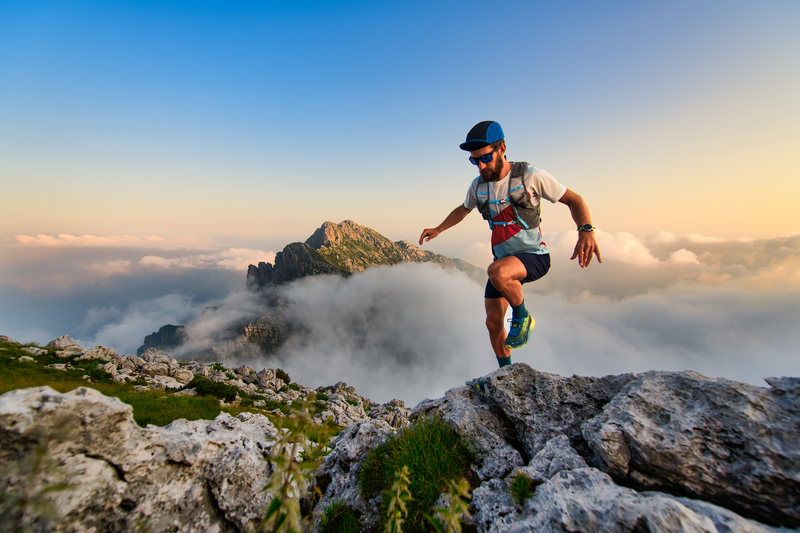
94% of researchers rate our articles as excellent or good
Learn more about the work of our research integrity team to safeguard the quality of each article we publish.
Find out more
ORIGINAL RESEARCH article
Front. Chem. , 05 November 2020
Sec. Medicinal and Pharmaceutical Chemistry
Volume 8 - 2020 | https://doi.org/10.3389/fchem.2020.592289
This article is part of the Research Topic Molecular Studies of COVID-19 Chemistry View all 19 articles
The coronavirus disease 19 (COVID-19) is a rapidly growing pandemic caused by the severe acute respiratory syndrome coronavirus 2 (SARS-CoV-2). Its papain-like protease (SARS-CoV-2 PLpro) is a crucial target to halt virus replication. SARS-CoV PLpro and SARS-CoV-2 PLpro share an 82.9% sequence identity and a 100% sequence identity for the binding site reported to accommodate small molecules in SARS-CoV. The flexible key binding site residues Tyr269 and Gln270 for small-molecule recognition in SARS-CoV PLpro exist also in SARS-CoV-2 PLpro. This inspired us to use the reported small-molecule binders to SARS-CoV PLpro to generate a high-quality DEKOIS 2.0 benchmark set. Accordingly, we used them in a cross-benchmarking study against SARS-CoV-2 PLpro. As there is no SARS-CoV-2 PLpro structure complexed with a small-molecule ligand publicly available at the time of manuscript submission, we built a homology model based on the ligand-bound SARS-CoV structure for benchmarking and docking purposes. Three publicly available docking tools FRED, AutoDock Vina, and PLANTS were benchmarked. All showed better-than-random performances, with FRED performing best against the built model. Detailed performance analysis via pROC-Chemotype plots showed a strong enrichment of the most potent bioactives in the early docking ranks. Cross-benchmarking against the X-ray structure complexed with a peptide-like inhibitor confirmed that FRED is the best-performing tool. Furthermore, we performed cross-benchmarking against the newly introduced X-ray structure complexed with a small-molecule ligand. Interestingly, its benchmarking profile and chemotype enrichment were comparable to the built model. Accordingly, we used FRED in a prospective virtual screen of the DrugBank1 database. In conclusion, this study provides an example of how to harness a custom-made DEKOIS 2.0 benchmark set as an approach to enhance the virtual screening success rate against a vital target of the rapidly emerging pandemic.
The latest situation report of the World Health Organization (WHO), of May 6, 2020, reported that COVID-19 is highly spreading worldwide in over 184 countries and responsible so far for >3.6 million cases and >260,000 fatalities. Severe acute respiratory syndrome coronavirus 2 (SARS-CoV-2) is the causative virus for COVID-19 and was recognized in Wuhan, China (Li et al., 2020b; Qian et al., 2020; Rabi et al., 2020; Tilocca et al., 2020). Coronaviruses belong to a large family of enveloped single-stranded RNA genome (ssRNA) that belong to the Coronaviridae family and divided into four genera: alpha, beta, gamma, and delta coronaviruses (Yang and Leibowitz, 2015). Among coronaviruses, some instigated several respiratory diseases, such as SARS-CoV (Drosten et al., 2003), Middle East respiratory syndrome coronavirus (MERS-CoV) (Zaki et al., 2012), and the pandemic COVID-19 (Rabi et al., 2020). SARS-CoV-2 are beta coronaviruses (Li et al., 2020a; Rabi et al., 2020) with symptoms usually resembling other respiratory virus infections like influenza and rhinovirus (Hsih et al., 2020).
Upon the virion entry to the host cell, translation of 5′-terminal open reading frames (ORF1a and ORF1ab) is initiated to produce two large polyproteins, pp1a and pp1ab, which are then processed by papain-like protease (PLpro) and 3C-like protease (3CLpro), also called main protease (Mpro) (Barretto et al., 2005; Mielech et al., 2015). This processing is crucial for the release of 16 non-structural proteins (nsps1–16). The formation of the replicase complex essential for viral genome replication is dependent on nsps (Fehr and Perlman, 2015). PLpro plays an essential role for the release of nsp1–3 from the viral polyprotein which are indispensable for viral replication. Also, PLpro has been observed to negatively regulate the host innate immune response toward the viral infection by its deubiquitinating and deISGylating effect (Báez-Santos et al., 2015; Clemente et al., 2020). As a result, PLpro has been recognized as an important target for viral replication suppression endeavors in SARS-CoV and SARS-CoV-2 (Báez-Santos et al., 2015; Freitas et al., 2020).
Structure-based virtual screening (SBVS) remained a crucial technique in modern drug discovery (Schapira et al., 2003; Schneider, 2010; Santiago et al., 2012; Scior et al., 2012). Molecular docking is widely employed in SBVS campaigns, which exploits the structural information of the molecular targets binding sites to assess large molecular databases and predict the preferred binding of compounds prior to the biological screening. Nevertheless, the docking tool and the VS workflow selection must be assessed using benchmarking molecular sets. The benchmarking depends on challenging the VS workflow to enrich known bioactives within a set of decoys (Bauer et al., 2013; Ibrahim et al., 2015a).
In this study, we benchmark three publicly available docking tools, AutoDock Vina, PLANTS, and FRED against SARS-CoV-2 PLpro. One challenge comprises the absence of small molecules known to inhibit SARS-CoV-2 PLpro and consequently to generate a matching decoy set. Another challenge encompasses the absence of structural conformation of the SARS-CoV-2 PLpro binding site when complexed with conventional small molecules. To overcome these challenges, we conducted a cross benchmark by generating a DEKOIS 2.0 benchmark set of known SARS-CoV PLpro bioactives for SARS-CoV-2 PLpro, using the advantage of the high similarity between both enzymes and identical binding site residues. Furthermore, we modeled the conformation of SARS-CoV-2 complexed with small molecule based on its co-crystal structure homolog, SARS-CoV PLpro. Guided by the benchmarking outcome, we performed a VS effort against the DrugBank database and discuss the most promising hits. This study offers an example of how to employ a DEKOIS 2.0 benchmark set to enhance virtual screening success against a vital target of SARS-CoV-2. This procedure may facilitate virtual finding also against other rapidly resolved protein structures of SARS-CoV-2.
Genome sequencing showed an 80% similarity between SARS-CoV and SARS-CoV-2 genetic sequences (Rabaan et al., 2020; Rabi et al., 2020; Zhou et al., 2020). The multiple-sequence alignment (MSA) of PLpro from the most clinically relevant human corona viruses, e.g., SADS, MERS, SARS-CoV-2, and SARS-CoV PLpro, is portrayed in Figure 1. Comparing the percentage sequence identity of SARS-CoV-2 PLpro with other human corona viruses reveals that SARS-CoV PLpro is the closest strain to the SARS-CoV-2 PLpro with 82.9% identity. Interestingly, SARS-CoV PLpro and SARS-CoV-2 PLpro share identical binding site residues for small-molecule binding, as marked by the blue-dashed rectangle in Figure 1. Residues Tyr269 and Gln270 in SARS-CoV, marked by the red-dashed rectangle, play an important role in small molecule-protein binding event (Ratia et al., 2008; Ghosh et al., 2009). They encompass a flexible loop capable of accommodating different backbone and side chain conformations. Interestingly, it was reported that small-molecule inhibitors of SARS-CoV PLpro were not able to recognize and specifically inhibit MERS-PLpro (Lee et al., 2015). This is attributed to many factors among which is the lack of the key residues Tyr269 and Gln270 of SARS-CoV PLpro in MERS-CoV PLpro (Lee et al., 2015), as shown by the red arrow of Figure 1. Interestingly, such key residues are present in SARS-CoV-2 PLpro (Tyr268 and Gln269).
Figure 1. Multiple-sequence alignment for PLpro sequences of some clinically relevant corona virus strains (SADS, MERS, SARS-CoV-2, and SARS-CoV). Identical and less conserved residues are highlighted red and yellow, respectively. Red arrow and red-dashed rectangle indicate the flexible loop residues Tyr269 and Gln270 as a part of the binding site. Residues of the binding site are marked by blue-dashed rectangles.
Binding site residues of both SARS-CoV and SARS-CoV-2 PLpro are 100% identical. The PDB structures of both (SARS-CoV and SARS-CoV-2 PLpro) proteins appear to show a comparable fold and do not deviate substantially in backbone conformations. For SARS-CoV-2 PLpro, three crystal structures are available in the apo form, with an average pairwise RMSD matrix for the backbone around 1 Å, as seen in Figure 2. The binding site exhibits more diverse conformations for the backbone and side chains of the flexible loop (i.e., Tyr268 and Gln269) where the side chains mostly appear to point outward to the solvent exposed area, as shown in Figure 2B. These conformations represent only the unbound state (apo state) for the binding site. It is noteworthy that some SARS-CoV-2 PLpro structures complexed with peptide-like binders were introduced in the PDB, while none complexed with conventional small molecules are available yet at the time of manuscript submission. Figure 2 shows a depiction of these SARS-CoV-2 PLpro structures.
Figure 2. (A) Superposition of the different SARS-CoV-2 PLpro structures including three apo structures and two complexed with peptide binders, namely, PDB ID: 6W9C, 6WRH, 6YVA, 6WUU, and 6WX4 as green, yellow, simon, purple, and gray cartoon representation, respectively. (B) Different conformations of the backbone and side chains of the key Tyr268 and Gln269 residues. (C) Pairwise RMSD matrix for all structures calculated for their α carbon atoms.
To have a clue on the possible rearrangement of SARS-CoV-2 PLpro upon conventional small-molecule binding, we investigated its homolog, the SARS-CoV PLpro co-crystal structures complexed with small molecules. For this, we retrieved 11 high-quality crystal structures of SARS-CoV PLpro for both small-molecule complexes and the unbound structures to small molecules (referred to as apo structures in this study). Like SARS-CoV-2, the apo SARS-CoV PLpro (seven crystal structures) displayed a wide range of conformations for the backbone and side chains of the flexible loop (residues Tyr269 and Gln270 in Figure 3A) with an average pairwise RMSD values for the backbone <2 Å (data not shown). On the other hand, the co-crystal structures with small molecules (four crystal structures) showed more ordered rearrangement of Tyr269 for all of them. This is likely to offer a hydrophobic wall for optimum interactions with the aromatic substructure of the bound small molecule (Figure 3B; Lee et al., 2015), while Gln270 appeared to adapt more conformations depending on ligand topology and size. Based on the previous, it is likely that SARS-CoV-2 PLpro would behave in a similar fashion to its analog, SARS-CoV PLpro, upon small-molecule binding. Therefore, for docking and benchmarking purposes, and due to the lack of co-crystallized structures of SARS-CoV-2 PLpro with small molecules, we constructed a homology model for SARS-CoV-2 PLpro complexed with small molecule, based on its co-crystallized homolog SARS-CoV PLpro.
Figure 3. (A) Superposition of the SARS-CoV PLpro apo structures for PDB ID: 4M0W (Chou et al., 2014), 4MM3 (Ratia et al., 2014), 5E6J (Bekes et al., 2016), 5TL6 (Daczkowski et al., 2017), 5TL7 (Daczkowski et al., 2017), 5Y3Q (Lin et al., 2018) (conjugated with beta-mercaptoethanol), and 2FE8 (Ratia et al., 2006) as cyan, pale blue, green, yellow, orange, dark orange, and gray cartoon representation, respectively, showing vast conformations of Tyr269 and Gln270 residues. (B) Superposition of the SARS-CoV PLpro co-crystal structures with small molecules for PDB ID: 3MJ5 (Ghosh et al., 2010), 4OVZ (Baez-Santos et al., 2014), 4OW0 (Baez-Santos et al., 2014), and 3E9S as cyan, pale violet, purple, and gold cartoon representation, respectively, exhibiting more ordered conformations of Tyr269 and Gln270 residues.
A model of SARS-CoV-2 PLpro (317 residues) complexed with a small-molecule ligand (compound TTT, “5-amino-2-methyl-N-[(1R)-1-naphthalen-1-ylethyl]benzamide”) is built by the aid of the automated homology modeling, SWISS-MODEL (Waterhouse et al., 2018) web server, using SARS-CoV PLpro (PDB ID: 3E9S, chain A) as a template, as shown in Figure 4. The model has a high sequence identity (82.9%) to the template. Quality estimates for the built model indicated high reliability of the model, with a QMEAN (Benkert et al., 2011) value of −0.22 and GMQE (Global Model Quality Estimation) (Waterhouse et al., 2018) value of 0.95. The Ramachandran plot, in Figure 4B, shows that 100% of the residues are in the allowed regions. Also, it displayed that 94.9% of the residues, including the binding site residues, are in the most favored region. In addition, the validation web servers (SAVES, 2020) presented that 98.73% of the residues have averaged 3D-1D score ≥0.2 on the Verify 3D module. The overall quality factor of ERRAT is 92.8 %. Globally, these values indicate a valid and a high-quality homology model.
Figure 4. (A) The newly emerged SARS-CoV-2 PLpro ligand-protein-complexed model built by SWISS-MODEL in the blue cartoon and its bound ligand in gold sticks. (B) Ramachandran plot for SARS-CoV-2 PLpro model. (C) The enlarged part of the binding site showing the conformations of the key Tyr268 and Gln269 residues.
Figure 4C exhibits a noticeable difference in the side chain conformations of the key Tyr298 and Gln269 between the model and the X-ray structure complexed with a peptide-like inhibitor (Figure 2B). Unlike the model, both key residues of the latter structure (i.e., PDB ID: 6WUU) appear to point outward to the solvent-exposed area.
While our manuscript was under review, new X-ray co-crystal structures with impact on our study were released. Thus, we closely investigated an example of these structures in comparison to the homology model we built. For instance, we considered the recently introduced X-ray co-crystal structure complexed with compound TTT (PDB ID: 7JRN). This structure is for the wild type and with best resolution available for a SARS-CoV2 PLpro-TTT complex. We did not observe a significant difference between both the model and the X-ray structure (average RMSD for the whole proteins = 0.98 Å). Interestingly, unlike the X-ray structure complexed with the peptide-like inhibitor (PDB ID: 6WUU), both the homology model and the SARS-CoV2 PLpro-TTT complex (PDB ID: 7JRN) exhibited almost similar conformations for the key residues Tyr298 and Gln269, as well as for the pose of TTT, as shown in Figure 5. This reflects the high reliability and quality of our predicted model.
Figure 5. (A) Superposition of three SARS-CoV2 PLpro structures involving the homology model, the X-ray structure complexed with peptide-like inhibitor (PDB ID: 6WUU), and the recently introduced X-ray structure complexed with TTT (PDB ID: 7JRN) as gray, purple, and gold cartoon representation, respectively. (B) The enlarged part of the binding site showing comparable conformations of the key Tyr268 and Gln269 residues and the bound ligand (TTT) for both the homology and the X-ray structure (PDB ID: 7JRN). The co-crystal peptide-like ligand (PDB ID: 6WUU) was omitted for clarity.
Generally, it was reported that VS performance depends strongly on the respective target properties (Bauer et al., 2013). Accordingly, diverse docking methods and scoring schemes may work better on some targets than others. To avert delays and unnecessary efforts on unproductive VS strategies, it is crucial to evaluate the performance of different VS setups in order to select the most effective workflow (Ibrahim et al., 2015a). Screening performance can be assessed using molecular benchmark sets, such as DEKOIS 2.0 (Vogel et al., 2011; Bauer et al., 2013; Boeckler et al., 2014) and DUD-E (Mysinger et al., 2012). The idea aims at recognizing the suitable docking tool that can efficiently differentiate between the bioactive ligands and the generated challenging decoys. The higher the number of bioactives at the top of the score-ordered list of screened molecules, the better is the respective screening performance.
Due to the lack of small-molecule ligands for SARS-CoV-2 PLpro, and the high similarity of both SARS-CoV and SARS-CoV-2 PLpro enzymes, we performed cross benchmarking of SARS-CoV-2 PLpro based on SARS-CoV PLpro reported small-molecule ligands. We generated a challenging decoy set by our DEKOIS 2.0 (Vogel et al., 2011; Bauer et al., 2013; Boeckler et al., 2014) protocol from the available bioactives of SARS-CoV PLpro (retrieved from BindingDB; Liu et al., 2007). Then, we conducted a benchmarking study using three publicly available docking tools, namely, AutoDock Vina, PLANTS, and FRED. Pleasingly, a recent study by Freitas et al. confirmed our cross benchmarking approach since the naphthalene-based SARS-CoV PLpro inhibitors showed inhibitory activities against SARS-CoV-2 PLpro and stopped the SARS-CoV-2 replication (Freitas et al., 2020).
The SARS-CoV-2 PLpro homology model benchmarking results revealed that FRED screening performance exhibited the best performance with a pROC-AUC value of 2.15, compared to pROC-AUC values of 1.35 and 0.98 for AutoDock Vina and PLANTS, respectively (Figure 6A). Interestingly, the screening performance against the co-crystal SARS-CoV PLpro structure (PDB ID: 3E9S) yielded a comparable outcome for FRED, and non-significant differences (i.e., ΔpROC-AUC values ≤ 0.05; Bauer et al., 2013) for AutoDock Vina and PLANTS docking tools (Figure 6B). Therefore, these results emphasize also the druggability of the SARS-CoV-2 PLpro homology model by the SARS-CoV PLpro benchmark set. Interestingly, all docking tools exhibited better-than-random performance, i.e., pROC-AUC value >0.43.
Figure 6. pROC plots of docking experiments showing the screening performance of both ligand-bound conformations of the SARS-CoV-2 PLpro model, the co-crystal structure of SARS-CoV PLpro (PDB ID: 3E9S), and the co-crystal structure SARS-CoV2 PLpro (PDB ID: 6WUU) as (A–C), respectively. (D,E) are for the screening performance of both apo structures of SARS-CoV-2 PLpro (PDB ID: 6W9C) and of SARS-CoV PLpro (PDB ID: 2FE8), respectively. (F) The screening performance of the recently introduced X-ray SARS-CoV-2 PLpro complexed with TTT (PDB ID: 7JRN). The graphs of the docking tools FRED, AutoDock Vina, and PLANTS screening results are shown in green, orange, and blue, respectively. The true positive rate (TPR) is the fraction of recovered bioactives; the false-positive rate (FPR) is the fraction of recovered decoys from a score-ordered list of all decoys. The gray line corresponds to a random screening performance.
In addition, benchmarking results of the co-crystal X-ray structure of SARS-CoV2 with a peptide-like inhibitor (e.g., PDB ID: 6WUU) emphasizes that FRED screening performance appeared to be superior to AutoDock Vina and PLANTS, with pROC-AUC values of 0.95, 0.61, and 0.51, respectively (Figure 6C). Nonetheless, in this case, the three docking tools exhibited significant lower performances compared to the homology model. This is likely attributed to the differences in the backbone and side-chain conformations of the key Tyr298 and Gln269 between the model and the X-ray structure complexed with a peptide-like inhibitor (as shown earlier in Figure 5). We also assessed the in silico druggability of the unbound conformation (i.e., apo form) of the binding site of SARS-CoV-2 PLpro (PDB ID: 6W9C) and SARS-CoV PLpro (PDB ID: 2FE8) using the generated DEKOIS 2.0 benchmark set. Most of the docking tools showed significant lower performance compared to the bound state as shown in Figures 6D,E.
The benchmarking outcome of the newly introduced X-ray complexed with TTT (PDB ID: 7JRN) displayed non-significant differences from the homology model for FRED and PLANTS, and a slightly improved performance for AutoDock Vina, as seen in Figure 6F. This is likely attributed to the comparable conformations of the key residues (Tyr298 and Gln269) for both protein structures.
We analyzed the chemotype enrichment with the “pROC-Chemotype” (Ibrahim et al., 2014, 2015b) plot (see Figure 7) for the benchmarking of the SARS-CoV-2 PLpro model using the FRED docking tool. Only 32 small-molecule binders to SARS-CoV PLpro were introduced and collected by the BindingDB repository (Liu et al., 2007) when searching with the keyword “SARS coronavirus papain-like protease.” These molecules were collected mainly from Ghosh et al. (2009) and Lee et al. (2015). This ended up to 24 small-molecule binders after we curated them and removed the duplicates.
Figure 7. (A) pROC-Chemotype plot (Ibrahim et al., 2015b) of the SARS-CoV-2 PLpro model using the FRED docking tool. The docking information is matched with the chemotype represented by the cluster number and the bioactivity information. The bioactivity information is represented as the rank of the ligand bioactivity, where the color scales from yellow (less potent) to red (more potent). The red-dashed line indicates an enrichment of bioactives as at the 1% database. Enrichment Factor (EF) evaluates the capability of the docking tool to find true positives in the score-ranked list compared to the random selection. EF is calculated based on the succeeding equation (Wei et al., 2002) . (B,C) The best docking and the second-best docking poses of the bioactive set overlaid on the model ligand as orange and cyan sticks, respectively. (D) Box plot of the fitness vs. chemotype clusters illustrating the bioactive molecules distribution. Fitness is expressed as the FRED score multiplied by −1 for comparison purposes. (E) Heat map of the three chemotype clusters of the SARS-CoV PLpro benchmark set based on the average Tanimoto similarity (Ts) over all cross-cluster pairs. The color gradient represents changes in the average Ts. Green indicates maximum dissimilarity (Ts ≈ 0), and red indicates maximum similarity (Ts = 1).
Maximum common substructure (MCS) (Ibrahim et al., 2015b) chemotype clustering demonstrates 3 main clusters representing different chemotype classes. Clusters 1 and 2 represent singletons (i.e., a compound per cluster), while cluster 3 (methyl naphthalene substructure) represents the rest of the bioactive compounds. Therefore, the average Tanimoto similarity (Ts) was determined by using definition 1 for clusters 1 and 2, while showing Ts <1 for cluster 3, as shown in the relative intercluster (dis)similarity (Figure 7E). Generally, such MCS clustering behavior reflects the narrow diversity of the known chemotypes, emphasizing the need of developing more diverse small-molecule inhibitors for SARS-CoV PLpro and eventually for SARS-CoV-2 PLpro. The bioactivity data are represented by level of activity (LOA) ranging from 10−4 to 10−7 M and recorded as IC50, Ki, or Kd as a type of data (TOD), as seen in Figure 7.
The pROC-Chemotype plot visualized that the applied docking protocol is likely capable of detecting high-affinity binders at early enrichment, as seen in Figure 7. For instance, the best two docked active molecules (docking rank 1 and 2) are also the highest in bioactivity (i.e., with bioactivity rank 2 and 1, respectively, Figure 7A) with IC50 values of 230 and 460 nM against SARS-CoV PLpro (Ghosh et al., 2009; Lee et al., 2015). Visualizing their docking poses emphasizes that they reproduced the key interactions of the model ligand, as shown in Figures 7B,C. It is worth mentioning that such model ligand (TTT) is included in the bioactive set with bioactivity rank 1 and docking rank 2, as shown in Figure 7C. Furthermore, at 1% of the score-ordered database, only bioactive molecules were enriched and none of the decoys were recognized, resulting in an Enrichment Factor (EF 1%) of 30.0. This highlights promising enrichment power for the tool under investigation for such a target.
Figure 7D shows the docking fitness distribution of the bioactive compounds. The docking score ranges from −16.51 (best score) to 1.00 (worse score) and presented as fitness values of 16.51 to −1.00 in Figure 7D. Also, the majority of cluster 3 compounds lie in the superior region of fitness (i.e., fitness >12). Such superior scores can be attributed mainly to the fact that the naphthyl substructures of their docking poses are involved in hydrophobic interactions and packed between the side chain of the key residue Tyr268 and the side chains of Pro247 and Pro248, as seen in e.g., Figures 7B,C.
Visualizing the benchmarking results for the experimental X-ray co-crystal structure (e.g., PDB ID: 6WUU), Figure 8 displays the pROC-Chemotype plot using FRED docking. Unlike the high value of EF 1% for the SARS-CoV2 model (Figure 7), the screening performance of the X-ray co-crystal structure did not enrich any bioactive compounds at 1% of the database. Unlike the best enriched bioactive compounds for the SARS-CoV2 model (Figures 7B,C), the best enriched bioactive (Figure 8B) appeared to lose some contacts with the side chains of the key Tyr268 and Gln269 where their side chains appear to be solvent-exposed and directed outward.
Figure 8. (A) pROC-Chemotype plot of SARS-CoV-2 PLpro (PDB ID: 6WUU) using the FRED docking tool. The docking information is matched with the chemotype represented by the cluster number and the bioactivity information. (B) The best enriched bioactive compound in the binding site of the protein. (C) Box plot of the fitness vs. chemotype clusters illustrating the bioactive molecules distribution.
Furthermore, the docking fitness distribution of the bioactive compounds in this case is narrower with inferior score range compared to the model performance. For instance, the docking score ranges from −9.89 (best score) to −6.23 (worst score) and presented as fitness values of 9.89–6.23 in Figure 8C. In this case, molecules of cluster 3 did not gain significant advantage since side chains of the key residues Tyr268 and Gln269 are not likely able to optimally interact with their naphthyl substructures.
The pROC-Chemotype plot of the recently introduced X-ray SARS-CoV2 complexed with TTT (PDB ID: 7JRN) for FRED docking displayed comparable results to the homology model, as seen in Figure 9. Both protein structures exhibited similar pROC-AUC and EF 1% values. Additionally, similar bioactive molecules (6 out of 7 molecules) were enriched at EF 1% for both protein structures. Also, the best two bioactive compounds in the bioactive set (i.e., with bioactivity rank 1 and 2) exhibited similar poses in the binding site (Figures 9B,C) compared to their respective poses in the homology model (Figures 7C,B). The docking fitness distribution of the bioactive compounds in this case (Figure 9D) appeared to be related to their distribution in the case of the homology model (Figure 7D). Generally, such behavior is not surprising since both the X-ray SARS-CoV2 PLpro (PDB ID: 7JRN) and the model protein structures exhibit similar conformations for the key residues of the binding site, as discussed earlier (see Figure 5).
Figure 9. (A) pROC-Chemotype plot of the recently introduced SARS-CoV-2 PLpro (PDB ID: 7JRN) using the FRED docking tool. The docking information is matched with the chemotype represented by the cluster number and the bioactivity information. (B,C) The docking poses of the two best bioactive molecules (i.e., bioactivity rank 1 and 2) overlaid on the co-crystal ligand as salmon and cyan sticks, respectively. (D) Box plot of the fitness vs. chemotype clusters illustrating the bioactive molecule distribution.
These promising benchmarking outcomes encouraged us to employ FRED in a virtual screening campaign to screen the FDA-approved drugs from the DrugBank (Wishart et al., 2018) database against SARS-CoV-2 PLpro. We used the homology model, the X-ray co-crystal structure with a peptide inhibitor (PDB ID: 6WUU), as well as the recently introduced X-ray structure complexed with TTT (PDB ID: 7JRN). We utilized these three structures as an approach to target diverse conformations of the ligand-bound state of the binding site and to extract consensus ranking of the screened drugs. The results of the best enriched 1% of the DrugBank database are shown in Table 1.
Table 1. The best-ranked 1% of the VS efforts for FDA-approved drugs (DrugBank—release March 2020) against the SARS-CoV-2 PLpro homology model, the co-crystal structure (PDB ID: 6WUU), and the recently introduced co-crystal structure (PDB ID: 7JRN) for (A–C), respectively.
As a consensus, both the model and the X-ray structure complexed with TTT (PDB ID: 7JRN) enriched similar 10 out of 25 drugs at 1% of the DrugBank database, as seen in Tables 1A,C. However, only 2 drugs out of 25 drugs were enriched together for the model and the X-ray structure complexed with peptide-like inhibitor (PDB ID: 6WUU), as shown in Tables 1A,B. Interestingly, as a consensus for all the three SARS-CoV2 PLpro structures, two drugs appeared to be commonly enriched, namely: Benserazide and Midodrine. However, the latter is in its prodrug form and therefore is not considered in our investigation.
It is worth mentioning that Perphenazine, Benserazide, and Isocarboxazid appeared to be the best-ranked drugs for the three SARS-CoV2 PLpro structures: the model, PDB ID: 6WUU and PDB ID: 7JRN, respectively.
Elucidating the postulated binding interactions of a consensus binder from the DrugBank to the three PLpro protein structures, Figure 10 shows the binding pose of Benserazide in the binding site of the X-ray SARS-CoV2 PLpro structure (PDB ID: 7JRN). Benserazide is a decarboxylase inhibitor usually combined with levodopa to treat Parkinson's disease. Also, benserazide has been conferred by European Medicines Agency as an orphan designation since 2015 for its potential to be used as a therapy for beta thalassaemia. It was marketed since 1977 by Hoffmann La Roche. Its postulated binding pose in the SARS-CoV-2 PLpro binding site exhibited H-bonding interactions via its hydrazide group with side chains of Asp164 and the key residue Gln296, as seen in Figure 10. Also, its trihydroxy phenyl group appeared to be packed in the hydrophobic cleft (green surface in Figure 10A) formed by the key residue Tyr268 with residues Pro247 and Pro248. It is worthy to mention that this binding pose of Benserazide is reproduced for the homology model, while differences were observed for 6WUU (data not shown). Again, this is not surprising due to the high similarity of key residues conformations between the model and 7JRN.
Figure 10. Docking pose of benserazide as cyan sticks in the binding site of SARS-CoV-2 PLpro (PDB ID: 7JRN) in three- and two- dimensional depictions for (A,B), respectively. Polar and non-polar regions of the binding site were presented by red- and green-colored molecular surfaces, respectively. Dashed lines indicate favorable interactions. Non-polar hydrogen atoms were omitted for clarity.
MSA and protein structure superposition revealed high sequence identity between SARS-CoV PLpro and SARS-CoV-2 PLpro with 82.9 and 100% identity for the binding site. The key residues Tyr269 and Gln270 of the binding site of SARS-CoV PLpro for small-molecule recognition are also present in SARS-CoV-2 PLpro. This encouraged us to use the reported small-molecule binders to SARS-CoV PLpro to generate a high-quality DEKOIS 2.0 benchmark set. Accordingly, we performed a cross-benchmarking study using the SARS-CoV PLpro benchmark set against SARS-CoV-2 PLpro. There is no reported co-crystal structure of SARS-CoV-2 PLpro with the conventional small-molecule inhibitor; hence, there is a lack of information for the binding site in a ligand-protein conformation. Thus, we built a homology model for SARS-CoV-2 PLpro complexed with a small-molecule ligand for benchmarking and docking purposes. Three publicly available docking tools were employed in the benchmarking study against the model, FRED, AutoDock Vina, and PLANTS. All showed better-than-random performances with pROC-AUC values of 2.34 for FRED, compared to pROC-AUC values of 1.35 and 0.98 for AutoDock Vina and PLANTS, respectively. Visualizing the FRED performance via the pROC-Chemotype plot emphasizes that this docking tool can enrich the best bioactivity in the early docking rank. Cross-benchmarking against the X-ray co-crystal structure with a peptide-like inhibitor (PDB ID: 6WUU) confirmed that FRED is the best-performing tool. Furthermore, we performed cross-benchmarking against the recently introduced X-ray structure complexed with a small-molecule ligand (PDB ID: 7JRN). Interestingly, its screening performance and chemotype enrichment were comparable to the built model signifying the high quality of the built model. This encourages us to employ FRED in a VS campaign using the FDA-reported drugs (from DrugBank) against SARS-CoV-2 PLpro. In general, this study offers an example of how to employ a DEKOIS 2.0 benchmark set against a vital target of SARS-CoV-2. This can help improve the success rate for many virtual screening campaigns against the rapidly resolved protein structures of SARS-CoV-2, for fighting the quickly emerging COVID-19.
The protein sequences of SADS, MERS, SARS-CoV-2, and SARS-CoV PLpro were retrieved as FASTA format from the Protein Data Bank (PDB) using the PDB IDs: 6L5T, 5W8U, 6W9C, and 2FE8, respectively. The multiple sequence alignment is performed using Clustal Omega (Sievers et al., 2011) and presented by ESpript v3.0 (Robert and Gouet, 2014) web server.
SWISS-MODEL (Waterhouse et al., 2018) web server is used to build a homology model for the small-molecule-bound conformation of SARS-CoV-2 PLpro using its automated mode. The template (PDB ID: 3E9S, chain A for SARS-CoV PLpro) was the best recommended for ligand-bound conformation via quality estimate metrics of SWISS-MODEL (Benkert et al., 2011; Waterhouse et al., 2018). The template X-ray crystal structure is with 2.5 Å resolution and R-value free of 0.261. The small-molecule co-crystal ligand is with chemical name “5-amino-2-methyl-N-[(1R)-1-naphthalen-1-ylethyl]benzamide” and involved in the bioactive set for benchmarking with bioactivity rank 1 and IC50 value 230 nM (Lee et al., 2015). This small molecule is included in the built homology model. The Ramachandran plot of SWISS-MODEL was used to test the validity of the model. Furthermore, the structure analysis and verification server (SAVES, 2020) of the University of California Los Angles (UCLA) is used to assess the model, using PROCHECK (Laskowski et al., 1993), Verify 3D (Bowie et al., 1991), PROVE (Pontius et al., 1996), and ERRAT (Colovos and Yeates, 1993).
Molecular Operating Environment (MOE) was used to prepare the protein structures for docking experiments, including (i) the homology model complex of SARS-CoV-2 PLpro, (ii) the apo forms of SARS-CoV-2 PLpro (PDB ID: 6W9C) and SARS-CoV PLpro (PDB ID: 2FE8), (iii) the co-crystal structure of SARS-CoV PLpro (PDB ID: 3E9S), (iv) the co-crystal structure SARS-CoV2 PLpro (PDB ID: 6WUU), and (v) the recently introduced co-crystal structure SARS-CoV2 PLpro (PDB ID: 7JRN). Module “Quickprep” of MOE was used at default settings after removing the redundant chains, irrelevant ions, molecules of crystallization, and solvent atoms (if any). Briefly, these settings include using the “Protonate 3D” function to optimize the H-bonding network and allow ASN/GLN/HIS to flip during protonation. Also, these settings involve refining the ligand and binding site atoms via energy minimization to an RMS gradient of 0.1 kcal/mol/A, while a force constant (strength = 10) was applied for the restraints of receptor atoms. The rest of the receptor atoms outside the binding site were kept fixed. These settings produced a non-significant change of the binding site/ligand coordinates. Also, none of the HIS residues were inspected in the binding site which can be affected by certain protonation/tautomerization state. Conformations of GLN and ASN can be depicted in the respective Figures (in the Results and Discussion section) of the binding site. The prepared structures were saved as mol2 for docking experiments.
The DEKOIS 2.0 (Bauer et al., 2013) protocol was applied on 24 SARS-CoV PLpro bioactives, which were extracted from BindingDB, to generate 720 challenging decoys (1:30 ratio). Then, all molecules were prepared by MOE with comparable settings to the previous report (Bekhit et al., 2019). Only one conformer was retrieved, and one protonation state was generated at pH 7.0 for each molecule. The specified stereo configuration of all bioactives, decoys, and DrugBank molecules was retained. All prepared molecules were saved as SD files. The SD files were converted and split into PDBQT files by OpenBabel (O'Boyle et al., 2011) for AutoDock Vina docking experiments and into mol2 files for PLANTS docking experiments.
For AutoDock Vina (version 1.1.2) (Trott and Olson, 2010) docking, the protein files were converted to PDBQT files by employing a python script (prepare_receptor4.py) provided by the MGLTools package (version 1.5.4) (Sanner, 1999). The search efficiency of the docking algorithm was kept at default level, while the size of the docking grid was 22.5 Å × 22.5 Å × 22.5 Å, with a grid spacing of 1 Å to make sure to cover all geometries of the docked compounds. For PLANTS (Korb et al., 2009) docking, the scoring function used was “ChemPLP,” with the “screen” mode selected. The binding site was defined within 5 Å of the coordinates of the complexed ligand, and the apo structures were superposed on the complexed ones to extract similar binding site surroundings. For the OEDocking v3.2.0.2 docking (McGann, 2011, 2012), the FRED docking module (McGann, 2011, 2012) was used at default settings. MakeReceptor GUI of OpenEye was used to define the binding site as a search box around the complexed ligand with 19.69 Å × 16 Å × 15.67 Å dimensions.
The docking rank was used in calculating the pROC-AUC employing “R-Snippet” component of KNIME (Berthold et al., 2007) according to the following equation (Clark and Webster-Clark, 2008):
where n is the number of bioactives and Di is the fraction of decoys ranked higher than the ith bioactive found.
The pROC-Chemotype plots were generated by the “pROC-Chemotype plot” tool which is available in http://www.dekois.com/ (Ibrahim et al., 2014, 2015b).
Protein structure Figures were rendered using Pymol2 and MOE.
The SARS-CoV PLpro active and decoy sets (DEKOIS 2.0 set) can be found in the Supplementary Material. The rest of raw data supporting the conclusions of this article will be made available by the authors, without undue reservation.
TI designed the experiments. TI and MI carried out all experiments. All authors have given approval to the final version of the manuscript and agree to be accountable for the content of the work.
MB was employed by the company AstraZeneca (Cambridge, UK).
The remaining authors declare that the research was conducted in the absence of any commercial or financial relationships that could be construed as a potential conflict of interest.
We thank Marina M. Habashy, Noha Galal, Reem Ghazal, and Mohammed A. Elalmawy, undergraduate students in the Faculty of Pharmacy, Kafrelsheikh University, for their literature evaluations and discussions about COVID-19.
The Supplementary Material for this article can be found online at: https://www.frontiersin.org/articles/10.3389/fchem.2020.592289/full#supplementary-material
Supplementary Table 1. The active set of SARS-CoV PLpro.
Supplementary Table 2. The challenging DEKOIS 2.0 decoy set.
WHO, World Health Organization; COVID-19, corona virus disease 19; SARS-CoV-2, severe acute respiratory syndrome coronavirus 2; ssRNA, single stranded RNA genome; MERS-CoV, middle east respiratory syndrome coronavirus; PLpro, papain-like protease; 3CLpro, 3C-like protease; Mpro, main protease; DEKOIS, Demanding Evaluation Kits for Objective In silico Screening; VS, virtual screening.
2. ^The PyMOL Molecular Graphics System, Schrödinger, LLC.
Baez-Santos, Y. M., Barraza, S. J., Wilson, M. W., Agius, M. P., Mielech, A. M., Davis, N. M., et al. (2014). X-ray structural and biological evaluation of a series of potent and highly selective inhibitors of human coronavirus papain-like proteases. J. Med. Chem. 57, 2393–2412. doi: 10.1021/jm401712t
Báez-Santos, Y. M., John, S. E. S., and Mesecar, A. D. (2015). The SARS-coronavirus papain-like protease: structure, function and inhibition by designed antiviral compounds. Antiviral Res. 115, 21–38. doi: 10.1016/j.antiviral.2014.12.015
Barretto, N., Jukneliene, D., Ratia, K., Chen, Z., Mesecar, A. D., and Baker, S. C. (2005). The papain-like protease of severe acute respiratory syndrome coronavirus has deubiquitinating activity. J. Virol. 79, 15189–15198. doi: 10.1128/JVI.79.24.15189-15198.2005
Bauer, M. R., Ibrahim, T. M., Vogel, S. M., and Boeckler, F. M. (2013). Evaluation and optimization of virtual screening workflows with DEKOIS 2.0–a public library of challenging docking benchmark sets. J. Chem. Inf. Model. 53, 1447–1462. doi: 10.1021/ci400115b
Bekes, M., van der Heden van Noort, G. J., Ekkebus, R., Ovaa, H., Huang, T. T., and Lima, C. D. (2016). Recognition of Lys48-linked Di-ubiquitin and deubiquitinating activities of the SARS coronavirus papain-like protease. Mol. Cell 62, 572–585. doi: 10.1016/j.molcel.2016.04.016
Bekhit, A. A., Saudi, M. N., Hassan, A. M. M., Fahmy, S. M., Ibrahim, T. M., Ghareeb, D., et al. (2019). Synthesis, in silico experiments and biological evaluation of 1,3,4-trisubstituted pyrazole derivatives as antimalarial agents. Eur. J. Med. Chem. 163, 353–366. doi: 10.1016/j.ejmech.2018.11.067
Benkert, P., Biasini, M., and Schwede, T. (2011). Toward the estimation of the absolute quality of individual protein structure models. Bioinformatics 27, 343–350. doi: 10.1093/bioinformatics/btq662
Berthold, M. R., Cebron, N., Dill, F., Gabriel, T. R., Kötter, T., Meinl, T., et al. (2007). KNIME: The Konstanz Information Miner. Studies in Classification, Data Analysis, and Knowledge Organization (GfKL 2007). Heidelberg; Berlin: Springer-Verlag. doi: 10.1007/978-3-540-78246-9_38
Boeckler, F. M., Bauer, M. R., Ibrahim, T. M., and Vogel, S. M. (2014). Use of DEKOIS 2.0 to gain insights for virtual screening. J. Cheminform 6(Suppl.):O24. doi: 10.1186/1758-2946-6-S1-O24
Bowie, J. U., Luthy, R., and Eisenberg, D. (1991). A method to identify protein sequences that fold into a known three-dimensional structure. Science 253, 164–170. doi: 10.1126/science.1853201
Chou, C. Y., Lai, H. Y., Chen, H. Y., Cheng, S. C., Cheng, K. W., and Chou, Y. W. (2014). Structural basis for catalysis and ubiquitin recognition by the severe acute respiratory syndrome coronavirus papain-like protease. Acta Crystallogr. D Biol. Crystallogr. 70(Pt 2), 572–581. doi: 10.1107/S1399004713031040
Clark, R. D., and Webster-Clark, D. J. (2008). Managing bias in ROC curves. J. Comput. Aided Mol. Des. 22, 141–146. doi: 10.1007/s10822-008-9181-z
Clemente, V., D'Arcy, P., and Bazzaro, M. (2020). Deubiquitinating enzymes in coronaviruses and possible therapeutic opportunities for COVID-19. Int. J. Mol. Sci. 21:3492. doi: 10.3390/ijms21103492
Colovos, C., and Yeates, T. O. (1993). Verification of protein structures: patterns of nonbonded atomic interactions. Protein Sci. 2, 1511–1519. doi: 10.1002/pro.5560020916
Daczkowski, C. M., Dzimianski, J. V., Clasman, J. R., Goodwin, O., Mesecar, A. D., and Pegan, S. D. (2017). Structural insights into the interaction of coronavirus papain-like proteases and interferon-stimulated gene product 15 from different species. J. Mol. Biol. 429, 1661–1683. doi: 10.1016/j.jmb.2017.04.011
Drosten, C., Günther, S., Preiser, W., van Der Werf, S., Brodt, H.-R., Becker, S., et al. (2003). Identification of a novel coronavirus in patients with severe acute respiratory syndrome. N Engl. Med. 348, 1967–1976. doi: 10.1056/NEJMoa030747
Fehr, A. R., and Perlman, S. (2015). “Coronaviruses: an overview of their replication and pathogenesis,” in Coronaviruses: Methods and Protocols, eds H. J. Maier, E. Bickerton and P. Britton (New York, NY: Springer New York), 1–23. doi: 10.1007/978-1-4939-2438-7_1
Freitas, B. T., Durie, I. A., Murray, J., Longo, J. E., Miller, H. C., Crich, D., et al. (2020). Characterization and noncovalent inhibition of the deubiquitinase and deISGylase activity of SARS-CoV-2 papain-like protease. ACS Infect. Dis. 6, 2099–2109. doi: 10.1021/acsinfecdis.0c00168
Ghosh, A. K., Takayama, J., Aubin, Y., Ratia, K., Chaudhuri, R., Baez, Y., et al. (2009). Structure-based design, synthesis, and biological evaluation of a series of novel and reversible inhibitors for the severe acute respiratory syndrome-coronavirus papain-like protease. J. Med. Chem. 52, 5228–5240. doi: 10.1021/jm900611t
Ghosh, A. K., Takayama, J., Rao, K. V., Ratia, K., Chaudhuri, R., Mulhearn, D. C., et al. (2010). Severe acute respiratory syndrome coronavirus papain-like novel protease inhibitors: design, synthesis, protein-ligand X-ray structure and biological evaluation. J. Med. Chem. 53, 4968–4979. doi: 10.1021/jm1004489
Hsih, W.-H., Cheng, M.-Y., Ho, M.-W., Chou, C.-H., Lin, P.-C., Chi, C.-Y., et al. (2020). Featuring COVID-19 cases via screening symptomatic patients with epidemiologic link during flu season in a medical center of central Taiwan. J. Microbiol. Immunol. Infect. 53, 459–466. doi: 10.1016/j.jmii.2020.03.008
Ibrahim, T. M., Bauer, M. R., and Boeckler, F. M. (2014). Probing the impact of protein and ligand preparation procedures on chemotype enrichment in structure-based virtual screening using DEKOIS 2.0 benchmark sets. J. Cheminform. 6(Suppl. 1):P19. doi: 10.1186/1758-2946-6-S1-P19
Ibrahim, T. M., Bauer, M. R., and Boeckler, F. M. (2015a). Applying DEKOIS 2.0 in structure-based virtual screening to probe the impact of preparation procedures and score normalization. J. Cheminform. 7:21. doi: 10.1186/s13321-015-0074-6
Ibrahim, T. M., Bauer, M. R., Dorr, A., Veyisoglu, E., and Boeckler, F. M. (2015b). pROC-chemotype plots enhance the interpretability of benchmarking results in structure-based virtual screening. J. Chem. Inf. Model. 55, 2297–2307. doi: 10.1021/acs.jcim.5b00475
Korb, O., Stutzle, T., and Exner, T. E. (2009). Empirical scoring functions for advanced protein-ligand docking with PLANTS. J. Chem. Inf. Model. 49, 84–96. doi: 10.1021/ci800298z
Laskowski, R. A., MacArthur, M. W., Moss, D. S., and Thornton, J. M. (1993). PROCHECK: a program to check the stereochemical quality of protein structures. J. Appl. Crystallogr. 26, 283–291. doi: 10.1107/S0021889892009944
Lee, H., Lei, H., Santarsiero, B. D., Gatuz, J. L., Cao, S., Rice, A. J., et al. (2015). Inhibitor recognition specificity of MERS-CoV papain-like protease may differ from that of SARS-CoV. ACS Chem. Biol. 10, 1456–1465. doi: 10.1021/cb500917m
Li, X., Geng, M., Peng, Y., Meng, L., and Lu, S. (2020a). Molecular immune pathogenesis and diagnosis of COVID-19. J. Pharm. Anal. 10, 102–108. doi: 10.1016/j.jpha.2020.03.001
Li, X., Wang, W., Zhao, X., Zai, J., Zhao, Q., Li, Y., et al. (2020b). Transmission dynamics and evolutionary history of 2019-nCoV. J. Med. Virol. 92, 501–511. doi: 10.1002/jmv.25701
Lin, M. H., Moses, D. C., Hsieh, C. H., Cheng, S. C., Chen, Y. H., Sun, C. Y., et al. (2018). Disulfiram can inhibit MERS and SARS coronavirus papain-like proteases via different modes. Antiviral Res. 150, 155–163. doi: 10.1016/j.antiviral.2017.12.015
Liu, T., Lin, Y., Wen, X., Jorissen, R. N., and Gilson, M. K. (2007). BindingDB: a web-accessible database of experimentally determined protein-ligand binding affinities. Nucleic Acids Res. 35, D198–D201. doi: 10.1093/nar/gkl999
McGann, M. (2011). FRED pose prediction and virtual screening accuracy. J. Chem. Inf. Model. 51, 578–596. doi: 10.1021/ci100436p
McGann, M. (2012). FRED and HYBRID docking performance on standardized datasets. J. Comput. Aided Mol. Des. 26, 897–906. doi: 10.1007/s10822-012-9584-8
Mielech, A. M., Deng, X., Chen, Y., Kindler, E., Wheeler, D. L., Mesecar, A. D., et al. (2015). Murine coronavirus ubiquitin-like domain is important for papain-like protease stability and viral pathogenesis. J. Virol. 89, 4907–4917. doi: 10.1128/JVI.00338-15
Molecular Operating Environment (2018). Montreal, QC: Chemical Computing Group Inc. Available online at: http://www.chemcomp.com (accessed 2019).
Mysinger, M. M., Carchia, M., Irwin, J. J., and Shoichet, B. K. (2012). Directory of useful decoys, enhanced (DUD-E): better ligands and decoys for better benchmarking. J. Med. Chem. 55, 6582–6594. doi: 10.1021/jm300687e
O'Boyle, N. M., Banck, M., James, C. A., Morley, C., Vandermeersch, T., and Hutchison, G. R. (2011). Open babel: an open chemical toolbox. J. Cheminform. 3:33. doi: 10.1186/1758-2946-3-33
Pontius, J., Richelle, J., and Wodak, S. J. (1996). Deviations from standard atomic volumes as a quality measure for protein crystal structures. J. Mol. Biol. 264, 121–136. doi: 10.1006/jmbi.1996.0628
Qian, X., Ren, R., Wang, Y., Guo, Y., Fang, J., Wu, Z.-D., et al. (2020). Fighting against the common enemy of COVID-19: a practice of building a community with a shared future for mankind. Infect. Dis. Poverty 9:34. doi: 10.1186/s40249-020-00650-1
Rabaan, A. A., Al-Ahmed, S. H., Haque, S., Sah, R., Tiwari, R., Malik, Y. S., et al. (2020). SARS-CoV-2, SARS-CoV, and MERS-CoV: a comparative overview. Infez. Med. 28, 174–184. [Epub ahead of print].
Rabi, F. A., Al Zoubi, M. S., Kasasbeh, G. A., Salameh, D. M., and Al-Nasser, A. D. (2020). SARS-CoV-2 and coronavirus disease 2019: what we know so far. Pathogens 9:231. doi: 10.3390/pathogens9030231
Ratia, K., Kilianski, A., Baez-Santos, Y. M., Baker, S. C., and Mesecar, A. (2014). Structural basis for the ubiquitin-linkage specificity and deISGylating activity of SARS-CoV papain-like protease. PLoS Pathog. 10:e1004113. doi: 10.1371/journal.ppat.1004113
Ratia, K., Pegan, S., Takayama, J., Sleeman, K., Coughlin, M., Baliji, S., et al. (2008). A noncovalent class of papain-like protease/deubiquitinase inhibitors blocks SARS virus replication. Proc. Natl. Acad. Sci. U.S.A. 105, 16119–16124. doi: 10.1073/pnas.0805240105
Ratia, K., Saikatendu, K. S., Santarsiero, B. D., Barretto, N., Baker, S. C., Stevens, R. C., et al. (2006). Severe acute respiratory syndrome coronavirus papain-like protease: structure of a viral deubiquitinating enzyme. Proc. Natl. Acad. Sci. U.S.A. 103, 5717–5722. doi: 10.1073/pnas.0510851103
Robert, X., and Gouet, P. (2014). Deciphering key features in protein structures with the new ENDscript server. Nucleic Acids Res. 42, W320–W324. doi: 10.1093/nar/gku316
Sanner, M. F. (1999). Python: a programming language for software integration and development. J. Mol. Graph. Model. 17, 57–61.
Santiago, D. N., Pevzner, Y., Durand, A. A., Tran, M., Scheerer, R. R., Daniel, K., et al. (2012). Virtual target screening: validation using kinase inhibitors. J. Chem. Inf. Model. 52, 2192–2203. doi: 10.1021/ci300073m
SAVES (2020). Structural Analysis and Verification Server Website. SAVES v5.0. Available online at: https://servicesn.mbi.ucla.edu/SAVES/.
Schapira, M., Abagyan, R., and Totrov, M. (2003). Nuclear hormone receptor targeted virtual screening. J. Med. Chem. 46, 3045–3059. doi: 10.1021/jm0300173
Schneider, G. (2010). Virtual screening: an endless staircase? Nat. Rev. Drug Discov. 9, 273–276. doi: 10.1038/nrd3139
Scior, T., Bender, A., Tresadern, G., Medina-Franco, J. L., Martínez-Mayorga, K., Langer, T., et al. (2012). Recognizing pitfalls in virtual screening: a critical review. J. Chem. Inf. Model. 52, 867–881. doi: 10.1021/ci200528d
Sievers, F., Wilm, A., Dineen, D., Gibson, T. J., Karplus, K., Li, W., et al. (2011). Fast, scalable generation of high-quality protein multiple sequence alignments using clustal Omega. Mol. Syst. Biol. 7:539. doi: 10.1038/msb.2011.75
Tilocca, B., Soggiu, A., Sanguinetti, M., Musella, V., Britti, D., Bonizzi, L., et al. (2020). Comparative computational analysis of SARS-CoV-2 nucleocapsid protein epitopes in taxonomically related coronaviruses. Microbes Infect. 22, 188–194. doi: 10.1016/j.micinf.2020.04.002
Trott, O., and Olson, A. J. (2010). AutoDock Vina: improving the speed and accuracy of docking with a new scoring function, efficient optimization, and multithreading. J. Comput. Chem. 31, 455–461. doi: 10.1002/jcc.21334
Vogel, S. M., Bauer, M. R., and Boeckler, F. M. (2011). DEKOIS: demanding evaluation kits for objective in silico screening–a versatile tool for benchmarking docking programs and scoring functions. J. Chem. Inf. Model. 51, 2650–2665. doi: 10.1021/ci2001549
Waterhouse, A., Bertoni, M., Bienert, S., Studer, G., Tauriello, G., Gumienny, R., et al. (2018). SWISS-MODEL: homology modelling of protein structures and complexes. Nucleic Acids Res. 46, W296–W303. doi: 10.1093/nar/gky427
Wei, B. Q., Baase, W. A., Weaver, L. H., Matthews, B. W., and Shoichet, B. K. (2002). A model binding site for testing scoring functions in molecular docking. J. Mol. Biol. 322, 339–355. doi: 10.1016/S0022-2836(02)00777-5
Wishart, D. S., Feunang, Y. D., Guo, A. C., Lo, E. J., Marcu, A., Grant, J. R., et al. (2018). DrugBank 5.0: a major update to the DrugBank database for 2018. Nucleic Acids Res. 46, D1074–D1082. doi: 10.1093/nar/gkx1037
Yang, D., and Leibowitz, J. L. (2015). The structure and functions of coronavirus genomic 3′ and 5′ ends. Virus Res. 206, 120–133. doi: 10.1016/j.virusres.2015.02.025
Zaki, A. M., Van Boheemen, S., Bestebroer, T. M., Osterhaus, A. D., and Fouchier, R. A. (2012). Isolation of a novel coronavirus from a man with pneumonia in Saudi Arabia. N Engl. J. Med. 367, 1814–1820. doi: 10.1056/NEJMoa1211721
Keywords: COVID-19, docking, VS, benchmarking, DEKOIS 2.0, PLpro
Citation: Ibrahim TM, Ismail MI, Bauer MR, Bekhit AA and Boeckler FM (2020) Supporting SARS-CoV-2 Papain-Like Protease Drug Discovery: In silico Methods and Benchmarking. Front. Chem. 8:592289. doi: 10.3389/fchem.2020.592289
Received: 10 August 2020; Accepted: 29 September 2020;
Published: 05 November 2020.
Edited by:
Domenica Capasso, University of Naples Federico II, ItalyReviewed by:
Ramar Vanajothi, Bharathidasan University, IndiaCopyright © 2020 Ibrahim, Ismail, Bauer, Bekhit and Boeckler. This is an open-access article distributed under the terms of the Creative Commons Attribution License (CC BY). The use, distribution or reproduction in other forums is permitted, provided the original author(s) and the copyright owner(s) are credited and that the original publication in this journal is cited, in accordance with accepted academic practice. No use, distribution or reproduction is permitted which does not comply with these terms.
*Correspondence: Tamer M. Ibrahim, dGFtZXJfbW9oYW1hZEBwaGFybS5rZnMuZWR1LmVn; dGFtZXIuaWJyYWhpbTJAZ21haWwuY29t
Disclaimer: All claims expressed in this article are solely those of the authors and do not necessarily represent those of their affiliated organizations, or those of the publisher, the editors and the reviewers. Any product that may be evaluated in this article or claim that may be made by its manufacturer is not guaranteed or endorsed by the publisher.
Research integrity at Frontiers
Learn more about the work of our research integrity team to safeguard the quality of each article we publish.