- 1Key Laboratory of Big Data Mining and Precision Drug Design of Guangdong Medical University, Research Platform Service Management Center, Dongguan, China
- 2Key Laboratory of Pesticide & Chemical Biology, Ministry of Education, College of Chemistry, Central China Normal University, Wuhan, China
- 3The Second School of Clinical Medicine, Guangdong Medical University, Dongguan, China
- 4Key Laboratory for Research and Development of Natural Drugs of Guangdong Province, School of Pharmacy, Guangdong Medical University, Dongguan, China
- 5Marine Biomedical Research Institute of Guangdong Zhanjiang, Zhanjiang, China
Because undesirable pharmacokinetics and toxicity are significant reasons for the failure of drug development in the costly late stage, it has been widely recognized that drug ADMET properties should be considered as early as possible to reduce failure rates in the clinical phase of drug discovery. Concurrently, drug recalls have become increasingly common in recent years, prompting pharmaceutical companies to increase attention toward the safety evaluation of preclinical drugs. In vitro and in vivo drug evaluation techniques are currently more mature in preclinical applications, but these technologies are costly. In recent years, with the rapid development of computer science, in silico technology has been widely used to evaluate the relevant properties of drugs in the preclinical stage and has produced many software programs and in silico models, further promoting the study of ADMET in vitro. In this review, we first introduce the two ADMET prediction categories (molecular modeling and data modeling). Then, we perform a systematic classification and description of the databases and software commonly used for ADMET prediction. We focus on some widely studied ADMT properties as well as PBPK simulation, and we list some applications that are related to the prediction categories and web tools. Finally, we discuss challenges and limitations in the preclinical area and propose some suggestions and prospects for the future.
Introduction
Drug development is a complicated, risky, and time-consuming process that can be divided into several stages, including disease-related genomics, target identification and validation, lead discovery and optimization, preclinical studies, and clinical trials (Tang et al., 2006) (Figure 1). During early drug discovery, the activities and specificities of candidate drugs are usually assessed at an early stage, and pharmacokinetics and toxicities are evaluated at a relatively late stage (Selick et al., 2002). However, the undesirable efficacy and safety, mainly caused by absorption, distribution, metabolism, excretion, and toxicity (ADMET) characteristics, resulted in the failure of many candidate drugs in the final stage (Caldwell et al., 2009). Cook et al. (2014) comprehensively reviewed the results of AstraZeneca's small-molecule drug projects from 2005 to 2010 based on a longitudinal study. They found that unacceptable safety and toxicity were the most important reasons for the failure of more than half of all project closures. As with the development of drug discovery, it was realized that it is important to filter and optimize the ADMET properties for drugs at an early stage, which has been accepted and widely used to reduce the attrition rate in drug research and development. A “fail early, fail cheap” strategy has been employed by many pharmaceutical companies (Yu and Adedoyin, 2003). Pharmacokinetics and toxicity assessments of preclinical drugs are of great value in reducing the failure rate of new chemical entities (NCEs) in clinical trials (Kola and Landis, 2004; Yang et al., 2018b; Ferreira and Andricopulo, 2019). In recent years, in vitro and in vivo ADMET prediction methods have been widely used, but it is impractical to perform complex and expensive ADMET experiments on a large number of compounds (Cheng et al., 2013; Patel C. N. et al., 2020). Thus, an in silico strategy to predict ADMET properties has become very attractive as a cost-saving and high-throughput alternative to experimental measurement methods.
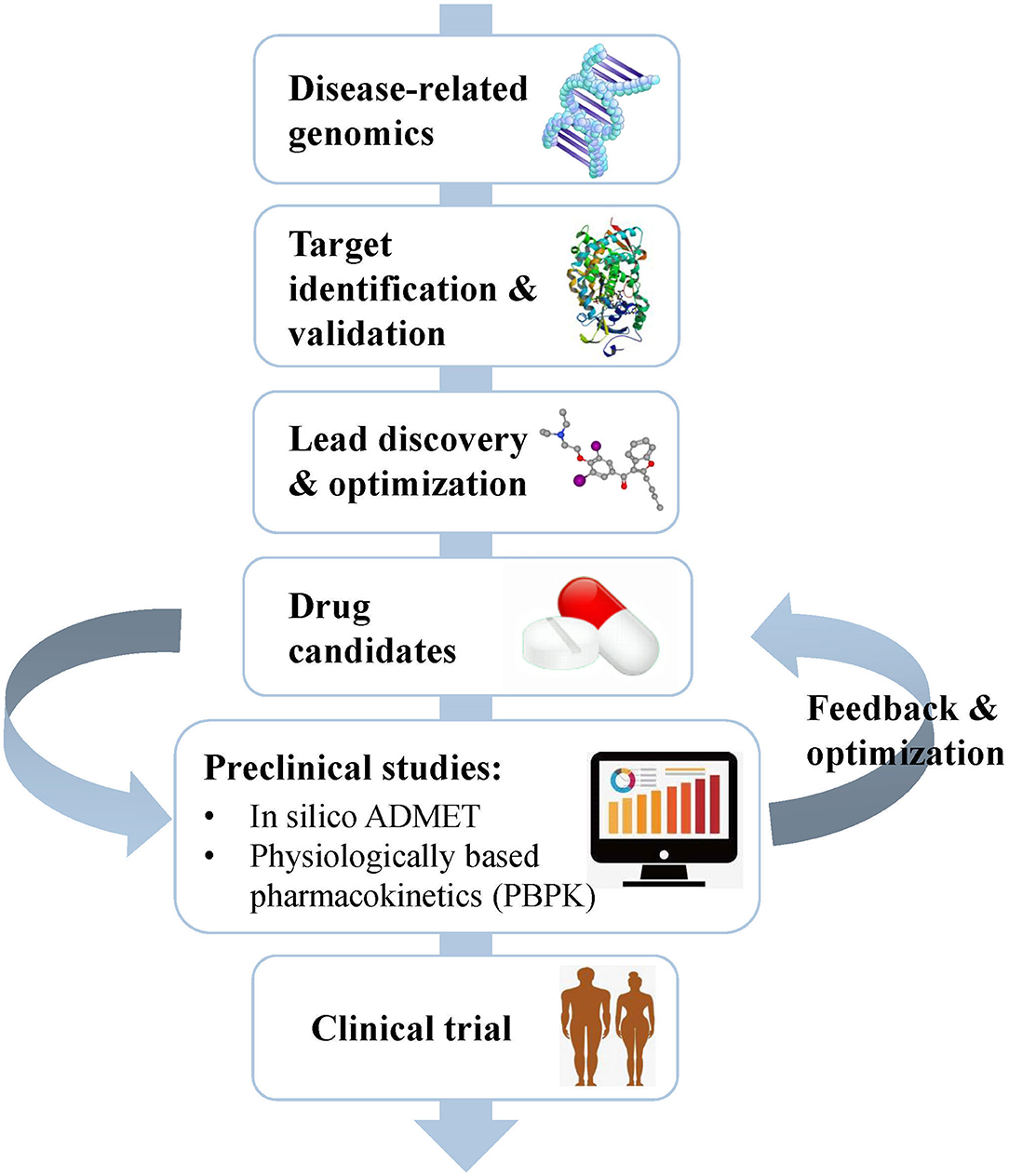
Figure 1. Schematic flow chart summarizing the process of drug discovery and the main content of the preclinical study. Preclinical studies mainly include in silico ADMET prediction and PBPK simulation, which play important roles in helping the selection and optimization of drug candidates.
With the rapid development of computer technologies, the high-throughput screening of compounds, application of combinatorial chemistry, and ability of compound synthesis have increased dramatically. The early demands for ADMET data on lead compounds have also significantly increased, and methods for evaluating ADMET in vitro are gradually increasing. Many in silico methods have been successfully applied to the in vitro prediction of ADMET, and in silico models have also been developed to replace in vivo models for the prediction of pharmacokinetics, toxicity, and other parameters (Zhu et al., 2011; Wang et al., 2015; Alqahtani, 2017). In silico ADMET prediction has progressed with the continuous development of cheminformatics and has entered the era of big data (Ferreira and Andricopulo, 2019). Two in silico approach categories can be used for ADMET prediction: molecular modeling and data modeling. Molecular modeling is based on the three-dimensional structures of proteins. It includes multiple methods such as molecular docking, molecular dynamics (MD) simulation, and quantum mechanics (QM) calculation (Bowen and Guener, 2013; Cheng et al., 2013; Silva-Junior et al., 2017). Data modeling includes quantitative structure–activity relationship (QSAR) (Cumming et al., 2013) and physiologically-based pharmacokinetic (PBPK) modeling (Fan and de Lannoy, 2014). Due to the increase in number of properties that need to be predicted, a series of ADMET software programs capable of comprehensive property prediction have been developed. The development from in silico approaches to ADMET software has undergone a long process of predicting property parameters from less to more at early to late timepoints (Figure 2). This review first provides a detailed introduction to the two in silico approaches of ADMET prediction. Then, we summarize the widely used databases and software related to ADMET prediction. Finally, we analyze the problems and challenges faced by computer model prediction methods as well as the tools, and we propose some of our own prospects for future development in this area.
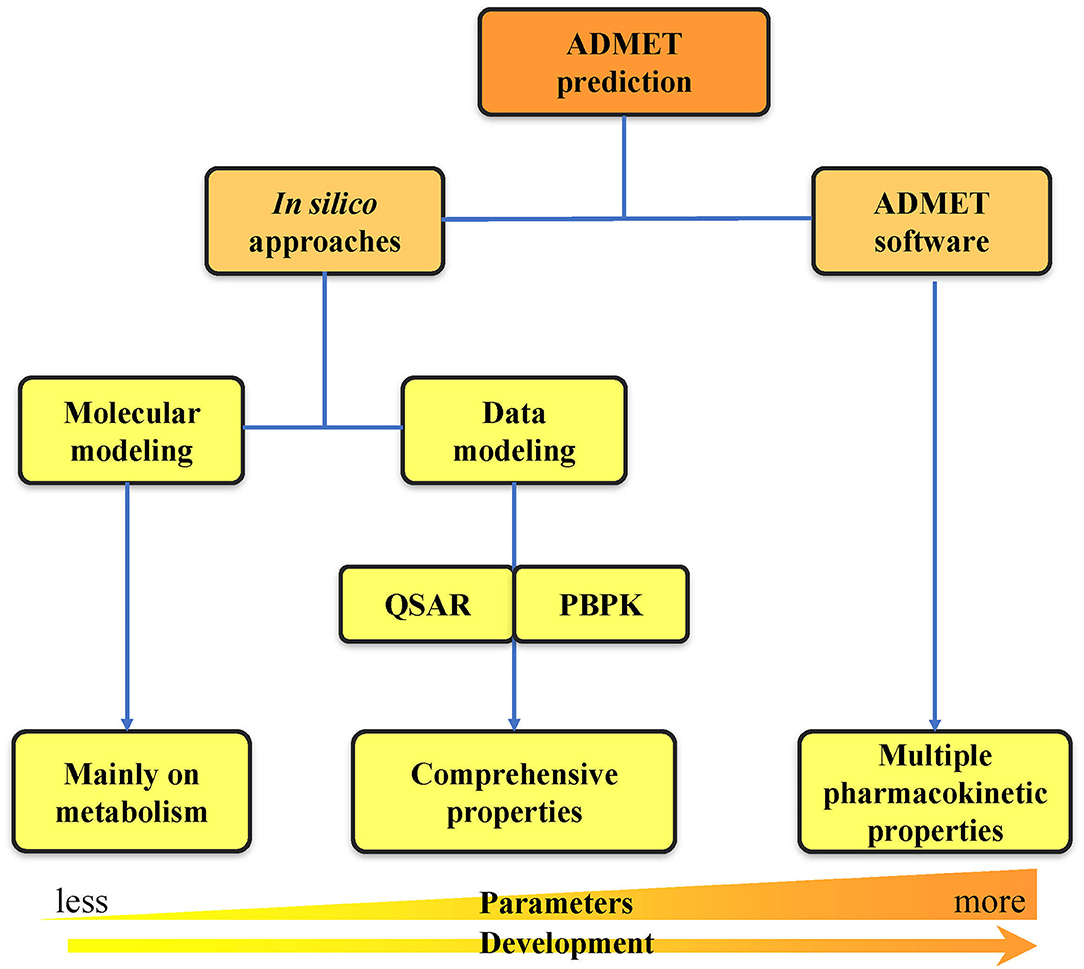
Figure 2. Classification of ADMET prediction strategies. The ADMET prediction includes the primary in silico approaches and the usage of ADMET software. The development from in silico approaches to ADMET software has undergone a long process of predicting property parameters from less to more.
In silico Approaches
Molecular Modeling
Molecular modeling, based on the three-dimensional structures of proteins, is an important category in predicting ADMET properties and includes methods such as pharmacophore modeling, molecular docking, MD simulations, and QM calculations (Figure 3). As more and more three-dimensional structures of ADMET proteins become available, molecular modeling can complement or even surpass QSAR studies (Moroy et al., 2012). Applying molecular modeling to perform ADMET prediction is a challenge because the ADMET proteins usually have flexible and large binding cavities. Many promising results of molecular modeling in predicting compound metabolism have been reported. The methods in these cases can be generally divided into ligand-based and structure-based and help not only to analyze metabolic properties but also to further optimize compound toxicity, bioavailability, and other parameters (Lin et al., 2003).
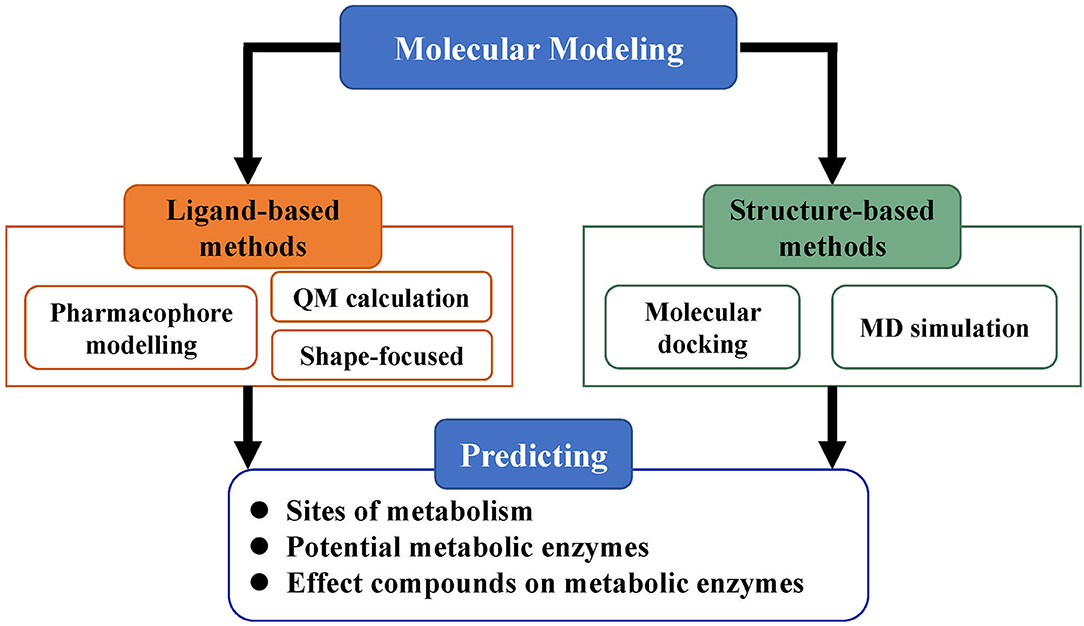
Figure 3. Strategy of molecular modeling in ADMET prediction. Molecular modeling is divided into ligand-based methods and structure-based methods and mainly used for the prediction of metabolic sites, potential metabolic enzymes, and effects of compounds on metabolic enzymes.
Ligand-Based Methods
Ligand-based methods derive information on proteins' active sites based on the shapes, electronic properties, and conformations of inhibitors, substrates or metabolites; this information depends on the assumption that the metabolic properties of compounds are entirely the result of their chemical structures and characteristics (de Groot et al., 2004; Andrade et al., 2014). In this category, pharmacophore modeling is one of the most widely used methods. The interactions between ligands and receptors can be predicted by constructing a pharmacophore model to cover the structures or properties of ligands in three-dimensional space and then to simulate the spatial and chemical properties of binding sites (de Groot, 2006). Therefore, the availability of ligand data is essential to the construction of pharmacophore models. In recent years, there have been many cases of using pharmacophore models to screen promising compounds with outstanding ADMET properties (Nandekar et al., 2016; El-Zahabi et al., 2019; Mohan et al., 2020; Patel D. B. et al., 2020; Rawat and Verma, 2020). For example, Nandekar et al. (2016) generated and validated a pharmacophore model to screen anticancer compounds acting via cytochrome P450 1A1 (CYP1A1). Nine compounds that have preferred pharmacophore characteristics and are capable of generating reactive metabolites were finally selected for further study. Rawat and Verma (2020) developed a pharmacophore model to discover new dual target inhibitors of Plasmodium falciparum dihydroorotate dehydrogenase (PfDHODH) and cytochrome bc1 complex (PfCytbc1) to treat malaria. The molecule MMV007571, which has been validated as an efficient multi-target inhibitor, was used to extract features from the binding information for the model construction. The model was used to screen a library including more than 40,000 molecules. After a series of experiments, two compounds were developed with the desired properties in binding potential and pharmacokinetic characters.
The shape-focused method is another category of ligand-based methods. This method is based on the fact that the shapes of a ligand and the binding site of its receptor should be complementary. Thus, molecules that have a comparable shape should be able to bind to the same receptor. It is more possible for a ligand to bind with the same target if it has greater similarity with the reference molecule (Putta and Beroza, 2007). This method requires only one reference molecule (shape) to perform a screening. However, more models should be constructed to cover more diverse chemical space if different shapes are available (Perez-Nueno and Ritchie, 2011), particularly for highly flexible proteins. Some studies using the shape-focused method have been reported in recent years (Reddy et al., 2013; Chen et al., 2015; Kumar et al., 2015; Prabhu and Singh, 2019). For example, Chen et al. (2015) presented a shape-based virtual screening to find new cores for the design of acetylcholinesterase (AChE) inhibitors. The shape of commercial inhibitor tacrine was used to search for new potential inhibitors. Two hit compounds were finally identified with good ADMET properties and better activity than tacrine.
With the improvement of computer hardware performance, the time-consuming QM calculation in ADMET prediction has become possible and popular. The QM calculation can be used to evaluate the bond break, which is a step required for metabolic transformation (Andrade et al., 2014). Moreover, this calculation uses an accurate means of describing electrons in atoms and molecules (Modi, 2003). Hence, QM calculation is very helpful in ADMET prediction. Extensively increasing studies involving the application of QM methods have been conducted to describe ADMET properties of new compounds (Li et al., 2012; Taxak et al., 2013; Kavitha et al., 2015; Sasahara et al., 2015; Evangelista et al., 2016; Mondal et al., 2017). The ab initio (Hartree-Fock), semiempirical (AM1 and PM3), and density functional theory (DFT) approaches are most commonly used in these studies (Silva-Junior et al., 2017). For example, Mondal et al. (2017) used the DFT method to study the absorption profile and antimicrobial activity for five sulfonamide Schiff bases. The geometries compared well with the experimental value. Sasahara et al. (2015) used the DFT method to evaluate the metabolic selectivity of antipsychotic thioridazine by CYP450 2D6. This study revealed the importance of the substrate orientation in the reaction center of this enzyme for the metabolic reaction.
All ligand-based methods need to address the problem of the uncertainty of metabolic enzyme binding sites. If reliable structural data for a metabolic enzyme are lacking, the properties of ligand binding to the enzyme can only be speculative, and minor modifications to a ligand may cause a significant decrease in ligand-protein affinity (Kirchmair et al., 2012). Therefore, it is difficult to predict ADMET using ligand-based methods without reliable protein structure data. However, the use of ligand-based methods in metabolism prediction can easily eliminate inappropriate compounds and reduce the number of compounds that fail during the synthetic evaluation cycle and more expensive late stages.
Structure-Based Methods
Structure-based methods can be used not only to predict the ADMET properties of compounds, but also to study specific interactions between small molecules and ADMET proteins. In general, they focus on obtaining binding modes from the static structures of protein-ligand complexes, regardless of time-dependent conformational fluctuations (Cheng T. et al., 2012). For structure-based methods, changes in receptor conformation must be considered mainly because of the possible interaction between the existing large and flexible binding cavities with diverse ligands. By performing MD calculation to simulate the dynamic changes of spatial shape, we may obtain an adequate conformation sampling to search a stable and reliable binding mode for ADMET prediction. In recent years, structure-based methods have been widely used, for instance, to predict the binding patterns of substrates (Macalino et al., 2015), conformational changes in enzymes (Cheng T. et al., 2012), and their catalytic effects on physiological systems (Cui and Karplus, 2003), to evaluate substrate affinity, instability and metabolic pathways (Sun and Scott, 2010), and to assess the relationship between metabolism and carcinogenicity (Fratev and Benfenati, 2008).
The molecular modeling strategy makes important contributions to the rationalization of metabolic reactions of compounds, allowing the simulation of binding modes between drugs and macromolecules in the ADMET process at atomic or molecular levels. With the rapid development of structural elucidation and pharmacokinetic calculation techniques, structure-based methods are becoming increasingly predictive and accurate. However, the molecular modeling strategy is still limited by drawbacks such as a requirement to accurately analyze the structural flexibility of proteins (Kazmi et al., 2019). Additionally, high-resolution experimental structural data of the target will be more conducive to our accurate prediction of the drug metabolic fate (Doss et al., 2014). Surely, combining improved structure-based and ligand-based methods can create synergistic effects in metabolic prediction, enabling more comprehensive descriptions of metabolic reactions (Issa et al., 2017; Kar and Leszczynski, 2017).
Data Modeling
There are two widely used data modeling methods to predict ADMET-related properties, QSAR, and PBPK modeling (Cheng et al., 2013). ADMET analysis and prediction in QSAR mainly depends on many molecular descriptors, including topological, geometrical physicochemical, or electronic descriptors. Many properties, such as blood-brain barrier (BBB), clinical adverse effects, percent protein binding (%PPB), lipophilicity (logP), preclinical toxicological endpoints, and metabolism of pharmaceutical substances, can be predicted using the QSAR method. The PBPK modeling always predicts parameters concerning the dose size and dose frequency, such as the volume of distribution at steady-state (Vss), total drug clearance (CL), and fraction of dose that reaches the portal vein (Fabs), because most drugs are taken orally.
QSAR
QSAR, which employs mathematical models to describe relationships between molecular structures and their biological activities, has been used in pharmaceutical chemistry since the 1960s (Hansch, 1981). The classical QSAR developed by Hansch was used to predict ADMET (Hansch, 1981). It is mainly based on the hypothesis that similar molecules exhibit similar properties (Patterson et al., 1996). Thus, two prediction methods are primarily considered: (1) prediction based on molecular similarity (pharmacophore- and molecular fragment-based methods) and (2) prediction based on property similarity (log P, log D, and others) (Yongye and Medina-Franco, 2013). In these two methods, the accuracy of prediction depends on the attribute characteristics in the applicability domain contained in the training set. Thus, when using a model constructed with a specific training set, prediction should be performed using compounds that have a similar structure space to those in the training set to improve the prediction accuracy of the QSAR model, since compounds with similar distribution in chemical space are more likely to exhibit similar biological activities (Huang and Fan, 2011).
QSAR is a method for using various biochemical and physical data to construct models. In QSAR studies, compounds can be mathematically codified as molecular descriptors, and the relationship between molecular descriptors and defined properties is constructed by statistical methods, after which a generated model is used to predict the corresponding properties of new compounds (Michielan and Moro, 2010). This method first transforms molecular structure into molecular descriptors, which are then used to establish prediction models by using statistical approaches or machine learning techniques such as support vector machine (SVM) and K Nearest Neighbor (kNN) (Wang S. et al., 2016; Wu et al., 2019; Yang et al., 2019; Fu et al., 2020). For example, Schyman et al. (2017) used the variable nearest neighbor (vNN) method to develop 15 ADMET prediction models and to use them to quickly assess some potential drug candidates, including toxicity, microsomal stability, mutagenicity, and likelihood of causing drug-induced liver injury. Belekar et al. (2015) developed a computational model to identify compounds as breast cancer resistance protein (BCRP) inhibitors or not by using various machine learning approaches like SVM, k-NN, and the artificial neural network (ANN). The prediction accuracy of all three approaches was over 85%. Finally, internal and external cross-validation were performed to confirm the reliability of the model before it is used on new predictions to find molecules outside the training set (Figure 4).
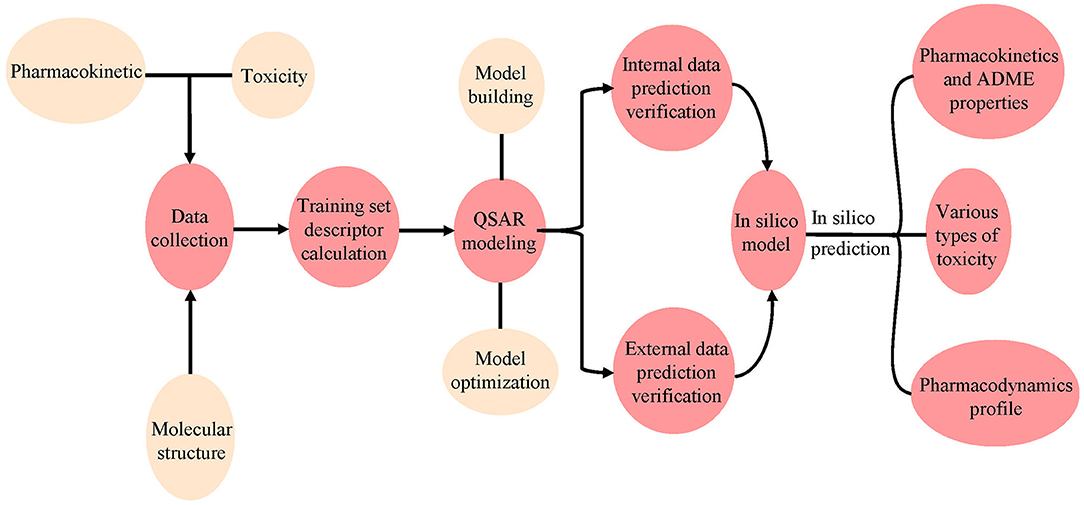
Figure 4. Workflow for the use of pharmacodynamic, pharmacokinetic, and toxicity databases and models. The molecular data were first collected from different databases, and the QSAR model was constructed using the collected or calculated molecular descriptors. Internal and external validation were then performed using the model. Finally, the validated model was used to predict ADMET properties for new chemicals.
QSAR modeling uses a large number of descriptors that allow lookups, enable structure/response associations, and help with similarity and substructure searches (Khan, 2010). Most of the available descriptors can be divided into three categories: (1) two-dimensional molecular topology information; (2) three-dimensional molecular structure; and (3) physicochemical and electronic descriptors, which are commonly used to predict ADMET-related properties (Danishuddin and Khan, 2016; Tabeshpour et al., 2018; Zhang et al., 2018). Jiang et al. (2020) developed a series of QSAR models by using 379 molecular descriptors to discriminate BCRP inhibitors. The descriptors characterized the physicochemical, two-dimensional substructures, and drug-like properties of the studied compounds. Lapins et al. (2018) constructed a QSAR model to predict the lipophilicity of compounds by using a signature molecular descriptor, which is related to the molecular two-dimensional topology information from 1.6 million compounds. Xu et al. (2017) developed three deep learning-based QSAR models to evaluate the acute oral toxicity (AOT) of compounds. The atom and bond information extracted from over 2,000 two-dimensional molecule structures were used as descriptors to construct the models. The best model achieved an external prediction accuracy over 94%, which is more efficient than traditional fingerprints or descriptors. Bujak et al. (2015) predicted the permeability of the BBB of chemical compounds using molecular energy-related descriptors in combination with the well-known lipophilicity descriptors. The data indicate that the QSAR model has important information value, and these descriptors may have supportive value in predicting the blood brain distribution (Bujak et al., 2015). Therefore, accurate prediction of ADMET parameters mainly depends on the selection of a suitable modeling method, molecular descriptors of specific ADMET endpoints, and large experimental data sets related to these endpoints. Only in this way can the ADMET properties of the candidate compounds be predicted precisely.
At present, many tools used for ADMET prediction have been developed based on QSAR methods. These tools utilize different descriptors to define the collected data, and then the mathematical model fitted from the training set is used to predict the properties. We listed three widely used QSAR-based ADEMT prediction tools and related studies herein. (1) The Danish QSAR Database (http://qsar.food.dtu.dk/) collected estimates from over 200 QSAR models from free and commercial platforms, including descriptors like ecotoxicity, environmental fate, physicochemical properties, and ADMET. Trivedi et al. (2019, 2020) used the online tool Danish QSAR database to determine the ADMET properties for potential hits for Dengue fever and H1N1 flu, respectively. Multicase acute aquatic toxicity, carcinogenicity, arylhydroxylase activity, lethal body burden, bioconcentration, mutagenicity, biodegradation, environmental partitioning, and general properties are included in the ADMET properties. Finally, 12 compounds were identified as potential leads against dengue fever and 18 compounds against H1N1 flu. (2) The OCED Toolbox (https://www.oecd.org/chemicalsafety/risk-assessment/oecd-qsar-toolbox.htm), a package for toxicity prediction, was also developed based on QSAR. Han et al. (2019) used OECD QSAR Toolbox 4.1 to predict the genotoxicity for ceftazidime (CAZ) and its impurities to improve quality control of drugs. (3) ADMET PredictorTM (https://www.simulations-plus.com/software/admetpredictor/) is another tool utilizing QSAR to predict ADMET parameters of compounds. Takac et al. (2019) used ADMET PredictorTM to investigate the potential impact and safety profile with respect to the environment and health for 25 selected entactogen molecules. The chemical structure (including 1D and 2D) information was used as the input for ADMET PredictorTM. Lipophilicity parameters, volume of distribution, jejunal permeability, solubility, and logarithm of the brain/blood partition coefficient were predicted in this case. Alarn and Khan (2019) used the ADMET PredictorTM to predict pharmacokinetics, pharmacodynamics, and toxicity parameters of flavone analogs to reveal their anticancer activity. Different physicochemical properties were calculated as descriptors to build the model, and then numerous properties were predicted, such as solubility, lipophilicity, permeability, absorption, bioavailability, BBB, transporters, plasma-protein binding, and volume of distribution.
Although the use of QSAR models has made considerable progress in ADMET prediction, these models cannot yet be used to replace in vitro or in vivo studies for all endpoints. The QSAR method is always limited by its model expansion capability, and large experimental data are always needed for model construction. The narrow data distribution may induce over fitting and lead to inaccurate prediction results. For example, Verheyen et al. (2017) estimated the QSAR models used for the prediction of eye and skin irritation/corrosion in Derek Nexus, Toxtree and Case Ultra. They found that the prediction results were unsatisfactory because of the narrow application range and low accuracy. Thus, validation and documentation for a constructed model is important prior to use.
PBPK Modeling
Most traditional models for predicting drug pharmacokinetics are empirical models. With a deeper understanding of the pharmacokinetic mechanism of drugs, PBPK models have been developed to predict PK properties (De Buck and Mackie, 2007). The PBPK model is an arithmetical model that combines drug data (e.g., drug concentration and clearance rate) and species physiology parameters to replicate the PK profile of a drug in plasma and tissues, aiming to describe in vivo drug pharmacokinetics that are related to tissue volume, administration routes, blood flow, biotransformation pathways, and interactions with tissues or organs in the body (Espie et al., 2009; Jones et al., 2015). The origin of the PBPK models can be traced back to Teorell's work in 1935. Teorell introduced a multicompartment model to simulate pharmacokinetics, which organically combined physiology and biology for the first time (Teorell, 1935; Zhao et al., 2011). Teorell's work has since attracted serious attention to the PBPK model.
In recent years, PBPK modeling has been substantially improved, making it more widely applicable for the research and development of drugs (Edginton et al., 2008; Rowland et al., 2011; Zhuang and Lu, 2016). Moreover, the increase in preclinical data, especially in vitro data, has promoted the development of PBPK models and simulations in drug discovery (Zhuang and Lu, 2016). PBPK modeling describes the physical and biological disposition of each compartment by dividing organisms into individual organs, and the most common processes are related to blood transportation and penetration, distribution between blood and organ tissue, and metabolic excretion, among others (Schmitt and Willmann, 2004). Since PBPK integrates large amounts of drug-specific data, parameters, and species physiology (systematic data), there are two kinds of parameters in PBPK models, which use the concentration-time curves of all organs and blood as output information (Nestorov, 2003). The first type consists of physiological parameters, such as tissue volume, blood flow, and cardiac output. Recently, due to the extensive application of in vitro-in vivo extrapolation (IVIVE) in PBPK, many researchers have predicted the disposal of drugs in vivo through in vitro metabolism and transport data, indicating that metabolic enzymes and transporter expression data have become important physiological parameters (Rostami-Hodjegan, 2012). The second type consists of drug-related parameters, such as the plasma ratio, organ/blood partition coefficient, and permeability (Nestorov, 2003). Recently, PBPK models have been widely constructed to predict drug-related parameters (Chow et al., 2016; Pathak et al., 2019; Song et al., 2020). For example, Chow et al. used a physiologically based model to predict drug solubility and effective permeability (Chow et al., 2016) to examine the potential impact of excipients on oral drug absorption.
Databases
In the past 10 years, with rapid development, a number of related databases storing pharmacokinetic parameters have emerged. We collected some of the most commonly used databases and classified them as ADMET-related databases and auxiliary databases. A brief introduction to these databases, including website links, data scales, and descriptions, is provided in Tables 1, 2. For the ADMET-related databases, users can submit information on the compounds they want to query through the corresponding modules. Then, shape screening or pharmacophore screening will be performed to obtain additional targets or bioactivity information on similar ligands that match the query molecule. The ADMET-related properties can also be obtained from the query result. The auxiliary databases mainly focus on providing structural information about compounds. Although some ADMET-related information is provided in the search results, it is not complete, and not every compound is associated with such information.
ADMET-Related Databases
At present, many in silico models are used to predict ADMET, but massive amounts of data are needed to build them. The quality and quantity of the data are closely related to the accuracy of model prediction, so reliable experimental data are the key to successful prediction (Dearden, 2007; Alqahtani, 2017). Currently, there are some databases that can help ADMET prediction, such as the ADME Database (Shang et al., 2017), SuperToxic (Schmidt et al., 2009), PKKB (Cao et al., 2012), and DSSTox (Williams et al., 2017). By using these databases, users can obtain helpful data sets for use in external algorithms to generate prediction models. The databases can also be used directly to perform prediction through search functions, such as the similarity search or prediction. In addition, these models can be updated as new experimental data are added to the database.
The ADME Database (https://www.fujitsu.com/jp/group/kyushu/en/solutions/industry/lifescience/admedatabase/), developed by Zagreb University and Fujitsu in 2004, is a commercial database that specializes in pharmacokinetics information. It provides comprehensive data on drug-metabolizing enzymes and drug transporters that are specific to humans. The data have been widely used in drug research and development, such as ADME prediction and drug-drug interactions. Users can search for classification, metabolic reactions, and kinetics-related information about compounds by structure or substructure. However, the database currently limits large-scale downloads of user data, as well as public dissemination of some models.
SuperToxic collects toxins from different sources (animals, plants, synthetic, etc.), compiles ~60 000 compounds with their structures, and integrates some chemical properties and commercial availability information (Schmidt et al., 2009). These compounds are classified based on their toxicity, which derives from more than 2 million measurements. The values can be used to study the relationship between chemical structures and functions of toxins for evaluating the risk of their use. Users can easily query the structure and toxicity information of all compounds with corresponding properties through a structure search, name search, or property search. SuperToxic also allows users to browse the data by choosing an alphabetic character or numbers to present all entries starting with the selection (Schmidt et al., 2009). The available CASRN or NSC numbers in the database can also be recorded. The toxicity information retrieved from the database includes the dosage, type of test (toxicity measurement, such as LD50), and cell lines or organisms that determine the toxicity (Schmidt et al., 2009). The database also integrates software packages that are widely used in modern composite database construction, such as Marvin Sketch (molecule drawing), JMol (visual inspection), and MyChem/OpenBabel (property calculation). Furthermore, SuperToxic was connected to the Protein Data Bank, UniProt, and KEGG databases to identify potential targets in biochemical pathways to search for compounds (Schmidt et al., 2009).
The EPA Distributed Structure-Searchable Toxicity (DSSTox) database, which provides a series of documented, standardized and complete structure annotated toxicity information files, can be very useful for SAR model development (Richard and Williams, 2002; Williams et al., 2017). To allow wider use of the database, DSSTox was designed to use a structure data file (SDF), a public and industry-standard import/export file format storing chemical structures and property information that can be used as input for any chemical relational database (CRD) application or converted to data tables. It is one of the best-curated public datasets available at present, and the data stored in it are regarded as a standard reference for publicly available structural toxicity-based data.
Except for the databases introduced above, some newly constructed ADMET-related databases should also be of concern, for example, Comparative Toxicogenomics Database (CTD) (Davis et al., 2019), The Toxicity Reference Database (ToxRefDB) (Watford et al., 2019), and The Chemical Effects in Biological Systems database (CEBS) (Lea et al., 2017). CTD is a powerful and public database designed to enhance understanding of how environmental exposures influence human health. It provides data on chemical–gene/protein interactions, chemical–disease and gene–disease relationships that are combined with pathway and function data to help develop hypotheses about the inherent mechanisms of diseases affected by the environment (Davis et al., 2019). ToxRefDB collects data from in vivo animal toxicity tests and provides toxicity endpoints for predictive modeling. Approximately 28,000 datasets from nearly 400 endpoints have been generated and stored. The recent update of ToxRefDB has added connections to other resources and significantly enhanced the utility of predicting toxicology (Watford et al., 2019). CEBS offers a toxicology resource that compiles individual and concise animal data from 11,000 test articles and more than 8,000 studies encompassing all available National Toxicology Program (NTP) carcinogenicity, genetic toxicity, and short-term toxicity studies. The high-quality data in CEBS is very useful for constructing a more accurate model for toxicity prediction (Lea et al., 2017). We may infer from the recent constructed databases that the quantity and quality of the data determine the quality of the model and will be our focus in the future.
Auxiliary Databases
In addition to ADMET-related databases, databases of biological activity, pathways, and side effects are important for ADMET prediction. Most of these databases are free and open to visitation, such as DrugBank (Wishart et al., 2018), PubChem (Kim et al., 2019), and ChEMBL (Gaulton et al., 2017). Although they are rarely used to predict ADMET-related properties directly, they can provide structural information to build models or be queried for information about compounds. Users can also download the predicted compound structure and use it as an input file for other software.
DrugBank, a comprehensive database, integrated thousands of well-studied drugs and drug targets with their physical, chemical, biological, and pharmaceutical data (Wishart et al., 2006, 2008). DrugBank 4.0 was further expanded to contain data on ADMET and other kinds of QSAR information (Law et al., 2013). DrugBank 5.0, the latest version, has further updated this information (Wishart et al., 2018). Users can use chemical shifts or mass-to-charge ratio (m/z) lists to search DrugBank's spectral library for approximate or exact matches. DrugBank also systematically classifies compounds into different types based on structural features and structural similarities and allows users to query it by using a simple text (Law et al., 2013).
Pubchem, a public database of small molecules with their biological properties, consists of three interconnected parts: (1) Compound, storing over 102 million unique chemical structures provided by various depositors; (2) Substance, containing more than 251 million records including complexes, extracts, mixtures, and non-characteristics; and (3) BioAssay, containing more than 1,067,000 bioassays, providing composite adjacent structures, substructures, similar structures, bioactivity data, and other search functions (Kim et al., 2019).
ChEMBL is an open data database containing two-dimensional structures, calculated properties (molecular weight, lipophilicity, etc.) and abstract biological activities (pharmacology and ADMET data) for numerous drug-like bioactive compounds (Gaulton et al., 2017). It is composed of three different datasets that were originally developed by Inpharmatica, including StARlite, CandiStore, and DrugStore (Overington, 2009). The data in ChEMBL were extracted from the scientific literature and designed to meet the needs of users to intelligently cluster relevant information and integrate data across therapeutic studies and areas.
Software
Favorable ADMET characteristics are important as early requirements for drug candidates to reduce late failure and cost. However, many ADMET properties are highly dependent on each other, so they need to be optimized simultaneously in preclinical studies on drug discovery and development. Nevertheless, concurrent optimization of multiparameter ADMET is the most difficult and least attractive stage. As a result, early prediction of ADMET involved only some simple properties, such as logP, logD, and logS. With increasing experimental data, an increasing number of in silico models were developed to predict more complex ADMET parameters, such as the human intestinal absorption rate, oral bioavailability, blood-brain barrier permeation rate, Caco-2 permeability, human intestinal absorption, drug interactions, P-glycoprotein, plasma protein binding rate, CYP metabolic enzymes, and kidney clearance (Pires et al., 2015; Dong et al., 2018) (Figure 5). People have also attempted to integrate these models to predict ADMET parameters concurrently, and many studies have described these in silico models and their predicted properties (Dickins and van de Waterbeemd, 2004; Wang et al., 2015). In addition, software integrating these models to predict ADMET parameters concurrently has been developed. Tables 3, 4 list some of these software packages (free and commercial) with their functions. We also compared five commonly used software in Table 5 with each other to visualize their detailed functions.
SwissADME is a hybrid web server that was developed by the Swiss Institute of Bioinformatics (Daina et al., 2017). It supports diverse input formats and can predict and analyze the ADME properties of numerous compounds in batches submitted from all over the world. This software outputs different types of physicochemical properties of drugs: water solubility, lipophilicity, physicochemical properties, druglikeness, pharmacokinetics, and medicinal chemistry, which can be directly exported and saved as a data file in CSV (comma-separated values) format and read by programs such as WordPad and Excel (Daina et al., 2017). In addition, it supplies a bioavailability radar map to quickly and intuitively evaluate the druglikeness of small molecules, facilitating its use for non-experts without professional knowledge (Daina et al., 2017). The server uses a variety of rules to evaluate the same property and provides the evaluation criteria and basis for most of the predicted data. However, in the prediction of whether the compound is a substrate or an inhibitor of the CYP enzyme, only a propensity is output, rather than a probability output similar to that of admetSAR. SwissADME also supplies a link for the one-click submission of the queried molecules to other servers in the Swiss series for further analysis (Daina et al., 2017).
Although in vivo toxicology is still the gold standard for identifying drug side effects, it is now believed that this method will not help reduce the large consumption rate in late clinical development (Merlot, 2010). Many computational tools have been developed to predict drug toxicity, helping to decrease the attrition rate of molecular compounds in drug discovery and reduce drug development time and cost. In recent years, the predictive power of these toxicology prediction systems has tremendously improved, covering more complex toxicological endpoints, such as hepatotoxicity, teratogenicity, nephrotoxicity, and carcinogenicity (Muster et al., 2008; Yang et al., 2018b). Currently, many commercial and free web-based toxicity predictors are available, such as Lazar (Maunz et al., 2013) and Toxtree (Patlewicz et al., 2008; Bhhatarai et al., 2016).
Lazar, developed by in silico toxicology GMBH, is a tool based on OpenTox (an integrated interface for an interoperable prognostic toxicology framework) to predict toxicological endpoints such as carcinogenicity, reproductive toxicity, and long-term toxicity (Hardy et al., 2010). It uses data mining algorithms to predict the toxicity of new compounds based on the experimental training data. Data sets with chemical structure and biological activity can be used as training data. Thus, Lazar can serve as a universal predictive algorithm for any biological endpoint if sufficient experimental data are available, so users no longer need to consider chemical, biological, or toxicological expertise but derive in silico models from statistical standards (Maunz et al., 2013). Users need only to input the structure of the compound, and Lazar will search the database for a series of similar compounds and corresponding experimental data, which will be used to construct a local QSAR model. The prediction results using the model will be displayed in a graphical interface, which provides structural features and compounds similar to the query compounds and toxicity properties for each fragment (Helma, 2006; Maunz et al., 2013).
Toxtree is a free software program that was commissioned by the European Chemicals Agency (ECB), the joint research center of the European Commission (Bhatia et al., 2015). It was originally designed to enable effective development of the Cramer decision tree. The latest version of Toxtree included additional projects, such as corrosion rules, BfR/SICRET skin irritation, and the Verhaar scheme, with a total of 14 functional modules (Bhatia et al., 2015). It includes physiochemical exclusion rules and structural alert inclusion rules, which are used to categorize compounds (Bhhatarai et al., 2016). Unlike Lazar, it has no training set. Its prediction is based on structural filters, so there is no applicability domain. It handles molecular structure information by using a decision tree model for risk assessment (Bhhatarai et al., 2016). Users can access it at http://toxtree.sourceforge.net/ to predict the toxicity of structures of interest.
ADMETlab is a platform for systematic ADMET estimation based on a comprehensive collection of ADMET databases (Dong et al., 2018). The platform includes four functional modules, which are used for drug similarity assessment (based on Lipinski's rule of five and the Druglikeness model), ADMET endpoint prediction, system evaluation, and database/similarity search (Dong et al., 2018). Among them, “ADMET prediction” is the main module used; the other three are auxiliary modules. Users can query one or more compounds with the platform by using the SMILES, uploading an SDF format file, or drawing the chemical structure using the embedded JME editor. After the compound is uploaded, the platform will use multiple pharmacokinetics models built by the different integrated data sets to make extensive predictions of the ADMET properties. Model prediction results are output in an interactive data table containing predicted values and structures. The software allows batch prediction, and users can apply the “drug similarity assessment” module to filter out compounds that are unlikely to be lead compounds or drugs, achieving the purpose of preliminary screening (Dong et al., 2018). Users must select one model to acquire results for one or multiple molecules, which is proper for screening compounds at specific endpoints, and the results will provide reasonable ADMET recommendations for each endpoint. Therefore, users can perform rapid screening of ADMET properties based on these independent specific prediction models and even further deliberately optimize the chemical structures of compounds, making them more likely to become drugs (Dong et al., 2018). Considering the very large amount of collected data and numerous constructed QSPR models, ADMETlab is currently one of the most comprehensive platforms used in ADMET prediction.
AdmetSAR is a free and comprehensive tool for ADMET property prediction (Cheng F. et al., 2012). The ADMET-related property data stored in AdmetSAR were collected from published literature. AdmetSAR also includes a searchable tool called ADMET-Simulator, which combines predictive and superior QSAR models in a toolbox based on chemical informatics and can predict ~50 ADMET endpoints. AdmetSAR enables users to easily search for ADMET properties by querying CASRN, the common name, or the structure (Yang et al., 2018a). The new version of admetSAR (version 2.0) mainly focuses on in silico prediction of chemical ADMET properties (Yang et al., 2018a). More than 40 predictive models trained by state-of-the-art machine learning methods were implemented in admetSAR. Four functions were developed: (1) customizable ADMET risk filters, (2) QSAR-based ADMET property prediction, (3) toxicity prediction for environmental chemicals, and (4) environmental hazard assessment. ADMETopt (Yang et al., 2018c) is a new module added in version 2.0 for lead compound optimization according to the predicted ADMET properties.
Except for ADMET software, an increasing number of PBPK software have been developed to perform systematics of the drug process in human body. The development of PBPK software has further promoted the use of PBPK modeling methods (Bouzom and Walther, 2008; Edginton et al., 2008; Perdaems et al., 2010). PBPK software was used to build and use the PBPK models, which can be useful for the estimation of pharmacokinetic parameters during the drug development process. At present, PBPK software can be roughly divided into two categories, the user customized software and user-friendly software. A brief introduction to the two types of software is provided in Table 6, including the company/institute and website links. In Table 7, we compare the functions of the commonly used software packages for both categories, such as WinNonlin (https://www.certara.com/) and GastroPlus (https://www.simulations-plus.com/).
It was initially used in engineering and mathematics of the user customized software because the essence of PBPK modeling is mathematical modeling (Bouzom et al., 2012). Hence, the application of these software programs in PBPK modeling is a natural transformation. The user customized software requires users to write their own programs to build the PK model at the beginning stage of development. This procedure requires users to have proficient programming skills as well as expertise in the field. Recently, specific PK or PBPK modules and equation libraries, as well as visual graphical interfaces, have been added to some of these software programs (Bouzom et al., 2012). By using these software programs, users can quickly generate standard PBPK models by following existing templates that already contain standard codes and equations, greatly facilitating user operation.
The user-friendly type, customized for PBPK modeling, has a graphical interface. It requires no modeling language and programming, so it is relatively simple to operate. Originally, some such software programs were specifically modeled for predicting a specific property of the ADME process, such as absorption (GastroPlus, Tian et al., 2011) or metabolism (SimCYP, Jamei et al., 2009). These software programs gradually evolved into complex PBPK modeling tools for the entire body. Recently, the function of updated versions has become increasingly sophisticated. Now, they not only model their specific areas but also simulate the whole-body pharmacokinetic process, which is absorption, metabolism, and excretion, etc. (Li M. et al., 2017; Byun et al., 2020). These software programs can perform various tasks, such as simulation, parameter evaluation, and sensitivity analysis, simply by inputting specific drug parameters and choosing certain model options (Bouzom et al., 2012).
Applications
The in silico applications of predicting ADMET profiles in 2016-2018 were collected by searching PubMed. We analyzed the search results and briefly introduce how software programs and methods predict the properties of ADMETs.
Molecular Modeling
Most applications of molecular modeling focus on predicting the strength of the interaction between a molecule and a metabolic enzyme or transporter. For instance, Niu et al. (2016) performed docking studies of flavokawain A (FKA) and its target CYP450. FKA shows obvious inhibition of different CYP isoforms, and subsequent inhibition experiments showed that CYP3A2 was the primary isoform contributing to the metabolism of FKA. Gong et al. (2018) performed a molecular docking experiment to study the binding mode between sauchinone and CYPs. The results showed the interactions of sauchinone in the active site of CYP2B6, 2C19, 2E1, and 3A4. In addition to the above examples, the details of 22 representative studies are included in Table 8.
QSAR
Prediction of the pharmacokinetic properties of compounds using QSAR relies mainly on traditional models or software developed based on constructed data sets. Table 9 lists seven typical applications of the QSAR method. For example, Khan et al. (2016) utilized the QSAR model in ACD/I-lab to determine multiple ADMET properties (such as logS, logP, logD, BBB) for 6 compounds targeting heat-shock protein 90 (Hsp90). Then, six compounds were designed according to BBB and antiangiogenic properties. One molecule (compound 6) was observed to inhibit Hsp90 with a predicted efficiency of BBB permeation of 0.55 kcal/mol in comparison to the experimental value of 0.625 kcal/mol. Ajay Kumar et al. (2018) performed 3D-QSAR studies to filter compounds based on ADME properties by using Schrodinger. Fifty hit compounds targeted to transforming growth factor-β (TGF-β) type I were screened based on predicted ADME properties (such as BBB, logS, and Lipinski's rule of five). Seven molecules were finally selected as the lead compound for subsequent research.
PBPK
PBPK modeling has been consistently performed to predict pharmacokinetics with the help of some widely used software programs (Table 7). Seventy-four applications using three software packages are listed in Table 10. WinNonlin is one of the most widely used software programs in the prediction of pharmacokinetics. For example, Gestrich et al. (2018) used WinNonlin (v6.4) to analyze compartmental and non-compartmental gentamicin plasma concentrations vs. time. The peak drug concentrations and AUCs in young adults and older alpacas were compared, and both were significantly lower in young adults than in geriatric alpacas. The increased drug exposure and decreased clearance in geriatric alpacas created a greater risk of ADRs and/or therapeutic failure. Another software, NONMEM, is also widely used to predict the impact of drugs on the target population. It is the “gold standard” software package for analysis of population PK/PD data. For example, Polepally et al. (2018) used non-linear mixed-effects modeling in NONMEM (version 7.3) to analyze concentration-time data to estimate the effect of age on the pharmacokinetic parameters of lamotrigine (LTG). By comparing the pharmacokinetic characteristics of young adult and elderly epilepsy patients, it was concluded that the bioavailability of LTG was not affected by age (Polepally et al., 2018). However, LTG CL in the elderly was 27.2% lower than in young epilepsy patients. These findings are very useful for clinicians to offer optimal epilepsy care and support to elderly patients starting low-dose treatment (Polepally et al., 2018).
GastroPlus and SimCYP are the mainstream PBPK emulation software programs. In recent years, applications have shown a growing trend in the use of these software programs along with the improvement of software functions. For example, Ye et al. (2018) constructed an absorption model using GastroPlus to predict the potential effects of different gastric pH levels on the pharmacokinetics of lanabecestat and found that changes in gastric pH had a minimal influence on clinical exposure to lanabecestat. They also compared the bioavailability of two tablet formulations and an oral solution. The results showed that the 90% confidence intervals for geometric mean ratios were within standard bioequivalence boundaries for all other pharmacokinetic parameters, indicating that both tablet formulations were located in the accepted bioequivalence criteria compared with the oral solution (Ye et al., 2018). Boland et al. (2018) used SIMCYP to generate a dose-concentration model by using data from different genders, ages, and oral morphine formulations. The model was then validated against clinical pharmacokinetics data and used to calculate the association of the morphine dose with the plasma concentration. Finally, the analysis showed that older age, female sex, modified-release formulation, and inferior renal function were related to higher plasma concentrations (Boland et al., 2018). This result can help clinicians provide personalized prescription decisions.
ADMET
We listed ADMET prediction applications by using AdmetSAR, SwissADME, Lazar, or Toxtree in Table 11. For example, Petrescu et al. (2019) used the AdmetSAR computational program to study the cytotoxicity of 15 phenolic compounds. The results showed that these compounds were much less toxic to aquatic life than synthetic pesticides. Roman et al. (2018) used SwissADME, FAFDrugs4, and admetSAR to predict the ADMET profiles and pharmacokinetics of 31 anabolic and androgen steroids in humans. The results revealed that the investigated steroids showed high gastrointestinal absorption and good oral bioavailability, which may be useful in the inhibition of human cytochromes associated with the metabolism of xenobiotics. In addition, the side effects of the studied steroids in humans were also predicted. Silva et al. (2019) predicted the theoretical toxicity of fluconazole (FNZ) by using Lazar to study the toxicity profile of FNZ toward human peripheral blood mononuclear cells (PBMCs) cultured in vitro. The results showed that FNZ had potential mutagenic, tumorigenic, stimulating, and carcinogenic effects (Silva et al., 2019). Zhuang J. et al. (2018) used Toxtree (version 2.6.13) to evaluate the toxicity of extractables from multilayer coextrusion bags, and their prediction results revealed the types of extractables as well as the bioaccumulation factor and mutagenicity.
Discussion
Deficiencies in Current in silico Methods
Each in silico method has its own characteristics and application scope. Hence, we need to select the most proper method for more accurate prediction. However, some methods have obvious deficiencies that may affect the prediction results. For example, molecular modeling plays a major role only in predicting metabolism and can assess only the possible interactions between compounds and metabolic enzymes; it cannot explicitly evaluate the ADMET risks of candidate compounds. The scoring function in molecular docking also affects the accuracy of ADMET prediction (de Graaf et al., 2006; Zhou et al., 2006). For instance, Kemp et al. (2004) and de Graaf et al. (2006) used different scoring functions to evaluate the binding affinities between cytochrome P450 and its inhibitors or substrates, respectively. Kemp et al. (2004) docked 33 compounds to P450, and the results revealed a correlation coefficient of R2 = 0.61 between the docking scores and active compounds. The docking scores were only able to identify several compounds as CYP2D6 inhibitors. Although de Graaf et al. (2006) integrated six scoring functions to identify the substrates of P450, the highest predicted accuracy (GOLD-Chemscore) identified 60% known substrates in the top 5% results during the virtual screening. Only high-affinity CYP2D6 ligands could be predicted. Therefore, docking methods with scoring functions are mostly applied for coarse screening of a series of compounds.
Compared with molecular modeling, data modeling can predict more properties, but its prediction accuracy depends on the quality and quantity of data. The QSAR method, the main strategy in data modeling, has limited value without an estimated model applicability domain for predicting biological or physicochemical properties (Sushko et al., 2010). The predictive ability of models will be limited if the predicted chemical is outside the chemical space where the models were developed (Sheridan et al., 2004). Furthermore, the descriptors used in the model construction for structural transformation are too simplistic and inadequate to predict the behavior of a drug in a whole organism. Therefore, there is a need to develop molecular descriptors containing more information. Many developed QSAR models have been validated only by internal validation without sufficient external validation, which is considered a necessary factor to build a reliable QSAR model (Roy et al., 2012). QSAR prediction is based on the principle that similar molecules have similar properties (Patterson et al., 1996), but in some special cases, such as CYP metabolism, similar molecules may have different activities, which are known as activity cliffs (Guha, 2011). Therefore, the ADMET properties of the compounds in the human body are not independent but are also affected by other factors. The PBPK method can predict multiple properties, but only provides common information about the biological behavior of organs or tissues. It is also limited by the mathematical form of the PBPK equations, which ignores the structural and physical properties of drug compounds (Huynh et al., 2009). Moreover, a large amount of experimental data is required when constructing models. Due to the lack of proper and easily accessible databases related to physiological properties, the data used to build models can be obtained only from the literature (Rowland et al., 2004). However, the obtained data are relatively limited, which reduces the predictability of the models. Finally, although some PBPK software programs have been developed, most of them are commercial, and users must participate in the training of software companies to make them more useful (Lave et al., 2007).
The existing ADMET software can perform faster and more convenient predictions of multiple properties to obtain more comprehensive prediction results. However, we can see in practical applications that the software is applicable only for qualitative analysis of compounds and cannot accurately predict the quantitative values of some properties. Moreover, issues are observed in the data quality and quantity of these software. While more experimental data are needed to further optimize the software, integrating unconfirmed data into the software to predict new compounds will decrease the prediction accuracy.
Comparison of the Applications of Three Methods in Predicting ADMET Properties
To compare the application trends of each method more intuitively, we counted the number of applications of these three methods from 2016 to 2018 (Figure 6). Applications using PBPK modeling software have exhibited an upward trend in recent years, which means that this approach will be the mainstream pharmacokinetic evaluation in the future. We believe that more user-friendly software will be developed, making the prediction process more convenient for users. Applications using ADMET software for forecasting are also on the rise. The main reason for these changes may be the demand for multi-property prediction and the phenomenon of drug recall, leading to the hope of predicting more pharmacokinetic properties in advance to reduce drug development costs. This increase also confirms that researchers have gradually integrated software predictions into the early stages of drug discovery to improve the success rate of drug development through complementarity and collaboration. In addition, we found that the QSAR method is rarely used alone, potentially due to the limitations of the QSAR method prediction. Therefore, we can increase or integrate the data volume of some specific QSAR models to generate a more comprehensive prediction model. Researchers can also combine different modeling methods and then perform relevant predictions. If a combined model can predict the properties well, then it can be used as a consensus approach to improve ADMET prediction accuracy.
Previous Review and Prospective Studies of ADMET and PBPK Simulations
To date, there have been many reviews on ADMET and PBPK, which provide summaries from different perspectives. We found 11 articles that are closely related to this review after careful retrieval of the published literature. We have classified these articles into three categories based on the perspective of the descriptions: (1) directly related to our review, (2) machine learning (ML) methods, and (3) advances in in silico ADMET modeling.
Five reviews are directly related to this article. Yamashita and Hashida (2004) reviewed the application of structure-based methods and QSAR methods in predicting ADMET in detail. Cheng et al. (2013) introduced the recent progress and current challenges of QSAR in predicting ADMET and then discussed several new promising research directions that could be employed for systemic in silico ADMET prediction. Alqahtani (2017) reviewed the in silico models for predicting the ADMET properties of compounds and provided a comprehensive overview of the latest modeling methods and algorithms, as well as the application prospects of PBPK in predicting pharmacokinetics. Wang et al. (2015) briefly introduced the development of in silico models for ADMET prediction. They also focused on the modeling approaches, related applications, and potential advantages or disadvantages of these models used in drug discovery. Wishart (2007) highlighted ADMET property prediction, as well as ADME-related databases and software, and briefly introduced the application of PBPK and related software in ADMET.
Three out of 11 reviews are about machine learning methods for predicting ADMET. Ferreira and Andricopulo (2019) provided a detailed description of current machine learning approaches to ADMET modeling, focusing on key advances from 2017 to 2018. Tao et al. (2015) reviewed the progress of machine learning methods in ADMET prediction and discussed the performance, applications, and challenges in developing machine learning methods. Maltarollo et al. (2015) described the applications of ML methods in ADMET prediction.
The remaining three reviews addressed the progress of ADMET modeling. Specifically, Lin et al. (2003) introduced the desirable properties of new chemical entities (NCEs) from an ADMET perspective and discussed basic concepts, important tools, reagents, and experimental approaches used by researchers in predicting human pharmacokinetics. van de Waterbeemd and Gifford (2003) summarized the endpoints of pharmacokinetics, metabolism, and toxicity. Wang and Hou (2009) introduced the properties of ADMET, discussed the latest corresponding in silico models, and provided a brief summary of some software and databases. These articles introduced ADMET, PBPK, and related research progress from different perspectives.
Conclusion
In this review, we provide a detailed and comprehensive introduction to currently used approaches or tools in predicting ADMET properties, including the basic principles, classification, and applications. In addition, we collect related applications from published articles over the past 3 years and analyze the trends in these applications. The purpose of this review is to help readers quickly understand these approaches and the characteristics of the related tools (databases and software). It may also provide readers with a better understanding of how existing tools can be applied to pharmacokinetic predictions. We are convinced that more accurate predictions due to users' familiarity with existing online services will increase the importance of in silico ADMET prediction in pharmacokinetics. In the future, we expect not only a reduced failure rate in drug development and drug recalls but also a faster timeline from R&D to market, as well as decreased costs during the late stage of development.
Author Contributions
ZH, FW, and YZ contributed to the design and conception of the study. LL, YZ, FW, XS, GC, XW, XL, and MT performed information retrieval and analysis. FW, YZ, LL, and XS wrote the manuscript. YZ, FW, LL, XS, GC, XW, XL, and MT created the tables and figures. ZH and FW guided the manuscript writing and revised the manuscript. ZH provided financial support. All authors contributed to manuscript revision and have read and approved the submitted version.
Funding
This work was supported by the National Natural Science Foundation of China (31770774), the Provincial Major Project of Basic or Applied Research in Natural Science, the Guangdong Provincial Education Department (2016KZDXM038), and the Higher Education Reform Project of Guangdong Province (2019268).
Conflict of Interest
The authors declare that the research was conducted in the absence of any commercial or financial relationships that could be construed as a potential conflict of interest.
References
Abdelmonsef, A. H., Dulapalli, R., Dasari, T., Padmarao, L. S., Mukkera, T., and Vuruputuri, U. (2016). Identification of novel antagonists for rab38 protein by homology modeling and virtual screening. Comb. Chem. High Throughput Screen. 19, 875–892. doi: 10.2174/1386207319666161026153237
Abid, S. M. A., Younus, H. A., Al-Rashida, M., Arshad, Z., Maryum, T., Gilani, M. A., et al. (2017). Sulfonyl hydrazones derived from 3-formylchromone as non-selective inhibitors of MAO-A and MAO-B: synthesis, molecular modelling and in-silico ADME evaluation. Bioorg. Chem. 75, 291–302. doi: 10.1016/j.bioorg.2017.10.001
Absalan, A., Mesbah-Namin, S. A., Tiraihi, T., and Taheri, T. (2016). The effects of cinnamaldehyde and eugenol on human adipose-derived mesenchymal stem cells viability, growth and differentiation: a cheminformatics and in vitro study. Avicenna J. Phytomed. 6, 643–657.
Agoni, C., Ramharack, P., and Soliman, M. E. S. (2018). Allosteric inhibition induces an open WPD-loop: a new avenue towards glioblastoma therapy. RSC Adv. 8, 40187–40197. doi: 10.1039/C8RA08427K
Ajay Kumar, T. V., Athavan, A. A. S., Loganathan, C., Saravanan, K., Kabilan, S., and Parthasarathy, V. (2018). Design, 3D QSAR modeling and docking of TGF-beta type I inhibitors to target cancer. Comput. Biol. Chem. 76, 232–244. doi: 10.1016/j.compbiolchem.2018.07.011
Alarn, S., and Khan, F. (2019). 3D-QSAR, docking, ADME/Tox studies on flavone analogs reveal anticancer activity through Tankyrase inhibition. Sci. Rep. 9:5414. doi: 10.1038/s41598-019-41984-7
Alqahtani, S. (2017). In silico ADME-Tox modeling: progress and prospects. Expert Opin. Drug Metab. Toxicol. 13, 1147–1158. doi: 10.1080/17425255.2017.1389897
Andrade, C. H., Silva, D. C., and Braga, R. C. (2014). In silico prediction of drug metabolism by P450. Curr. Drug Metab. 15, 514–525. doi: 10.2174/1389200215666140908102530
Arora, P., Adams, C. H., Gudelsky, G., DasGupta, B., and Desai, P. B. (2018). Plasma and brain pharmacokinetics of letrozole and drug interaction studies with temozolomide in NOD-scid gamma mice and sprague dawley rats. Cancer Chemother. Pharmacol. 83, 81–89. doi: 10.1007/s00280-018-3705-6
Arora, R., Sharma, M., Monif, T., and Iyer, S. (2016). A multi-centric bioequivalence trial in Ph+ chronic myeloid leukemia patients to assess bioequivalence and safety evaluation of generic imatinib mesylate 400 mg tablets. Cancer Res. Treat. 48, 1120–1129. doi: 10.4143/crt.2015.436
Aswathy, L., Jisha, R. S., Masand, V. H., Gajbhiye, J. M., and Shibi, I. G. (2018). Design of novel amyloid beta aggregation inhibitors using QSAR, pharmacophore modeling, molecular docking and ADME prediction. In Silico Pharmacol. 6:12. doi: 10.1007/s40203-018-0049-1
Azad, I., Nasibullah, M., Khan, T., Hassan, F., and Akhter, Y. (2018). Exploring the novel heterocyclic derivatives as lead molecules for design and development of potent anticancer agents. J. Mol. Graph Model. 81, 211–228. doi: 10.1016/j.jmgm.2018.02.013
Basheer, L., Schultz, K., Guttman, Y., and Kerem, Z. (2017). In silico and in vitro inhibition of cytochrome P450 3A by synthetic stilbenoids. Food Chem. 237, 895–903. doi: 10.1016/j.foodchem.2017.06.040
Basheer, L., Schultz, K., and Kerem, Z. (2016). Inhibition of cytochrome P450 3A by acetoxylated analogues of resveratrol in in vitro and in silico models. Sci. Rep. 6:31557. doi: 10.1038/srep31557
Belekar, V., Lingineni, K., and Garg, P. (2015). Classification of breast cancer resistant protein (BCRP) inhibitors and non-inhibitors using machine learning approaches. Comb. Chem. High Throughput Screen. 18, 476–485. doi: 10.2174/1386207318666150525094503
Berry, S. A., Vockley, J., Vinks, A. A., Dong, M., Diaz, G. A., McCandless, S. E., et al. (2018). Pharmacokinetics of glycerol phenylbutyrate in pediatric patients 2 months to 2 years of age with urea cycle disorders. Mol. Genet. Metab. 125, 251–257. doi: 10.1016/j.ymgme.2018.09.001
Bhatia, S., Schultz, T., Roberts, D., Shen, J., Kromidas, L., and Marie Api, A. (2015). Comparison of cramer classification between toxtree, the OECD QSAR toolbox and expert judgment. Regul. Toxicol. Pharmacol. 71, 52–62. doi: 10.1016/j.yrtph.2014.11.005
Bhhatarai, B., Wilson, D. M., Parks, A. K., Carney, E. W., and Spencer, P. J. (2016). Evaluation of TOPKAT, toxtree, and derek nexus in silico models for ocular irritation and development of a knowledge-based framework to improve the prediction of severe irritation. Chem. Res. Toxicol. 29, 810–22. doi: 10.1021/acs.chemrestox.5b00531
Boetsch, C., Parrott, N., Fowler, S., Poirier, A., Hainzl, D., Banken, L., et al. (2016). Effects of cytochrome P450 3A4 inhibitors-ketoconazole and erythromycin-on bitopertin pharmacokinetics and comparison with physiologically based modelling predictions. Clin. Pharmacokinet. 55, 237–247. doi: 10.1007/s40262-015-0312-0
Boland, J. W., Johnson, M., Ferreira, D., and Berry, D. J. (2018). In silico (computed) modelling of doses and dosing regimens associated with morphine levels above international legal driving limits. Palliat. Med. 32, 1222–1232. doi: 10.1177/0269216318773956
Bouzom, F., Ball, K., Perdaems, N., and Walther, B. (2012). Physiologically based pharmacokinetic (PBPK) modelling tools: how to fit with our needs? Biopharm. Drug Dispos. 33, 55–71. doi: 10.1002/bdd.1767
Bouzom, F., and Walther, B. (2008). Pharmacokinetic predictions in children by using the physiologically based pharmacokinetic modelling. Fundam. Clin. Pharmacol. 22, 579–587. doi: 10.1111/j.1472-8206.2008.00648.x
Bowen, J. P., and Guener, O. F. (2013). A perspective on quantum mechanics calculations in ADMET predictions. Curr. Top. Med. Chem. 13, 1257–1272. doi: 10.2174/15680266113139990032
Braeuning, C., Braeuning, A., Mielke, H., Holzwarth, A., and Peiser, M. (2018). Evaluation and improvement of QSAR predictions of skin sensitization for pesticides. SAR QSAR Environ. Res. 29, 823–846. doi: 10.1080/1062936X.2018.1518261
Brown, R. T., Nicholas, C. R., Cozzi, N. V., Gassman, M. C., Cooper, K. M., Muller, D., et al. (2017). Pharmacokinetics of escalating doses of oral psilocybin in healthy adults. Clin. Pharmacokinet. 56, 1543–1554. doi: 10.1007/s40262-017-0540-6
Bujak, R., Struck-Lewicka, W., Kaliszan, M., Kaliszan, R., and Markuszewski, M. J. (2015). Blood-brain barrier permeability mechanisms in view of quantitative structure-activity relationships (QSAR). J. Pharm. Biomed. Anal. 108, 29–37. doi: 10.1016/j.jpba.2015.01.046
Burmanczuk, A., Grabowski, T., Osypiuk, M., Polska, B., and Kowalski, C. (2017). Determination of cloxacillin residues in dairy cows after intramammary administration. J. Vet. Pharmacol. Ther. 40, 552–560. doi: 10.1111/jvp.12391
Byun, J. H., Han, D. G., Cho, H. J., Yoon, I. S., and Jung, I. H. (2020). Recent advances in physiologically based pharmacokinetic and pharmacodynamic models for anticancer nanomedicines. Arch. Pharm. Res. 43, 80–99. doi: 10.1007/s12272-020-01209-2
Caldwell, G. W., Yan, Z., Tang, W., Dasgupta, M., and Hasting, B. (2009). ADME optimization and toxicity assessment in early- and late-phase drug discovery. Curr. Top. Med. Chem. 9, 965–980. doi: 10.2174/156802609789630929
Cao, D., Wang, J., Zhou, R., Li, Y., Yu, H., and Hou, T. (2012). ADMET evaluation in drug discovery. 11. Pharmacokinetics knowledge base (PKKB): a comprehensive database of pharmacokinetic and toxic properties for drugs. J. Chem. Inf. Model. 52, 1132–1137. doi: 10.1021/ci300112j
Chedik, L., Mias-Lucquin, D., Bruyere, A., and Fardel, O. (2017). In silico prediction for intestinal absorption and brain penetration of chemical pesticides in humans. Int. J. Environ. Res. Public Health 14:708. doi: 10.3390/ijerph14070708
Chen, J. Y., Yi, M., Yao, S. L., and Zhang, X. P. (2016). Propofol target-controlled infusion modeling in rabbits: pharmacokinetic and pharmacodynamic analysis. J. Huazhong Univ. Sci. Technol. Med. Sci. 36, 428–433. doi: 10.1007/s11596-016-1604-9
Chen, L., and Gao, Z. (2018). Continuous hypoxia reduces the concentration of streptomycin in the blood. BMC Infect. Dis. 18:120. doi: 10.1186/s12879-018-3027-7
Chen, W., Desai, D., Good, D., Crison, J., Timmins, P., Paruchuri, S., et al. (2016). Mathematical model-based accelerated development of extended-release metformin hydrochloride tablet formulation. AAPS PharmSciTech. 17, 1007–1013. doi: 10.1208/s12249-015-0423-9
Chen, Y., Liu, Z. L., Fu, T. M., Li, W., Xu, X. L., and Sun, H. P. (2015). Discovery of new acetylcholinesterase inhibitors with small core structures through shape-based virtual screening. Bioorg. Med. Chem. Lett. 25, 3442–3446. doi: 10.1016/j.bmcl.2015.07.026
Cheng, F., Li, W., Liu, G., and Tang, Y. (2013). In silico ADMET prediction: recent advances, current challenges and future trends. Curr. Top. Med. Chem. 13, 1273–1289. doi: 10.2174/15680266113139990033
Cheng, F., Li, W., Zhou, Y., Shen, J., Wu, Z., Liu, G., et al. (2012). admetSAR: a comprehensive source and free tool for assessment of chemical ADMET properties. J. Chem. Inf. Model. 52, 3099–3105. doi: 10.1021/ci300367a
Cheng, P., Li, J., Wang, J., Zhang, X., and Zhai, H. (2018). Investigations of FAK inhibitors: a combination of 3D-QSAR, docking, and molecular dynamics simulations studies. J. Biomol. Struct. Dyn. 36, 1529–1549. doi: 10.1080/07391102.2017.1329095
Cheng, T., Li, Q., Zhou, Z., Wang, Y., and Bryant, S. H. (2012). Structure-based virtual screening for drug discovery: a problem-centric review. AAPS J. 14, 133–141. doi: 10.1208/s12248-012-9322-0
Cheng, X., Li, P., Chen, Z., Zhang, N., Zhen, Y., Zhao, L., et al. (2018). Break-through bleeding in relation to pharmacokinetics of factor VIII in paediatric patients with severe haemophilia A. Haemophilia 24, 120–125. doi: 10.1111/hae.13373
Chow, E. C. Y., Talattof, A., Tsakalozou, E., Fan, J., Zhao, L., and Zhang, X. (2016). Using physiologically based pharmacokinetic (PBPK) modeling to evaluate the impact of pharmaceutical excipients on oral drug absorption: sensitivity analyses. AAPS J. 18, 1500–1511. doi: 10.1208/s12248-016-9964-4
Cook, D., Brown, D., Alexander, R., March, R., Morgan, P., Satterthwaite, G., et al. (2014). Lessons learned from the fate of AstraZeneca's drug pipeline: a five-dimensional framework. Nat. Rev. Drug Discov. 13, 419–431. doi: 10.1038/nrd4309
Cui, Q., and Karplus, M. (2003). Catalysis and specificity in enzymes: a study of triosephosphate isomerase and comparison with methyl glyoxal synthase. Adv. Protein Chem. 66, 315–372. doi: 10.1016/S0065-3233(03)66008-0
Cumming, J. G., Davis, A. M., Muresan, S., Haeberlein, M., and Chen, H. (2013). Chemical predictive modelling to improve compound quality. Nat. Rev. Drug Discov. 12, 948–962. doi: 10.1038/nrd4128
Daga, P. R., Bolger, M. B., Haworth, I. S., Clark, R. D., and Martin, E. J. (2018). Physiologically based pharmacokinetic modeling in lead optimization. 1. Evaluation and adaptation of gastroplus to predict bioavailability of medchem series. Mol. Pharm. 15, 821–830. doi: 10.1021/acs.molpharmaceut.7b00972
Daina, A., Michielin, O., and Zoete, V. (2017). SwissADME: a free web tool to evaluate pharmacokinetics, drug-likeness and medicinal chemistry friendliness of small molecules. Sci. Rep. 7:42717. doi: 10.1038/srep42717
Dallmann, A., Ince, I., Solodenko, J., Meyer, M., Willmann, S., Eissing, T., et al. (2017). Physiologically based pharmacokinetic modeling of renally cleared drugs in pregnant women. Clin. Pharmacokinet. 56, 1525–1541. doi: 10.1007/s40262-017-0538-0
Danishuddin, and Khan, A. U. (2016). Descriptors and their selection methods in QSAR analysis: paradigm for drug design. Drug Discov. Today 21, 1291–1302. doi: 10.1016/j.drudis.2016.06.013
Das, S., Laskar, M. A., Sarker, S. D., Choudhury, M. D., Choudhury, P. R., Mitra, A., et al. (2017). Prediction of anti-alzheimer's activity of flavonoids targeting acetylcholinesterase in silico. Phytochem. Anal. 28, 324–331. doi: 10.1002/pca.2679
David-Neto, E., Romano, P., Kamada Triboni, A. H., Ramos, F., Agena, F., Almeida Rezende Ebner, P., et al. (2017). Longitudinal pharmacokinetics of tacrolimus in elderly compared with younger recipients in the first 6 months after renal transplantation. Transplantation 101, 1365–1372. doi: 10.1097/TP.0000000000001369
Davis, A. P., Grondin, C. J., Johnson, R. J., Sciaky, D., McMorran, R., Wiegers, J., et al. (2019). The comparative toxicogenomics database: update 2019. Nucleic Acids Res. 47, D948–D954. doi: 10.1093/nar/gky868
De Buck, S. S., and Mackie, C. E. (2007). Physiologically based approaches towards the prediction of pharmacokinetics: in vitro-in vivo extrapolation. Expert Opin. Drug Metab. Toxicol. 3, 865–878. doi: 10.1517/17425255.3.6.865
de Castro, F. A., Simoes, B. P., Godoy, A. L., Bertagnoli Trigo, F. M., Coelho, E. B., and Lanchote, V. L. (2016). Use of an oral busulfan test dose in patients undergoing hematopoietic stem cell transplantation treated with or without fludarabine. J. Clin. Pharmacol. 56, 1555–1562. doi: 10.1002/jcph.758
de Graaf, C., Oostenbrink, C., Keizers, P. H., van der Wijst, T., Jongejan, A., and Vermeulen, N. P. (2006). Catalytic site prediction and virtual screening of cytochrome P450 2D6 substrates by consideration of water and rescoring in automated docking. J. Med. Chem. 49, 2417–2430. doi: 10.1021/jm0508538
de Groot, M. J. (2006). Designing better drugs: predicting cytochrome P450 metabolism. Drug Discov. Today 11, 601–606. doi: 10.1016/j.drudis.2006.05.001
de Groot, M. J., Kirton, S. B., and Sutcliffe, M. J. (2004). In silico methods for predicting ligand binding determinants of cytochromes P450. Curr. Top. Med. Chem. 4, 1803–1824. doi: 10.2174/1568026043387061
De Velde, F., De Winter, B. C. M., Koch, B. C. P., Van Gelder, T., Mouton, J. W., and COMBACTE-NET consortium (2018). Highly variable absorption of clavulanic acid during the day: a population pharmacokinetic analysis. J. Antimicrob. Chemother. 73, 469–476. doi: 10.1093/jac/dkx376
Dearden, J. C. (2007). In silico prediction of ADMET properties: how far have we come? Expert Opin. Drug Metab. Toxicol. 3, 635–639. doi: 10.1517/17425255.3.5.635
Delaney, S. R., Malik, P. R. V., Stefan, C., Edginton, A. N., Colantonio, D. A., and Ito, S. (2018). Predicting escitalopram exposure to breastfeeding infants: integrating analytical and in silico techniques. Clin. Pharmacokinet. 57, 1603–1611. doi: 10.1007/s40262-018-0657-2
Dickins, M., and van de Waterbeemd, H. (2004). Simulation models for drug disposition and drug interactions. Drug Discov. Today BIOSILICO 2, 38–45. doi: 10.1016/S1741-8364(04)02388-1
Dong, J., Wang, N. N., Yao, Z. J., Zhang, L., Cheng, Y., Ouyang, D., et al. (2018). ADMETlab: a platform for systematic ADMET evaluation based on a comprehensively collected ADMET database. J. Cheminform. 10:29. doi: 10.1186/s13321-018-0283-x
Doss, C. G. P., Chakraborty, C., Narayan, V., and Kumar, D. T. (2014). “Computational approaches and resources in single amino acid substitutions analysis toward clinical research,” in Advances in Protein Chemistry and Structural Biology, Vol. 94, ed R. Donev (San Diego, CA: Elsevier Academic Press), 365–423. doi: 10.1016/B978-0-12-800168-4.00010-X
Du, H., Li, J., Cai, Y., Zhang, H., Liu, G., Tang, Y., et al. (2017). Computational investigation of ligand binding to the peripheral site in CYP3A4: conformational dynamics and inhibitor discovery. J. Chem. Inf. Model. 57, 616–626. doi: 10.1021/acs.jcim.7b00012
Du, X., Kou, H., Fu, Q., Li, Y., Zhu, Z., and Li, T. (2017). Steady-state pharmacokinetics of tenofovir disoproxil fumarate in human immunodeficiency virus-infected Chinese patients. Expert Rev. Clin. Pharmacol. 10, 783–788. doi: 10.1080/17512433.2017.1321480
Dutour, R., Cortes-Benitez, F., Roy, J., and Poirier, D. (2017). Structure-based design and synthesis of new estrane-pyridine derivatives as cytochrome P450 (CYP) 1B1 inhibitors. ACS Med. Chem. Lett. 8, 1159–1164. doi: 10.1021/acsmedchemlett.7b00265
Edginton, A. N., Theil, F. P., Schmitt, W., and Willmann, S. (2008). Whole body physiologically-based pharmacokinetic models: their use in clinical drug development. Expert Opin. Drug Metab. Toxicol. 4, 1143–1152. doi: 10.1517/17425255.4.9.1143
El-Zahabi, H. S. A., Khalifa, M. M. A., Gado, Y. M. H., Farrag, A. M., Elaasser, M. M., Safwat, N. A., et al. (2019). New thiobarbituric acid scaffold-based small molecules: synthesis, cytotoxicity, 2D-QSAR, pharmacophore modelling and in-silico ADME screening. Eur. J. Pharm. Sci. 130, 124–136. doi: 10.1016/j.ejps.2019.01.023
Espie, P., Tytgat, D., Sargentini-Maier, M. L., Poggesi, I., and Watelet, J. B. (2009). Physiologically based pharmacokinetics (PBPK). Drug Metab. Rev. 41, 391–407. doi: 10.1080/10837450902891360
Evangelista, W., Weir, R. L., Ellingson, S. R., Harris, J. B., Kapoor, K., Smith, J. C., et al. (2016). Ensemble-based docking: from hit discovery to metabolism and toxicity predictions. Bioorg. Med. Chem. 24, 4928–4935. doi: 10.1016/j.bmc.2016.07.064
Fan, J., and de Lannoy, I. A. (2014). Pharmacokinetics. Biochem. Pharmacol. 87, 93–120. doi: 10.1016/j.bcp.2013.09.007
Ferreira, L. L. G., and Andricopulo, A. D. (2019). ADMET modeling approaches in drug discovery. Drug Discov. Today 24, 1157–1165. doi: 10.1016/j.drudis.2019.03.015
Franquiz, M. J., Kalaria, S. N., Armahizer, M. J., Gopalakrishnan, M., McCarthy, P. J., and Badjatia, N. (2018). Lacosamide pharmacokinetics in a critically Ill patient receiving continuous venovenous hemofiltration. Pharmacotherapy 38, E17–E21. doi: 10.1002/phar.2063
Fratev, F., and Benfenati, E. (2008). A combination of 3D-QSAR, docking, local-binding energy (LBE) and GRID study of the species differences in the carcinogenicity of benzene derivatives chemicals. J. Mol. Graph Model. 27, 147–160. doi: 10.1016/j.jmgm.2008.04.004
Frenzel, F., Buhrke, T., Wenzel, I., Andrack, J., Hielscher, J., and Lampen, A. (2017). Use of in silico models for prioritization of heat-induced food contaminants in mutagenicity and carcinogenicity testing. Arch. Toxicol. 91, 3157–3174. doi: 10.1007/s00204-016-1924-3
Fu, L., Liu, L., Yang, Z. J., Li, P., Ding, J. J., Yun, Y. H., et al. (2020). Systematic modeling of logD7.4 based on ensemble machine learning, group contribution, and matched molecular pair analysis. J. Chem. Inf. Model. 60, 63–76. doi: 10.1021/acs.jcim.9b00718
Gaulton, A., Hersey, A., Nowotka, M., Bento, A. P., Chambers, J., Mendez, D., et al. (2017). The ChEMBL database in 2017. Nucleic Acids Res. 45, D945–D954. doi: 10.1093/nar/gkw1074
Geerts, L., Adriaens, E., Alepee, N., Guest, R., Willoughby, J. A. Sr., Kandarova, H., et al. (2017). CON4EI: evaluation of QSAR models for hazard identification and labelling of eye irritating chemicals. Toxicol. In Vitro 49, 90–98. doi: 10.1016/j.tiv.2017.09.004
George, J. K., Singh, S. K., and Verma, P. R. (2016). In vivo in silico pharmacokinetic simulation studies of carvedilol-loaded nanocapsules using GastroPlus. Ther. Deliv. 7, 305–318. doi: 10.4155/tde-2015-0004
Gestrich, A., Bedenice, D., Ceresia, M., and Zaghloul, I. (2018). Pharmacokinetics of intravenous gentamicin in healthy young-adult compared to aged alpacas. J. Vet. Pharmacol. Ther. 41, 581–587. doi: 10.1111/jvp.12506
Gluck, J., Buhrke, T., Frenzel, F., Braeuning, A., and Lampen, A. (2018). In silico genotoxicity and carcinogenicity prediction for food-relevant secondary plant metabolites. Food Chem. Toxicol. 116, 298–306. doi: 10.1016/j.fct.2018.04.024
Gong, E. C., Chea, S., Balupuri, A., Kang, N. S., Chin, Y. W., and Choi, Y. H. (2018). Enzyme kinetics and molecular docking studies on cytochrome 2B6, 2C19, 2E1, and 3A4 activities by sauchinone. Molecules 23:555. doi: 10.3390/molecules23030555
Guerra, L. R., de Souza, A. M. T., Cortes, J. A., Lione, V. O. F., Castro, H. C., and Alves, G. G. (2017). Assessment of predictivity of volatile organic compounds carcinogenicity and mutagenicity by freeware in silico models. Regul. Toxicol. Pharmacol. 91, 1–8. doi: 10.1016/j.yrtph.2017.09.030
Guha, R. (2011). The ups and downs of structure-activity landscapes. Methods Mol. Biol. 672, 101–117. doi: 10.1007/978-1-60761-839-3_3
Gupta, P., Kamath, A. V., Park, S., Chiu, H., Lutman, J., Maia, M., et al. (2016). Preclinical pharmacokinetics of MHAA4549A, a human monoclonal antibody to influenza A virus, and the prediction of its efficacious clinical dose for the treatment of patients hospitalized with influenza A. MAbs 8, 991–997. doi: 10.1080/19420862.2016.1167294
Hagihara, M., Kato, H., Hamada, Y., Hirai, J., Sakanashi, D., Suematsu, H., et al. (2016). Population pharmacokinetics of arbekacin in different infectious disease settings and evaluation of dosing regimens. J. Infect. Chemother. 22, 436–443. doi: 10.1016/j.jiac.2016.03.008
Han, Y., Zhang, J. P., Hu, C. Q., Zhang, X., Ma, B. F., and Zhang, P. P. (2019). In silico ADME and toxicity prediction of ceftazidime and its impurities. Front. Pharmacol. 10:434. doi: 10.3389/fphar.2019.00434
Hansch, C. (1981). The physicochemical approach to drug design and discovery (QSAR). Drug Dev. Res. 1, 267–309. doi: 10.1002/ddr.430010403
Hansmann, S., Darwich, A., Margolskee, A., Aarons, L., and Dressman, J. (2016). Forecasting oral absorption across biopharmaceutics classification system classes with physiologically based pharmacokinetic models. J. Pharm. Pharmacol. 68, 1501–1515. doi: 10.1111/jphp.12618
Haque, A., Leong, K. H., Lo, Y. L., Awang, K., and Nagoor, N. H. (2017). In vitro inhibitory mechanisms and molecular docking of 1'-S-1'-acetoxychavicol acetate on human cytochrome P450 enzymes. Phytomedicine 31, 1–9. doi: 10.1016/j.phymed.2017.05.002
Hardy, B., Douglas, N., Helma, C., Rautenberg, M., Jeliazkova, N., Jeliazkov, V., et al. (2010). Collaborative development of predictive toxicology applications. J. Cheminform. 2:7. doi: 10.1186/1758-2946-2-7
Helma, C. (2006). Lazy structure-activity relationships (lazar) for the prediction of rodent carcinogenicity and salmonella mutagenicity. Mol. Divers. 10, 147–158. doi: 10.1007/s11030-005-9001-5
Hu, C., Wang, Y., Song, R., Yu, C., Luo, X., and Jia, J. (2017). Single- and multiple-dose pharmacokinetics, safety and tolerability of lurasidone in healthy Chinese subjects. Clin. Drug Investig. 37, 861–871. doi: 10.1007/s40261-017-0546-8
Hu, Y., and Smith, D. E. (2018). In silico prediction of the absorption and disposition of cefadroxil in humans using an intestinal permeability method scaled from humanized PepT1 mice. Drug Metab. Dispos. 47, 173–183. doi: 10.1124/dmd.118.084236
Huang, J., and Fan, X. (2011). Why QSAR fails: an empirical evaluation using conventional computational approach. Mol. Pharm. 8, 600–608. doi: 10.1021/mp100423u
Huang, X., Zhang, S., Ma, Y., Yang, H., He, C., Tian, R., et al. (2018). Bioequivalence of two quetiapine extended release tablets in Chinese healthy volunteers under fasting and fed conditions and effects of food on pharmacokinetic profiles. Drug Des. Devel. Ther. 13, 255–264. doi: 10.2147/DDDT.S182965
Huynh, L., Masereeuw, R., Friedberg, T., Ingelman-Sundberg, M., and Manivet, P. (2009). In silico platform for xenobiotics ADME-T pharmacological properties modeling and prediction. Part I: beyond the reduction of animal model use. Drug Discov. Today 14, 401–405. doi: 10.1016/j.drudis.2009.01.009
Ibarra, M., Valiante, C., Sopena, P., Schiavo, A., Lorier, M., Vazquez, M., et al. (2018). Integration of in vitro biorelevant dissolution and in silico PBPK model of carvedilol to predict bioequivalence of oral drug products. Eur. J. Pharm. Sci. 118, 176–182. doi: 10.1016/j.ejps.2018.03.032
Ibrahim, M. E., Brooks, K. M., Castillo-Mancilla, J. R., McHugh, C., Morrow, M., Brothers, J., et al. (2018). Short communication: bioequivalence of tenofovir and emtricitabine after coencapsulation with the proteus ingestible sensor. AIDS Res. Hum. Retroviruses 34, 835–837. doi: 10.1089/aid.2018.0081
Idkaidek, N., Agha, H., and Arafat, T. (2018a). Saliva versus plasma bioequivalence of valsartan/hydrochlorothiazide in humans: validation of classes II and IV drugs of the salivary excretion classification system. Drug Res. 68, 54–59. doi: 10.1055/s-0043-117775
Idkaidek, N., Hamadi, S., El-Assi, M., Al-Shalalfeh, A., and Al-Ghazawi, A. (2018b). Saliva versus plasma therapeutic drug monitoring of pregabalin in jordanian patients. Drug Res. 68, 596–600. doi: 10.1055/a-0600-2113
Issa, N. T., Wathieu, H., Ojo, A., Byers, S. W., and Dakshanamurthy, S. (2017). Drug metabolism in preclinical drug development: a survey of the discovery process, toxicology, and computational tools. Curr. Drug Metab. 18, 556–565. doi: 10.2174/1389200218666170316093301
Jamei, M., Marciniak, S., Feng, K., Barnett, A., Tucker, G., and Rostami-Hodjegan, A. (2009). The simcyp population-based ADME simulator. Expert Opin. Drug Metab. Toxicol. 5, 211–223. doi: 10.1517/17425250802691074
Jiang, D., Lei, T., Wang, Z., Shen, C., Cao, D., and Hou, T. (2020). ADMET evaluation in drug discovery. 20. Prediction of breast cancer resistance protein inhibition through machine learning. J. Cheminform. 12:16. doi: 10.1186/s13321-020-00421-y
Jin, Y., Borell, H., Gardin, A., Ufer, M., Huth, F., and Camenisch, G. (2018). In vitro studies and in silico predictions of fluconazole and CYP2C9 genetic polymorphism impact on siponimod metabolism and pharmacokinetics. Eur. J. Clin. Pharmacol. 74, 455–464. doi: 10.1007/s00228-017-2404-2
Johnson, T. N., Whitaker, M. J., Keevil, B., and Ross, R. J. (2018). Bioavailability of oral hydrocortisone corrected for binding proteins and measured by LC-MS/MS using serum cortisol and salivary cortisone. J. Bioequivalence Bioavailab. 10, 001–003. doi: 10.4172/jbb.1000365
Jones, H., Chen, Y., Gibson, C., Heimbach, T., Parrott, N., Peters, S., et al. (2015). Physiologically based pharmacokinetic modeling in drug discovery and development: a pharmaceutical industry perspective. Clin. Pharmacol. Ther. 97, 247–262. doi: 10.1002/cpt.37
Kar, S., and Leszczynski, J. (2017). Recent advances of computational modeling for predicting drug metabolism: a perspective. Curr. Drug Metab. 18, 1106–1122. doi: 10.2174/1389200218666170607102104
Kato, H., Yamaotsu, N., Iwazaki, N., Okamura, S., Kume, T., and Hirono, S. (2017). Precise prediction of activators for the human constitutive androstane receptor using structure-based three-dimensional quantitative structure-activity relationship methods. Drug Metab. Pharmacokinet. 32, 179–188. doi: 10.1016/j.dmpk.2017.02.001
Kavitha, R., Karunagaran, S., Chandrabose, S. S., Lee, K. W., and Meganathan, C. (2015). Pharmacophore modeling, virtual screening, molecular docking studies and density functional theory approaches to identify novel ketohexokinase (KHK) inhibitors. Biosystems 138, 39–52. doi: 10.1016/j.biosystems.2015.10.005
Kazmi, S. R., Jun, R., Yu, M.-S., Jung, C., and Na, D. (2019). In silico approaches and tools for the prediction of drug metabolism and fate: a review. Comput. Biol. Med. 106, 54–64. doi: 10.1016/j.compbiomed.2019.01.008
Keemink, J., Sjogren, E., Holm, R., and Bergstrom, C. A. S. (2018). Does the intake of ethanol affect oral absorption of poorly soluble drugs? J. Pharm. Sci. 108, 1765–1771. doi: 10.1016/j.xphs.2018.12.006
Kemp, C. A., Flanagan, J. U., van Eldik, A. J., Marechal, J. D., Wolf, C. R., Roberts, G. C., et al. (2004). Validation of model of cytochrome P450 2D6: an in silico tool for predicting metabolism and inhibition. J. Med. Chem. 47, 5340–5346. doi: 10.1021/jm049934e
Khalid, S., Zahid, M. A., Ali, H., Kim, Y. S., and Khan, S. (2018). Biaryl scaffold-focused virtual screening for anti-aggregatory and neuroprotective effects in alzheimer's disease. BMC Neurosci. 19:74. doi: 10.1186/s12868-018-0472-6
Khan, M. S., Majid, A. M., Iqbal, M. A., Majid, A. S., Al-Mansoub, M., and Haque, R. S. (2016). Designing the angiogenic inhibitor for brain tumor via disruption of VEGF and IL17A expression. Eur. J. Pharm. Sci. 93, 304–318. doi: 10.1016/j.ejps.2016.08.032
Khan, M. T. (2010). Predictions of the ADMET properties of candidate drug molecules utilizing different QSAR/QSPR modelling approaches. Curr. Drug Metab. 11, 285–295. doi: 10.2174/138920010791514306
Khurana, R. K., Kaur, R., Kaur, M., Kaur, R., Kaur, J., Kaur, H., et al. (2017). Exploring and validating physicochemical properties of mangiferin through GastroPlus((R)) software. Future Sci. OA 3:FSO167. doi: 10.4155/fsoa-2016-0055
Kim, S., Chen, J., Cheng, T., Gindulyte, A., He, J., He, S., et al. (2019). PubChem 2019 update: improved access to chemical data. Nucleic Acids Res. 47, D1102–D1109. doi: 10.1093/nar/gky1033
Kim, S. J., Heo, S. H., Lee, D. S., Hwang, I. G., Lee, Y. B., and Cho, H. Y. (2016). Gender differences in pharmacokinetics and tissue distribution of 3 perfluoroalkyl and polyfluoroalkyl substances in rats. Food Chem. Toxicol. 97, 243–255. doi: 10.1016/j.fct.2016.09.017
Kirchmair, J., Williamson, M. J., Tyzack, J. D., Tan, L., Bond, P. J., Bender, A., et al. (2012). Computational prediction of metabolism: sites, products, SAR, P450 enzyme dynamics, and mechanisms. J. Chem. Inf. Model. 52, 617–648. doi: 10.1021/ci200542m
Kola, I., and Landis, J. (2004). Can the pharmaceutical industry reduce attrition rates? Nat. Rev. Drug Discov. 3, 711–715. doi: 10.1038/nrd1470
Kollar, J., and Frecer, V. (2018). Diarylcyclopropane hydroxamic acid inhibitors of histone deacetylase 4 designed by combinatorial approach and QM/MM calculations. J. Mol. Graph Model. 85, 97–110. doi: 10.1016/j.jmgm.2018.08.008
Kong, L., Tang, Y., Zhang, X., Lu, G., Yu, M., Shi, Q., et al. (2017). Pharmacokinetic/pharmacodynamic analysis of meropenem for the treatment of nosocomial pneumonia in intracerebral hemorrhage patients by monte carlo simulation. Ann. Pharmacother. 51, 970–975. doi: 10.1177/1060028017719715
Kou, D., Zhang, C., Yiu, H., Ng, T., Lubach, J. W., Janson, M., et al. (2018). In vitro, in silico, and in vivo assessments of intestinal precipitation and its impact on bioavailability of a BCS class 2 basic compound. Mol. Pharm. 15, 1607–1617. doi: 10.1021/acs.molpharmaceut.7b01143
Kumar, R., Garg, P., and Bharatam, P. V. (2015). Shape-based virtual screening, docking, and molecular dynamics simulations to identify Mtb-ASADH inhibitors. J. Biomol. Struct. Dyn. 33, 1082–1093. doi: 10.1080/07391102.2014.929535
Lapins, M., Arvidsson, S., Lampa, S., Berg, A., Schaal, W., Alvarsson, J., et al. (2018). A confidence predictor for logD using conformal regression and a support-vector machine. J. Cheminform. 10:17. doi: 10.1186/s13321-018-0271-1
Lauro, G., Cantone, V., Potenza, M., Fischer, K., Koeberle, A., Werz, O., et al. (2018). Discovery of 3-hydroxy-3-pyrrolin-2-one-based mPGES-1 inhibitors using a multi-step virtual screening protocol. Medchemcomm 9, 2028–2036. doi: 10.1039/C8MD00497H
Lave, T., Parrott, N., Grimm, H. P., Fleury, A., and Reddy, M. (2007). Challenges and opportunities with modelling and simulation in drug discovery and drug development. Xenobiotica 37, 1295–1310. doi: 10.1080/00498250701534885
Law, V., Knox, C., Djoumbou, Y., Jewison, T., Guo, A. C., Liu, Y., et al. (2013). DrugBank 4.0: shedding new light on drug metabolism. Nucleic Acids Res. 42, D1091–D1097. doi: 10.1093/nar/gkt1068
Lea, I. A., Gong, H., Paleja, A., Rashid, A., and Fostel, J. (2017). CEBS: a comprehensive annotated database of toxicological data. Nucleic Acids Res. 45, D964–D971. doi: 10.1093/nar/gkw1077
Lee, L. S., Seng, K. Y., Wang, L. Z., Yong, W. P., Hee, K. H., Soh, T. I., et al. (2016). Phenotyping of UGT1A1 activity using raltegravir predicts pharmacokinetics and toxicity of irinotecan in FOLFIRI. PLoS ONE 11:e0147681. doi: 10.1371/journal.pone.0147681
Li, D., Wang, Y., and Han, K. (2012). Recent density functional theory model calculations of drug metabolism by cytochrome P450. Coord Chem. Rev. 256, 1137–1150. doi: 10.1016/j.ccr.2012.01.016
Li, J., Du, H., Wu, Z., Su, H., Liu, G., Tang, Y., et al. (2016). Interactions of omeprazole-based analogues with cytochrome P450 2C19: a computational study. Mol. Biosyst. 12, 1913–1921. doi: 10.1039/C6MB00139D
Li, M., Zou, P., Tyner, K., and Lee, S. (2017). Physiologically based pharmacokinetic (PBPK) modeling of pharmaceutical nanoparticles. AAPS J. 19, 26–42. doi: 10.1208/s12248-016-0010-3
Li, X., Wang, R., Liu, Y., Liu, Y., Zheng, H., Feng, Y., et al. (2017). Pharmacokinetic study of single- and multiple-dosing with metolazone tablets in healthy Chinese population. BMC Pharmacol. Toxicol. 18:73. doi: 10.1186/s40360-017-0178-x
Li, Y. F., Guo, H. Y., Yang, F., Zhou, L. G., Huang, X. H., Ding, H. Z., et al. (2017). Comparative pharmacokinetics of diaveridine in pigs and chickens following single intravenous and oral administration. J. Vet. Pharmacol. Ther. 40, 500–504. doi: 10.1111/jvp.12384
Lin, J., Sahakian, D. C., de Morais, S. M., Xu, J. J., Polzer, R. J., and Winter, S. M. (2003). The role of absorption, distribution, metabolism, excretion and toxicity in drug discovery. Curr. Top. Med. Chem. 3, 1125–1154. doi: 10.2174/1568026033452096
Lindstrom, A., Anantpadma, M., Baker, L., Raghavendra, N. M., Davey, R., and Davisson, V. J. (2018). Phenotypic prioritization of diphyllin derivatives that block filoviral cell entry by vacuolar (H+)-ATPase inhibition. Chemmedchem 13, 2664–2676. doi: 10.1002/cmdc.201800587
Luo, J., Lai, T., Guo, T., Chen, F., Zhang, L., Ding, W., et al. (2018). Synthesis and acaricidal activities of scopoletin phenolic ether derivatives: QSAR, molecular docking study and in Silico ADME predictions. Molecules 23:995. doi: 10.3390/molecules23050995
Ma, Y., Wei, H. Y., Zhang, Y. Z., Jin, W. Y., Li, H. L., Zhou, H., et al. (2017). Synthesis, bioactivity, 3D-QSAR studies of novel dibenzofuran derivatives as PTP-MEG2 inhibitors. Oncotarget 8, 38466–38481. doi: 10.18632/oncotarget.16595
Macalino, S. J., Gosu, V., Hong, S., and Choi, S. (2015). Role of computer-aided drug design in modern drug discovery. Arch. Pharm. Res. 38, 1686–1701. doi: 10.1007/s12272-015-0640-5
Malik, R., Mehta, P., Srivastava, S., Choudhary, B. S., and Sharma, M. (2016). Pharmacophore modeling, 3D-QSAR, and in silico ADME prediction of N-pyridyl and pyrimidine benzamides as potent antiepileptic agents. J. Recept. Signal. Transduct. Res. 37, 259–266. doi: 10.1080/10799893.2016.1217883
Maltarollo, V. G., Gertrudes, J. C., Oliveira, P. R., and Honorio, K. M. (2015). Applying machine learning techniques for ADME-Tox prediction: a review. Expert Opin. Drug Metab. Toxicol. 11, 259–271. doi: 10.1517/17425255.2015.980814
Maunz, A., Gutlein, M., Rautenberg, M., Vorgrimmler, D., Gebele, D., and Helma, C. (2013). lazar: a modular predictive toxicology framework. Front. Pharmacol. 4:38. doi: 10.3389/fphar.2013.00038
Merlot, C. (2010). Computational toxicology—a tool for early safety evaluation. Drug Discov. Today 15, 16–22. doi: 10.1016/j.drudis.2009.09.010
Mertens, B., Simon, C., Van Bossuyt, M., Onghena, M., Vandermarken, T., Van Langenhove, K., et al. (2016). Investigation of the genotoxicity of substances migrating from polycarbonate replacement baby bottles to identify chemicals of high concern. Food Chem. Toxicol. 89, 126–137. doi: 10.1016/j.fct.2016.01.009
Michielan, L., and Moro, S. (2010). Pharmaceutical perspectives of nonlinear QSAR strategies. J. Chem. Inf. Model. 50, 961–978. doi: 10.1021/ci100072z
Modi, S. (2003). Computational approaches to the understanding of ADMET properties and problems. Drug Discov. Today 8, 621–623. doi: 10.1016/S1359-6446(03)02739-9
Mohan, A., Kirubakaran, R., Parray, J. A., Sivakumar, R., Murugesh, E., and Govarthanan, M. (2020). Ligand-based pharmacophore filtering, atom based 3D-QSAR, virtual screening and ADME studies for the discovery of potential ck2 inhibitors. J. Mol. Struct. 1205:127670. doi: 10.1016/j.molstruc.2019.127670
Moj, D., Britz, H., Burhenne, J., Stewart, C. F., Egerer, G., Haefeli, W. E., et al. (2017). A physiologically based pharmacokinetic and pharmacodynamic (PBPK/PD) model of the histone deacetylase (HDAC) inhibitor vorinostat for pediatric and adult patients and its application for dose specification. Cancer Chemother. Pharmacol. 80, 1013–1026. doi: 10.1007/s00280-017-3447-x
Molina, I., Salvador, F., Sanchez-Montalva, A., Artaza, M. A., Moreno, R., Perin, L., et al. (2017). Pharmacokinetics of benznidazole in healthy volunteers and implications in future clinical trials. Antimicrob. Agents Chemother. 61, e01912–16. doi: 10.1128/AAC.01912-16
Mondal, S., Mandal, S. M., Mondal, T. K., and Sinha, C. (2017). Spectroscopic characterization, antimicrobial activity, DFT computation and docking studies of sulfonamide schiff bases. J. Mol. Struct. 1127, 557–567. doi: 10.1016/j.molstruc.2016.08.011
Moroy, G., Martiny, V. Y., Vayer, P., Villoutreix, B. O., and Miteva, M. A. (2012). Toward in silico structure-based ADMET prediction in drug discovery. Drug Discov. Today 17, 44–55. doi: 10.1016/j.drudis.2011.10.023
Muster, W., Breidenbach, A., Fischer, H., Kirchner, S., Muller, L., and Pahler, A. (2008). Computational toxicology in drug development. Drug Discov. Today 13, 303–310. doi: 10.1016/j.drudis.2007.12.007
Nan, J., Hao, H., Xie, S., Pan, Y., Xi, C., Mao, F., et al. (2017). Pharmacokinetic and pharmacodynamic integration and modeling of acetylkitasamycin in swine for clostridium perfringens. J. Vet. Pharmacol. Ther. 40, 641–655. doi: 10.1111/jvp.12404
Nandekar, P. P., Khomane, K., Chaudhary, V., Rathod, V. P., Borkar, R. M., Bhandi, M. M., et al. (2016). Identification of leads for antiproliferative activity on MDA-MB-435 human breast cancer cells through pharmacophore and CYP1A1-mediated metabolism. Eur. J. Med. Chem. 115, 82–93. doi: 10.1016/j.ejmech.2016.02.061
Nasab, R. R., Mansourian, M., Hassanzadeh, F., and Shahlaei, M. (2018). Exploring the interaction between epidermal growth factor receptor tyrosine kinase and some of the synthesized inhibitors using combination of in-silico and in-vitro cytotoxicity methods. Res. Pharm. Sci. 13, 509–522. doi: 10.4103/1735-5362.245963
Nestorov, I. (2003). Whole body pharmacokinetic models. Clin. Pharmacokinet. 42, 883–908. doi: 10.2165/00003088-200342100-00002
Niederalt, C., Kuepfer, L., Solodenko, J., Eissing, T., Siegmund, H. U., Block, M., et al. (2017). A generic whole body physiologically based pharmacokinetic model for therapeutic proteins in PK-sim. J. Pharmacokinet. Pharmacodyn. 45, 235–257. doi: 10.1007/s10928-017-9559-4
Nisha, C. M., Kumar, A., Vimal, A., Bai, B. M., Pal, D., and Kumar, A. (2016). Docking and ADMET prediction of few GSK-3 inhibitors divulges 6-bromoindirubin-3-oxime as a potential inhibitor. J. Mol. Graph Model. 65, 100–107. doi: 10.1016/j.jmgm.2016.03.001
Niu, L., Ding, L., Lu, C., Zuo, F., Yao, K., Xu, S., et al. (2016). Flavokawain A inhibits Cytochrome P450 in in vitro metabolic and inhibitory investigations. J. Ethnopharmacol. 191, 350–359. doi: 10.1016/j.jep.2016.06.039
Nosrati, M., Shakeran, Z., and Shakeran, Z. (2018). Frangulosid as a novel hepatitis B virus DNA polymerase inhibitor: a virtual screening study. In Silico Pharmacol. 6:10. doi: 10.1007/s40203-018-0047-3
Olguin, H. J., Martinez, H. O., Perez, C. F., Mendiola, B. R., Espinosa, L. R., Pacheco, J. L. C., et al. (2017). Pharmacokinetics of sildenafil in children with pulmonary arterial hypertension. World J. Pediatr. 13, 588–592. doi: 10.1007/s12519-017-0043-4
Onghena, M., Van Hoeck, E., Van Loco, J., Ibanez, M., Cherta, L., Portoles, T., et al. (2015). Identification of substances migrating from plastic baby bottles using a combination of low-resolution and high-resolution mass spectrometric analysers coupled to gas and liquid chromatography. J. Mass Spectrom. 50, 1234–1244. doi: 10.1002/jms.3644
Onghena, M., van Hoeck, E., Vervliet, P., Scippo, M. L., Simon, C., van Loco, J., et al. (2014). Development and application of a non-targeted extraction method for the analysis of migrating compounds from plastic baby bottles by GC-MS. Food Addit. Contam. Part A Chem. Anal. Control Expo. Risk Assess. 31, 2090–2102. doi: 10.1080/19440049.2014.979372
Overington, J. (2009). ChEMBL. an interview with john overington, team leader, chemogenomics at the European bioinformatics institute outstation of the European molecular biology laboratory (EMBL-EBI). interview by wendy A. Warr. J. Comput. Aided Mol. Des. 23, 195–198. doi: 10.1007/s10822-009-9260-9
Padari, H., Metsvaht, T., Germovsek, E., Barker, C. I., Kipper, K., Herodes, K., et al. (2018). Pharmacokinetics of penicillin g in preterm and term neonates. Antimicrob. Agents Chemother. 62, 1995–2000. doi: 10.1128/AAC.02238-17
Parrott, N. J., Yu, L. J., Takano, R., Nakamura, M., and Morcos, P. N. (2016). Physiologically based absorption modeling to explore the impact of food and gastric pH changes on the pharmacokinetics of alectinib. AAPS J. 18, 1464–1474. doi: 10.1208/s12248-016-9957-3
Patel, C. N., Kumar, S. P., Rawal, R. M., Patel, D. P., Gonzalez, F. J., and Pandya, H. A. (2020). A multiparametric organ toxicity predictor for drug discovery. Toxicol. Mech. Methods 30, 159–166. doi: 10.1080/15376516.2019.1681044
Patel, D. B., Darji, D. G., Patel, K. R., Rajani, D. P., Rajani, S. D., and Patel, H. D. (2020). Synthesis of novel quinoline-thiosemicarbazide hybrids and evaluation of their biological activities, molecular docking, molecular dynamics, pharmacophore model studies, and ADME-tox properties. J. Heterocyclic Chem. 57, 1183–1200. doi: 10.1002/jhet.3855
Patel, N., Jain, S., and Lin, S. (2016). Transdermal iontophoretic delivery of tacrine hydrochloride: correlation between in vitro permeation and in vivo performance in rats. Int. J. Pharm. 513, 393–403. doi: 10.1016/j.ijpharm.2016.09.038
Pathak, S. M., Schaefer, K. J., Jamei, M., and Turner, D. B. (2019). Biopharmaceutic IVIVE-mechanistic modeling of single- and two-phase in vitro experiments to obtain drug-specific parameters for incorporation into PBPK models. J. Pharm. Sci. 108, 1604–1618. doi: 10.1016/j.xphs.2018.11.034
Patlewicz, G., Jeliazkova, N., Safford, R. J., Worth, A. P., and Aleksiev, B. (2008). An evaluation of the implementation of the cramer classification scheme in the toxtree software. SAR QSAR Environ. Res. 19, 495–524. doi: 10.1080/10629360802083871
Patterson, D. E., Cramer, R. D., Ferguson, A. M., Clark, R. D., and Weinberger, L. E. (1996). Neighborhood behavior: a useful concept for validation of “molecular diversity” descriptors. J. Med. Chem. 39, 3049–3059. doi: 10.1021/jm960290n
Perdaems, N., Blasco, H., Vinson, C., Chenel, M., Whalley, S., Cazade, F., et al. (2010). Predictions of metabolic drug-drug interactions using physiologically based modelling: two cytochrome P450 3A4 substrates coadministered with ketoconazole or verapamil. Clin. Pharmacokinet. 49, 239–258. doi: 10.2165/11318130-000000000-00000
Perez-Nueno, V. I., and Ritchie, D. W. (2011). Using consensus-shape clustering to identify promiscuous ligands and protein targets and to choose the right query for shape-based virtual screening. J. Chem. Inf. Model. 51, 1233–1248. doi: 10.1021/ci100492r
Petrescu, A. M., Paunescu, V., and Ilia, G. (2019). The antiviral activity and cytotoxicity of 15 natural phenolic compounds with previously demonstrated antifungal activity. J. Environ. Sci. Health B 54, 498–504. doi: 10.1080/03601234.2019.1574176
Pilari, S., Gaub, T., Block, M., and Gorlitz, L. (2017). Development of physiologically based organ models to evaluate the pharmacokinetics of drugs in the testes and the thyroid gland. CPT Pharmacometr. Syst. Pharmacol. 6, 532–542. doi: 10.1002/psp4.12205
Pires, D. E., Blundell, T. L., and Ascher, D. B. (2015). pkCSM: predicting small-molecule pharmacokinetic and toxicity properties using graph-based signatures. J. Med. Chem. 58, 4066–4072. doi: 10.1021/acs.jmedchem.5b00104
Poirier, D., Roy, J., Cortes-Benitez, F., and Dutour, R. (2016). Targeting cytochrome P450 (CYP) 1B1 with steroid derivatives. Bioorg. Med. Chem. Lett. 26, 5272–5276. doi: 10.1016/j.bmcl.2016.09.046
Polepally, A. R., Brundage, R. C., Remmel, R. P., Leppik, I. E., Pennell, P. B., White, J. R., et al. (2018). Lamotrigine pharmacokinetics following oral and stable-labeled intravenous administration in young and elderly adult epilepsy patients: effect of age. Epilepsia 59, 1718–1726. doi: 10.1111/epi.14519
Prabhu, S. V., and Singh, S. K. (2019). Identification of potential dual negative allosteric modulators of group I mGluR family: a shape based screening, ADME prediction, induced fit docking and molecular dynamics approach against neurodegenerative diseases. Curr. Top. Med. Chem. 19, 2687–2707. doi: 10.2174/1568026619666191105112800
Pradeep, P., Povinelli, R. J., White, S., and Merrill, S. J. (2016). An ensemble model of QSAR tools for regulatory risk assessment. J. Cheminform 8:48. doi: 10.1186/s13321-016-0164-0
Prokop, J. W., Lazar, J., Crapitto, G., Smith, D. C., Worthey, E. A., and Jacob, H. J. (2017). Molecular modeling in the age of clinical genomics, the enterprise of the next generation. J. Mol. Model. 23:75. doi: 10.1007/s00894-017-3258-3
Putta, S., and Beroza, P. (2007). Shapes of things: computer modeling of molecular shape in drug discovery. Curr. Top. Med. Chem. 7, 1514–1524. doi: 10.2174/156802607782194770
Rachar, V., Czejka, M., Kitzmueller, M., Buchner, P., Lichtneckert, M., Greil, R., et al. (2016). Assessment of pharmacokinetic interaction between capecitabine and cetuximab in metastatic colorectal cancer patients. Anticancer Res. 36, 4715–4723. doi: 10.21873/anticanres.11026
Ramatenki, V., Dumpati, R., Vadija, R., Vellanki, S., Potlapally, S. R., Rondla, R., et al. (2016). Targeting the ubiquitin-conjugating enzyme E2D4 for cancer drug discovery-a structure-based approach. J. Chem. Biol. 10, 51–67. doi: 10.1007/s12154-016-0164-6
Ramatenki, V., Dumpati, R., Vadija, R., Vellanki, S., Potlapally, S. R., Rondla, R., et al. (2017). Identification of new lead molecules against UBE2NL enzyme for cancer therapy. Appl. Biochem. Biotechnol. 182, 1497–1517. doi: 10.1007/s12010-017-2414-7
Rambiritch, V., Naidoo, P., Maharaj, B., and Pillai, G. (2016). Population pharmacokinetic modeling of glibenclamide in poorly controlled South African type 2 diabetic subjects. Clin. Pharmacol. 8, 83–92. doi: 10.2147/CPAA.S102676
Rawat, R., and Verma, S. M. (2020). High-throughput virtual screening approach involving pharmacophore mapping, ADME filtering, molecular docking and MM-GBSA to identify new dual target inhibitors of PfDHODH and PfCytbc1 complex to combat drug resistant malaria. J. Biomol. Struct. Dyn. doi: 10.1080/07391102.2020.1784288. [Epub ahead of print].
Reddy, K. K., Singh, S. K., Tripathi, S. K., Selvaraj, C., and Suryanarayanan, V. (2013). Shape and pharmacophore-based virtual screening to identify potential cytochrome P450 sterol 14 alpha-demethylase inhibitors. J. Recept. Signal. Transd. 33, 234–243. doi: 10.3109/10799893.2013.789912
Richard, A. M., and Williams, C. R. (2002). Distributed structure-searchable toxicity (DSSTox) public database network: a proposal. Mutat. Res. 499, 27–52. doi: 10.1016/S0027-5107(01)00289-5
Roman, M., Roman, D. L., Ostafe, V., Ciorsac, A., and Isvoran, A. (2018). Computational assessment of pharmacokinetics and biological effects of some anabolic and androgen steroids. Pharm. Res. 35:41. doi: 10.1007/s11095-018-2353-1
Romanski, M., Kasprzyk, A., Karbownik, A., and Glowka, F. K. (2016). Ocular disposition of treosulfan and its active epoxy-transformers following intravenous administration in rabbits. Drug Metab. Pharmacokinet. 31, 356–362. doi: 10.1016/j.dmpk.2016.07.001
Romanski, M., Kasprzyk, A., Walczak, M., Ziolkowska, A., and Glowka, F. (2017). Disposition of treosulfan and its active monoepoxide in a bone marrow, liver, lungs, brain, and muscle: studies in a rat model with clinical relevance. Eur. J. Pharm. Sci. 109, 616–623. doi: 10.1016/j.ejps.2017.09.011
Rostami-Hodjegan, A. (2012). Physiologically based pharmacokinetics joined with in vitro-in vivo extrapolation of ADME: a marriage under the arch of systems pharmacology. Clin. Pharmacol. Ther. 92, 50–61. doi: 10.1038/clpt.2012.65
Rowland, M., Balant, L., and Peck, C. (2004). Physiologically based pharmacokinetics in drug development and regulatory science: a workshop report (Georgetown University, Washington, DC, May 29-30, 2002). AAPS PharmSci. 6:E6. doi: 10.1208/ps060106
Rowland, M., Peck, C., and Tucker, G. (2011). Physiologically-based pharmacokinetics in drug development and regulatory science. Annu. Rev. Pharmacol. Toxicol. 51, 45–73. doi: 10.1146/annurev-pharmtox-010510-100540
Roy, K., Mitra, I., Kar, S., Ojha, P. K., Das, R. N., and Kabir, H. (2012). Comparative studies on some metrics for external validation of QSPR models. J. Chem. Inf. Model. 52, 396–408. doi: 10.1021/ci200520g
Saba, N., and Seal, A. (2018). Identification of a less toxic vinca alkaloid derivative for use as a chemotherapeutic agent, based on in silico structural insights and metabolic interactions with CYP3A4 and CYP3A5. J. Mol. Model. 24:82. doi: 10.1007/s00894-018-3611-1
Sangle, G. V., Patil, M., Deshmukh, N. J., Shengule, S. A., Kamble, S., Vuppalavanchu, K. K., et al. (2018). Evaluation of pharmacokinetic and pharmacodynamic parameters following single dose of sitagliptin in healthy Indian males. Eur. J. Clin. Pharmacol. 74, 561–569. doi: 10.1007/s00228-018-2433-5
Sanni, D. M., and Fatoki, T. H. (2017). Computational evaluation of pharmacokinetics and potential protein targets of ginger (Zingiber officinale). J. Microbiol. Biotechnol. Res. 7:14. doi: 10.24896/jmbr.2017713
Sanni, D. M., Fatoki, T. H., Kolawole, A. O., and Akinmoladun, A. C. (2017). Xeronine structure and function: computational comparative mastery of its mystery. In Silico Pharmacol. 5:8. doi: 10.1007/s40203-017-0028-y
Santes-Palacios, R., Romo-Mancillas, A., Camacho-Carranza, R., and Espinosa-Aguirre, J. J. (2016). Inhibition of human and rat CYP1A1 enzyme by grapefruit juice compounds. Toxicol. Lett. 258, 267–275. doi: 10.1016/j.toxlet.2016.07.023
Sasahara, K., Mashima, A., Yoshida, T., and Chuman, H. (2015). Molecular dynamics and density functional studies on the metabolic selectivity of antipsychotic thioridazine by cytochrome P450 2D6: connection with crystallographic and metabolic results. Bioorg. Med. Chem. 23, 5459–5465. doi: 10.1016/j.bmc.2015.07.043
Schlender, J. F., Meyer, M., Thelen, K., Krauss, M., Willmann, S., Eissing, T., et al. (2016). Development of a whole-body physiologically based pharmacokinetic approach to assess the pharmacokinetics of drugs in elderly individuals. Clin. Pharmacokinet. 55, 1573–1589. doi: 10.1007/s40262-016-0422-3
Schmidt, U., Struck, S., Gruening, B., Hossbach, J., Jaeger, I. S., Parol, R., et al. (2009). SuperToxic: a comprehensive database of toxic compounds. Nucleic Acids Res. 37, D295–D299. doi: 10.1093/nar/gkn850
Schmitt, W., and Willmann, S. (2004). Physiology-based pharmacokinetic modeling: ready to be used. Drug Discov. Today Technol. 1, 449–456. doi: 10.1016/j.ddtec.2004.09.006
Schyman, P., Liu, R., Desai, V., and Wallqvist, A. (2017). vNN web server for ADMET predictions. Front. Pharmacol. 8:889. doi: 10.3389/fphar.2017.00889
Selick, H. E., Beresford, A. P., and Tarbit, M. H. (2002). The emerging importance of predictive ADME simulation in drug discovery. Drug Discov. Today 7, 109–116. doi: 10.1016/S1359-6446(01)02100-6
Shaaban, S., Vervandier-Fasseur, D., Andreoletti, P., Zarrouk, A., Richard, P., Negm, A., et al. (2018). Cytoprotective and antioxidant properties of organic selenides for the myelin-forming cells, oligodendrocytes. Bioorg. Chem. 80, 43–56. doi: 10.1016/j.bioorg.2018.05.019
Shaik, M., Shaik, S., and Kilari, E. K. (2018). Population pharmacokinetics of gliclazide in normal and diabetic rabbits. Biopharm. Drug Dispos. 39, 265–274. doi: 10.1002/bdd.2132
Shaji, D. (2018). Molecular docking studies of human MCT8 protein with soy isoflavones in allan-herndon-dudley syndrome (AHDS). J. Pharm. Anal. 8, 318–323. doi: 10.1016/j.jpha.2018.07.001
Shakhnovich, V., Smith, P. B., Guptill, J. T., James, L. P., Collier, D. N., Wu, H., et al. (2018). Obese children require lower doses of pantoprazole than nonobese peers to achieve equal systemic drug exposures. J. Pediatr. 193, 102–108.e101. doi: 10.1016/j.jpeds.2017.10.011
Shan, Q., Fan, J., Wang, J., Zhu, X., Yin, Y., and Zheng, G. (2017). Pharmacokinetics of enrofloxacin after oral, intramuscular and bath administration in crucian carp (Carassius auratus gibelio). J. Vet. Pharmacol. Ther. 41, 159–162. doi: 10.1111/jvp.12428
Shan, Q., Wang, J., Yang, F., Ma, L., Yin, Y., Liu, S., et al. (2018). Pharmacokinetics of cefquinome in crucian carp (Carassius auratus gibelio) after oral, intramuscular, intraperitoneal, and bath administration. J. Vet. Pharmacol. Ther. 41, 734–738. doi: 10.1111/jvp.12510
Shang, J., Sun, H., Liu, H., Chen, F., Tian, S., Pan, P., et al. (2017). Comparative analyses of structural features and scaffold diversity for purchasable compound libraries. J. Cheminform. 9:25. doi: 10.1186/s13321-017-0212-4
Sheridan, R. P., Feuston, B. P., Maiorov, V. N., and Kearsley, S. K. (2004). Similarity to molecules in the training set is a good discriminator for prediction accuracy in QSAR. J. Chem. Inf. Comput. Sci. 44, 1912–1928. doi: 10.1021/ci049782w
Shukla, R., Singh, A. P., Sonar, P. K., Mishra, M., and Saraf, S. K. (2016). Schiff bases of benzothiazol-2-ylamine and thiazolo[5,4-b] pyridin-2-ylamine as anticonvulsants: synthesis, characterization and toxicity profiling. Cent. Nerv. Syst. Agents Med. Chem. 16, 240–248. doi: 10.2174/1871524916666160428110728
Silva, G., Zuravski, L., Duarte, M., Machado, M., and Oliveira, L. (2019). Fluconazole induces genotoxicity in cultured human peripheral blood mononuclear cells via immunomodulation of TNF-α, IL-6, and IL-10: new challenges for safe therapeutic regimens. Immunopharmacol. Immunotoxicol. 41:123–129. doi: 10.1080/08923973.2019.1566357
Silva-Junior, E. F., Aquino, T. M., and Araujo-Junior, J. X. (2017). Quantum mechanical (QM) calculations applied to ADMET drug prediction: a review. Curr. Drug Metab. 18, 511–526. doi: 10.2174/1389200218666170316094514
Simhadri Vsdna, N., Muniappan, M., Kannan, I., and Viswanathan, S. (2017). Phytochemical analysis and docking study of compounds present in a polyherbal preparation used in the treatment of dermatophytosis. Curr. Med. Mycol. 3, 6–14. doi: 10.29252/cmm.3.4.6
Simoneau, C., Van den Eede, L., and Valzacchi, S. (2012). Identification and quantification of the migration of chemicals from plastic baby bottles used as substitutes for polycarbonate. Food Addit. Contam. Part A Chem. Anal. Control Expo. Risk Assess. 29, 469–480. doi: 10.1080/19440049.2011.644588
Song, J. C., Gao, H., Qiu, H. B., Chen, Q. B., Cai, M. H., Zhang, M. Z., et al. (2018). The pharmacokinetics of dexmedetomidine in patients with obstructive jaundice: a clinical trial. PLoS ONE 13:e0207427. doi: 10.1371/journal.pone.0207427
Song, L., Yu, Z., Xu, Y., Li, X., Liu, X., Liu, D., et al. (2020). Preliminary physiologically based pharmacokinetic modeling of renally cleared drugs in chinese pregnant women. Biopharm. Drug Dispos. 41:248–267. doi: 10.1002/bdd.2243
Stillhart, C., Parrott, N. J., Lindenberg, M., Chalus, P., Bentley, D., and Szepes, A. (2017). Characterising drug release from immediate-release formulations of a poorly soluble compound, basmisanil, through absorption modelling and dissolution testing. AAPS J. 19, 827–836. doi: 10.1208/s12248-017-0060-1
Sun, H., and Scott, D. O. (2010). Structure-based drug metabolism predictions for drug design. Chem. Biol. Drug Des. 75, 3–17. doi: 10.1111/j.1747-0285.2009.00899.x
Sushko, I., Novotarskyi, S., Korner, R., Pandey, A. K., Cherkasov, A., Li, J., et al. (2010). Applicability domains for classification problems: benchmarking of distance to models for ames mutagenicity set. J. Chem. Inf. Model. 50, 2094–2111. doi: 10.1021/ci100253r
Tabeshpour, J., Sahebkar, A., Zirak, M. R., Zeinali, M., Hashemzaei, M., Rakhshani, S., et al. (2018). Computer-aided drug design and drug pharmacokinetic prediction: a mini-review. Curr. Pharm. Des. 24, 3014–3019. doi: 10.2174/1381612824666180903123423
Takac, M. J.-M., Casimiro Magina, J. D., and Takac, T. (2019). Evaluation of phenylethylamine type entactogens and their metabolites relevant to ecotoxicology - a QSAR study. Acta Pharm. 69, 563–584. doi: 10.2478/acph-2019-0038
Tan, B. H., Ahemad, N., Pan, Y., Palanisamy, U. D., Othman, I., Yiap, B. C., et al. (2018). Cytochrome P450 2C9-natural antiarthritic interactions: evaluation of inhibition magnitude and prediction from in vitro data. Biopharm. Drug Dispos. 39, 205–217. doi: 10.1002/bdd.2127
Tang, Y., Zhu, W., Chen, K., and Jiang, H. (2006). New technologies in computer-aided drug design: toward target identification and new chemical entity discovery. Drug Discov. Today Technol. 3, 307–313. doi: 10.1016/j.ddtec.2006.09.004
Tao, L., Zhang, P., Qin, C., Chen, S. Y., Zhang, C., Chen, Z., et al. (2015). Recent progresses in the exploration of machine learning methods as in-silico ADME prediction tools. Adv Drug Deliv. Rev. 86, 83–100. doi: 10.1016/j.addr.2015.03.014
Taroncher, M., Tolosa, J., Prosperini, A., and Ruiz, M. J. (2018). In silico and in vitro prediction of the toxicological effects of individual and combined mycotoxins. Food Chem. Toxicol. 122, 194–202. doi: 10.1016/j.fct.2018.09.055
Taxak, N., Prasad, K. C., and Bharatam, P. V. (2013). Mechanistic insights into the bioactivation of phenacetin to reactive metabolites: a DFT study. Comput. Theor. Chem. 1007, 48–56. doi: 10.1016/j.comptc.2012.11.018
Teorell, T. (1935). Studies on the “Diffusion Effect” upon ionic distribution. Some theoretical considerations. Proc. Natl. Acad. Sci. U.S.A. 21, 152–161. doi: 10.1073/pnas.21.3.152
Thiel, C., Cordes, H., Conde, I., Castell, J. V., Blank, L. M., and Kuepfer, L. (2016). Model-based contextualization of in vitro toxicity data quantitatively predicts in vivo drug response in patients. Arch. Toxicol. 91, 865–883. doi: 10.1007/s00204-016-1723-x
Tian, S., Li, Y., Wang, J., Zhang, J., and Hou, T. (2011). ADME evaluation in drug discovery. 9. Prediction of oral bioavailability in humans based on molecular properties and structural fingerprints. Mol. Pharm. 8, 841–851. doi: 10.1021/mp100444g
T'Jollyn, H., Vermeulen, A., and Van Bocxlaer, J. (2018). PBPK and its virtual populations: the impact of physiology on pediatric pharmacokinetic predictions of tramadol. AAPS J. 21:8. doi: 10.1208/s12248-018-0277-7
Tripathi, A. C., Upadhyay, S., Paliwal, S., and Saraf, S. K. (2018). N1-benzenesulfonyl-2-pyrazoline hybrids in neurological disorders: syntheses, biological screening and computational studies. EXCLI J. 17, 126–148. doi: 10.17179/excli2017-871
Trivedi, A., Dave, S., Luhana, K., and Trivedi, S. (2020). Design and prediction of absorption, distribution, metabolism, excretion and toxicity properties of drugs for H1N1 Flu (Swine Flu) using in-silico approaches. Biosci. Biotech. Res. Commun. 13, 326–334. doi: 10.21786/bbrc/13.1/52
Trivedi, A., Dave, S. M., and Patel, J. S. (2019). Design and prediction of absorption, distribution, metabolism, excretion and toxicity properties of drugs for dengue fever using in-silico approaches. Biosci. Biotech. Res. Commun. 12, 1201–1209. doi: 10.21786/bbrc/12.4/45
Uba, A. I., and Yelekci, K. (2017). Identification of potential isoform-selective histone deacetylase inhibitors for cancer therapy: a combined approach of structure-based virtual screening, ADMET prediction and molecular dynamics simulation assay. J. Biomol. Struct. Dyn. 36, 3231–3245. doi: 10.1080/07391102.2017.1384402
Vaidhyanathan, S., Wang, X., Crison, J., Varia, S., Gao, J. Z. H., Saxena, A., et al. (2018). Bioequivalence comparison of pediatric dasatinib formulations and elucidation of absorption mechanisms through integrated PBPK modeling. J. Pharm. Sci. 108, 741–749. doi: 10.1016/j.xphs.2018.11.005
Van Bossuyt, M., Van Hoeck, E., Raitano, G., Vanhaecke, T., Benfenati, E., Mertens, B., et al. (2018). Performance of in silico models for mutagenicity prediction of food contact materials. Toxicol. Sci. 163, 632–638. doi: 10.1093/toxsci/kfy057
van de Waterbeemd, H., and Gifford, E. (2003). ADMET in silico modelling: towards prediction paradise? Nat. Rev. Drug Discov. 2, 192–204. doi: 10.1038/nrd1032
Vaz, R. J., Li, Y., Munson, M., Elliot, M., and Thurairatnam, S. (2018). Amelioration of mechanism-based inactivation of CYP3A4 by a H-PGDS inhibitor. Bioorg. Med. Chem. Lett. 28, 3046–3049. doi: 10.1016/j.bmcl.2018.07.049
Verheyen, G. R., Braeken, E., Van Deun, K., and Van Miert, S. (2017). Evaluation of existing (Q)SAR models for skin and eye irritation and corrosion to use for REACH registration. Toxicol. Lett. 265, 47–52. doi: 10.1016/j.toxlet.2016.11.007
Verma, S. K., and Thareja, S. (2017). Structure based comprehensive modelling, spatial fingerprints mapping and ADME screening of curcumin analogues as novel ALR2 inhibitors. PLoS ONE 12:e0175318. doi: 10.1371/journal.pone.0175318
Voelkner, N. M. F., Voelkner, A., Costa, J., Sy, S. K. B., Hermes, J., Weitzel, J., et al. (2017). Dermal pharmacokinetics of pyrazinamide determined by microdialysis sampling in rats. Int. J. Antimicrob. Agents 51, 190–196. doi: 10.1016/j.ijantimicag.2017.10.001
Vuu, I., Coles, L. D., Maglalang, P., Leppik, I. E., Worrell, G., Crepeau, D., et al. (2016). Intravenous topiramate: pharmacokinetics in dogs with naturally occurring epilepsy. Front. Vet. Sci. 3:107. doi: 10.3389/fvets.2016.00107
Wang, J., and Hou, T. (2009). Recent advances on in silico ADME modeling. Annu. Rep. Comput. Chem. 5, 101–127. doi: 10.1016/S1574-1400(09)00505-2
Wang, J., Peng, H., Kong, J., Zhao, T., Zhang, S., and Cao, X. (2017). Pharmacokinetic profile of ceftiofur hydrochloride injection in lactating holstein dairy cows. J. Vet. Pharmacol. Ther. 41, 301–306. doi: 10.1111/jvp.12469
Wang, J., Xue, J., Kong, J., Li, J., Zhang, S., and Cao, X. (2018a). Evaluation of pharmacokinetic properties of vitacoxib in fasted and fed horses. J. Vet. Pharmacol. Ther. 41, 843–847. doi: 10.1111/jvp.12703
Wang, J., Zhao, T., Sun, X., Liu, Y., Zhu, J., Zhang, S., et al. (2018b). Pharmacokinetics of tildipirosin in beagle dogs. J. Vet. Pharmacol. Ther. 41, E49–E52. doi: 10.1111/jvp.12453
Wang, J. H., Endsley, A. N., Green, C. E., and Matin, A. C. (2016). Utilizing native fluorescence imaging, modeling and simulation to examine pharmacokinetics and therapeutic regimen of a novel anticancer prodrug. BMC Cancer 16:524. doi: 10.1186/s12885-016-2508-6
Wang, J. Y., Chen, H., Wang, Y. Y., Wang, X. Q., Chen, H. Y., Zhang, M., et al. (2017). Network pharmacological mechanisms of Vernonia anthelmintica (L.) in the treatment of vitiligo: isorhamnetin induction of melanogenesis via up-regulation of melanin-biosynthetic genes. BMC Syst. Biol. 11:103. doi: 10.1186/s12918-017-0486-1
Wang, L., Hai, Y., An, L., Chen, J., Liang, R., and He, X. (2017). Rapid screening the potential mechanism-based inhibitors of CYP3A4 from Tripterygium wilfordi based on computer approaches combined with in vitro bioassay. Bioorg. Med. Chem. 25, 2689–2700. doi: 10.1016/j.bmc.2017.03.037
Wang, L., Yue, H., Huang, N., Xue, G., Liu, W., and Xin, H. (2018). Human cytochrome P450 enzyme inhibition profile of three flavonoids isolated from Psoralea corylifolia: in silico predictions and experimental validation. N J. Chem. 42, 10922–10934. doi: 10.1039/C7NJ00884H
Wang, S., Sun, H., Liu, H., Li, D., Li, Y., and Hou, T. (2016). ADMET evaluation in drug discovery. 16. predicting hERG blockers by combining multiple pharmacophores and machine learning approaches. Mol. Pharm. 13, 2855–66. doi: 10.1021/acs.molpharmaceut.6b00471
Wang, Y., Lin, W., Wu, N., He, X., Wang, J., Feng, Z., et al. (2018). An insight into paracetamol and its metabolites using molecular docking and molecular dynamics simulation. J. Mol. Model. 24:243. doi: 10.1007/s00894-018-3790-9
Wang, Y., Xing, J., Xu, Y., Zhou, N., Peng, J., Xiong, Z., et al. (2015). In silico ADME/T modelling for rational drug design. Q. Rev. Biophys. 48, 488–515. doi: 10.1017/S0033583515000190
Waseem, D., Butt, A. F., Haq, I. U., Bhatti, M. H., and Khan, G. M. (2017). Daru 25:8. doi: 10.1186/s40199-017-0174-0
Watanabe, Y., Fukuyoshi, S., Kato, K., Hiratsuka, M., Yamaotsu, N., Hirono, S., et al. (2017). Investigation of substrate recognition for cytochrome P450 1A2 mediated by water molecules using docking and molecular dynamics simulations. J. Mol. Graph Model. 74, 326–336. doi: 10.1016/j.jmgm.2017.04.006
Watford, S., Ly Ly, P., Wignall, J., Shin, R., Martin, M. T., and Friedman, K. P. (2019). ToxRefDB version 2.0: improved utility for predictive and retrospective toxicology analyses. Reprod. Toxicol. 89, 145–158. doi: 10.1016/j.reprotox.2019.07.012
White, J., Wrzesinski, C., Green, M., Johnson, G. T., McCluskey, J. D., Abritis, A., et al. (2016). A novel method for deriving thresholds of toxicological concern for vaccine constituents. Toxicol. Mech. Methods 26, 270–275. doi: 10.3109/15376516.2016.1170250
Williams, A. J., Grulke, C. M., Edwards, J., McEachran, A. D., Mansouri, K., Baker, N. C., et al. (2017). The comptox chemistry dashboard: a community data resource for environmental chemistry. J. Cheminform. 9:61. doi: 10.1186/s13321-017-0247-6
Wishart, D. S. (2007). Improving early drug discovery through ADME modelling: an overview. Drugs R D 8, 349–362. doi: 10.2165/00126839-200708060-00003
Wishart, D. S., Feunang, Y. D., Guo, A. C., Lo, E. J., Marcu, A., Grant, J. R., et al. (2018). DrugBank 5.0: a major update to the drugbank database for 2018. Nucleic Acids Res. 46, D1074–D1082. doi: 10.1093/nar/gkx1037
Wishart, D. S., Knox, C., Guo, A. C., Cheng, D., Shrivastava, S., Tzur, D., et al. (2008). DrugBank: a knowledgebase for drugs, drug actions and drug targets. Nucleic Acids Res. 36, D901–D906. doi: 10.1093/nar/gkm958
Wishart, D. S., Knox, C., Guo, A. C., Shrivastava, S., Hassanali, M., Stothard, P., et al. (2006). DrugBank: a comprehensive resource for in silico drug discovery and exploration. Nucleic Acids Res. 34, D668–D672. doi: 10.1093/nar/gkj067
Wu, Z., Lei, T., Shen, C., Wang, Z., Cao, D., and Hou, T. (2019). ADMET evaluation in drug discovery. 19. reliable prediction of human cytochrome P450 inhibition using artificial intelligence approaches. J. Chem. Inf. Model. 59, 4587–4601. doi: 10.1021/acs.jcim.9b00801
Xiao, H., Peng, H., Zhao, T., Kong, J., Xue, J., Wang, J., et al. (2018). The pharmacokinetics of moxidectin following intravenous and topical administration to swine. J. Vet. Pharmacol. Ther. 42, 111–115. doi: 10.1111/jvp.12693
Xu, Y., Pei, J., and Lai, L. (2017). Deep learning based regression and multiclass models for acute oral toxicity prediction with automatic chemical feature extraction. J. Chem. Inf. Model. 57, 2672–2685. doi: 10.1021/acs.jcim.7b00244
Yadav, S., Pandey, S. K., Singh, V. K., Goel, Y., Kumar, A., and Singh, S. M. (2017). Molecular docking studies of 3-bromopyruvate and its derivatives to metabolic regulatory enzymes: implication in designing of novel anticancer therapeutic strategies. PLoS ONE 12:e0176403. doi: 10.1371/journal.pone.0176403
Yamashita, F., and Hashida, M. (2004). In silico approaches for predicting ADME properties of drugs. Drug Metab. Pharmacokinet. 19, 327–338. doi: 10.2133/dmpk.19.327
Yang, F., Wang, B., Liu, Z., Xia, X., Wang, W., Yin, D., et al. (2017). Prediction of a therapeutic dose for buagafuran, a potent anxiolytic agent by physiologically based pharmacokinetic/pharmacodynamic modeling starting from pharmacokinetics in rats and human. Front. Pharmacol. 8:683. doi: 10.3389/fphar.2017.00683
Yang, H., Lou, C., Sun, L., Li, J., Cai, Y., Wang, Z., et al. (2018a). admetSAR 2.0: web-service for prediction and optimization of chemical ADMET properties. Bioinformatics 35, 1067–1069. doi: 10.1093/bioinformatics/bty707
Yang, H., Sun, L., Li, W., Liu, G., and Tang, Y. (2018b). In silico prediction of chemical toxicity for drug design using machine learning methods and structural alerts. Front. Chem. 6:30. doi: 10.3389/fchem.2018.00129
Yang, H., Sun, L., Wang, Z., Li, W., Liu, G., and Tang, Y. (2018c). ADMETopt: a web server for ADMET optimization in drug design via scaffold hopping. J. Chem. Inf. Model. 58, 2051–2056. doi: 10.1021/acs.jcim.8b00532
Yang, Z. Y., Yang, Z. J., Dong, J., Wang, L. L., Zhang, L. X., Ding, J. J., et al. (2019). Structural analysis and identification of colloidal aggregators in drug discovery. J. Chem. Inf. Model. 59, 3714–3726. doi: 10.1021/acs.jcim.9b00541
Ye, N., Monk, S. A., Daga, P., Bender, D. M., Rosen, L. B., Mullen, J., et al. (2018). Clinical bioavailability of the novel BACE1 inhibitor lanabecestat (AZD3293): assessment of tablet formulations versus an oral solution and the impact of gastric pH on pharmacokinetics. Clin. Pharmacol. Drug Dev. 7, 233–243. doi: 10.1002/cpdd.422
Yongye, A. B., and Medina-Franco, J. L. (2013). Systematic characterization of structure-activity relationships and ADMET compliance: a case study. Drug Discov. Today 18, 732–739. doi: 10.1016/j.drudis.2013.04.002
Yu, H., and Adedoyin, A. (2003). ADME-Tox in drug discovery: integration of experimental and computational technologies. Drug Discov. Today 8, 852–861. doi: 10.1016/S1359-6446(03)02828-9
Yu, Y., Zhou, Y. F., Sun, J., Shi, W., Liao, X. P., and Liu, Y. H. (2017). Pharmacokinetic and pharmacodynamic modeling of sarafloxacin against avian pathogenic Escherichia coli in muscovy ducks. BMC Vet. Res. 13:47. doi: 10.1186/s12917-017-0964-0
Yugandhar, P., Kumar, K. K., Neeraja, P., and Savithramma, N. (2017). Isolation, characterization and in silico docking studies of synergistic estrogen receptor a anticancer polyphenols from Syzygium alternifolium (Wt.) Walp. J. Intercult. Ethnopharmacol. 6, 296–310. doi: 10.5455/jice.20170709031835
Zhang, C., Ding, S., Fang, Y., Zhang, L., Hu, W., Lu, J., et al. (2017). Iohexol clearance for determination of glomerular filtration rate in cynomolgus monkeys (Macaca fascicularis). J Am Assoc Lab Anim Sci. 56, 330–333.
Zhang, L., Zhang, H., Ai, H. X., Hu, H., Li, S. M., Zhao, J., et al. (2018). Applications of machine learning methods in drug toxicity prediction. Curr. Top. Med. Chem. 18, 987–997. doi: 10.2174/1568026618666180727152557
Zhang, L., Zhao, L., Liu, Y., Liu, J., and Li, X. (2017). Pharmacokinetics of tilmicosin in healthy pigs and in pigs experimentally infected with haemophilus parasuis. J. Vet. Sci. 18, 431–437. doi: 10.4142/jvs.2017.18.4.431
Zhang, N., Wu, Y., Huang, Z., Yao, L., Zhang, L., Cai, Q., et al. (2017). The PK-PD relationship and resistance development of danofloxacin against mycoplasma gallisepticum in an in vivo infection model. Front. Microbiol. 8:926. doi: 10.3389/fmicb.2017.00926
Zhang, P., Hao, H., Li, J., Ahmad, I., Cheng, G., Chen, D., et al. (2016). The epidemiologic and pharmacodynamic cutoff values of tilmicosin against haemophilus parasuis. Front. Microbiol. 7:385. doi: 10.3389/fmicb.2016.00385
Zhao, P., Zhang, L., Grillo, J. A., Liu, Q., Bullock, J. M., Moon, Y. J., et al. (2011). Applications of physiologically based pharmacokinetic (PBPK) modeling and simulation during regulatory review. Clin. Pharmacol. Ther. 89, 259–267. doi: 10.1038/clpt.2010.298
Zhou, D., Afzelius, L., Grimm, S. W., Andersson, T. B., Zauhar, R. J., and Zamora, I. (2006). Comparison of methods for the prediction of the metabolic sites for CYP3A4-mediated metabolic reactions. Drug Metab. Dispos. 34, 976–983. doi: 10.1124/dmd.105.008631
Zhou, Q., Zhang, G., Wang, Q., Liu, W., Huang, Y., Yu, P., et al. (2017). Pharmacokinetic/pharmacodynamic modeling of tulathromycin against pasteurella multocida in a porcine tissue cage model. Front. Pharmacol. 8:392. doi: 10.3389/fphar.2017.00392
Zhu, J., Wang, J., Yu, H., Li, Y., and Hou, T. (2011). Recent developments of in silico predictions of oral bioavailability. Comb. Chem. High Throughput Screen. 14, 362–374. doi: 10.2174/138620711795508368
Zhu, L., Zhang, Y., Yang, J., Wang, Y., Zhang, J., Zhao, Y., et al. (2016). Prediction of the pharmacokinetics and tissue distribution of levofloxacin in humans based on an extrapolated PBPK model. Eur. J. Drug Metab. Pharmacokinet. 41, 395–402. doi: 10.1007/s13318-015-0271-8
Zhuang, J., Xing, X., Wang, D., Du, Z., Wang, J., Dong, Y., et al. (2018). Toxicity assessment of the extractables from multi-layer coextrusion poly ethylene bags exposed to pH=5 solution containing 4% benzyl alcohol and 0.1M sodium acetate. Regul. Toxicol. Pharmacol. 94, 47–56. doi: 10.1016/j.yrtph.2018.01.015
Zhuang, S., Zhang, L., Zhan, T., Lu, L., Zhao, L., Wang, H., et al. (2018). Binding specificity determines the cytochrome P450 3A4 mediated enantioselective metabolism of metconazole. J. Phys. Chem. B 122, 1176–1184. doi: 10.1021/acs.jpcb.7b11170
Keywords: drug discovery, pre-clinical studies, ADMET, pharmacokinetics, PBPK modeling
Citation: Wu F, Zhou Y, Li L, Shen X, Chen G, Wang X, Liang X, Tan M and Huang Z (2020) Computational Approaches in Preclinical Studies on Drug Discovery and Development. Front. Chem. 8:726. doi: 10.3389/fchem.2020.00726
Received: 29 March 2020; Accepted: 14 July 2020;
Published: 11 September 2020.
Edited by:
Simone Brogi, University of Pisa, ItalyReviewed by:
Hongbin Yang, University of Cambridge, United KingdomBruno Villoutreix, Institut National de la Santé et de la Recherche Médicale (INSERM), France
Copyright © 2020 Wu, Zhou, Li, Shen, Chen, Wang, Liang, Tan and Huang. This is an open-access article distributed under the terms of the Creative Commons Attribution License (CC BY). The use, distribution or reproduction in other forums is permitted, provided the original author(s) and the copyright owner(s) are credited and that the original publication in this journal is cited, in accordance with accepted academic practice. No use, distribution or reproduction is permitted which does not comply with these terms.
*Correspondence: Zunnan Huang, zn_huang@yahoo.com
†These authors have contributed equally to this work