- Laboratory of Protein Chemistry and Biochemistry, Department of Cell Biology, University of Brasilia, Brasilia, Brazil
The central nervous system is responsible for an array of cognitive functions such as memory, learning, language, and attention. These processes tend to take place in distinct brain regions; yet, they need to be integrated to give rise to adaptive or meaningful behavior. Since cognitive processes result from underlying cellular and molecular changes, genomics and transcriptomics assays have been applied to human and animal models to understand such events. Nevertheless, genes and RNAs are not the end products of most biological functions. In order to gain further insights toward the understanding of brain processes, the field of proteomics has been of increasing importance in the past years. Advancements in liquid chromatography-tandem mass spectrometry (LC-MS/MS) have enabled the identification and quantification of thousands of proteins with high accuracy and sensitivity, fostering a revolution in the neurosciences. Herein, we review the molecular bases of explicit memory in the hippocampus. We outline the principles of mass spectrometry (MS)-based proteomics, highlighting the use of this analytical tool to study memory formation. In addition, we discuss MS-based targeted approaches as the future of protein analysis.
Introduction
Memory is one of the most important components of cognition and it refers to the capacity to store and retrieve new information about the physical world (Kandel et al., 2014). Memory emerged very early in the evolutionary history of the nervous system, and has played a central role in helping organisms to adapt and survive the challenges faced in their environments (Emes et al., 2008; Ryan and Grant, 2009). This cognitive process has reached one of its most complex forms in humans, where deficits can have devastating consequences for the individual. In Alzheimer disease, for example, the build-up of misfolded proteins in the brain disrupts the connectivity between nerve cells, leading to memory impairments and dementia (Ross and Poirier, 2004; Musunuri et al., 2014). In Huntington's disease, the expansion of a CAG triplet in the huntingtin gene leads to motor abnormalities and cognitive decline such as memory loss (Paulsen, 2011). As a result, memory has been the focus of intensive investigation in different research fields such as neurobiology, psychology, and anthropology (Zola-Morgan et al., 1986; Rempel-Clower et al., 1996; Tronson and Taylor, 2007).
The first scientific studies attempting to understand the cellular and molecular basis of memory formation date back to the earliest neuroscientists like Santiago Ramón y Cajal, who formulated the idea that information storage occurs by the physical rearrangement in the connections between nerve cells (Mayford et al., 2012; Bailey et al., 2015). To this day, a plethora of studies in organisms ranging from the nematode Caenorhabditis elegans to humans have established that this postulation is correct; hence, Ramón y Cajal's hypothesis has been used as a major conceptual framework for the understanding of memory formation (Rongo and Kaplan, 1999; Tsai et al., 2005; Cooke and Bliss, 2006; Groszer et al., 2008). However, a complete comprehension of the mechanisms underlying information storage is still lacking, since (1) we do not know all the molecular processes that happen at the synapses and (2) how they are related to one another.
Several techniques have been employed to address such questions (Tsien et al., 1996b; Wang et al., 2003; Boyden et al., 2005; Whitlock et al., 2006; Grover et al., 2009; Zheng et al., 2015). For instance, restricted gene knockout, which consists in the deletion of a gene by homologous recombination in a specific region or tissue, has been extensively used in the past few years (Gu et al., 1994; Li et al., 1994; Tsien, 2016). One common type of restricted gene knockout exploits the Cre/loxP system, where the bacteriophage P1 enzyme Cre recombinase catalyzes homologous recombination between the loxP recognition sequences (Tsien et al., 1996a). In a typical experiment, a line of mice having a gene of interest flanked by the loxP sequences is generated. Next, a second line of transgenic mice is created, where the Cre recombinase is expressed under the control of a neural promoter sequence that is activated only in specific brain regions. By crossing the two lines of animals, the targeted gene will be deleted in those cells that express the Cre recombinase (McHugh et al., 1996; Gong et al., 2007; Taniguchi et al., 2011). Because this system is restricted to a particular tissue and region, it became a powerful tool to investigate the underlying mechanisms of memory formation. Nevertheless, this assay tends to be extremely laborious, expensive and confined to a specific sequence of interest.
Recently, high-resolution mass spectrometry (MS) has emerged as an alternative to classical assays such as restricted gene knockout. MS has a high-throughput capability and can identify thousands of proteins and their post-translational modifications (PTMs) in a single experiment (Low et al., 2013; Lin et al., 2015). Notably, the power of this analytical tool has been used to identify novel proteins that participate in important cellular activities and disease states (Old et al., 2005; de Godoy et al., 2008; Azimifar et al., 2014; Tyanova et al., 2016). In this review, we present an overview of the cellular processes responsible for memory formation in the hippocampus. In addition, we highlight how MS-based approaches can be used to study the molecular processes encompassing memory formation.
Molecular Basis of Explicit Memory
Memory has always been of great interest to the scientific community. Early studies on animal models and patients with lesions on specific brain regions revealed that this cognitive function is not an unitary cerebral faculty, but can be organized into at least two general forms: implicit and explicit memory (Barco et al., 2006; Kandel, 2012). Implicit or non-declarative memory stores information that does not rely on conscious effort, like habits and motor skills (Lynch, 2004). This type of memory has been widely studied on the marine mollusk Aplysia califorica, and, in mammals, many neural structures have been associated with it, such as the amygdala, the cerebellum, and reflex pathways (Barzilai et al., 1989; Hawkins et al., 2006; Voss and Paller, 2008). Explicit or declarative memory, on the other hand, deals with the storage and recall of knowledge about facts and events, which depend on conscious awareness. Here, the main neural substrates are the neocortex and the hippocampus (Eichenbaum, 2000; Kandel, 2001).
The hippocampus, a seahorse-shaped structure located in the medial temporal lobe of the vertebrate brain, has long been used as a model system to understand the mechanisms of explicit memory formation (Bliss and Collingridge, 1993; Moser et al., 1993; Whitlock et al., 2006). It can be anatomically divided into three distinct regions: the dentate gyrus (DG), the hippocampus proper—composed of the CA1, CA2, and CA3 areas—and the subiculum (Strange et al., 2014). Each of these sections harbors different populations of cells that communicate through two main pathways, namely the direct perforant pathway and the trisynaptic circuit, also known as the indirect perforant pathway (Kandel et al., 2012). The former transmits multimodal sensory and spatial information directly from the entorhinal cortex (EC) to the CA1 area, a major output of the hippocampus. By contrast, in the trisynaptic circuit, information is sent from the EC to the CA1 area following this route: EC–DG–CA3–CA1 (Figure 1) (Neves et al., 2008; Deng et al., 2010; Suh et al., 2011). Experiments carried out in those distinct populations of cells have demonstrated that they work as cellular engrams (i.e., cells that are the loci of information storage) (Ryan et al., 2015; Tonegawa et al., 2015).
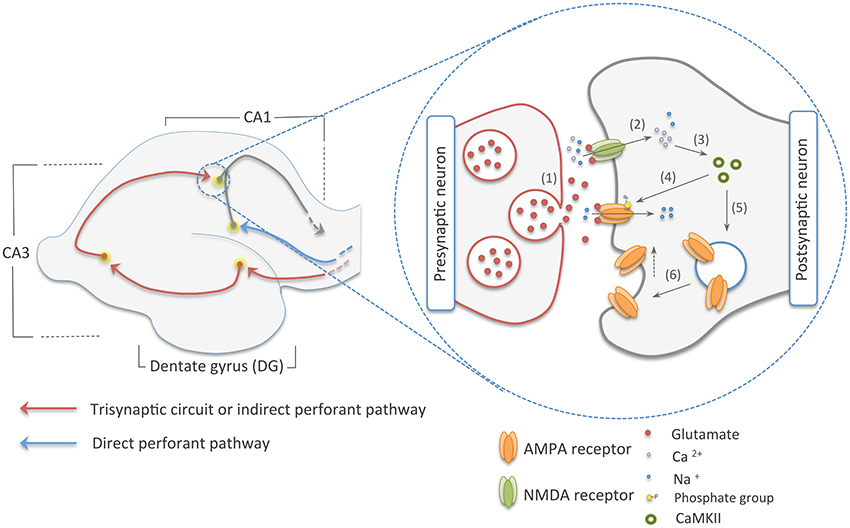
Figure 1. The two main pathways to the CA1 area of the hippocampus on the left, and early-phase NMDA dependent-LTP on the right. The red arrows in the picture on the left show the trisynaptic circuit of the hippocampus, where multimodal sensory and spatial information coming from the entorhinal cortex (EC) is relayed to the CA1 area following this route: EC–DG–CA3–CA1. In blue, we illustrated the direct perforant pathway, which directly connects the EC to the CA1 region. On the picture in right, we show an illustration of the early-phase LTP. Here, (1) glutamate from the presynaptic neuron is released into the synaptic cleft. (2) This neurotransmitter reaches ionic channels of the postsynaptic cell causing depolarization of this neuron by the influx on sodium and calcium cations. (3) Calcium, in its turn, activates CaMKII that (4) phosphorylates ionic channels in the PSDs and (5, 6) induces the addition of AMPA receptors to the postsynaptic membrane, increasing synaptic efficiency.
The molecular mechanisms underlying memory formation only began to be comprehended after the discovery of long-term potentiation (LTP), which is a form of experience-dependent plasticity and is believed to be the major cellular correlate of learning and memory (Govindarajan et al., 2011; Nabavi et al., 2014). Induction of LTP occurs throughout the hippocampus via distinct signaling cascades, which can differ greatly based on the animal age and region of the hippocampus (Yasuda et al., 2003; Malenka and Bear, 2004). N-methyl-D-aspartate (NMDA)-dependent LTP is the best-characterized type of LTP (Lüscher and Malenka, 2012). This type of LTP occurs at the major excitatory synapses and follows the Hebbian model of neural selectivity, which postulates that synaptic plasticity is dependent on the simultaneous association of pre and postsynaptic neurons (Bi and Poo, 2001; Lu et al., 2001). Furthermore, NMDA-dependent LTP can be divided into two distinct phases: an early phase that facilitates the transmission of action potentials and lasts from minutes to hours, and a late phase, which is dependent on protein synthesis and lasts from hours to a life time (Abel et al., 1997; Govindarajan et al., 2011; Granger et al., 2013).
The early phase of NMDA-dependent LTP, also referred as the induction phase, begins by the release of glutamate from the presynaptic terminal into the synaptic cleft. Glutamate, the main excitatory neurotransmitter in the brain, diffuses through the cleft and reaches the post-synaptic neuron, where it binds to ligand-gated ion channels. The interaction between the neurotransmitter and the ionotropic NMDA receptor results in the influx of Ca2+ and Na+ into the cell (Schiller et al., 1998; Dingledine et al., 1999; Castillo, 2012). The Na+ helps to bring about a depolarization of the postsynaptic neuron that last a few milliseconds, while the Ca2+ promotes the activation of protein kinases such as calcium/calmodulin-dependent protein kinase (CaMKII) (Lisman et al., 2012; Lüscher and Malenka, 2012). CaMKII and other kinases prompt the introduction of other ionotropic channels called α-amino-3-hydroxy-5-methyl-4-isoxazolepropionic acid (AMPA) receptors into the postsynaptic density (PSD)—a structure located in the tip of the dendritic spine of postsynaptic cells composed of ionic receptors and a dense network of proteins that regulate synapse strength (Hayashi et al., 2000; Chen et al., 2008; Lu et al., 2010). This traffic of new AMPA receptors to the membrane ultimately leads to an improvement in synaptic communication (Figure 1).
If the activation of the pre and postsynaptic neurons persists for longer periods of time—something that can also be accomplished in vitro by repeated stimulation of the cells by high frequency tetanus pulses of 100 Hz, a number of signaling cascades are activated, leading to protein synthesis and synapse rearrangement (Nguyen et al., 1994; Hölscher et al., 1997; Ryan et al., 2015). This is known as the late phase LTP, also called the expression phase. In this stage, the rise in Ca2+ ions inside the cell, caused by the constant release of glutamate by the presynaptic cell, induces the increase in the production of cyclic adenosine monophosphate (cAMP) by adenyl cyclase (Wong et al., 1999; Poser and Storm, 2001). cAMP, in turn, activates protein kinase A (PKA) that switches on mitogen activated protein kinase (MAPK) (Abel et al., 1997; Roberson et al., 1999). This kinase is translocated to the nucleus and phosphorylates cAMP response element binding protein 1 (CREB-1), an important transcription factor (Viola et al., 2000; Patterson et al., 2001). The phosphorylation activates CREB-1, resulting in increased transcription of a number of target genes and their subsequent translation into proteins responsible for the formation of new synaptic connections (Figure 2) (Deisseroth et al., 1996; Ahmed and Frey, 2005; Benito and Barco, 2010).
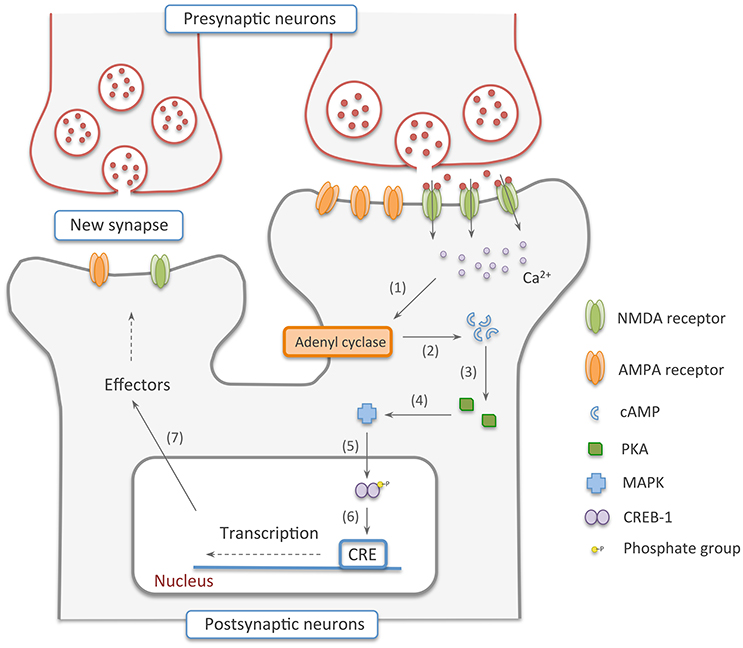
Figure 2. Late-phase NMDA dependent-LTP. In this stage, (1, 2) Ca2+ ions inside the cell recruit adenyl cyclase to produce cAMP. (3, 4) Cyclic adenosine monophosphate, in turn, activates PKA that switches on MAPK. (5) This kinase is translocated to the nucleus and phosphorylates CREB-1, an important transcription factor. (6, 7) The phosphorylation activates CREB-1, resulting in increased transcription of a number of target genes and their subsequent translation into proteins responsible for the formation of new synaptic connections.
The signaling pathways outlined above provide a broad understanding about the order and timing of molecular events governing the early and late phase of NMDA-dependent LTP. However, this model lacks information about regulatory changes that might be occurring translationally and post-translationally. In addition, this picture only focuses on a limited number of molecular players and little or no information on their stoichiometry is known. In the next sections, we describe the use of mass spectrometry-based proteomics as a tool to help to elucidate those questions.
Mass Spectrometry (MS)-based Proteomics
Proteomics is a system-wide analysis of the proteins expressed in a specific cell, tissue, or organism at a given time (Anderson and Anderson, 1996; Zhang et al., 2013). Although the term proteomics relates to the use of any technology that seeks to interrogate a large number of proteins, it is nowadays used to refer to works where the central platform is mass spectrometry. Currently, the gold standard strategy in MS-based proteomics is shotgun proteomics (Mann and Kelleher, 2008; Domon and Aebersold, 2010). Here, a complex mixture of proteins is digested into peptides with a protease of interest, usually trypsin, which cleaves on the C-terminal side of lysine and arginine. Subsequently, the peptides are separated online by reverse-phase liquid chromatography (LC) and analyzed by mass spectrometers such as quadrupole/time-of-flight (QTOF), ion trap (IT), orbitrap (OT), or ion cyclotron resonance (ICR) (Marshall et al., 1998; Michalski et al., 2011; Thakur et al., 2011; Beck et al., 2015).
In a typical shotgun experiment, the LC-MS/MS run takes up to 120 min and is composed of thousands of cycles, each one made of a MS1 scan—also known as a full scan—that measures the peptides' mass to charge ratio (m/z) and intensity, and a MS2 or MS/MS scan. During the MS2 scan, the 20 most intense peptides in each cycle are fragmented in a collision cell, usually filled with an inert gas such as nitrogen or helium, and their spectra are again measured to obtain sequence information (Geiger et al., 2011; Thakur et al., 2011). Once acquired, the LC-MS/MS data are used in searches against databases containing peptides digested in silico to identify the proteins present in the sample(s) (Figure 3) (Sadygov et al., 2004).

Figure 3. Workflow of the gold standard strategy in shotgun proteomics. Here, peptides are separated online in a reverse-phase liquid chromatography and electrosprayed into the mass analyzer. The mass spectrometer measures the peptides' m/z and intensity in the MS1 cycle. Upon fragmentation, the product ions of each peptide are reanalyzed to obtain sequence information of the analyte in the MS2 cycle. Once this experimental data is acquired, information is searched against a database of the organism of interest to identify the proteins in the sample.
In addition to protein identification, mass spectrometry can be used to extract quantitative information from samples. Protein quantification can be absolute, if known amounts of a heavy analog of the analyte of interest is added prior to the analysis in a mass spectrometer, or relative, if samples in different states are compared (e.g., brain tissue from rodents trained in a behavioral paradigm vs. controls) (Kettenbach et al., 2011; Bantscheff et al., 2012). The most popular strategies for relative quantification are label-free, metabolic labeling, and chemical labeling (Figure 4).
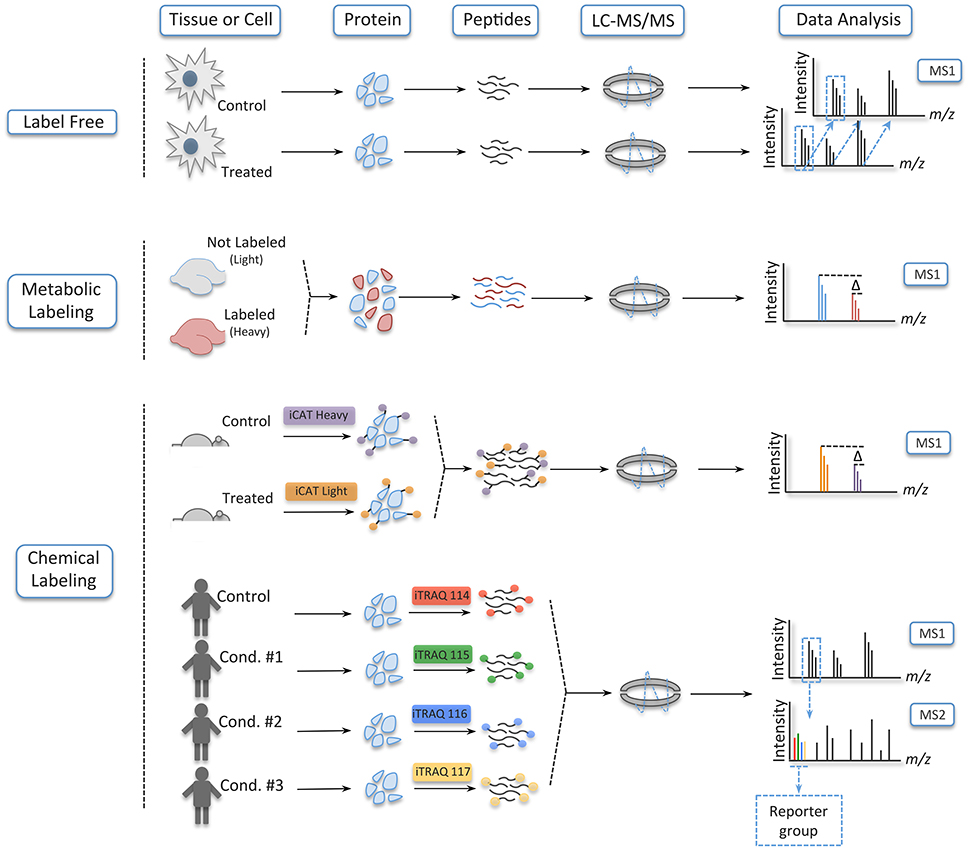
Figure 4. Different strategies to quantify peptides. In label free experiments, samples are digested and ran separately in a mass spectrometer; they are combined only in the data analysis. In metabolic labeling, on its turn, one of the conditions is grown in medium containing amino acids labeled with heavy isotopes or heavy nitrogen. Here, the samples are combined very early, and sample handling and analysis are done concomitantly. Lastly, in chemical labeling, tags are incorporated at the protein level, as is the case of iCAT, or at the peptide level with iTRAQ and TMT. In these strategies, the samples are combined early in the workflow of the experiment without increasing the complexity of the samples in the MS1, since the tags of iTRAQ and TMT are isobaric—being distinguished only upon fragmentation in a collision cell.
In label-free quantification, as the name suggests, no label is added to the samples, which are digested and run individually in the mass spectrometer—being the results computationally combined after the acquisition of the data (Filiou et al., 2012). In this strategy, quantification takes advantage of the area plotted over time for each ion as it elutes from the chromatographic column. Later, this extracted ion chromatogram (XIC) is aligned across different samples, and a ratio for each peptide is obtained. Another mode of quantification in label-free experiments is spectrum counting. Here, quantification is based on the number a particular peptide is fragmented during a LC-MS/MS run, which serves as a proxy for abundance and can be compared between conditions (Bantscheff et al., 2007; Hernández et al., 2012). Label-free is regarded as the least accurate strategy of relative quantification, but it has gained momentum due to its low cost, improvements in sample handling, refinement of the chromatographic setup, and development of software for accurate data analysis (Ong and Mann, 2005; Altelaar and Heck, 2012).
By contrast, in metabolic labeling, prior to protein extraction at least one of the conditions is labeled with a heavy stable isotope such as 15N or heavy amino acids such as lysine, arginine, or both (Ong, 2002; Rauniyar et al., 2013). The use of a heavy analog prevents the variation usually encountered in label-free experiments, since the samples are mixed, digested and analyzed simultaneously in the LC-MS/MS run. This can be accomplished because heavy (labeled) and light (non-labeled) peptides retain the same physicochemical properties (e.g., retention time during the LC), but a mass shift between them enables their distinction latter in the data analysis. The only exception to this rule is deuterium (2H), which is more hydrophilic than hydrogen; this creates a delay in the retention time between the labeled and non-labeled conditions (Yi et al., 2005). Here, as it is also the case in label-free experiments, quantification is acquired by the peak area ratios of the heavy and light peptides in the XIC (Ong, 2002; Bantscheff et al., 2007).
Even though metabolic labeling is the most accurate relative quantification strategy, it has a restricted capacity to multiplexing due to the limitation on the isotopes that can be added to an amino acid and the increase in sample complexity in the MS1 (Hebert et al., 2013). Chemical labeling, in its turn, is able to circumvent those limitations. To this day, many chemical labeling reagents have been developed, but the most used are isotope-coded affinity tags (iCAT), tandem mass tags (TMT), and isobaric tags for relative and absolute quantification (iTRAQ) (Gygi et al., 1999; Thompson et al., 2003; Ross, 2004). iCAT, which labels samples at the protein level, uses the tags that are composed of a reactive group that binds to reduced cysteine residues, a linker group that incorporates isotopes in the heavy reagent, and a biotin affinity group for the isolation of the iCAT-labeled peptides. In a typical experiment using iCAT, the reagent labels protein samples at two different conditions (the light and heavy version of the tags), which are then mixed together and enzymatically cleaved. Next, the peptides with the tags are enriched by avidin affinity chromatography and analyzed in a LC-MS/MS run. Here, quantification is obtained by the peak area ratios of the heavy and light peptides (Yi et al., 2005).
iCAT possesses the same limitation in multiplexing as metabolic labeling, yet iTRAQ and TMT enables from 8 to 10 samples, respectively, to be analyzed in a single experiment. iTRAQ and TMT are isobaric tags that label analytes at the peptide level. Their tags are composed of a reactive group that binds to the N-terminal of peptides and lysine residues, a balance group—which ensures that the same peptides in the different conditions elute together and are indistinguishable in the MS1 scan—and a reporter group. Unlike other quantitative strategies, quantification on iTRAQ and TMT is based on the intensity signal of the reporter group that is released from the analytes upon fragmentation in a collision cell. Nevertheless, chemical labeling also has limitations and some considerations have to be taken to get around these drawbacks (For in depth information on iTRAQ and TMT strategies see Bantscheff et al., 2008; Karp et al., 2010; Ting et al., 2011; Wenger et al., 2011).
Proteomic Studies of Memory
The shotgun quantitative strategies described above have fostered a revolution in many fields of biology such as cancer, immunology, and neuroscience by improving our understanding of the systemic cellular response of stimulated or disease states vs. control (Krüger et al., 2008; Dahlhaus et al., 2011; Geiger et al., 2012; Boersema et al., 2013; Meissner and Mann, 2014; Nascimento and Martins-de-Souza, 2015). Yet, when it comes to the proteomic study of memory, very few MS-based experiments have been carried out so far. Some authors believe that such discrepancy is due to the difficulty to characterize proteins that are genuinely associated with this cognitive process due to high biological variability among individuals within areas related to memory such as the hippocampus (Dieterich and Kreutz, 2015). Nevertheless, this explanation falls short, since transcriptomics assays to investigate memory have been conducted before with success (Ponomarev et al., 2010; Bero et al., 2014).
In addition, two recent proteomic studies have demonstrated that those kinds of experiments are feasible. In the first one, Borovok et al. used a radial arm maze (RAM) paradigm to understand the process of memory consolidation in the hippocampus of mice (Borovok et al., 2016). In summary, the RAM behavioral task works as follows: a central circular chamber is connected to eight long arms, which are open to the animal upon the removal of a guillotine door. In the end of four of the arms, for example, visual clues are set to mark where the food are placed. During the experiment, the animals are positioned in the central chamber and learn to associate the visual clues to where the baits are (Götz and Ittner, 2008). Hence, using this paradigm, Borovok and colleagues extracted proteins at different time points during the learning period and quantified those samples by the label-free strategy. They showed the protein abundance profiles during spatial memory formation in the hippocampus, and demonstrated a clear correlation between temporal memory formation and proteins belonging to specific functional groups using gene ontology and signaling pathways analysis.
In the other study, Rao-Ruiz et al. used contextual fear conditioning to understand membrane protein dynamics of mice at 1 and 4 h after training (Rao-Ruiz et al., 2015). Contextual fear conditioning is a form of associative learning, which works as this: mice are placed inside a chamber with a stainless steel grid floor, so that a foot shock can be administered after the mice have explored the box (called delayed-shock paradigm) or as soon as the animal has been placed into the cage (called immediate-shock paradigm) (Rao-Ruiz et al., 2011). Using both training protocols, in addition to untrained control mice, Rao-Ruiz et al., 2015 compared the groups using iTRAQ at 1 and 4 h after conditioning. They found no difference in protein abundance between the experimental groups at 1 h after training; however, 164 proteins in the delayed-shock group and 273 proteins in the immediate-shock group exhibited different abundances at the 4 h time point. Functional protein group analysis of those analytes were carried out, which showed that the most prominent proteins are linked to endocytosis, glutamate signaling and neurotransmitter metabolism (For other proteomic studies on memory see Henninger et al., 2007; Piccoli et al., 2007; Freeman et al., 2009; Zheng et al., 2009; Rosenegger et al., 2010; Li et al., 2011; Monopoli et al., 2011; Kähne et al., 2012; Monje et al., 2012; Lee et al., 2013; Matsuura et al., 2013; Trinidad et al., 2013; Végh et al., 2014; Zhang et al., 2014).
Targeted Proteomics
The shotgun strategy has been considered the gold standard approach to identify and quantify proteins in complex proteomes (Aebersold and Mann, 2003; Smith et al., 2013). However, this discovery-based method has inherently poor reproducibility due to its stochastic selection of parent ions and its modest dynamic range, which renders the information acquired incomplete or biased toward a subset of proteins (Domon and Aebersold, 2006). These drawbacks have lead to the maintenance of immunochemical assays such as antibody arrays and ELISA—techniques that quantitatively measure a small set of targets with high accuracy and reproducibility—very popular in the scientific community (Edwards et al., 2011). However, advancements in targeted proteomic approaches such as selected reaction monitoring (SRM), also known as multiple reaction monitoring (MRM), will likely change this scenario (Picotti et al., 2013).
Selected reaction monitoring (SRM) is a strategy that dates back to the development of quadrupole mass analyzers (Yost and Enke, 1979). However, only in the last few years this method has reached its full potential as a high precision analytical tool (Kuhn et al., 2004). As in shotgun proteomics, SRM uses peptides as surrogates to protein identification and quantification, but this approach is not a discovery assay. SRM is a hypothesis-driven strategy like other classical techniques, and prior information about the analytes being interrogated need to be known, regardless if its sources are from previous discovery experiments, proteomic data repositories such as PeptideAtlas and PRIDE or predicted by using computational algorithms (Deutsch et al., 2008; Jones et al., 2008; Lange et al., 2008; Gallien et al., 2011; Picotti and Aebersold, 2012).
The classical SRM experiment is carried out in triple quadrupole instruments, where the three analyzers are aligned in tandem. The first and third mass analyzers, also known as Q1 and Q3, respectively, function as ion filters, selecting only the analytes with a specific m/z value. The second quadrupole (Q2), on the other hand, is used as a collision cell to fragment the parent ions selected in the Q1; the combination of parent ion and its fragments is called a “transition” (Figure 5) (Gallien et al., 2011). In addition, such experiments usually use an isotopic-labeled internal analog for all the peptides under investigation. This confers SRM assays quality control for the sample preparation procedures as well as compensation for discrepancies that can arise through ion suppression and spray instability (Brun et al., 2007; Carr et al., 2014). Quantification per-se is obtained through the peak area ratios of the transition ions from the endogenous peptide and its heavy analog (Brun et al., 2007).

Figure 5. Selected reaction monitoring (SRM) pipeline. In a typical SRM experiment, a triple quadrupole instrument is used. Here, the first and third mass analyzers, also known as Q1 and Q3, respectively, function as ion filters, selecting only the analytes with a specific m/z value at a predetermined retention time. The second quadrupole (Q2), in its turn, works as a collision cell to fragment the parent ions selected in the Q1. In this strategy, quantification per-se is obtained through the peak area ratios of the transition ions from an endogenous peptide and a reference analog.
Those features confer targeted proteomics with the high accuracy and reproducibility usually required in the biological and clinical sciences. A number of recent studies have taken advantage of the power of SRM to provide a deeper understanding of mechanisms and structures related to memory (Munton et al., 2007; Trinidad et al., 2013; Lowenthal et al., 2015). For example, Cheng et al. compared purified postsynaptic densities of the forebrain and cerebellum (Cheng et al., 2006). Employing the ICAT strategy, they identified 43 proteins displaying statistically significant abundance change between the PSDs and monitored 32 of them using synthetic isotopic-labeled analogs in a SRM pipeline. Measuring their molar abundances, Cheng et al. demonstrated that these postsynaptic regions possess unexpected stoichiometric differences, something that might also be true to hippocampal PSDs.
Conclusion
The study of the molecular mechanisms of memory is an area of great interest to the scientific community, where the first studies date back to Santiago Ramón y Cajal and the experimental psychologists such as the behaviorists (Moser et al., 2015). Nonetheless, much of the work carried out until the present time has concentrated in the use of classical methods of molecular biology, genetics, and biochemistry, which target a limited number of analytes, and are time consuming and laborious. On that account, we have a restricted understanding about the processes that happen in the brain cells and how they are related to one another during memory formation (Dieterich and Kreutz, 2015).
Shotgun and targeted proteomics approaches might be able to speed up this process. Discovery proteomics allows thousands of proteins, including PTMs such as phosphorylation, to be identified and quantified in a single experiment (Wu et al., 2011; Weekes et al., 2014; Humphrey et al., 2015; Peshkin et al., 2015; Sharma et al., 2015; Wühr et al., 2015). The SRM strategy, on its turn, is able to determine with high accuracy and reproducibility the stoichiometry of proteins in a sample, multiplexing up to 100 peptides per experiment (Kuhn et al., 2011; Ebhardt et al., 2015). Moreover, as the number of discovery experiments increase, improving our understanding of the molecular players involved in memory formation, more accurate measurements will be required to develop models to understand how proteins work together. Hence, we expect that soon targeted proteomics will become the flagship of MS-based proteomics.
Author Contributions
All authors listed, have made substantial, direct and intellectual contribution to the work, and approved it for publication.
Conflict of Interest Statement
The authors declare that the research was conducted in the absence of any commercial or financial relationships that could be construed as a potential conflict of interest.
Acknowledgments
We thank Maurício Henriques Pontes and Micaella Pereira da Fonseca for their helpful discussions and for critically reading this manuscript.
References
Abel, T., Nguyen, P. V., Barad, M., Deuel, T. A., Kandel, E. R., and Bourtchouladze, R. (1997). Genetic demonstration of a role for PKA in the late phase of LTP and in hippocampus-based long-term memory. Cell 88, 615–626. doi: 10.1016/S0092-8674(00)81904-2
Aebersold, R., and Mann, M. (2003). Mass spectrometry-based proteomics. Nature 422, 198–207. doi: 10.1038/nature01511
Ahmed, T., and Frey, J. U. (2005). Plasticity-specific phosphorylation of CaMKII, MAP-kinases and CREB during late-LTP in rat hippocampal slices in vitro. Neuropharmacology 49, 477–492. doi: 10.1016/j.neuropharm.2005.04.018
Altelaar, A. F. M., and Heck, A. J. R. (2012). Trends in ultrasensitive proteomics. Curr. Opin. Chem. Biol. 16, 206–213. doi: 10.1016/j.cbpa.2011.12.011
Anderson, N. G., and Anderson, N. L. (1996). Twenty years of two-dimensional electrophoresis: past, present and future. Electrophoresis 17, 443–453. doi: 10.1002/elps.1150170303
Azimifar, S. B., Nagaraj, N., Cox, J., and Mann, M. (2014). Cell-type-resolved quantitative proteomics of murine liver. Cell Metab. 20, 1076–1087. doi: 10.1016/j.cmet.2014.11.002
Bailey, C. H., Kandel, E. R., and Harris, K. M. (2015). Structural components of synaptic plasticity and memory consolidation. Cold Spring Harb. Perspect. Biol. 7:a021758. doi: 10.1101/cshperspect.a021758
Bantscheff, M., Boesche, M., Eberhard, D., Matthieson, T., Sweetman, G., and Kuster, B. (2008). Robust and sensitive iTRAQ quantification on an LTQ orbitrap mass spectrometer. Mol. Cell. Proteomics 7, 1702–1713. doi: 10.1074/mcp.m800029-mcp200
Bantscheff, M., Lemeer, S., Savitski, M. M., and Kuster, B. (2012). Quantitative mass spectrometry in proteomics: critical review update from 2007 to the present. Anal. Bioanal. Chem. 404, 939–965. doi: 10.1007/s00216-012-6203-4
Bantscheff, M., Schirle, M., Sweetman, G., Rick, J., and Kuster, B. (2007). Quantitative mass spectrometry in proteomics: a critical review. Anal. Bioanal. Chem. 389, 1017–1031. doi: 10.1007/s00216-007-1486-6
Barco, A., Bailey, C. H., and Kandel, E. R. (2006). Common molecular mechanisms in explicit and implicit memory. J. Neurochem. 97, 1520–1533. doi: 10.1111/j.1471-4159.2006.03870.x
Barzilai, A., Kennedy, T. E., Sweatt, J. D., and Kandel, E. R. (1989). 5-HT modulates protein synthesis and the expression of specific proteins during long-term facilitation in Aplysia sensory neurons. Neuron 2, 1577–1586. doi: 10.1016/0896-6273(89)90046-9
Beck, S., Michalski, A., Raether, O., Lubeck, M., Kaspar, S., Goedecke, N., et al. (2015). The impact II, a very high-resolution quadrupole time-of-flight instrument (QTOF) for deep shotgun proteomics. Mol. Cell. Proteomics 14, 2014–2029. doi: 10.1074/mcp.m114.047407
Benito, E., and Barco, A. (2010). CREB's control of intrinsic and synaptic plasticity: implications for CREB-dependent memory models. Trends Neurosci. 33, 230–240. doi: 10.1016/j.tins.2010.02.001
Bero, A. W., Meng, J., Cho, S., Shen, A. H., Canter, R. G., Ericsson, M., et al. (2014). Early remodeling of the neocortex upon episodic memory encoding. Proc. Natl. Acad. Sci. U.S.A. 111, 11852–11857. doi: 10.1073/pnas.1408378111
Bi, G.-Q., and Poo, M.-M. (2001). Synaptic modification by correlated activity: Hebb's postulate revisited. Annu. Rev. Neurosci. 24, 139–166. doi: 10.1146/annurev.neuro.24.1.139
Bliss, T. V., and Collingridge, G. L. (1993). A synaptic model of memory: long-term potentiation in the hippocampus. Nature 361, 31–39. doi: 10.1038/361031a0
Boersema, P. J., Geiger, T., Wisniewski, J. R., and Mann, M. (2013). Quantification of the N-glycosylated secretome by super-SILAC during breast cancer progression and in human blood samples. Mol. Cell. Proteomics 12, 158–171. doi: 10.1074/mcp.M112.023614
Borovok, N., Nesher, E., Levin, Y., Reichenstein, M., Pinhasov, A., and Michaelevski, I. (2016). Dynamics of hippocampal protein expression during long-term spatial memory formation. Mol. Cell. Proteomics 15, 523–541. doi: 10.1074/mcp.M115.051318
Boyden, E. S., Zhang, F., Bamberg, E., Nagel, G., and Deisseroth, K. (2005). Millisecond-timescale, genetically targeted optical control of neural activity. Nat. Neurosci. 8, 1263–1268. doi: 10.1038/nn1525
Brun, V., Dupuis, A., Adrait, A., Marcellin, M., Thomas, D., Court, M., et al. (2007). Isotope-labeled protein standards: toward absolute quantitative proteomics. Mol. Cell. Proteomics 6, 2139–2149. doi: 10.1074/mcp.m700163-mcp200
Carr, S. A., Abbatiello, S. E., Ackermann, B. L., Borchers, C., Domon, B., Deutsch, E. W., et al. (2014). Targeted peptide measurements in biology and medicine: best practices for mass spectrometry-based assay development using a fit-for-purpose approach. Mol. Cell. Proteomics 13, 907–917. doi: 10.1074/mcp.M113.036095
Castillo, P. E. (2012). Presynaptic LTP and LTD of excitatory and inhibitory synapses. Cold Spring Harb. Perspect. Biol. 4:a005728. doi: 10.1101/cshperspect.a005728
Chen, X., Winters, C., Azzam, R., Li, X., Galbraith, J. A., Leapman, R. D., et al. (2008). Organization of the core structure of the postsynaptic density. Proc. Natl. Acad. Sci. U.S.A. 105, 4453–4458. doi: 10.1073/pnas.0800897105
Cheng, D., Hoogenraad, C. C., Rush, J., Ramm, E., Schlager, M. A., Duong, D. M., et al. (2006). Relative and absolute quantification of postsynaptic density proteome isolated from rat forebrain and cerebellum. Mol. Cell. Proteomics 5, 1158–1170. doi: 10.1074/mcp.D500009-MCP200
Cooke, S. F., and Bliss, T. V. (2006). Plasticity in the human central nervous system. Brain 129, 1659–1673. doi: 10.1093/brain/awl082
Dahlhaus, M., Li, K. W., van der Schors, R. C., Saiepour, M. H., van Nierop, P., Heimel, J. A., et al. (2011). The synaptic proteome during development and plasticity of the mouse visual cortex. Mol. Cell. Proteomics 10:M110.005413. doi: 10.1074/mcp.M110.005413
de Godoy, L. M., Olsen, J. V., Cox, J., Nielsen, M. L., Hubner, N. C., Fröhlich, F., et al. (2008). Comprehensive mass-spectrometry-based proteome quantification of haploid versus diploid yeast. Nature 455, 1251–1254. doi: 10.1038/nature07341
Deisseroth, K., Bito, H., and Tsien, R. W. (1996). Signaling from synapse to nucleus: postsynaptic CREB phosphorylation during multiple forms of hippocampal synaptic plasticity. Neuron 16, 89–101. doi: 10.1016/S0896-6273(00)80026-4
Deng, W., Aimone, J. B., and Gage, F. H. (2010). New neurons and new memories: how does adult hippocampal neurogenesis affect learning and memory? Nat. Rev. Neurosci. 11, 339–350. doi: 10.1038/nrn2822
Deutsch, E. W., Lam, H., and Aebersold, R. (2008). PeptideAtlas: a resource for target selection for emerging targeted proteomics workflows. EMBO Rep. 9, 429–434. doi: 10.1038/embor.2008.56
Dieterich, D. C., and Kreutz, M. R. (2015). Proteomics of the synapse – a quantitative approach to neuronal plasticity. Mol. Cell. Proteomics 15, 368–381. doi: 10.1074/mcp.r115.051482
Dingledine, R., Borges, K., Bowie, D., and Traynelis, S. F. (1999). The glutamate receptor ion channels. Pharmacol. Rev. 51, 7–61.
Domon, B., and Aebersold, R. (2006). Mass spectrometry and protein analysis. Science 312, 212–217. doi: 10.1126/science.1124619
Domon, B., and Aebersold, R. (2010). Options and considerations when selecting a quantitative proteomics strategy. Nat. Biotechnol. 28, 710–721. doi: 10.1038/nbt.1661
Ebhardt, H. A., Root, A., Sander, C., and Aebersold, R. (2015). Applications of targeted proteomics in systems biology and translational medicine. Proteomics 15, 3193–3208. doi: 10.1002/pmic.201500004
Edwards, A. M., Isserlin, R., Bader, G. D., Frye, S. V., Willson, T. M., and Yu, F. H. (2011). Too many roads not taken. Nature 470, 163–165. doi: 10.1038/470163a
Eichenbaum, H. (2000). A cortical-hippocampal system for declarative memory. Nat. Rev. Neurosci. 1, 41–50. doi: 10.1038/35036213
Emes, R. D., Pocklington, A. J., Anderson, C. N., Bayes, A., Collins, M. O., Vickers, C. A., et al. (2008). Evolutionary expansion and anatomical specialization of synapse proteome complexity. Nat. Neurosci. 11, 799–806. doi: 10.1038/nn.2135
Filiou, M. D., Martins-de-Souza, D., Guest, P. C., Bahn, S., and Turck, C. W. (2012). To label or not to label: applications of quantitative proteomics in neuroscience research. Proteomics 12, 736–747. doi: 10.1002/pmic.201100350
Freeman, W. M., VanGuilder, H. D., Bennett, C., and Sonntag, W. E. (2009). Cognitive performance and age-related changes in the hippocampal proteome. Neuroscience 159, 183–195. doi: 10.1016/j.neuroscience.2008.12.004
Gallien, S., Duriez, E., and Domon, B. (2011). Selected reaction monitoring applied to proteomics. J. Mass Spectr. 46, 298–312. doi: 10.1002/jms.1895
Geiger, T., Wehner, A., Schaab, C., Cox, J., and Mann, M. (2012). Comparative proteomic analysis of eleven common cell lines reveals ubiquitous but varying expression of most proteins. Mol. Cell. Proteomics 11:M111.014050. doi: 10.1074/mcp.M111.014050
Geiger, T., Wisniewski, J. R., Cox, J., Zanivan, S., Kruger, M., Ishihama, Y., et al. (2011). Use of stable isotope labeling by amino acids in cell culture as a spike-in standard in quantitative proteomics. Nat. Protoc. 6, 147–157. doi: 10.1038/nprot.2010.192
Gong, S., Doughty, M., Harbaugh, C. R., Cummins, A., Hatten, M. E., Heintz, N., et al. (2007). Targeting Cre recombinase to specific neuron populations with bacterial artificial chromosome constructs. J. Neurosci. 27, 9817–9823. doi: 10.1523/JNEUROSCI.2707-07.2007
Götz, J., and Ittner, L. M. (2008). Animal models of Alzheimer's disease and frontotemporal dementia. Nat. Rev. Neurosci. 9, 532–544. doi: 10.1038/nrn2420
Govindarajan, A., Israely, I., Huang, S.-Y., and Tonegawa, S. (2011). The dendritic branch is the preferred integrative unit for protein synthesis-dependent LTP. Neuron 69, 132–146. doi: 10.1016/j.neuron.2010.12.008
Granger, A. J., Shi, Y., Lu, W., Cerpas, M., and Nicoll, R. A. (2013). LTP requires a reserve pool of glutamate receptors independent of subunit type. Nature 493, 495–500. doi: 10.1038/nature11775
Groszer, M., Keays, D. A., Deacon, R. M., de Bono, J. P., Prasad-Mulcare, S., Gaub, S., et al. (2008). Impaired synaptic plasticity and motor learning in mice with a point mutation implicated in human speech deficits. Curr. Biol. 18, 354–362. doi: 10.1016/j.cub.2008.01.060
Grover, L. M., Kim, E., Cooke, J. D., and Holmes, W. R. (2009). LTP in hippocampal area CA1 is induced by burst stimulation over a broad frequency range centered around delta. Learn. Mem. 16, 69–81. doi: 10.1101/lm.1179109
Gu, H., Marth, J. D., Orban, P. C., Mossmann, H., and Rajewsky, K. (1994). Deletion of a DNA polymerase beta gene segment in T cells using cell type-specific gene targeting. Science 265, 103–106. doi: 10.1126/science.8016642
Gygi, S. P., Rist, B., Gerber, S. A., Turecek, F., Gelb, M. H., and Aebersold, R. (1999). Quantitative analysis of complex protein mixtures using isotope-coded affinity tags. Nat. Biotechnol. 17, 994–999. doi: 10.1038/13690
Hawkins, R. D., Kandel, E. R., and Bailey, C. H. (2006). Molecular mechanisms of memory storage in Aplysia. Biol. Bull. 210, 174–191. doi: 10.2307/4134556
Hayashi, Y., Shi, S. H., Esteban, J. A., Piccini, A., Poncer, J. C., and Malinow, R. (2000). Driving AMPA receptors into synapses by LTP and CaMKII: requirement for GluR1 and PDZ domain interaction. Science 287, 2262–2267. doi: 10.1126/science.287.5461.2262
Hebert, A. S., Merrill, A. E., Bailey, D. J., Still, A. J., Westphall, M. S., Strieter, E. R., et al. (2013). Neutron-encoded mass signatures for multiplexed proteome quantification. Nat. Methods 10, 332–334. doi: 10.1038/nmeth.2378
Henninger, N., Feldmann, R. E. Jr., Fütterer, C. D., Schrempp, C., Maurer, M. H., Waschke, K. F., et al. (2007). Spatial learning induces predominant downregulation of cytosolic proteins in the rat hippocampus. Genes Brain Behav. 6, 128–140. doi: 10.1111/j.1601-183X.2006.00239.x
Hernández, L. G., Lu, B., da Cruz, G. C., Calábria, L. K., Martins, N. F., Togawa, R., et al. (2012). Worker honeybee brain proteome. J. Proteome Res. 11, 1485–1493. doi: 10.1021/pr2007818
Hölscher, C., Anwyl, R., and Rowan, M. J. (1997). Stimulation on the positive phase of hippocampal theta rhythm induces long-term potentiation that can Be depotentiated by stimulation on the negative phase in area CA1 in vivo. J. Neurosci. 17, 6470–6477.
Humphrey, S. J., Azimifar, S. B., and Mann, M. (2015). High-throughput phosphoproteomics reveals in vivo insulin signaling dynamics. Nat. Biotechnol. 33, 990–995. doi: 10.1038/nbt.3327
Jones, P., Côté, R. G., Cho, S. Y., Klie, S., Martens, L., Quinn, A. F., et al. (2008). PRIDE: new developments and newdatasets. Nucleic Acids Res. 36, D878–D883. doi: 10.1093/nar/gkm1021
Kähne, T., Kolodziej, A., Smalla, K.-H., Eisenschmidt, E., Haus, U.-U., Weismantel, R., et al. (2012). Synaptic proteome changes in mouse brain regions upon auditory discrimination learning. Proteomics 12, 2433–2444. doi: 10.1002/pmic.201100669
Kandel, E. R. (2001). The molecular biology of memory storage: a dialogue between genes and synapses. Science 294, 1030–1038. doi: 10.1126/science.1067020
Kandel, E. R. (2012). The molecular biology of memory: cAMP, PKA, CRE, CREB-1, CREB-2, and CPEB. Mol. Brain 5:14. doi: 10.1186/1756-6606-5-14
Kandel, E. R., Dudai, Y., and Mayford, M. R. (2014). The molecular and systems biology of memory. Cell 157, 163–186. doi: 10.1016/j.cell.2014.03.001
Kandel, E. R., Schwartz, J. H., Jessell, T. M., Siegelbaum, S. A., and Hudspeth, A. J. (2012). Principles of Neural Science, 5th Edn. New York, NY: McGraw–Hill.
Karp, N. A., Huber, W., Sadowski, P. G., Charles, P. D., Hester, S. V., and Lilley, K. S. (2010). Addressing accuracy and precision issues in iTRAQ quantitation. Mol. Cell. Proteomics 9, 1885–1897. doi: 10.1074/mcp.m900628-mcp200
Kettenbach, A. N., Rush, J., and Gerber, S. A. (2011). Absolute quantification of protein and post-translational modification abundance with stable isotope–labeled synthetic peptides. Nat. Protoc. 6, 175–186. doi: 10.1038/nprot.2010.196
Krüger, M., Moser, M., Ussar, S., Thievessen, I., Luber, C. A., Forner, F., et al. (2008). SILAC mouse for quantitative proteomics uncovers kindlin-3 as an essential factor for red blood cell function. Cell 134, 353–364. doi: 10.1016/j.cell.2008.05.033
Kuhn, E., Whiteaker, J. R., Mani, D. R., Jackson, A. M., Zhao, L., Pope, M. E., et al. (2011). Interlaboratory evaluation of automated, multiplexed peptide immunoaffinity enrichment coupled to multiple reaction monitoring mass spectrometry for quantifying proteins in plasma. Mol. Cell. Proteomics 11:M111.013854. doi: 10.1074/mcp.m111.013854
Kuhn, E., Wu, J., Karl, J., Liao, H., Zolg, W., and Guild, B. (2004). Quantification of C-reactive protein in the serum of patients with rheumatoid arthritis using multiple reaction monitoring mass spectrometry and13C-labeled peptide standards. Proteomics 4, 1175–1186. doi: 10.1002/pmic.200300670
Lange, V., Picotti, P., Domon, B., and Aebersold, R. (2008). Selected reaction monitoring for quantitative proteomics: a tutorial. Mol. Syst. Biol. 4, 222. doi: 10.1038/msb.2008.61
Lee, T.-R., Lee, H.-Y., Huang, S.-H., Chan, H.-T., Lyu, P.-C., and Chan, H.-L. (2013). Comparative proteomics analysis of normal and memory-deficient Drosophila melanogaster heads. Zool. Stud. 52:10. doi: 10.1186/1810-522x-52-10
Li, L., Boddul, S. V., Patil, S. S., Zheng, J.-F., An, G., Höger, H., et al. (2011). Proteins linked to extinction in contextual fear conditioning in the C57BL/6J mouse. Proteomics 11, 3706–3724. doi: 10.1002/pmic.201000444
Li, Y., Erzurumlu, R. S., Chen, C., Jhaveri, S., and Tonegawa, S. (1994). Whisker-related neuronal patterns fail to develop in the trigeminal brainstem nuclei of NMDAR1 knockout mice. Cell 76, 427–437. doi: 10.1016/0092-8674(94)90108-2
Lin, M.-H., Sugiyama, N., and Ishihama, Y. (2015). Systematic profiling of the bacterial phosphoproteome reveals bacterium-specific features of phosphorylation. Sci. Signal. 8, rs10. doi: 10.1126/scisignal.aaa3117
Lisman, J., Yasuda, R., and Raghavachari, S. (2012). Mechanisms of CaMKII action in long-term potentiation. Nat. Rev. Neurosci. 13, 169–182. doi: 10.1038/nrn3192
Low, T. Y., van Heesch, S., van den Toorn, H., Giansanti, P., Cristobal, A., Toonen, P., et al. (2013). Quantitative and qualitative proteome characteristics extracted from in-depth integrated genomics and proteomics analysis. Cell Rep. 5, 1469–1478. doi: 10.1016/j.celrep.2013.10.041
Lowenthal, M. S., Markey, S. P., and Dosemeci, A. (2015). Quantitative mass spectrometry measurements reveal stoichiometry of principal postsynaptic density proteins. J. Proteome Res. 14, 2528–2538. doi: 10.1021/acs.jproteome.5b00109
Lüscher, C., and Malenka, R. C. (2012). NMDA receptor-dependent long-term potentiation and long-term depression (LTP/LTD). Cold Spring Harb. Perspect. Biol. 4:a005710. doi: 10.1101/cshperspect.a005710
Lu, W., Isozaki, K., Roche, K. W., and Nicoll, R. A. (2010). Synaptic targeting of AMPA receptors is regulated by a CaMKII site in the first intracellular loop of GluA1. Proc. Natl. Acad. Sci. U.S.A. 107, 22266–22271. doi: 10.1073/pnas.1016289107
Lu, W., Man, H., Ju, W., Trimble, W. S., MacDonald, J. F., and Wang, Y. T. (2001). Activation of synaptic NMDA receptors induces membrane insertion of new AMPA receptors and LTP in cultured hippocampal neurons. Neuron 29, 243–254. doi: 10.1016/S0896-6273(01)00194-5
Lynch, M. A. (2004). Long-term potentiation and memory. Physiol. Rev. 84, 87–136. doi: 10.1152/physrev.00014.2003
Malenka, R. C., and Bear, M. F. (2004). LTP and LTD: an embarrassment of riches. Neuron 44, 5–21. doi: 10.1016/j.neuron.2004.09.012
Mann, M., and Kelleher, N. L. (2008). Precision proteomics: the case for high resolution and high mass accuracy. Proc. Natl. Acad. Sci. U.S.A. 105, 18132–18138. doi: 10.1073/pnas.0800788105
Marshall, A. G., Hendrickson, C. L., and Jackson, G. S. (1998). Fourier transform ion cyclotron resonance mass spectrometry: a primer. Mass Spectr. Rev. 17, 1–35. doi: 10.1002/(sici)1098-2787(1998)17:1<1::aid-mas1>3.0.co;2-k
Matsuura, K., Nakamura-Hirota, T., Takano, M., Otani, M., Kadoyama, K., and Matsuyama, S. (2013). Proteomic analysis of time-dependent changes in proteins expressed in mouse hippocampus during synaptic plasticity induced by GABAA receptor blockade. Neurosci. Lett. 555, 18–23. doi: 10.1016/j.neulet.2013.07.036
Mayford, M., Siegelbaum, S. A., and Kandel, E. R. (2012). Synapses and memory storage. Cold Spring Harb. Perspect. Biol. 4:a005751. doi: 10.1101/cshperspect.a005751
McHugh, T. J., Blum, K. I., Tsien, J. Z., Tonegawa, S., and Wilson, M. A. (1996). Impaired hippocampal representation of space in CA1-specific NMDAR1 knockout mice. Cell 87, 1339–1349. doi: 10.1016/S0092-8674(00)81828-0
Meissner, F., and Mann, M. (2014). Quantitative shotgun proteomics: considerations for a high-quality workflow in immunology. Nat. Immunol. 15, 112–117. doi: 10.1038/ni.2781
Michalski, A., Damoc, E., Lange, O., Denisov, E., Nolting, D., Müller, M., et al. (2011). Ultra high resolution linear ion trap orbitrap mass spectrometer (Orbitrap Elite) facilitates top down LC MS/MS and versatile peptide fragmentation modes. Mol. Cell. Proteomics 11:O111.013698. doi: 10.1074/mcp.o111.013698
Monje, F. J., Birner-Gruenberger, R., Darnhofer, B., Divisch, I., Pollak, D. D., and Lubec, G. (2012). Proteomics reveals selective regulation of proteins in response to memory-related serotonin stimulation in Aplysia californica ganglia. Proteomics 12, 490–499. doi: 10.1002/pmic.201100418
Monopoli, M. P., Raghnaill, M. N., Loscher, J. S., O'Sullivan, N. C., Pangalos, M. N., Ring, R. H., et al. (2011). Temporal proteomic profile of memory consolidation in the rat hippocampal dentate gyrus. Proteomics 11, 4189–4201. doi: 10.1002/pmic.201100072
Moser, E., Moser, M. B., and Andersen, P. (1993). Spatial learning impairment parallels the magnitude of dorsal hippocampal lesions, but is hardly present following ventral lesions. J. Neurosci. 13, 3916–3925.
Moser, M.-B., Rowland, D. C., and Moser, E. I. (2015). Place Cells, Grid Cells, and Memory. Cold Spring Harb. Perspect. Biol. 7:a021808. doi: 10.1101/cshperspect.a021808
Munton, R. P., Tweedie-Cullen, R., Livingstone-Zatchej, M., Weinandy, F., Waidelich, M., Longo, D., et al. (2007). Qualitative and quantitative analyses of protein phosphorylation in naive and stimulated mouse synaptosomal preparations. Mol. Cell. Proteomics 6, 283–293. doi: 10.1074/mcp.M600046-MCP200
Musunuri, S., Wetterhall, M., Ingelsson, M., Lannfelt, L., Artemenko, K., Bergquist, J., et al. (2014). Quantification of the brain proteome in Alzheimer's disease using multiplexed mass spectrometry. J. Proteome Res. 13, 2056–2068. doi: 10.1021/pr401202d
Nabavi, S., Fox, R., Proulx, C. D., Lin, J. Y., Tsien, R. Y., and Malinow, R. (2014). Engineering a memory with LTD and LTP. Nature 511, 348–352. doi: 10.1038/nature13294
Nascimento, J. M., and Martins-de-Souza, D. (2015). The proteome of schizophrenia. npj Schizophrenia 1, 14003. doi: 10.1038/npjschz.2014.3
Neves, G., Cooke, S. F., and Bliss, T. V. P. (2008). Synaptic plasticity, memory and the hippocampus: a neural network approach to causality. Nat. Rev. Neurosci. 9, 65–75. doi: 10.1038/nrn2303
Nguyen, P. V., Abel, T., and Kandel, E. R. (1994). Requirement of a critical period of transcription for induction of a late phase of LTP. Science 265, 1104–1107. doi: 10.1126/science.8066450
Old, W. M., Meyer-Arendt, K., Aveline-Wolf, L., Pierce, K. G., Mendoza, A., Sevinsky, J. R., et al. (2005). Comparison of label-free methods for quantifying human proteins by shotgun proteomics. Mol. Cell. Proteomics 4, 1487–1502. doi: 10.1074/mcp.M500084-MCP200
Ong, S. E. (2002). Stable isotope labeling by amino acids in cell culture, SILAC, as a simple and accurate approach to expression proteomics. Mol. Cell. Proteomics 1, 376–386. doi: 10.1074/mcp.m200025-mcp200
Ong, S.-E., and Mann, M. (2005). Mass spectrometry–based proteomics turns quantitative. Nat. Chem. Biol. 1, 252–262. doi: 10.1038/nchembio736
Patterson, S. L., Pittenger, C., Morozov, A., Martin, K. C., Scanlin, H., Drake, C., et al. (2001). Some forms of cAMP-mediated long-lasting potentiation are associated with release of BDNF and nuclear translocation of phospho-MAP kinase. Neuron 32, 123–140. doi: 10.1016/S0896-6273(01)00443-3
Paulsen, J. S. (2011). Cognitive impairment in huntington disease: diagnosis and treatment. Curr. Neurol. Neurosci. Rep. 11, 474–483. doi: 10.1007/s11910-011-0215-x
Peshkin, L., Wühr, M., Pearl, E., Haas, W., Freeman, R. M. Jr., Gerhart, J. C., et al. (2015). On the relationship of protein and mRNA dynamics in vertebrate embryonic development. Dev. Cell 35, 383–394. doi: 10.1016/j.devcel.2015.10.010
Piccoli, G., Verpelli, C., Tonna, N., Romorini, S., Alessio, M., Nairn, A. C., et al. (2007). Proteomic analysis of activity-dependent synaptic plasticity in hippocampal neurons. J. Proteome Res. 6, 3203–3215. doi: 10.1021/pr0701308
Picotti, P., and Aebersold, R. (2012). Selected reaction monitoring–based proteomics: workflows, potential, pitfalls and future directions. Nat. Methods 9, 555–566. doi: 10.1038/nmeth.2015
Picotti, P., Bodenmiller, B., and Aebersold, R. (2013). Proteomics meets the scientific method. Nat. Methods 10, 24–27. doi: 10.1038/nmeth.2291
Ponomarev, I., Rau, V., Eger, E. I., Harris, R. A., and Fanselow, M. S. (2010). Amygdala transcriptome and cellular mechanisms underlying stress-enhanced fear learning in a rat model of posttraumatic stress disorder. Neuropsychopharmacology 35, 1402–1411. doi: 10.1038/npp.2010.10
Poser, S., and Storm, D. R. (2001). Role of Ca2+-stimulated adenylyl cyclases in LTP and memory formation. Int. J. Dev. Neurosci. 19, 387–394. doi: 10.1016/S0736-5748(00)00094-0
Rao-Ruiz, P., Carney, K. E., Pandya, N., van der Loo, R. J., Verheijen, M. H., van Nierop, P., et al. (2015). Time-dependent changes in the mouse hippocampal synaptic membrane proteome after contextual fear conditioning. Hippocampus 25, 1250–1261. doi: 10.1002/hipo.22432
Rao-Ruiz, P., Rotaru, D. C., van der Loo, R. J., Mansvelder, H. D., Stiedl, O., Smit, A. B., et al. (2011). Retrieval-specific endocytosis of GluA2-AMPARs underlies adaptive reconsolidation of contextual fear. Nat. Neurosci. 14, 1302–1308. doi: 10.1038/nn.2907
Rauniyar, N., McClatchy, D. B., and Yates, J. R. (2013). Stable isotope labeling of mammals (SILAM) for in vivo quantitative proteomic analysis. Methods 61, 260–268. doi: 10.1016/j.ymeth.2013.03.008
Rempel-Clower, N. L., Zola, S. M., Squire, L. R., and Amaral, D. G. (1996). Three cases of enduring memory impairment after bilateral damage limited to the hippocampal formation. J. Neurosci. 16, 5233–5255. doi: 10.1016/S0960-9822(02)70778-1
Roberson, E. D., English, J. D., Adams, J. P., Selcher, J. C., Kondratick, C., and Sweatt, J. D. (1999). The mitogen-activated protein kinase cascade couples PKA and PKC to cAMP response element binding protein phosphorylation in area CA1 of hippocampus. J. Neurosci. 19, 4337–4348.
Rongo, C., and Kaplan, J. M. (1999). CaMKII regulates the density of central glutamatergic synapses in vivo. Nature 402, 195–199. doi: 10.1038/46065
Rosenegger, D., Wright, C., and Lukowiak, K. (2010). A quantitative proteomic analysis of long-term memory. Mol. Brain 3:9. doi: 10.1186/1756-6606-3-9
Ross, C. A., and Poirier, M. A. (2004). Protein aggregation and neurodegenerative disease. Nat. Med. 10(Suppl.), S10–S17. doi: 10.1038/nm1066
Ross, P. L. (2004). Multiplexed protein quantitation in saccharomyces cerevisiae using amine-reactive isobaric tagging reagents. Mol. Cell. Proteomics 3, 1154–1169. doi: 10.1074/mcp.m400129-mcp200
Ryan, T. J., and Grant, S. G. (2009). The origin and evolution of synapses. Nat. Rev. Neurosci. 10, 701–712. doi: 10.1038/nrn2717
Ryan, T. J., Roy, D. S., Pignatelli, M., Arons, A., and Tonegawa, S. (2015). Engram cells retain memory under retrograde amnesia. Science 348, 1007–1013. doi: 10.1126/science.aaa5542
Sadygov, R. G., Cociorva, D., and Yates, J. R. (2004). Large-scale database searching using tandem mass spectra: looking up the answer in the back of the book. Nat. Methods 1, 195–202. doi: 10.1038/nmeth725
Schiller, J., Schiller, Y., and Clapham, D. E. (1998). NMDA receptors amplify calcium influx into dendritic spines during associative pre- and postsynaptic activation. Nat. Neurosci. 1, 114–118. doi: 10.1038/363
Sharma, K., Schmitt, S., Bergner, C. G., Tyanova, S., Kannaiyan, N., Manrique-Hoyos, N., et al. (2015). Cell type– and brain region–resolved mouse brain proteome. Nat. Neurosci. 18, 1819–1831. doi: 10.1038/nn.4160
Smith, L. M., Kelleher, N. L., and Consortium for Top Down Proteomics. (2013). Proteoform: a single term describing protein complexity. Nat. Methods 10, 186–187. doi: 10.1038/nmeth.2369
Strange, B. A., Witter, M. P., Lein, E. S., and Moser, E. I. (2014). Functional organization of the hippocampal longitudinal axis. Nat. Rev. Neurosci. 15, 655–669. doi: 10.1038/nrn3785
Suh, J., Rivest, A. J., Nakashiba, T., Tominaga, T., and Tonegawa, S. (2011). Entorhinal cortex layer III input to the hippocampus is crucial for temporal association memory. Science 334, 141–1420. doi: 10.1126/science.1210125
Taniguchi, H., He, M., Wu, P., Kim, S., Paik, R., Sugino, K., et al. (2011). A resource of Cre driver lines for genetic targeting of GABAergic neurons in cerebral cortex. Neuron 71, 995–1013. doi: 10.1016/j.neuron.2011.07.026
Thakur, S. S., Geiger, T., Chatterjee, B., Bandilla, P., Fröhlich, F., Cox, J., et al. (2011). Deep and highly sensitive proteome coverage by LC-MS/MS without prefractionation. Mol. Cell. Proteomics 10:M110.003699. doi: 10.1074/mcp.m110.003699
Thompson, A., Schäfer, J., Kuhn, K., Kienle, S., Schwarz, J., Schmidt, G., et al. (2003). Tandem Mass Tags: a novel quantification strategy for comparative analysis of complex protein mixtures by MS/MS. Anal. Chem. 75, 1895–1904. doi: 10.1021/ac0262560
Ting, L., Rad, R., Gygi, S. P., and Haas, W. (2011). MS3 eliminates ratio distortion in isobaric multiplexed quantitative proteomics. Nat. Methods 8, 937–940. doi: 10.1038/nmeth.1714
Tonegawa, S., Liu, X., Ramirez, S., and Redondo, R. (2015). Memory engram cells have come of age. Neuron 87, 918–931. doi: 10.1016/j.neuron.2015.08.002
Trinidad, J. C., Thalhammer, A., Burlingame, A. L., and Schoepfer, R. (2013). Activity-dependent protein dynamics define interconnected cores of co-regulated postsynaptic proteins. Mol. Cell. Proteomics 12, 29–41. doi: 10.1074/mcp.M112.019976
Tronson, N. C., and Taylor, J. R. (2007). Molecular mechanisms of memory reconsolidation. Nat. Rev. Neurosci. 8, 262–275. doi: 10.1038/nrn2090
Tsai, L. Y., Tseng, S. H., and Yeh, S. R. (2005). Long-lasting potentiation of excitatory synaptic signaling to the crayfish lateral giant neuron. J. Comp. Physiol. A Neuroethol. Sens. Neural Behav. Physiol. 191, 347–354. doi: 10.1007/s00359-004-0589-1
Tsien, J. Z. (2016). Cre-lox neurogenetics: 20 years of versatile applications in brain research and counting.… Front. Genet. 7:19. doi: 10.3389/fgene.2016.00019
Tsien, J. Z., Chen, D. F., Gerber, D., Tom, C., Mercer, E. H., Anderson, D. J., et al. (1996a). Subregion- and cell type-restricted gene knockout in mouse brain. Cell 87, 1317–1326. doi: 10.1016/S0092-8674(00)81826-7
Tsien, J. Z., Huerta, P. T., and Tonegawa, S. (1996b). The essential role of hippocampal CA1 NMDA receptor-dependent synaptic plasticity in spatial memory. Cell 87, 1327–1338. doi: 10.1016/S0092-8674(00)81827-9
Tyanova, S., Albrechtsen, R., Kronqvist, P., Cox, J., Mann, M., and Geiger, T. (2016). Proteomic maps of breast cancer subtypes. Nat. Commun. 7:10259. doi: 10.1038/ncomms10259
Végh, M. J., Rausell, A., Loos, M., Heldring, C. M., Jurkowski, W., van Nierop, P., et al. (2014). Hippocampal extracellular matrix levels and stochasticity in synaptic protein expression increase with age and are associated with age-dependent cognitive decline. Mol. Cell. Proteomics 13, 2975–2985. doi: 10.1074/mcp.M113.032086
Viola, H., Furman, M., Izquierdo, L. A., Alonso, M., Barros, D. M., de Souza, M. M., et al. (2000). Phosphorylated cAMP response element-binding protein as a molecular marker of memory processing in rat hippocampus: effect of novelty. J. Neurosci. 20, RC112.
Voss, J. L., and Paller, K. A. (2008). Brain substrates of implicit and explicit memory: the importance of concurrently acquired neural signals of both memory types. Neuropsychologia 46, 3021–3029. doi: 10.1016/j.neuropsychologia.2008.07.010
Wang, H., Shimizu, E., Tang, Y.-P., Cho, M., Kyin, M., Zuo, W., et al. (2003). Inducible protein knockout reveals temporal requirement of CaMKII reactivation for memory consolidation in the brain. Proc. Natl. Acad. Sci. U.S.A. 100, 4287–4292. doi: 10.1073/pnas.0636870100
Weekes, M. P., Tomasec, P., Huttlin, E. L., Fielding, C. A., Nusinow, D., Stanton, R. J., et al. (2014). Quantitative temporal viromics: an approach to investigate host-pathogen interaction. Cell 157, 1460–1472. doi: 10.1016/j.cell.2014.04.028
Wenger, C. D., Lee, M. V., Hebert, A. S., McAlister, G. C., Phanstiel, D. H., Westphall, M. S., et al. (2011). Gas-phase purification enables accurate, multiplexed proteome quantification with isobaric tagging. Nat. Methods 8, 933–935. doi: 10.1038/nmeth.1716
Whitlock, J. R., Heynen, A. J., Shuler, M. G., and Bear, M. F. (2006). Learning induces long-term potentiation in the hippocampus. Science 313, 1093–1097. doi: 10.1126/science.1128134
Wong, S. T., Athos, J., Figueroa, X. A., Pineda, V. V., Schaefer, M. L., Chavkin, C. C., et al. (1999). Calcium-stimulated adenylyl cyclase activity is critical for hippocampus-dependent long-term memory and late phase LTP. Neuron 23, 787–798. doi: 10.1016/S0896-6273(01)80036-2
Wühr, M., Güttler, T., Peshkin, L., McAlister, G. C., Sonnett, M., Ishihara, K., et al. (2015). The nuclear proteome of a vertebrate. Curr. Biol. 25, 2663–2671. doi: 10.1016/j.cub.2015.08.047
Wu, R., Haas, W., Dephoure, N., Huttlin, E. L., Zhai, B., Sowa, M. E., et al. (2011). A large-scale method to measure absolute protein phosphorylation stoichiometries. Nat. Methods 8, 677–683. doi: 10.1038/nmeth.1636
Yasuda, H., Barth, A. L., Stellwagen, D., and Malenka, R. C. (2003). A developmental switch in the signaling cascades for LTP induction. Nat. Neurosci. 6, 15–16. doi: 10.1038/nn985
Yi, E. C., Li, X.-J., Cooke, K., Lee, H., Raught, B., Page, A., et al. (2005). Increased quantitative proteome coverage with13C/12C-based, acid-cleavable isotope-coded affinity tag reagent and modified data acquisition scheme. Proteomics 5, 380–387. doi: 10.1002/pmic.200400970
Yost, R. A., and Enke, C. G. (1979). Triple quadrupole mass spectrometry for direct mixture analysis and structure elucidation. Anal. Chem. 51, 1251–1264. doi: 10.1021/ac50048a002
Zhang, Y., Fonslow, B. R., Shan, B., Baek, M.-C., and Yates, J. R. III. (2013). Protein analysis by shotgun/bottom-up proteomics. Chem. Rev. 113, 2343–2394. doi: 10.1021/cr3003533
Zhang, Y., Shan, B., Boyle, M., Liu, J., Liao, L., Xu, T., et al. (2014). Brain proteome changes induced by olfactory learning in Drosophila. J. Proteome Res. 13, 3763–3770. doi: 10.1021/pr500325q
Zheng, J.-F., Patil, S. S., Chen, W.-Q., An, W., He, J.-Q., Höger, H., et al. (2009). Hippocampal protein levels related to spatial memory are different in the Barnes maze and in the multipleT-maze. J. Proteome Res. 8, 4479–4486. doi: 10.1021/pr9002596
Zheng, N., Jeyifous, O., Munro, C., Montgomery, J. M., and Green, W. N. (2015). Synaptic activity regulates AMPA receptor trafficking through different recycling pathways. Elife 4:e06878. doi: 10.7554/eLife.06878
Keywords: memory, hippocampus, synapse, long-term potentiation (LTP), mass spectrometry, selected reaction monitoring (SRM), quantitative proteomics
Citation: Pontes AH and de Sousa MV (2016) Mass Spectrometry-Based Approaches to Understand the Molecular Basis of Memory. Front. Chem. 4:40. doi: 10.3389/fchem.2016.00040
Received: 09 August 2016; Accepted: 27 September 2016;
Published: 14 October 2016.
Edited by:
Aline Maria Araujo Martins, Federal University of Ceará, BrazilReviewed by:
Christian W. Gruber, Medical University of Vienna, AustriaMare Cudic, Florida Atlantic University, USA
Copyright © 2016 Pontes and de Sousa. This is an open-access article distributed under the terms of the Creative Commons Attribution License (CC BY). The use, distribution or reproduction in other forums is permitted, provided the original author(s) or licensor are credited and that the original publication in this journal is cited, in accordance with accepted academic practice. No use, distribution or reproduction is permitted which does not comply with these terms.
*Correspondence: Marcelo V. de Sousa, bXZzb3VzYUB1bmIuYnI=