- 1Laboratory of Genomics and BioEnergy (LGE), Department of Genetics, Evolution, Microbiology, and Immunology, Institute of Biology, State University of Campinas, Campinas, SP, Brazil
- 2Center for Computing in Engineering and Sciences, State University of Campinas, Campinas, SP, Brazil
- 3Institute of Computing, State University of Campinas, Campinas, SP, Brazil
Agave plants are well-known for their drought resilience and commercial applications. Among them, Agave sisalana (sisal) is the species most used to produce hard fibers, and it is of great importance for semiarid regions. Agaves also show potential as bioenergy feedstocks, as they can accumulate large amounts of biomass and fermentable sugar. This study aimed to reconstruct the A. sisalana interactome, and identify key genes and modules involved in multiple plant tissues (root, stem, and leaf) through RNA-Seq analysis. We integrated A. sisalana transcriptome sequences and gene expression generated from stem, leaf, and root tissues to build global and conditional co-expression networks across the entire transcriptome. By combining the co-expression network, module classification, and function enrichment tools, we identified 20 functional modules related to at least one A. sisalana tissue, covering functions such as photosynthesis, leaf formation, auxin-activated signaling pathway, floral organ abscission, response to farnesol, brassinosteroid mediated signaling pathway, and light-harvesting. The final interactome of A. sisalana contains 2,582 nodes and 15,083 edges. In the reconstructed interactome, we identified submodules related to plant processes to validate the reconstruction. In addition, we identified 6 hub genes that were searched for in the co-expression modules. The intersection of hub genes identified by both the protein-protein interaction networks (PPI networks) and co-expression analyses using gene significance and module membership revealed six potential candidate genes for key genes. In conclusion, we identified six potential key genes for specific studies in Agave transcriptome atlas studies, biological processes related to plant survival in unfavorable environments and provide strategies for breeding programs.
1 Introduction
Plants of the genus Agave are known for their drought resistance mechanisms and commercial applications. Agaves are native to semiarid and arid regions of North and Central America and possess several morphological, anatomical, and physiological drought resistance mechanisms (Eguiarte et al., 2021). One of the most important is CAM (crassulacean acid metabolism), the most water-use efficient type of photosynthesis (Borland et al., 2014; Yin et al., 2018). Also, several Agave species have established commercial uses, for instance, the production of tequila (Agave tequilana), mezcal (Agave angustifolia and others), and sisal fibers (mainly Agave sisalana). Sisal is the main source of natural hard fibers, and, due to its drought resistance, it has a great social-economical impact on semiarid regions (Broeren et al., 2017; Monja-Mio et al., 2019). In addition, A. sisalana has interesting characteristics to be used as a bioenergy feedstock in semi-arid regions (Davis et al., 2011), since only 4% of A. sisalana leaves are used to produce commercial fibers (Suinaga et al., 2007). The remaining waste could be used to produce biogas or second-generation ethanol (Mielenz et al., 2015). Brazil is the greatest sisal fiber producer in the world and has the capacity of increasing the area in which this crop is produced to keep up with the increasing demand for biofuels (International Energy Agency, 2021).
Transcriptomic atlases (Sarwar et al., 2019; Raya et al., 2021) and phylogeny and evolution studies of A. sisalana (Jiménez-Barron et al., 2020) are available and provide valuable information about its genetics. However, there are no analyses or available data on protein-protein interactions (PPI) in any Agave species. PPI networks represent physical interactions between proteins, which can lead to useful insights into cell physiology and help identify targets for genetic engineering (Ferreira et al., 2016; Van den Broeck et al., 2017; Khan et al., 2020; Yu et al., 2020; de Silva et al., 2022). PPI associations, for example, may represent a functional cell action and have already been used in several areas of the biological sciences (Braun et al., 2013; Yeger-Lotem and Sharan, 2015; Ding and Kihara, 2019; Yang et al., 2019). High-throughput technologies, such as mass spectrometers and genomic sequencers, generated a large amount of data allowing for an increase in the number of known protein interactions of various organisms, especially model organisms, and are available in several databases, such as STRING (Szklarczyk et al., 2011).
However, the interactions between proteins available to non-model organisms are very limited, so the complete mapping depends on bioinformatics strategies that aim to assign relationships through orthologs (Uhrig, 2006). These strategies for assembling PPI networks assume that proteins are conserved across different species, and therefore, the interactions between two proteins may also exist in those species (Wang and Jin, 2017). In addition, multi-omics analyses integrated with co-expression analysis in the understanding of complex phenotypes of non-model plants bring relevant information about the biological system, genes pattern, unexplored genes, and their relation with the environment (Abraham et al., 2016; Sarwar et al., 2019; Yu et al., 2020; Feng et al., 2021; Panahi and Hejazi, 2021; Li et al., 2022).
Here, we predict A. sisalana interactome using public transcriptome sequences generated from leaf, stem, and root tissues. Moreover, we developed a new strategy that integrates network analysis with weighted gene coexpression network analysis to identify correlations between genes across tissues based on coexpression relationships and focus on pointing out key genes and modules, which will aid current research and provide a framework for future Agave interactomics research.
2 Material and methods
2.1 RNA-Seq samples
In this study, we investigated the sisal using transcriptomic datasets previously generated by Raya et al. (2021). Our samples were extracted from sections of the leaves, stems, and roots from three different seven-year-old adult plants of A. sisalana collected at the EMBRAPA collection in Monteiro, in the state of Paraíba, Brazil (07°53′ S; 37°07′ W, elevation: 619 m). This region faced an irregular rainfall regime before sampling. For each cultivar, three biological replicates growing side-by-side were sampled. To maintain leaf maturity equivalency within the cultivars, we sampled the fifth leaf counted from the central spike of each plant. Although the plants were maintained in the field and exposed to long drought periods typical of the Caatinga biome, all the collected individuals were healthy, with the leaves showing homogenous green coloration, with no visible symptoms of diseases (necrosis, chlorosis, spots, etc.).
2.2 Transcriptomic analysis
The assembled transcripts and the raw RNA-Seq reads of three tissues (biological triplicates of leaf, stem, and root) of A. sisalana were downloaded from NCBI SRA (accession number PRJNA746623) (Raya et al., 2021). Poor quality reads and adapters were removed with the Trimmomatic v.0.39 (Bolger et al., 2014) software. Quantification of the assembled transcripts was performed by the software kallisto v.1.0.4 (Bray et al., 2016) and normalized by TMM using the edgeR package v3.38.2 (Robinson et al., 2010). The ORFs of the assembled transcripts were identified by the Transdecoder software with the parameter -m 300 (ORFs longer than 300 nucleotides). Transcripts that did not have ORFs and whose expressions were less than 1 TPM in all conditions were removed. After this removal step, a new round of quantification was performed. A. sisalana proteins were obtained from the translation of ORFs.
2.3 Grouping of orthologous families
Five organisms was selected to construct the orthogroups, together with A. sisalana proteins, based on their wide availability of PPI data in the STRING v.11 database: Arabidopsis thaliana, Musa acuminata, Oryza sativa, Sorghum bicolor, and Zea mays. Protein sequences from each organism were retrieved from the NCBI website. OrthoFinder v.2.3.3 software was used (Emms and Kelly, 2019) to construct orthogroups (gene families) from these organisms. From the gene families obtained, we selected those that are considered one-to-one or one-to-many. One-to-one gene families are those that have one copy of a gene in A. sisalana and one copy in every other organism. The one-to-many gene families mean that the gene of interest has one copy in A. sisalana and more than one ortholog in the other species (Zahn-Zabal et al., 2020). The reconstruction of the phylogenetic tree between the species was also generated by OrthoFinder from the default parameters.
2.4 Construction of A. sisalana interactome
For each gene family containing at least oneA. sisalana protein and proteins from other organisms, we identified the PPIs present in each orthologous organism in the STRING database, considering a cutoff score of 0.70 for each protein interaction. For example, if an A. sisalana gene A has as orthologs the gene B in S. bicolor and the gene C in M. acuminata, we assigned the interactions in the genes B and C to the gene A. Thus, the interactions are mapped according to their orthologs in A. sisalana. In the end, only interactions that have complete pairs with orthologs in A. sisalana were selected. To visualize the PPI network in A. sisalana, the predicted PPI data were loaded into Cytoscape v3.2.1 (Shannon et al., 2003).
2.5 Network analysis
After constructing the A. sisalana interactome and loading it into Cytoscape, the network metrics were analyzed with Network Analyzer v4.4.8. The top hub genes were selected from the intersection of the top 20 hub genes derived from three metrics: betweenness centrality, closeness centrality, and node connect degree, using Cytohubba v0.1 (Chin et al., 2014). The submodules were identified using MCODE v2.0.2 in Cytoscape with a cutoff of 5 in MCODE score.
2.6 Gene ontology (GO) analysis of PPI data
A. sisalana proteins were annotated using PANNZER2 software (Törönen et al., 2018). A table <gene> <go_id> was generated from the results, considering the Gene Ontology class “Biological Process (BP)" in the output of PANNZER2. The enrichment of biological processes was performed using the topGO package v2.48.0 (Alexa et al., 2006) using R 4.1.0.
2.7 KEGG orthology annotation and enrichment
The Kyoto Encyclopedia of Gene and Genomes (KEGG) orthology (KO) annotations were assigned using KofamKOALA 1.0.0 (Aramaki et al., 2020), which uses hmmsearch 3.1b2 against curated hidden Markov model (HMM) KO profiles. The enriched KEGG pathways and their modules have been identified through the enrichKEGG and enrichMKEGG functions of the ClusterProfiler package v4.4.4 (Wu et al., 2021) using R 4.1.0.
2.8 Tissue-specific analysis
Tau metric was used to measure expression specificity among all genes present in the A. sisalana interactome with the Tspex package (Camargo et al., 2020). Tau showed a robust behavior according to data normalization (Kryuchkova-Mostacci and Robinson-Rechavi, 2017), in our case, through the TMM normalization in gene expression data. We considered genes with Tau values greater than 0.90 as tissue specific.
2.9 Weighted correlation network analysis (WGCNA)
Gene expression matrix (normalized gene expression of biological replicates of leaf, stem, and root tissues) was used to construct an unsigned weighted gene co-expression network using the WGCNA R package v1.71 (Langfelder and Horvath, 2008). Hierarchical clustering of samples was conducted to remove outliers with a cut-off value of 80 to produce two stable clusters. Then, the soft threshold power β was determined to ensure a scale-free network. The power function was used to turn the Pearson correlation matrix into an adjacency matrix, which was then transformed into a topological overlap matrix (TOM). Using a dynamic cutting algorithm, hierarchical clustering was performed to cluster similar genes into the same module. Subsequently, we clustered the eigengenes according to the relationship and merged them into modules with an association >0.80. The association of each module with gene expressions in the tissues was evaluated based on Pearson correlation (|cor| > 0.5 and p-value <= 0.05). For each gene, module membership (MM) was characterized according to the association between module eigengene (ME) and its expression level. The association between gene expression and tissues was represented by gene significance (GS). Thus, for each module, GS and MM for each gene were computed and considered significant if |GS| > 0.2 and MM > 0.8.
3 Results and discussion
A detailed outline of our study are summarized in Figure 1. Firstly, we conducted an analysis of orthologous genes between several plant species and the transcriptome assembly of A. sisalana. From the identification of ortholog families, we mapped the interactions of these pairs of orthologs in the STRING database. And then, we predicted the A. sisalana interactome. In addition, we identified the interactome submodules and performed its GO enrichment to search for modules with specific functions in A. sisalana from known databases. In addition, we performed the analysis of hub genes, which play an important role in the interactome.
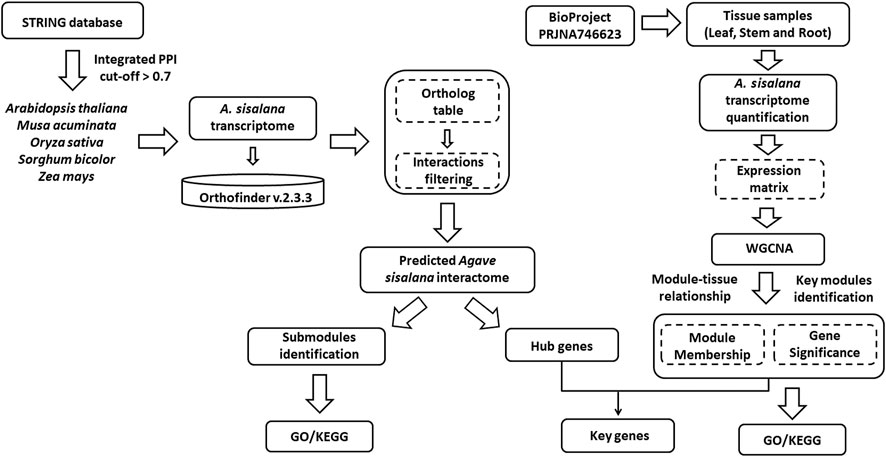
FIGURE 1. Flowchart illustrating the study design. Agave sisalana interactome was predicted from five orthologs organisms based on STRING database interactions in order to identify hub genes. Also, a WGCNA analysis was performed to identify key modules based on module-tissue relationships from three organs of A. sisalana (leaf, stem, and root). Both analyses were integrated to find key genes. The dashed lines indicate software outputs or in-house scripting processes.
Likewise, we performed weighted gene co-expression network analysis from stem, leaf, and root tissues studied in the A. sisalana transcriptome. From this analysis, we can identify the key modules, which are co-expression modules correlated with each tissue, and make hypotheses about the functionalities observed in the plant.
Finally, we cross-referenced the lists of hub genes with their presence in co-expression modules, evaluating module membership (MM) and gene significance (GS). If these hub genes pass through the co-expression module evaluation cut-off, they were considered key genes.
All steps and results present in the flowchart are described in the next sections.
3.1 A. sisalana orthogroups
Initially, a total of 23,794 Agave sisalana proteins (extracted from the transcriptomic atlas) were parsed and grouped into orthologous families using five plant species (A. thaliana, M. acuminata, O. sativa, S. bicolor, and Z. mays), selected by the wide availability of interactome data. Table 1 summarizes the orthogroups containing at least one protein of plant species. From the orthogroups obtained, we selected those that are considered one-to-one or one-to-many. A total of 4,501 A. sisalana proteins present in those orthogroups were eligible for the search for interactions in the STRING database.
3.2 A. sisalana interactome
A. sisalana interactome was reconstructed through the mapping of the ortholog groups and protein interactions extracted from the STRING database. The number of interactions obtained from the STRING database was 772,055 in A. thaliana, 1,384,645 in M. acuminata, 588, 907 in O. sativa, 618,973 in S. bicolor, and 1,368,899 in Zea may. Here, it was assumed that interactions of proteins in one organism are expected to be conserved in other related organisms (Fraser, 2005; Uhrig, 2006).
After predicting PPIs in A. sisalana, the PPI network was visualized using Cytoscape v3.8.1. The final interactome contains 2,582 nodes and 15,083 edges (Supplementary File S1). The topological metrics of the PPI network were calculated using Network Analyzer (Table 2). Analysis of the interaction network showed the short path length distribution, the node degree distribution, the neighborhood connectivity distribution, and the clustering coefficient distribution (Figure 2). The declining neighborhood connection trend reveals a weak clustering coefficient among neighbor nodes with lesser connectedness. On the other hand, the shortest path length distribution showed that the edges have a frequency dominated by a short path length (3–5), as path length means edge. The node degree distribution shows that the A. sisalana interactome has many nodes with a low degree, suggesting this possible short path length. In the clustering coefficient distribution, we note the presence of nodes with a low degree (clustering coefficient equal to zero), but also a group of highly connected genes, with a clustering coefficient equal to 1.
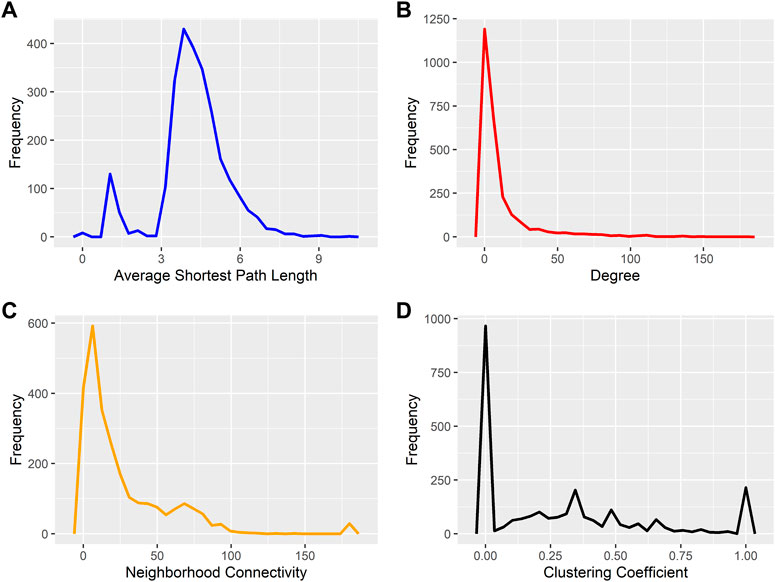
FIGURE 2. Agave sisalana interactome metrics. (A) short path length distribution (B) the node degree distribution, (C) the neighborhood connectivity distribution, and (D) the clustering coefficient distribution.
At the protein level, important genes are normally strongly interconnected hubs. The top 20 hubs were identified in the A. sisalana interactome (Table “hub genes” in Supplementary File S2) and most of them are functionally related to transcription, phosphorylation, response to stimulus, and nitrate assimilation. The D-fructose-1,6-bisphosphate 1-phosphohydrolase gene (AS_TRINITY_DN57407_c0_g1_i1) was identified as a hub and has an important regulatory role involved in photosynthetic CO2 assimilation (Chehebar and Wolosiuk, 1980). Another hub is an ATPase, F1 complex, OSCP/delta subunit (AS_TRINITY_DN7474_c0_g1_i1), which has a role in inorganic ion transmembrane transport and participates in many metabolic processes. Protein-serine/threonine phosphatase (AS_TRINITY_DN57726_c0_g1_i1) was identified as a hub in the A. sisalana interactome. For Agave, Protein-serine/threonine phosphatases are related to diel expression patterns and exhibit inverted temporal shifts in abundance when compared to the C3 plant A. thaliana (Abraham et al., 2016).
3.3 GO and KEGG enrichment analysis
We performed GO enrichment analysis of all genes present in the interactome. We identified biological processes related to protein import, protein modification, and methylation, such as methylation (GO:0032259; p-value 5.0e-07), protein peptidyl-prolyl isomerization (GO:0000413; p-value 5.0e-10) and protein import into chloroplast stroma (GO:0045037; p-value 1.7e-04). Basal processes related to the photosystem and chloroplast were also identified, such as photosynthesis (GO:0015979; p-value 1.4e-21), light harvesting (GO:0009765; p-value 5.4e-06), chloroplast fission (GO:0010020; p-value 4.5e-04), chlorophyll biosynthetic process (GO:0015995; p-value 1.1e-07), photosystem I assembly (GO:0048564; p-value 9.8e-04), photosystem II repair (GO:0010206; p-value 9.8e-04) and photosynthetic electron transport in photosystem I (GO:0009773; p-value 5.3e-05).
Regarding KEGG enrichment, some basic pathways detected in plants were enriched, such as Plant hormone signal transduction (ko04075), MAPK signaling pathway - plant (ko04016), Photosynthesis (ko00195), Circadian rhythm - plant (ko04712), Ribosome (ko03010), Biosynthesis of cofactors (ko01240), Plant-pathogen interaction (ko04626), Carbon fixation in photosynthetic organisms (ko00710), Porphyrin metabolism (ko00860), Ubiquinone and another terpenoid-quinone biosynthesis (ko00130), Protein export (ko03060), Biosynthesis of amino acids (ko01230), Protein processing in endoplasmic reticulum (ko04141), Glycerolipid metabolism (ko00561), Glycolysis/Gluconeogenesis (ko00010), Photosynthesis - antenna proteins (ko00196), Carotenoid biosynthesis (ko00906) and Flavonoid biosynthesis (ko00941). In addition, an enriched metabolic pathway of Plant-pathogen interaction (ko04626; p.adjust = 0.00014) was identified, suggesting the presence of a phytopathogen. Recent studies using this same transcriptomic dataset have described several viral (Quintanilha-Peixoto et al., 2021) and fungal (Marone et al., 2022) interactions in A. sisalana, so this metabolic pathway might be a response to these interactions. Furthermore, the flavonoid biosynthesis pathway (ko00941; p.adjust = 0.03169) enrichment and the accumulation of these bioactive compounds in Agave species (Barriada-Bernal et al., 2014; Ahumada-Santos et al., 2013; Morreeuw et al., 2021) may indicate protection against these phytopathogens (Ferreyra. 2012) or may be considered a physiological adaptation to stress conditions (exposure to UV radiation, high temperature, high luminosity (photoprotection) and nutrient deficiency) (Agati et al., 2012, 2013; Taiz and Zeiger, 2003; Yonekura-Sakakibara et al., 2019; Zahedi et al., 2021; Zeng et al., 2021).
3.4 Organ-specificity analysis
Tissue-specificity analysis (Tau metric of 0.90, see methods for details) identified transcripts expressed exclusively in one of the three tissues studied (leaf, stem, and root). From a total of 2,582 genes in the A. sisalana interactome, 2, 18, and 101 were identified in the stem, leaf, and root, respectively (Supplementary File S2). We found a leaf-specific LIM zinc finger protein (AS_TRINITY_DN73400_c0_g1_i1; Tau = 1), which may be involved in plant growth and development, as well as regulating resistance mechanisms to diverse biotic and abiotic stresses as observed in other plants (Gupta et al., 2012).
As a stem-specific transcript, we found an auxin transporter regulating intracellular auxin homeostasis and metabolism (AS_TRINITY_DN46645_c0_g1_i1; Tau = 0.941) which is crucial for plant development (Rosquete et al., 2012). However, auxin has differential distribution (gradients) within plant tissues, thus this gene may be acting as a gatekeeper controlling auxin traffic in and out of plant cells. Finally, among the root-specific genes, we found regulation of jasmonic acid mediated signaling pathway (GO:2000022; p-value: 0.00019), transfer of electrons from cytochrome c to oxygen (GO:0006123; p-value: 0.02372) and photosynthesis (GO:0015979; p-value:0.00022).
3.5 Submodule identification
A total of 16 submodules were identified in the A. sisalana interactome. Each submodule has highly connected genes and can suggest specific processes and roles. The GO enrichment of each submodule (Supplementary File S2) identified two submodules related to methylation and DNA repair/recombination: submodule S9 has 5 genes related to histone methylation (GO:0016571; p-value 8.2e-04), whose expression values are similar in the three tissues, with maximum values ranging from 10 to 20 TPM. Submodule S5 has 21 genes related to DNA repair (GO:0006281; p-value 5e-04), DNA topological change (GO:0006265; p-value 3.7e-06), and DNA recombination (GO:0006310; p-value 5.3e-06), which are all related to DNA damage response. Most genes in submodule 5 have expression values above 10 TPM in all tissues, but genes related to DNA recombination and DNA repair have TPM values from 20 to 142, suggesting these processes were highly demanded by the plant at the moment of sampling. Indeed, the samples were collected at noon and UV light exposure and/or the presence of Reactive Oxygen Species (ROS) can induce the plant response mechanism to fix any damage (Nisa et al., 2019).
We found 3 submodules related to carbohydrate metabolism (S7, S11, and S15). Among them, S11 is the only submodule presenting only biological processes associated with carbohydrate metabolism (GO:0005975; p-value 6.3e-06). Interestingly, the S11 submodule contains the Raffinose synthase gene (AS_TRINITY_DN57582_c0_g2_i1) with TPM values of 28 in the leaf tissue. Raffinose has been previously described as an important carbohydrate in Agave metabolism (Raya et al., 2021) and it is an osmolyte that can act both in drought and oxidative stress (Nishizawa et al., 2008). As for the S7 submodule, we identified GO terms sucrose biosynthetic process (GO:0005986; p-value 7.9e-06) and starch biosynthetic process (GO:0019252; p-value 1.9e-14) as well as terms related to gene regulation, like transcription from plastid promoter (GO:0042793; p-value 2.7e-04), cytidine to uridine editing (GO:0016554; p-value 7.6e-04), and Group II intron splicing (GO:0000373; p-value 5.1e-07). Finally, submodule S15 presented the term regulation of starch biosynthetic process (GO:0010581; p-value 8.8e-05) and several terms related to abiotic stress. Several heat-shock proteins (HSP) were found in this submodule in categories like response to heat (GO:0009408; p-value 1.4e-07), chaperone mediated protein folding requiring cofactor (GO:0051085; p-value 6.5e-04), and protein folding (GO:0006457; p-value 7.9e-06). Among this HSP, Small HSP and DnaJ molecular chaperone were more present. Curiously, the term histone H3 deacetylation was also present in this submodule. The level of histone acetylation has been shown to regulate plant response to drought stress, including ABA-responsive element binding protein (AREB) (Li et al., 2021). AREBs can regulate Dehydration responsive element binding (DREB), which are known for inducing HSP and Heat shock factors (HSF) (Agarwal et al., 2017).
Among the identified submodules, six of them were related to the photosynthesis process and were mostly composed of genes with higher expression in the leaf tissue (S1, S2, S3, S4, S10, and S13), which was expected due to the nature of the organism. Submodule S1 showed the following unique biological processes: nonphotochemical quenching (GO:0010196, p-value 1.4e-05), cellular response to high light intensity (GO:0071486, p-value 0.00024), photosystem II assembly (GO:0010207, p-value 1.4e-05), energy coupled proton transport, down electrochemical gradient (GO:0015985, p-value 2.0e-05), electron transport chain (GO:0022900, p-value 0.00020), starch biosynthetic process (GO:0019252, p-value 0.00014) and regulation of photosynthesis, dark reaction (GO:0010110, p-value 0.00024). The S2 submodule presented genes related to the unique processes protoporphyrinogen IX biosynthetic process (GO:0006782, p-value 0.00045), chloroplast-nucleus signaling pathway (GO:0010019, p-value 0.00058), DNA-templated transcription, termination (GO:0006353, p-value 0.00058) and cytidine to uridine editing (GO:0016554, p-value 0.00071). The biological processes unique to the S3 submodule were photosystem I assembly (GO:0048564, p-value 5.8e-06), protein import into chloroplast stroma (GO:0045037, p-value 0.00045), tetrahydrobiopterin biosynthetic process (GO:0006729, p-value 0.00096) and iron-sulfur cluster assembly (GO:0016226, p-value 0.00044). Submodule 10 has exclusives processes chlorophyll catabolic process (GO:0015996, p-value 2.5e-08), and a terpenoid biosynthetic process (GO:0016114, p-value 2e-04). The S13 submodule presented exclusive processes such as sucrose biosynthetic process (GO:0005986, p-value 2.6e-05), glycolytic process (GO:0006096, p-value 8.0e-05), isoprenoid biosynthetic process (GO:0008299, p-value 0.00036) and regulation of jasmonic acid mediated signaling pathway (GO:2000022, p-value 6.3e-10). By comparing the biological processes in common between these submodules, except for S10, we identified protein peptidyl-prolyl isomerization process, with the highest abundance of peptidylprolyl isomerase (PPIase) expression (AS_TRINITY_DN23174_c0_g1_i1) in leaf with 12 TPM. Interestingly, PPIase is responsible for the proper folding of proteins, being the only enzyme capable of catalyzing the cis-trans transition without energy requirement, unlike chaperones (Fischer et al., 1984; Fischer et al., 1989; Fanghänel and Fischer, 2004). Photosynthesis is a complex process involving several proteins, so the high expression of PPIase might be related to the correct folding of proteins without spending energy (Kirschbaum, 2004; Darko et al., 2014; Martin et al., 2018).
Lastly, submodule S14 has the processes of iron-sulfur (Fe-S) regulation (GO:0016226 and GO:0051176, p-values 4.6e-13 and 0.00038) and pyruvate metabolism (GO:0019450, p-value 0.00038). Plants have a high demand for iron in mitochondria and chloroplasts, mainly to ensure respiration and photosynthesis (Couturier et al., 2013). Iron-Sulphur is required in many metabolic pathways due to the presence of metalloproteins (Johnson et al., 2005). Moreover, Fe-S proteins play a wide range of functionality ranging from radical generation, electron transfer, participation in sulfur and nitrogen assimilation, DNA replication and repair, chlorophyll catabolism, and ribosome biogenesis (Johnson et al., 2005; Balk and Pilon, 2010). Regarding pyruvate metabolism, we found L-cysteine catabolic process to pyruvate (GO:0019450) was reported in this submodule, although the only gene present was L-cysteine desulfhydrase (LCD). LCD is responsible for cysteine degradation and is highly expressed in response to adverse environmental conditions and intracellular oxidative stress (Chen L Y et al., 2020; Wang et al., 2022), which agrees with agaves having a high capacity to be cultivated in places with more adverse environmental conditions.
3.6 Co-expression network analysis
We carried out a hierarchical clustering (WGCNA) to investigate the expression profile as a function of organs (Supplementary Figure S2A). During WGCNA analysis, we found β = 22 and R2 = 0.80 as the optimal soft threshold parameters to guarantee a scale-free network (Supplementary File 4: Figure 2S–B). We set the clustering height cut-off to 0.20 to merge similar modules, which resulted in 73 modules (Supplementary Figure S2C). Specifically, modules darkgreen, purple, darkred, darkturquoise, magenta, bisque4, mistyrose, lightyellow, pink, plum1, magenta4, darkolivegreen, blue4, turquoise, darkorange, salmon1, mediumpurple1, brown4, skyblue1, and plum4 were identified as statistically significant (|GS| > 0.2 and MM > 0.8; see methods for details) in at least one organ (Figure 3).
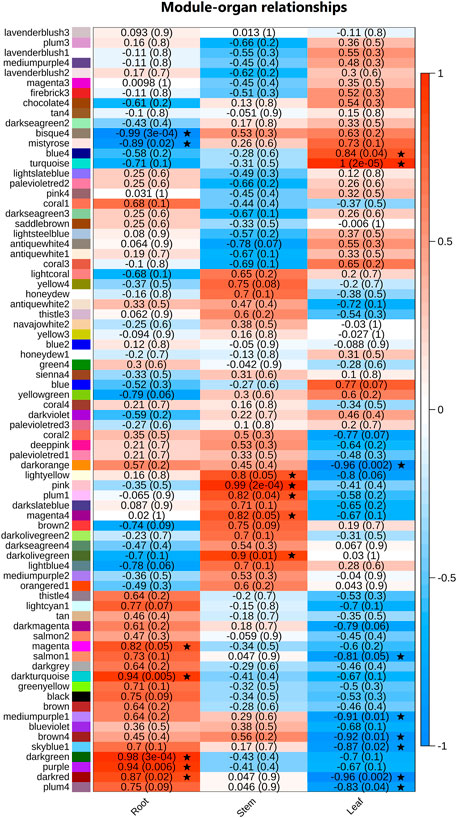
FIGURE 3. Heatmap of associations among module eigengenes in organ samples (stem, leaf, and root) from weighted correlation network analysis (WGCNA). A black star marks the key modules, i.e., modules with |cor| > 0.5 and p-value < = 0.05 in at least one organ.
To explore potential genes and pathways associated with each organ, we conducted GO enrichment analysis on the modules with the highest correlation with the organs. (Supplementary File S3). A total of 8 modules showed enriched GO terms: modules darkgreen, magenta, darkred, and purple are positively correlated with root; pink and darkolivegreen are positively correlated with stem, and turquoise and blue4 are positively correlated with leaf. Leaf was the only organ presenting negatively correlated modules: darkorange and darkred (Figure 3). All results of the main GO processes and KEGG pathways associated with each organ of A. sisalana are summarized in Figure 4.
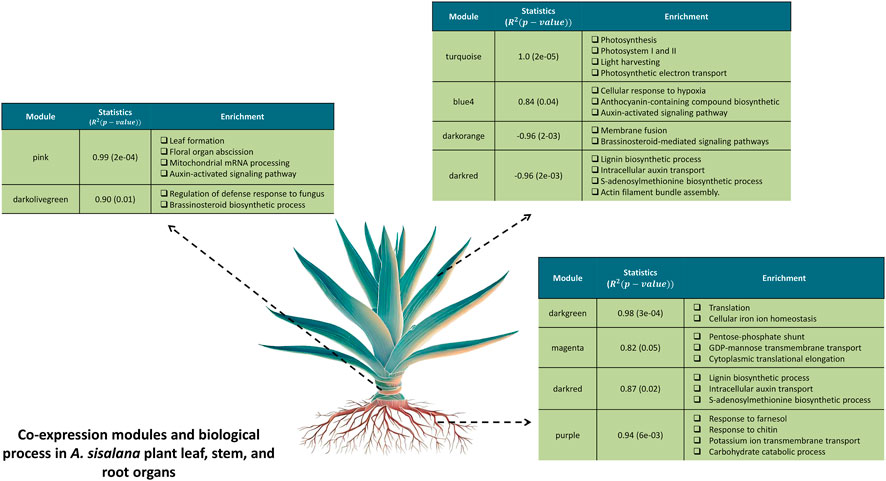
FIGURE 4. Co-expression modules and their associated biological processes in the leaf, stem, and root organs of the A. sisalana plant. For each co-expression module, we created summary tables highlighting the main enrichments observed, which included both correlation values and p-values obtained from the module-sample relationship analysis.
The darkgreen module genes (correlation of 0.99 with root expression profile) have enriched GO terms related to translation and cellular iron ion homeostasis (Supplementary Figure S1B). In addition, the magenta module showed enriched GO related to the pentose-phosphate shunt, GDP-mannose transmembrane transport, cytoplasmic translational elongation, and translation. The pentose-phosphate shunt may be acting on root ion transport systems. Studies suggest that the regulation of root nitrogen and sulfur acquisition by plant carbon status is governed by an unnamed oxidative pentose phosphate pathway-dependent sugar detection system, which coordinates the availability of these three elements for amino acid synthesis (Lejay et al., 2008).
The darkred module showed enriched GO terms related to the lignin biosynthetic process, protein localization to the membrane, intracellular auxin transport, S-adenosylmethionine biosynthetic process, and actin filament bundle assembly. The presence of the lignin biosynthetic process positively correlated with the root and negatively correlated with the leaf highlights the importance of lignin for different organs in the plant. This agrees with the low contents of lignin in leaf and stem in agaves (Raya et al., 2021), although there is no available quantification of lignin content in roots. Therefore, roots might possess more lignin to ensure mechanical support (Hoson and Wakabayashi, 2014), act as a defense barrier (Underwood, 2012), and a conduit for long-distance transportation of water and essential nutrients from the roots to the shoots (Boerjan et al., 2003; Zhong and Ye, 2015; Bonawitz and Chapple, 2010; Liu et al., 2018).
In the purple module, we observed the GO process related to response to farnesol, response to chitin, potassium ion transmembrane transport, transmembrane transport, and carbohydrate catabolic process. Here we highlight that the response process to farnesol is positively correlated with the root organ. Previous studies in Arabidopsis have shown that folk flowers, which lack farnesol kinase activity, accumulate farnesol and develop supernumerary carpels under water stress, providing evidence of a molecular link between the farnesol metabolism, abiotic stress signaling, and flower development (Fitzpatrick et al., 2011). Therefore, as the sampled plants were in a dry region, this group of genes was probably signaling the presence of this stress to the plant and activating another warning cascade. Moreover, we observed genes related to ABA signaling in submodule S15, which also shared a role with farnesol kinase in flower development (Fitzpatrick et al., 2011).
Regarding the modules highly correlated with the stem, pink module showed enriched GO terms related to protein phosphorylation, leaf formation, floral organ abscission, mitochondrial mRNA processing, auxin-activated signaling pathway, and regulation of transcription (Supplementary File S3). The process involving auxin signaling is responsible for ramet formation in Agave, involved in the survival and reproduction of a whole plant (Barreto et al., 2010). Another interesting process in the stem is the floral organ abscission. Abscission, in which plants shed unwanted organs, is a natural developmental program or in response to environmental stimuli (Patharkar and Walker, 2015). In A. sisalana, the genes related to abscission in this module, which are positively correlated with stem, could be floral repression genes, which can be part of further improvement studies to accelerate or repress flowering. Agaves are monocarpic, meaning they only flower once at the end of their lifespan, so better control of flowering time can help synchronize it in agave fields. Because agaves accumulate sugar in their stems to be used during flowering, this group of genes may be responsible for this accumulation. The relationship between each gene significance and module membership from the pink module presents a correlation of 0.9884 (Supplementary Figure S1C).
The GO enrichment of darkolivegreen module shows processes related to the regulation of defense response to fungus, brassinosteroid biosynthetic process, and positive regulation of transcription initiation from RNA polymerase II promoter. Indeed, fungal transcripts were described in the same dataset (Marone et al., 2022). Although most fungal transcripts were root-specific, some were present in the stem.
Regarding the modules highly correlated with the leaf, GO analysis of the turquoise module (positively correlated) demonstrated that genes in this module were primarily associated with photosynthesis, photosystem I and II, light harvesting, photosynthetic electron transport, and others (Supplementary File S3). Most of the processes are linked to photosynthesis processes, which makes the turquoise module a candidate module for the reconstruction of the interactome related to leaf genes. The findings of these photosynthesis-related genes revealed the strong linkage of these co-expressed genes to the cited GO terms in A. sisalana leaf. Previous findings in other plant transcriptomes also described that the primary function of light-harvesting complex (Lhc) proteins was related to light absorption by chlorophyll excitation and transfer of absorbed energy to photochemical reaction centers (Zhao et al., 2016; Ma et al., 2018). This relationship can be observed by the significance of the genes in relation to the module membership of the turquoise module, in which they present a correlation of 0.9989 (Supplementary Figure S1A).
The darkorange module is negatively correlated with the leaf (cor = −0.96 and p-value = 0.002). The GO enrichment shows processes related to membrane fusion and brassinosteroid-mediated signaling pathways. The brassinosteroid (BR) class of steroid hormones regulates plant development, growth, and physiology and is involved in mediating adaptation to abiotic stresses, such as drought, temperature changes, and salinity (Planas-Riverola et al., 2019). This result shows that these classes of genes play a key role in maintaining the delicate balance between growth and resilience to environmental threats. These mechanisms of hormonal responses and stress stimuli have already been observed in a previous study of A. sisalana (Sarwar et al., 2019).
Biological processes enriched in blue4 (positively correlated with leaf) are related to the cellular response to hypoxia, anthocyanin-containing compound biosynthetic, RNA biosynthetic process, and auxin-activated signaling pathway (Supplementary File S3). Anthocyanin and hypoxia-related genes may play a relevant role in the tolerance and adaptation of agave plants in stress conditions, such as high temperatures, high light, drought, and low O2 availability. Genes linked to the anthocyanin-containing compound biosynthetic process may have a role in response to high light incidence (Steyn et al., 2002; Tattini et al., 2014; Petrella et al., 2016; Trojak and Skowron, 2017.; Zheng et al., 2020) since anthocyanins are essential against UV radiation present in light stress conditions (Takahashi et al., 1991; Gould, 2004; Guo et al., 2010; Landi et al., 2015) and mitigate DNA damage in UV-B-irradiated (Takahashi et al., 1991; Kootstra, 1994; Stapleton and Walbot, 1994).
Also, plant responses to oxygen limitation (anoxia and hypoxia) are modulated by common signaling pathways, which target metabolic adaptations (Chang et al., 2011). Under oxygen limitations, tolerant plants increased glycolytic flux or metabolic depression, reducing ATP consumption (Sasidharan et al., 2017). Hypoxia induces a decline in stomatal conductance with a rapid decrease in the rate of photosynthesis (Malik et al., 2001; Araki et al., 2012), however, it was shown that leaves with high CAM activity (crassulacean acid metabolism) have higher liquid photosynthesis under hypoxia (Pedersen et al., 2011). Moreover, CAM plants have their stomata closed during the day and open only at night, when they fix CO2 (Males and Griffiths, 2017). Hypoxic pre-treated organs have been shown to have the ability to maintain a high glycolytic rate during prolonged periods of anoxia, as well as higher levels of ATP and energy load (Mugnai et al., 2011). There is a close correlation between anoxia tolerance and carbohydrate reserves (Zahra et al., 2021). Carbohydrates, specifically sugars, increase hypoxia tolerance in many species due to their close association as an energy-providing metabolite (Setter et al., 1997; Ram et al., 2002). In addition, drought stress quickly causes an osmotic imbalance (Gurrieri et al., 2020). As the severity of stress increases, plants face drought by accumulating high intracellular levels of osmoprotective compounds (i.e., sugars) to protect cellular components and restore osmotic balance (Singh et al., 2015; Sharma et al., 2019).
3.7 Selection of key genes
For the selection of key genes, we combined the results of interactome with the co-expression network analysis through the selection of hub genes with high significance and module membership in the module-organ relationship. Thus, a total of six hub genes were selected as key genes for participating in four WGCNA modules (turquoise, blue, and blue4) that are positively correlated to leaf, in addition to having a negative correlation with root and stem, revealing that they might also play roles in these two organs. The scoring of each hub gene in PPI and WGCNA is summarized in Table 3. For further validation of these potential key genes, we compared their expression values between organ samples in the dataset. Expression levels of these four key genes in the turquoise module were markedly elevated in leaf samples compared with stem and root samples (Figure 5).
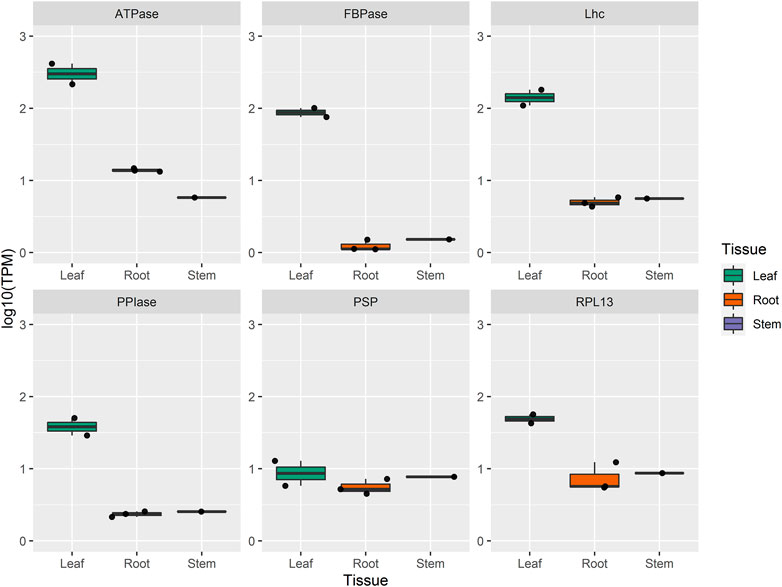
FIGURE 5. Per organ normalized expression of six hub genes identified in PPI of A. sisalana. AS_TRINITY_DN57726_c0_g1_i1 (PSP); AS_TRINITY_DN57407_c0_g1_i1 (FBPase); AS_TRINITY_DN51514_c0_g1_i1 (RPL13); AS_TRINITY_DN7474_c0_g1_i1 (ATPase); AS_TRINITY_DN57016_c0_g1_i1 (PPIase); AS_TRINITY_DN57140_c0_g1_i1 (Lhc).
One of these transcripts is the Protein-serine/threonine phosphatase gene (AS_TRINITY_DN57726_c0_g1_i1). This transcript, which was also identified as a highly connected hub, is a protein phosphatase 2C (PP2C). The ortholog of this gene in Arabidopsis (AtPP2C19, At2g20050) (Schweighofer et al., 2004; Xue et al., 2008) is a possible interacting protein of [Ca2+]cyt-assosiated protein kinase 1 (CAP1, At5G61350) (Yu et al., 2022) and it has been shown to contain the HSE motif, which is a cis-acting element involved in heat stress responsiveness (Xue et al., 2008). In A. thaliana, protein phosphatase 2Cs (PP2Cs) has been shown as a major negative regulator of ABA signaling (Umezawa et al., 2009), however, this gene does not group with genes related to this function (Schweighofer et al., 2004; Xue et al., 2008) and recent evidence indicates that it may be related to photosynthesis (chlorophyll molecule) and acquisition of magnesium ions, since it is co-expressed with magnesium transporters (Heidari et al., 2021). Furthermore, in Agave, the diel expression pattern of PP2C is inverted when compared to A. thaliana, and, for that, it has been proposed as one of the main regulators of stomata activity in CAM photosynthesis (Abraham et al., 2016). Moreover, D-fructose-1,6-bisphosphate1-phosphohydrolase (FBPase) (AS_TRINITY_DN57407_c0_g1_i1) is an important enzyme (EC 3.1.3.11) that acts mainly in the regulation of the Calvin cycle and glycogenesis. These reactions are involved in carbon fixation and sugar metabolism present in the chloroplast stroma and cytosol (Flechner et al., 1999). The ortholog in Arabidopsis encodes a chloroplastic FBPase (cFBP1, At3g54050) also known as HIGH CYCLIC ELECTRON FLOW 1 (HCEF1) involved in the regeneration of ribulose 1,5-bisphosphate (RuBP) and in the starch synthesis pathway (Livingston et al., 2010a; Rojas-González et al., 2015). The Arabidopsis mutant cfbp1/hcef1 shows a dwarf phenotype, chlorotic leaves, a low photosynthesis rate, high sucrose/starch rate (Rojas-González et al., 2015) and displays constitutively high cyclic electron flow (CEF) rates (Livingston et al., 2010b; Strand et al., 2015; Sharkey and Weise, 2016). Peptidylprolyl isomerase (PPIase) (AS_TRINITY_DN57016_c0_g1_i1) (EC 5.2.1.8), which are the only enzymes known that can catalyze cis-trans transition of peptide bonds, essential for the proper folding of proteins (Fischer et al., 1984) and belong to three major classes of proteins: cyclophilins, FK506-binding proteins or FKBPs, and parvulins (Singh et al., 2020). Interestingly, in our analyses, PPIase was reported to be present in all photosynthesis submodules. The ortholog in Arabidopsis encodes CYCLOPHILIN 38 (AtCYP38, At3g01480) and the mutant showed retarded growth and enhanced sensitivity to high light (Fu et al., 2007). In addition, Duan et al. (2021) reported on the importance of CYP38-dependent photosynthetic activity in supporting lateral root emergence. AtCYP38 in the thylakoid lumen functions in the assembly, maintenance of photosystem II (PSII; Fu et al., 2007; Sirpiö et al., 2008; Lepeduš et al., 2009; Vojta et al., 2019) and accumulation of PSII-LHCII supercomplexes (Zhu et al., 2022) but does not have PPIase activity in vitro (Vasudevan et al., 2012). Light-harvesting chlorophyll a/b-binding protein (Lhc) (AS_TRINITY_DN57140_c0_g1_i1) are a class of antennae proteins that play indispensable roles in capturing solar energy as well as photoprotection under stress conditions (Zhao et al., 2020). In Arabidopsis, the ortholog is known as PHOTOSYSTEM B PROTEIN 33 (AtPSB33, At1g71500) (Fristedt et al., 2015). The nucleus-encoded protein AtPSB33 has a crucial role in maintaining the stability of photosystem II complex and regulating photosynthesis, especially under fluctuating, high light and UV-A light stress conditions, and plants lacking PSB33 have a dysfunctional state transition (Fristedt et al., 2015; Fristedt et al., 2017; Kato et al., 2017; Nilsson et al., 2020). Lastly, ATPase, \F1 complex, OSCP/delta subunit (AS_TRINITY_DN7474_c0_g1_i1), which was also selected as a key gene, is present in the blue4 module of the WGCNA analysis. This specific module has a high correlation with the leaf (r = 0.84 and p = 0.04). cpATPase are essential for photosynthesis and their absence causes loss of photoautotrophy and increased photosensitivity. F-type ATP synthase uses the electrochemical proton gradient generated by photosynthesis to produce ATP from ADP and inorganic phosphate (Maiwald et al., 2003; Hahn et al., 2018).
4 Conclusion
In this study, we predicted 2,582 interactome components in A. sisalana using model organism PPI databases, with the numbers of A. sisalana interactome components determined from the numbers of model organism PPIs and A. sisalana orthologues. Also, we used RNA-Seq data from A. sisalana organs to identify co-expressed genes and, consequently, co-expression modules that were positively correlated with leaf, stem, and root organs in an analysis of the module-organ relationship. In the co-expression analysis with WGCNA, we found 72 co-expression modules, of which turquoise, blue, and blue4 were closely associated with leaf organ.
From the co-expression analysis of the module-organ relationship, we were able to identify key modules and their associations with three different organs of A. sisalana (leaf, stem, and root). These associations identified genes that play the role of abscission which can be part of further improvement studies to accelerate or repress flowering. Also, we found anthocyanin and hypoxia-related genes which may play a relevant role in the tolerance and adaptation of Agave plants, and genes with a role in adaptation to stress through brassinosteroid-mediated signaling pathways.
We identified key genes from the interactome network analysis through hub genes identification metrics and performed a correlation with their association in the module-organ relationship. Further analysis suggested that six candidate genes were positively significantly related to leaf organ and negatively related to stem and root, revealing that the key genes in the leaf have a role in maintaining the stability and organization of photosynthetic apparatus during stress conditions.
In conclusion, the predicted PPI network of A. sisalana expands the possibility of comparative analyses with other Agave species, thus providing additional insight into network evolution among species. Furthermore, the identification of co-expression modules and their module-organ relationship, together with the identification of key genes, suggest target genes for specific studies in Agave transcriptome studies, biological processes related to plant survival in unfavorable environments and provide strategies for breeding programs.
Data availability statement
The datasets presented in this study can be found in online repositories. The names of the repository/repositories and accession number(s) can be found in the article/Supplementary Material.
Author contributions
LC: Conceptualization, Methodology, Software, Validation, Formal analysis, Investigation, Data curation, Writing-Original draft preparation, Writing-Review and editing, Visualization. NS: Investigation, Data curation, Writing-Original draft preparation, Writing-Review and editing, Visualization. LA: Investigation, Data curation, Writing-Original draft preparation, Writing- Review and editing. MM: Investigation, Data curation, Writing-Original draft preparation, Writing-Review and editing. AC: Software, Writing-Review and editing. FR: Investigation, Data curation, Writing-Original draft preparation, Writing- Review and editing. GA: Writing- Review and editing. MC: Investigation, Methodology, Data curation, Writing-Original draft preparation, Writing- Review and editing. GG: Writing-Original draft preparation, Resources, Writing-Review and editing. All authors contributed to the article and approved the submitted version.
Funding
This work was financed by the Center for Computational Engineering and Sciences–FAPESP/Cepid (2013/08293-7) and the São Paulo Research Foundation (FAPESP) through grants 2019/12914-3 and 2020/02524-0.
Acknowledgments
We would like to thank the São Paulo Research Foundation (FAPESP) and the Center for Computational Engineering and Sciences-FAPESP/Cepid (2013/08293-7) for all infrastructure and financial support. Also, we would like to thank Shell Brasil and ANP (Brazilian National Agency of Petroleum, Natural Gas and Biofuels) for the strategic support provided through the regulatory incentive for Research, Development & Innovation.
Conflict of interest
The authors declare that the research was conducted in the absence of any commercial or financial relationships that could be construed as a potential conflict of interest.
Publisher’s note
All claims expressed in this article are solely those of the authors and do not necessarily represent those of their affiliated organizations, or those of the publisher, the editors and the reviewers. Any product that may be evaluated in this article, or claim that may be made by its manufacturer, is not guaranteed or endorsed by the publisher.
Supplementary material
The Supplementary Material for this article can be found online at: https://www.frontiersin.org/articles/10.3389/fceng.2023.1175235/full#supplementary-material
References
Abraham, P. E., Yin, H., Borland, A. M., Weighill, D., Lim, S. D., De Paoli, H. C., et al. (2016). Transcript, protein and metabolite temporal dynamics in the CAM plant Agave. Plants 2, 16178. doi:10.1038/nplants.2016.178
Agarwal, P. K., Gupta, K., Lopato, S., and Agarwal, P. (2017). Dehydration responsive element binding transcription factors and their applications for the engineering of stress tolerance. J. Exp. Bot. 68, 2135–2148. doi:10.1093/jxb/erx118
Agati, G., Azzarello, E., Pollastri, S., and Tattini, M. (2012). Flavonoids as antioxidants in plants: location and functional significance. Plant sci. 196, 67–76. doi:10.1016/j.plantsci.2012.07.014
Ahumada-Santos, Y. P., Montes-Avila, J., de Jesús Uribe-Beltrán, M., Díaz-Camacho, S. P., López-Angulo, G., Vega-Aviña, R., et al. (2013). Chemical characterization, antioxidant and antibacterial activities of six Agave species from Sinaloa, Mexico. Industrial Crops and Products 49, 143–149. doi:10.1016/j.indcrop.2013.04.050
Alexa, A., Rahnenführer, J., and Lengauer, T. (2006). Improved scoring of functional groups from gene expression data by decorrelating GO graph structure. Bioinformatics 22, 1600–1607. doi:10.1093/bioinformatics/btl140
Araki, H., Hossain, M. A., and Takahashi, T. (2012). Waterlogging and hypoxia have permanent effects on wheat root growth and respiration. J. Agron. Crop Sci. 198, 264–275. doi:10.1111/j.1439-037x.2012.00510.x
Aramaki, T., Blanc-Mathieu, R., Endo, H., Ohkubo, K., Kanehisa, M., Goto, S., et al. (2020). KofamKOALA: KEGG ortholog assignment based on profile HMM and adaptive score threshold. Bioinformatics 36, 2251–2252. doi:10.1093/bioinformatics/btz859
Balk, J., and Pilon, M. (2010). Ancient and essential: The assembly of iron–sulfur clusters in plants. Trends Plant Sci. 16, 218–226. doi:10.1016/j.tplants.2010.12.006
Barriada-Bernal, L. G., Almaraz-Abarca, N., Delgado-Alvarado, E. A., Gallardo-Velázquez, T., Ávila-Reyes, J. A., Torres-Morán, M. I., et al. (2014). Flavonoid composition and antioxidant capacity of the edible flowers of Agave durangensis (Agavaceae). CyTA-Journal of Food 12(2), 105–114. doi:10.1080/19476337.2013.801037
Barreto, R., Nieto-Sotelo, J., and Cassab, G. I. (2010). Influence of plant growth regulators and water stress on ramet induction, rosette engrossment, and fructan accumulation in Agave tequilana Weber var. Azul. Azul. Plant Cell. Tissue Organ Cult. 103, 93–101. doi:10.1007/s11240-010-9758-9
Boerjan, W., Ralph, J., and Baucher, M. (2003). Lignin biosynthesis. Annu. Rev. Plant Biol. 54, 519–546. doi:10.1146/annurev.arplant.54.031902.134938
Bolger, A. M., Lohse, M., and Usadel, B. (2014). Trimmomatic: A flexible trimmer for illumina sequence data. Bioinformatics 30, 2114–2120. doi:10.1093/bioinformatics/btu170
Bonawitz, N. D., and Chapple, C. (2010). The genetics of lignin biosynthesis: Connecting genotype to phenotype. Annu. Rev. Genet. 44, 337–363. doi:10.1146/annurev-genet-102209-163508
Borland, A. M., Hartwell, J., Weston, D. J., Schlauch, K. A., Tschaplinski, T. J., Tuskan, G. A., et al. (2014). Engineering crassulacean acid metabolism to improve water-use efficiency. Trends Plant Sci. 19, 327–338. doi:10.1016/j.tplants.2014.01.006
Braun, P., Aubourg, S., Van Leene, J., De Jaeger, G., and Lurin, C. (2013). Plant protein interactomes. Annu. Rev. Plant Biol. 64, 161–187. doi:10.1146/annurev-arplant-050312-120140
Bray, N. L., Pimentel, H., Melsted, P., and Pachter, L. (2016). Near-optimal probabilistic RNA-seq quantification. Nat. Biotechnol. 2016 345 (34), 525–527. doi:10.1038/nbt.3519
Broeren, M. L. M., Dellaert, S. N. C., Cok, B., Patel, M. K., Worrell, E., and Shen, L. (2017). Life cycle assessment of sisal fibre – exploring how local practices can influence environmental performance. J. Clean. Prod. 149, 818–827. doi:10.1016/j.jclepro.2017.02.073
Camargo, A. P., Vasconcelos, A. A., Fiamenghi, M. B., Pereira, G. A., and Carazzolle, M. F., (2020). tspex: a tissue-specificity calculator for gene expression data. Research Square. doi:10.21203/rs.3.rs-51998/v1
Chang, R., Jang, C. J. H., Branco-Price, C., Nghiem, P., and Bailey-Serres, J. (2011). Transient MPK6 activation in response to oxygen deprivation and reoxygenation is mediated by mitochondria and aids seedling survival in Arabidopsis. Plant Mol. Biol. 2011 781 (78), 109–122. doi:10.1007/s11103-011-9850-5
Chehebar, C., and Wolosiuk, R. A. (1980). Studies on the regulation of chloroplast fructose-1,6-bisphosphatase. Activation by fructose 1,6-bisphosphate. Biochim. Biophys. Acta 613, 429–438. doi:10.1016/0005-2744(80)90097-2
Chen, L. Y., Xin, Y., Wai, C. M., Liu, J., and Ming, R. (2020). The role of cis-elements in the evolution of crassulacean acid metabolism photosynthesis. Hortic. Res. 7, 41438. doi:10.1038/S41438-019-0229-0/41989378/41438
Chin, C. H., Chen, S. H., Wu, H. H., Ho, C. W., Ko, M. T., and Lin, C. Y. (2014). cytoHubba: identifying hub objects and sub-networks from complex interactome. BMC Syst. Biol. 8, S11–S17. doi:10.1186/1752-0509-8-s4-s11
Couturier, J., Touraine, B., Briat, J. F., Gaymard, F., and Rouhier, N. (2013). The iron-sulfur cluster assembly machineries in plants: Current knowledge and open questions. Plant Sci. 4, 259. doi:10.3389/fpls.2013.00259
Darko, E., Heydarizadeh, P., Schoefs, B., and Sabzalian, M. R. (2014). Photosynthesis under artificial light: The shift in primary and secondary metabolism. Philosophical Trans. R. Soc. B Biol. Sci. 369, 20130243. doi:10.1098/rstb.2013.0243
Davis, S. C., Dohleman, F. G., and Long, S. P. (2011). The global potential for Agave as a biofuel feedstock. GCB Bioenergy 3, 68–78. doi:10.1111/j.1757-1707.2010.01077.x
de Silva, K. K., Dunwell, J. M., and Wickramasuriya, A. M. (2022). Weighted Gene Correlation Network Analysis (WGCNA) of Arabidopsis somatic embryogenesis (SE) and identification of key gene modules to uncover SE-associated hub genes. Int. J. Genomics 24, 1–24. doi:10.1155/2022/7471063
Ding, Z., and Kihara, D. (2019). Computational identification of protein-protein interactions in model plant proteomes. Sci. Rep. 2019 91 (9), 8740–8813. doi:10.1038/s41598-019-45072-8
Duan, L., Pérez-Ruiz, J. M., Cejudo, F. J., and Dinneny, J. R. (2021). Characterization of CYCLOPHILLIN38 shows that a photosynthesis-derived systemic signal controls lateral root emergence. Plant Physiol. 185 (2), 503–518. doi:10.1093/plphys/kiaa032
Eguiarte, L. E., Jiménez Barrón, O. A., Aguirre-Planter, E., Scheinvar, E., Gámez, N., Gasca-Pineda, J., et al. (2021). Evolutionary ecology of agave: Distribution patterns, phylogeny, and coevolution (an homage to howard S. Gentry). Am. J. Bot. 108, 216–235. doi:10.1002/ajb2.1609
Emms, D. M., and Kelly, S. (2019). OrthoFinder: Phylogenetic orthology inference for comparative genomics. Genome Biol. 20, 238–314. doi:10.1186/s13059-019-1832-y
Fanghanel, J., and Fischer, G. (2004). Insights into the catalytic mechanism of peptidyl prolyl cis/trans isomerases. Front. Biosci. 9, 3453–3478. doi:10.2741/1494
Feng, X., Yang, Z., and Wang, X. (2021). Tissue-specific transcriptome analysis of drought stress and rehydration in Trachycarpus fortunei at seedling. PeerJ 9, e10933. doi:10.7717/peerj.10933
Ferreira, S. S., Hotta, C. T., Poelking, V. G. D. C., Leite, D. C. C., Buckeridge, M. S., Loureiro, M. E., et al. (2016). Co-expression network analysis reveals transcription factors associated to cell wall biosynthesis in sugarcane. Plant Mol. Biol. 91, 15–35. doi:10.1007/s11103-016-0434-2
Ferreyra, F., Rius, S. P., Casati, P., Rius, M. L., and Casati, S. P. (2012). Flavonoids: Biosynthesis, biological functions, and biotechnological applications. Front. Plant Sci. 3, 222. doi:10.3389/fpls.2012.00222
Fischer, G., Bang, H., Berger, E., and Schellenberger, A. (1984). Conformational specificity of chymotrypsin toward proline-containing substrates. Biochim. Biophys. Acta 791, 87–97. doi:10.1016/0167-4838(84)90285-1
Fischer, G., Wittmann-Liebold, B., Lang, K., Kiefhaber, T., and Schmid, F. X. (1989). Cyclophilin and peptidyl-prolyl cis-trans isomerase are probably identical proteins. Nature 337, 476–478. doi:10.1038/337476a0
Fitzpatrick, A. H., Shrestha, N., Bhandari, J., and Crowell, D. N. (2011). Roles for farnesol and ABA in Arabidopsis flower development. Plant Signal. Behav 6, 1189–1191. doi:10.4161/psb.6.8.15772
Flechner, A., Gross, W., Martin, W. F., and Schnarrenberger, C. (1999). Chloroplast class I and class II aldolases are bifunctional for fructose-1,6-biphosphate and sedoheptulose-1,7-biphosphate cleavage in the Calvin cycle. FEBS Lett. 447, 200–202. doi:10.1016/s0014-5793(99)00285-9
Fraser, H. B. (2005). Modularity and evolutionary constraint on proteins. Nat. Genet. 37, 351–352. doi:10.1038/ng1530
Fristedt, R., Herdean, A., Blaby-Haas, C. E., Mamedov, F., Merchant, S. S., Last, R. L., et al. (2015). PHOTOSYSTEM II PROTEIN33, a protein conserved in the plastid lineage, is associated with the chloroplast thylakoid membrane and provides stability to photosystem II supercomplexes in Arabidopsis. Plant Physiol. 167, 481–492. doi:10.1104/pp.114.253336
Fristedt, R., Trotta, A., Suorsa, M., Nilsson, A. K., Croce, R., Aro, E. M., et al. (2017). PSB33 sustains photosystem II D1 protein under fluctuating light conditions. J. Exp. Bot. 68 (15), 4281–4293. doi:10.1093/jxb/erx218
Fu, A., He, Z., Cho, H. S., Lima, A., Buchanan, B. B., and Luan, S. (2007). A chloroplast cyclophilin functions in the assembly and maintenance of photosystem II in Arabidopsis thaliana. Proc. Natl. Acad. Sci. U. S. A 104, 15947–15952. doi:10.1073/pnas.0707851104
Gould, K. S. (2004). Nature’s Swiss army knife: The diverse protective roles of anthocyanins in leaves. J. Biomed. Biotechnol. 2004, 314–320. doi:10.1155/s1110724304406147
Guo, J., Han, W., and Wang, M.-H. (2010). Ultraviolet and environmental stresses involved in the induction and regulation of anthocyanin biosynthesis: A review. African J. Biotechnol 7, 4966–4972. doi:10.4314/ajb.v7i25.59709
Gupta, S. K., Rai, A. K., Kanwar, S. S., and Sharma, T. R. (2012). Comparative analysis of zinc finger proteins involved in plant disease resistance. PLoS One 7 (8), e42578. doi:10.1371/journal.pone.0042578
Gurrieri, L., Merico, M., Trost, P., Forlani, G., and Sparla, F. (2020). Impact of drought on soluble sugars and free proline content in selected arabidopsis mutants. Biol. 2020 9, 367. doi:10.3390/biology9110367
Hahn, A., Vonck, J., Mills, D. J., Meier, T., and Kühlbrandt, W. (2018). Structure, mechanism, and regulation of the chloroplast ATP synthase. Science 360, 1. doi:10.1126/SCIENCE.AAT4318
Heidari, P., Faraji, S., and Poczai, P. (2021). Magnesium transporter gene family: Genome-wide identification and characterization in theobroma cacao, corchorus capsularis, and gossypium hirsutum of family malvaceae. Agronomy 11, 1651. doi:10.3390/agronomy11081651
Hoson, T., and Wakabayashi, K. (2014). Role of the plant cell wall in gravity resistance. Phytochemistry 112, 84–90. doi:10.1016/j.phytochem.2014.08.022
International Energy Agency (IEA). (2021). Renewables 2021. Available at: https://www.iea.org/reports/renewables-2021 (Accessed May 20, 2023).
Jiménez-Barron, O., García-Sandoval, R., Magallón, S., García-Mendoza, A., Nieto-Sotelo, J., Aguirre-Planter, E., et al. (2020). 2020. Phylogeny, diversification rate, and divergence time of agave sensu lato (asparagaceae), a group of recent origin in the process of diversification. Front. Plant Sci. 11, 1651. doi:10.3389/FPLS
Johnson, D. C., Dean, D. R., Smith, A. D., and Johnson, M. K. (2005). Structure, function, and formation of biological iron-sulfur clusters. Annu. Rev. Biochem. 74, 247–281. doi:10.1146/annurev.biochem.74.082803.133518
Kato, Y., Yokono, M., Akimoto, S., Takabayashi, A., Tanaka, A., and Tanaka, R. (2017). Deficiency of the stroma-lamellar protein LIL8/PSB33 affects energy transfer around PSI in Arabidopsis. Plant Cell Physiology 58 (11), 2026–2039. doi:10.1093/pcp/pcx124
Khan, Z. H., Agarwal, S., Rai, A., Memaya, M. B., Mehrotra, S., and Mehrotra, R. (2020). Co-expression network analysis of protein phosphatase 2A (PP2A) genes with stress-responsive genes in Arabidopsis thaliana reveals 13 key regulators. Sci. Rep. 10, 21480–21516. doi:10.1038/s41598-020-77746-z
Kirschbaum, M. U. F. (2004). Direct and indirect climate change effects on photosynthesis and transpiration. Plant Biol. 6, 242–253. doi:10.1055/s-2004-820883
Kootstra, A. (1994). Protection from UV-B-induced DNA damage by flavonoids. Plant Mol. Biol. 26, 771–774. doi:10.1007/bf00013762
Kryuchkova-Mostacci, N., and Robinson-Rechavi, M. (2017). A benchmark of gene expression tissue-specificity metrics. Bioinform 18, 205–214. doi:10.1093/bib/bbw008
Landi, M., Tattini, M., and Gould, K. S. (2015). Multiple functional roles of anthocyanins in plant-environment interactions. Environ. Exp. Bot. 119, 4–17. doi:10.1016/j.envexpbot.2015.05.012
Langfelder, P., and Horvath, S. (2008). An R package for weighted correlation network analysis. BMC Bioinforma. 9, 1. doi:10.1186/1471-2105-9-559
Lejay, L., Wirth, J., Pervent, M., Cross, J. M. F., Tillard, P., and Gojon, A. (2008). Oxidative pentose phosphate pathway-dependent sugar sensing as a mechanism for regulation of root ion transporters by photosynthesis. Plant Physiol. 146, 2036–2053. doi:10.1104/pp.107.114710
Lepeduš, H., Tomašić, A., Jurić, S., Katanić, Z., Cesar, V., and Fulgosi, H. (2009). Photochemistry of PSII in CYP38 Arabidopsis thaliana mutant. Food technol. Biotechnol 47, 275–280. Available at https://hrcak.srce.hr/39844 (Accessed May 20, 2023).
Li, J., Sun, M., Li, H., Ling, Z., Wang, D., Zhang, J., et al. (2022). Full-length transcriptome-referenced analysis reveals crucial roles of hormone and wounding during induction of aerial bulbils in lily. BMC Plant Biol. 22, 415. doi:10.1186/s12870-022-03801-8
Li, S., He, X., Gao, Y., Zhou, C., Chiang, V. L., and Li, W. (2021). Histone acetylation changes in plant response to drought stress. Basel: Genes. doi:10.3390/GENES1209140912
Liu, Q., Luo, L., and Zheng, L. (2018). Biosynthesis and biological functions in plants. Int. J. Mol. Sci. 19, 1. doi:10.3390/IJMS19020335
Livingston, A. K., Cruz, J. A., Kohzuma, K., Dhingra, A., and Kramer, D. M. (2010a). An Arabidopsis mutant with high cyclic electron flow around photosystem I (hcef) involving the NADPH dehydrogenase complex. Plant Cell 22 (1), 221–233. doi:10.1105/tpc.109.071084
Livingston, A. K., Kanazawa, A., Cruz, J. A., and Kramer, D. M. (2010b). Regulation of cyclic electron flow in C3 plants: Differential effects of limiting photosynthesis at ribulose-1, 5-bisphosphate carboxylase/oxygenase and glyceraldehyde-3-phosphate dehydrogenase. Plant, Cell & Environ. 33 (11), 1779–1788. doi:10.1111/j.1365-3040.2010.02183.x
Ma, X., Zhao, H., Xu, W., You, Q., Yan, H., Gao, Z., et al. (2018). Co-Expression gene network analysis and functional module identification in bamboo growth and development. Front. Genet. 9, 574. doi:10.3389/fgene.2018.00574
Maiwald, D., Dietzmann, A., Jahns, P., Pesaresi, P., Joliot, P., Joliot, A., et al. (2003). Knock-out of the genes coding for the rieske protein and the ATP-synthase δ-subunit of arabidopsis. Effects on photosynthesis, thylakoid protein composition, and nuclear chloroplast gene expression. Plant Physiol. 133, 191–202. doi:10.1104/pp.103.024190
Males, J., and Griffiths, H. (2017). Stomatal Biology of CAM plants. Plant Physiol. 174, 550–560. doi:10.1104/pp.17.00114
Malik, A. I., Colmer, T. D., Lambers, H., and Schortemeyer, M. (2001). Changes in physiological and morphological traits of roots and shoots of wheat in response to different depths of waterlogging. Funct. Plant Biol. 28, 1121–1131. doi:10.1071/pp01089
Marone, M. P., Campanari, M. F. Z., Raya, F. T., Pereira, G. A. G., and Carazzolle, M. F. (2022). Fungal communities represent the majority of root-specific transcripts in the transcriptomes of Agave plants grown in semiarid regions. PeerJ 10, e13252. doi:10.7717/peerj.13252
Martin, W. F., Bryant, D. A., and Beatty, J. T. (2018). A physiological perspective on the origin and evolution of photosynthesis. FEMS Microbiol. Rev. 42, 205–231. doi:10.1093/femsre/fux056
Mielenz, J. R., Rodriguez, M., Thompson, O. A., Yang, X., and Yin, H. (2015). Development of Agave as a dedicated biomass source: Production of biofuels from whole plants. Biotechnol. Biofuels 8, 79–13. doi:10.1186/s13068-015-0261-8
Monja-Mio, K. M., Herrera-Alamillo, M. A., Sánchez-Teyer, L. F., and Robert, M. L. (2019). Breeding strategies to improve production of agave (spp) adv plant breed. Strateg. Ind. Food Crop 6, 319–362. doi:10.1007/978-3-030-23265-8
Morreeuw, Z. P., Castillo-Quiroz, D., Ríos-González, L. J., Martínez-Rincón, R., Estrada, N., Melchor-Martínez, E. M., et al. (2021). High throughput profiling of flavonoid abundance in Agave lechuguilla residue-valorizing under explored mexican plant. Plants 10(4), 695. doi:10.3390/plants10040695
Mugnai, S., Marras, A. M., and Mancuso, S. (2011). Effect of hypoxic acclimation on anoxia tolerance in vitis roots: Response of metabolic activity and K+ fluxes. Plant Cell Physiol. 52, 1107–1116. doi:10.1093/pcp/pcr061
Nilsson, A. K., Pěnčík, A., Johansson, O. N., Bånkestad, D., Fristedt, R., Suorsa, M., et al. (2020). PSB33 protein sustains photosystem II in plant chloroplasts under UV-A light. J. Exp. Bot. 71 (22), 7210–7223. doi:10.1093/jxb/eraa427
Nisa, M. U., Huang, Y., Benhamed, M., and Raynaud, C. (2019). The plant DNA damage response: Signaling pathways leading to growth inhibition and putative role in response to stress conditions. Plant Sci. 10, 653. doi:10.3389/fpls.2019.00653
Nishizawa, A., Yabuta, Y., and Shigeoka, S. (2008). Galactinol and raffinose constitute a novel function to protect plants from oxidative damage. Plant Physiol. 147, 1251–1263. doi:10.1104/pp.108.122465
Panahi, B., and Hejazi, M. A. (2021). Weighted gene co-expression network analysis of the salt-responsive transcriptomes reveals novel hub genes in green halophytic microalgae Dunaliella salina. Sci. Rep. 11, 1–11. doi:10.1038/s41598-020-80945-3
Patharkar, O. R., and Walker, J. C. (2015). Floral organ abscission is regulated by a positive feedback loop. S. A 112, 2906–2911. doi:10.1073/pnas.1423595112
Pedersen, O., Rich, S. M., Pulido, C., Cawthray, G. R., and Colmer, T. D. (2011). Crassulacean acid metabolism enhances underwater photosynthesis and diminishes photorespiration in the aquatic plant Isoetes australis. New Phytol. 190, 332–339. doi:10.1111/j.1469-8137.2010.03522.x
Petrella, D. P., Metzger, J. D., Blakeslee, J. J., Nangle, E. J., and Gardner, D. S. (2016). Anthocyanin production using rough bluegrass treated with high-intensity light. HortScience 51, 1111–1120. doi:10.21273/hortsci10878-16
Planas-Riverola, A., Gupta, A., Betegoń-Putze, I., and Bosch, N. 2019. Brassinosteroid signaling in plant development and adaptation to stress. Development 146. 1, doi:10.1242/DEV.151894
Quintanilha-Peixoto, G., Fonseca, P. L. C., Raya, F. T., Marone, M. P., Bortolini, D. E., Mieczkowski, P., et al. (2021). The sisal virome: Uncovering the viral diversity of agave varieties reveals new and organ-specific viruses. Microorganisms 9, 1. doi:10.3390/MICROORGANISMS9081704/S1
Ram, P. C., Singh, B. B., Singh, A. K., Ram, P., Singh, P. N., Singh, H. P., et al. (2002). Submergence tolerance in rainfed lowland rice: Physiological basis and prospects for cultivar improvement through marker-aided breeding. F. Crop. Res. 76, 131–152. doi:10.1016/s0378-4290(02)00035-7
Raya, F. T., Marone, M. P., Carvalho, L. M., Rabelo, S. C., de Paula, M. S., Campanari, M. F. Z., et al. (2021). Extreme physiology: Biomass and transcriptional profiling of three abandoned Agave cultivars. Ind. Crops Prod. 172, 114043. doi:10.1016/j.indcrop.2021.114043
Robinson, M. D., McCarthy, D. J., and Smyth, G. K. (2010). edgeR: a Bioconductor package for differential expression analysis of digital gene expression data. Bioinformatics 26, 139–140. doi:10.1093/bioinformatics/btp616
Rojas-González, J. A., Soto-Súarez, M., García-Díaz, , Mérida, Á., et al. (2015). Disruption of both chloroplastic and cytosolic FBPase genes results in a dwarf phenotype and important starch and metabolite changes in Arabidopsis thaliana. J. Exp. Bot. 66 (9), 2673–2689. doi:10.1093/jxb/erv062
Rosquete, M. R., Barbez, E., and Kleine-Vehn, J. (2012). Cellular auxin homeostasis: Gatekeeping is housekeeping. Mol. plant 5, 4,772–786. doi:10.1093/mp/ssr109
Sarwar, M. B., Ahmad, Z., Rashid, B., Hassan, S., Gregersen, P. L., Leyva, M. D., et al. (2019). De novo assembly of Agave sisalana transcriptome in response to drought stress provides insight into the tolerance mechanisms. Sci. Rep. 2019 91 (9), 396–414. doi:10.1038/s41598-018-35891-6
Sasidharan, R., Bailey-Serres, J., Ashikari, M., Atwell, B. J., Colmer, T. D., Fagerstedt, K., et al. (2017). Community recommendations on terminology and procedures used in flooding and low oxygen stress research. New Phytol. 214, 1403–1407. doi:10.1111/nph.14519
Schweighofer, A., Hirt, H., and Meskiene, I. (2004). Plant PP2C phosphatases: Emerging functions in stress signaling. Trends Plant Sci. 5, 236–243. doi:10.1016/j.tplants.2004.03.007
Setter, T. L., Ellis, M., Laureles, E. V., Ella, E. S., Senadhira, D., Mishra, S. B., et al. (1997). Physiology and genetics of submergence tolerance in rice. Bot 79, 67–77. doi:10.1093/oxfordjournals.aob.a010308
Shannon, P., Markiel, A., Ozier, O., Baliga, N. S., Wang, J. T., Ramage, D., et al. (2003). Cytoscape: A software environment for integrated models of biomolecular interaction networks. Genome Res. 13, 2498–2504. doi:10.1101/gr.1239303
Sharkey, T. D., and Weise, S. E. (2016). The glucose 6-phosphate shunt around the Calvin–Benson cycle. n 14, 4067–4077. doi:10.1093/jxb/erv484
Sharma, A., Shahzad, B., Kumar, V., Kohli, S. K., Sidhu, G. P. S., Bali, A. S., et al. (2019). Phytohormones regulate accumulation of osmolytes under abiotic stress. 2019 9, 285. doi:10.3390/biom9070285
Singh, H., Kaur, K., Singh, M., Kaur, G., and Singh, P. (2020). 2020. Plant cyclophilins: Multifaceted proteins with versatile roles. Front. Plant Sci. 11, 1558. doi:10.3389/FPLS
Singh, M., Kumar, J., Singh, S., Singh, V. P., and Prasad, S. M. (2015). Roles of osmoprotectants in improving salinity and drought tolerance in plants: A review. Rev. Environ. Sci. Bio/Technology 2015 143 (14), 407–426. doi:10.1007/s11157-015-9372-8
Sirpiö, S., Khrouchtchova, A., Allahverdiyeva, Y., Hansson, M., Fristedt, R., Vener, A. V., et al. (2008). AtCYP38 ensures early biogenesis, correct assembly and sustenance of photosystem II. AtCYP38 ensures early biogenesis, correct assembly sustenance Photosyst. II. Plant J 55, 639–651. doi:10.1111/j.1365-313x.2008.03532.x
Stapleton, A. E., and Walbot, V. (1994). Flavonoids can protect maize DNA from the induction of ultraviolet radiation damage. Plant Physiol. 105, 881–889. doi:10.1104/pp.105.3.881
Steyn, W. J., Wand, S. J. E., Holcroft, D. M., and Jacobs, G. (2002). Anthocyanins in vegetative tissues: A proposed unified function in photoprotection. New Phytol. 155, 349–361. doi:10.1046/j.1469-8137.2002.00482.x
Strand, D. D., Livingston, A. K., Satoh-Cruz, M., Froehlich, J. E., Maurino, V. G., and Kramer, D. M. (2015). Activation of cyclic electron flow by hydrogen peroxide in vivo. Proc. Natl. Acad. Sci. 112 (17), 5539–5544. doi:10.1073/pnas.1418223112
Suinaga, F. A., Silva, O. R. R. F., Coutinho, W. M., Cartaxo, W. V., and Costa, L. B. (2007). Avaliação agronômica de Oito genótipos de Sisal (agave spp.), Técnico: Comun.
Szklarczyk, D., Franceschini, A., Kuhn, M., Simonovic, M., Roth, A., Minguez, P., et al. (2011). The STRING database in 2011: Functional interaction networks of proteins, globally integrated and scored. Nucleic Acids Res. 39, 1. doi:10.1093/nar/gkq973
Takahashi, A., Takeda, K., and Ohnishi, T. (1991). Light-Induced anthocyanin reduces the extent of damage to DNA in UV-irradiated centaurea cyanus cells in culture. Plant Cell Physiol. 32, 541–547. doi:10.1093/OXFORDJOURNALS.PCP.A078113
Tattini, M., Landi, M., Brunetti, C., Giordano, C., Remorini, D., Gould, K. S., et al. (2014). Epidermal coumaroyl anthocyanins protect sweet basil against excess light stress: Multiple consequences of light attenuation. Physiol. Plant 152, 585–598. doi:10.1111/ppl.12201
Törönen, P., Medlar, A., and Holm, L. (2018). PANNZER2: A rapid functional annotation web server. Nucleic Acids Res. 46, W84–W88. doi:10.1093/nar/gky350
Trojak, M., and Skowron, E. (2017). Role of anthocyanins in high-light stress response. World Sci. News 2, 150–168. Available at: http://www.worldscientificnews.com/wp-content/uploads/2017/07/WSN-812-2017-150-168.pdf (Accessed May 20, 2023).
Uhrig, J. F. (2006). Protein interaction networks in plants. Planta 2006 2244 (224), 771–781. doi:10.1007/s00425-006-0260-x
Umezawa, T., Sugiyama, N., Mizoguchi, M., Hayashi, S., Myouga, F., Yamaguchi-Shinozaki, K., et al. (2009). Type 2C protein phosphatases directly regulate abscisic acid-activated protein kinases in Arabidopsis. Proc. Natl. Acad. Sci. U. S. A 106, 17588–17593. doi:10.1073/pnas.0907095106
Underwood, W. (2012). The plant cell wall: A dynamic barrier against pathogen invasion. Plant Sci. 3, 85. doi:10.3389/fpls.2012.00085
Van den Broeck, L., Dubois, M., Vermeersch, M., Storme, V., Matsui, M., and Inzé, D. (2017). From network to phenotype: The dynamic wiring of an arabidopsis transcriptional network induced by osmotic stress. Mol. Syst. Biol. 13, 961. doi:10.15252/msb.20177840
Vasudevan, D., Fu, A., Luan, S., and Swaminathan, K. (2012). Crystal structure of Arabidopsis cyclophilin38 reveals a previously uncharacterized immunophilin fold and a possible auto inhibitory mechanism. Plant Cell 24, 2666–2674. doi:10.1105/tpc.111.093781
Vojta, L., Paić, A. T., Horvat, L., Rac, A., Lepeduš, H., and Fulgosi, H. (2019). Complex lumenal immunophilin AtCYP38 influences thylakoid remodelling in Arabidopsis thaliana. J. plant physiology 243, 153048. doi:10.1016/j.jplph.2019.153048
Wang, L., Mu, X., Chen, X., and Han, Y. (2022). Hydrogen sulfide attenuates intracellular oxidative stress via repressing glycolate oxidase activities in Arabidopsis thaliana. BMC Plant Biol. 221 (22), 1–12. doi:10.1186/S12870-022-03490-3
Wang, X., and Jin, Y. (2017). Predicted networks of protein-protein interactions in Stegodyphus mimosarum by cross-species comparisons. BMC Genomics 18, 1. doi:10.1186/S12864-017-4085-8
Wu, T., Hu, E., Xu, S., Chen, M., Guo, P., Dai, Z., et al. (2021). clusterProfiler 4.0: A universal enrichment tool for interpreting omics data. Innov 2, 100141. doi:10.1016/j.xinn.2021.100141
Xue, T., Wang, D., Zhang, S., Ehlting, J., Ni, F., Jakab, S., et al. (2008). Genome-wide and expression analysis of protein phosphatase 2C in rice and. Arab. BMC genomics 9, 1–21. doi:10.1186/1471-2164-9-550
Yang, S., Li, H., He, H., Zhou, Y., and Zhang, Z. (2019). Critical assessment and performance improvement of plant–pathogen protein–protein interaction prediction methods. Brief. Bioinform 20, 274–287. doi:10.1093/bib/bbx123
Yeger-Lotem, E., and Sharan, R. (2015). Human protein interaction networks across tissues and diseases. Front. Genet. 6, 257. doi:10.3389/fgene.2015.00257
Yin, H., Guo, H. B., Weston, D. J., Borland, A. M., Ranjan, P., Abraham, P. E., et al. (2018). Diel rewiring and positive selection of ancient plant proteins enabled evolution of CAM photosynthesis in Agave. BMC Genomics 19, 588–616. doi:10.1186/s12864-018-4964-7
Yonekura-Sakakibara, K., Higashi, Y., and Nakabayashi, R. (2019). The origin and evolution of plant flavonoid metabolism. Front. plant sci. 10, 943. doi:10.3389/fpls.2019.00943
Yu, B., Liu, J., Wu, D., Liu, Y., Cen, W., Wang, S., et al. (2020). Weighted gene coexpression network analysis-based identification of key modules and hub genes associated with drought sensitivity in rice. BMC plant Biol. 20, 478–521. doi:10.1186/s12870-020-02705-9
Yu, J., Ma, X., Wang, L., Dong, N., Wang, K., You, Q., et al. (2022). Arabidopsis CAP1 mediates ammonium-regulated root hair growth by influencing vesicle trafficking and the cytoskeletal arrangement in root hair cells. J. Genet. Genomics 49 (10), 986–989. doi:10.1016/j.jgg.2022.02.005
Zahedi, S. M., Hosseini, M. S., Daneshvar Hakimi Meybodi, N., and Peijnenburg, W. (2021). Mitigation of the effect of drought on growth and yield of pomegranates by foliar spraying of different sizes of selenium nanoparticles. J. Sci. Food Agricul. 101, 5202–5213. doi:10.1002/jsfa.11167
Zahn-Zabal, M., Dessimoz, C., and Glover, N. M. (2020). Identifying orthologs with oma: A primer. F1000Research 9, 1. doi:10.12688/F1000RESEARCH
Zahra, N., Hafeez, M. B., Shaukat, K., Wahid, A., Hussain, S., Naseer, R., et al. (2021). Hypoxia and anoxia stress: Plant responses and tolerance mechanisms. J. Agron. Crop Sci. 207, 249–284. doi:10.1111/jac.12471
Zeng, H., Wu, B., Zhang, M., Zhang, N., Elnashar, A., Zhu, L., et al. (2021). Dryland ecosystem dynamic change and its drivers in Mediterranean region. Curr. Opin. Environ. Sustain. 48, 59–67. doi:10.1016/j.cosust.2020.10.013
Zhao, H., Lou, Y., Sun, H., Li, L., Wang, L., Dong, L., et al. (2016). Transcriptome and comparative gene expression analysis of Phyllostachys edulis in response to high light. BMC Plant Biol. 16, 34–17. doi:10.1186/s12870-016-0720-9
Zhao, Y., Kong, H., Guo, Y., and Zou, Z. (2020). Light-harvesting chlorophyll a/b-binding protein-coding genes in jatropha and the comparison with castor, cassava and arabidopsis. PeerJ 2020, e8465. doi:10.7717/peerj.8465
Zheng, X. T., Yu, Z. C., Tang, J. W., Cai, M. L., Chen, Y. L., Yang, C. W., et al. (2020). The major photoprotective role of anthocyanins in leaves of Arabidopsis thaliana under long-term high light treatment: Antioxidant or light attenuator? Photosynth. Res. 2020 1491 (149), 25–40. doi:10.1007/s11120-020-00761-8
Zhong, R., and Ye, Z. H. (2015). Secondary cell walls: biosynthesis, patterned deposition and transcriptional regulation. Plant and cell physiology 56 (2), 195–214. doi:10.1093/pcp/pcu140
Keywords: agave, interactome, co-expression analysis, protein-protein interaction network, sisal
Citation: Carvalho LM, Silva NV, de Abreu LGF, Marone MP, Cardelli AR, Raya FT, Araújo G, Carazzolle MF and Guimarães Pereira GA (2023) Analysis of protein-protein interaction and weighted co-expression networks revealed key modules and genes in multiple organs of Agave sisalana. Front. Chem. Eng. 5:1175235. doi: 10.3389/fceng.2023.1175235
Received: 27 February 2023; Accepted: 09 May 2023;
Published: 26 May 2023.
Edited by:
Hugo Oscar Méndez-Acosta, University of Guadalajara, MexicoReviewed by:
María Esther Macías-Rodríguez, University of Guadalajara, MexicoNahomy Marino, CIATEJ, A. C., Mexico
Copyright © 2023 Carvalho, Silva, de Abreu, Marone, Cardelli, Raya, Araújo, Carazzolle and Guimarães Pereira. This is an open-access article distributed under the terms of the Creative Commons Attribution License (CC BY). The use, distribution or reproduction in other forums is permitted, provided the original author(s) and the copyright owner(s) are credited and that the original publication in this journal is cited, in accordance with accepted academic practice. No use, distribution or reproduction is permitted which does not comply with these terms.
*Correspondence: Gonçalo Amarante Guimarães Pereira, Z29uY2Fsb0B1bmljYW1wLmJy