- 1Department of Biosystems, Science and Engineering, ETH Zürich, Basel, Switzerland
- 2Swiss Institute of Bioinformatics, Basel, Switzerland
Organoids are self-organized three-dimensional (3D) multicellular tissue cultures which derive from cancerous and healthy stem cells, sharing a highly similarity to the corresponding in vivo organs. Since their introduction in 2009, they have emerged as a valuable model for studying early embryogenesis, organ and tissue development, as well as tools in drug screening, disease modeling and personalized therapy. Organoids can now be established for various tissues, including brain, retina, thyroid, gastrointestinal, lung, liver, pancreas, and kidney. These micro-tissues resemble the native organ in terms of gene expression, protein expression, tissue architecture and cell-cell interactions. Despite the success of organoid-based research and the advances in patient-derived organoid culture, important challenges remain. In this review, we briefly showcase the evolution from the primary 3D systems to complex, multilayered 3D structures such as assembloids, gastruloids and ETiX embryoids. We discuss current developments in organoid research and highlight developments in organoid culturing systems and analysis tools which make organoids accessible for high-throughput and high-content screening. Finally, we summarize the potential of machine learning and computational modeling in conjunction with organoid systems.
1 Introduction
Cell culture has been a powerful in vitro tool to study the mechanisms by which cells assemble and function into tissues and organs, while also understanding mechanisms of diseases and drug function. Although two-dimensional (2D) cell culture is one of the major techniques through which researchers have elucidated a variety of cellular functions, there are critical limitations. In adherent 2D cultures, cells grow as a monolayer attached to a plastic surface (Breslin and O’Driscoll, 2013); this culture method has numerous disadvantages. The most profound disadvantages are lack of interactions between cellular and extracellular environment, structures showing changed morphology compared to the natural tissue structures, and loss of epithelial polarity. Furthermore, cells grown in monolayer have unlimited access to the ingredients of the medium, e.g., nutrients, metabolites and signal molecules, which does not resemble the physiological condition, likely resulting in unnatural gene responses and cell biochemistry (Kapałczyńska et al., 2018), thus failing to mimic cellular functions and signaling pathways present in tissues. Due to the many limitations of the 2D culture system, there was a need for a better model to resemble native organs.
Three-dimensional (3D) cell culture, unlike 2D, provides an environment where cells can interact in all three dimensions. One such 3D cell culture technique is organoid culture. In classic developmental biology the term organoid first appeared in 1963 (Schneider et al., 1963). Ten years later, the first long-term culture of untransformed human cells was reported, initiating the field of organoids (Rheinwatd and Green, 1975). In 1987, using hydrogel, recapitulation of a pathological state in primary mammary epithelial cells was achieved, highlighting the importance of extracellular matrix in 3D cell culture (Li et al., 1987). The organoid field moved forward slowly until 2009, when the laboratory of Hans Clevers seeded single intestinal stem cells in Matrigel, which is nowadays the most commonly used extracellular matrix substitute, and essential niche factors, resulting in stem cells proliferating and forming complex 3D organized structures (Sato et al., 2009). Those complex 3D organized structures, which contained stem cells but also various differentiated cell types, while also resembling the architecture of the original tissue, are considered to be the first “modern-era” organoids (Clevers, 2016; Fatehullah et al., 2016).
Currently there are two major avenues for generating organoids. One way that organoids can be established is from embryonic stem cells, or induced pluripotent stem cells, leveraging on their intrinsic ability to self-organize and form 3D structures which resemble tissues in vivo. Because organoids come from active stem cell populations, researchers can expand their cultures repeatedly over time. Specific growth factors, proteins and extracellular matrix will direct the differentiation of the stem cells and their morphogenetic processes in order to form the type of organoid needed. Organoids deriving from embryonic stem cells or induced pluripotent stem cells are great models for organs where tissue is very difficult to be obtained e.g., brain or tissues which do not contain easily culturable stem cells (Hautefort et al., 2022). Those organoids bear a great degree of resemblance, both structural and functional, to the adult organ. Furthermore, PSC-derived organoids usually include more than one tissue type and neighboring cells to the tissue of interest, allowing intercellular interactions to be studied in vitro (Spence et al., 2011) The other method of establishing organoids is from organ-specific adult stem cells (ASCs), which can be defined as undifferentiated cells naturally capable of self-regenerating asymmetrically. They renew themselves and produce progenitor cells that will proliferate and differentiate into all of the functional cell types normally residing in the tissue from which they derive (Middendorp et al., 2014). In order to establish organoids from ASCs, a tissue biopsy is minced in several pieces using mechanical force. Incubation with enzymes such as collagenase and elastase will generate single cell suspension. Once single cell culture is established, the cells will be seeded in extracellular matrix, growth medium and tissue growth factors and will form organoids containing most of the different cell types normally present in the tissue of origin (Dutta et al., 2017) ASC-derived organoids can be expanded almost indefinitely, making them an incredibly valuable source of untransformed primary cells (Hofer and Lutolf, 2021).
Since the generation of the landmark intestinal organoids numerous types of organoids have been established, including but not limited to, brain (Lancaster et al., 2013), intestine (Spence et al., 2011), kidney (Takasato et al., 2014; Przepiorski et al., 2018), liver (Takebe et al., 2013), lung (Miller et al., 2019), pancreas (Huch et al., 2013) and retina (Hua et al., 2020) organoids. Their popularity has increased due to the availability of culturing protocols and their potential in drug screening and regenerative medicine.
2 Disease modeling using organoids
Organoids combined with gene editing technology have the potential to become a major weapon against cancer and numerous other diseases. So far, there are protocols available for deriving organoids from primary colon (van de Wetering et al., 2015), esophagus (Sato et al., 2011), bladder (Vasyutin et al., 2019), pancreas (Boj et al., 2015), stomach (Bartfeld et al., 2015), liver (Broutier et al., 2017), endometrium (Turco et al., 2017) cancer tissues, as well as from metastatic colon (Weeber et al., 2015), prostate (Gao et al., 2014; Drost et al., 2016) and breast (Sachs et al., 2018) cancer biopsy samples. Those organoids faithfully recapitulate characteristics of the parent tumor, mimicking key histopathological, genetic and phenotypic features of it. Cancer organoids have been used for patient-specific testing of clinical and emerging anticancer treatments as well as in cancer progression studies (Nanki et al., 2018; Seino et al., 2018). Large collections of tumor and matching healthy organoids are generated and biobanked, giving useful insights into cancer progression. Colorectal cancer (CRC), Pancreatic ductal adenocarcinoma (PDAC) and breast cancer biobanks have already been established. Recently a tumor organoid biobank deriving from patients with CRC was generated and consists of a set of 20 genetically diverse tumor organoid cultures and their matching normal tissue-derived organoids (van de Wetering et al., 2015). Tiriac et al. established pancreatic cancer organoids from a genetically and phenotypically comprehensive cohort of 138 patient tumor samples (Tiriac et al., 2018). Those studies allowed researchers to identify population-level genetic and transcriptomic signatures on anticancer drug responses which correlated with the clinical outcome of the patients. Increasing the number of biobanked organoids will allow more accurate correlations between genetic markers and drug responses. Interestingly, when protein profiles of tumor organoids and matching healthy organoids were investigated, they showed distinct differences in their profiles (Cristobal et al., 2017). This finding signifies the importance of personalized proteomic profiling in cancer research. In addition, patient-derived organoids are not able to grow in a “universal” medium, highlighting the diversity in genetic composition of cancer tissues, even of the same type. Furthermore, in a publication from Fujii et al., it was shown that xenotransplantation of kidney organoids in immunodeficient mice, was enough to introduce histopathologically identical tumors in those mice (Fujii et al., 2016). Organoid transplantations hold great potential in validating drug responses in an in vivo environment.
Organoid cancer models do not only derive from cancer cells but can also be generated from wild-type embryonic stem cells (ESCs) or from induced pluripotent stem cells (iPSCs) (Figure 1), which accounts for another major advantage of organoid technology, allowing screening for compounds which specifically target tumor cells while leaving wild type cells intact. Targeting oncogenes with gene engineering methods, such as Crispr-Cas9 or shRNA, researchers can produce cancer organoids in a dish. Double K.O of Cdh1 and Tp53 in stomach organoids enables the organoids to demonstrate invasive phenotypes in vitro and robust metastasis in vivo (Li et al., 2014). Modeling of colorectal carcinoma has been achieved using organoids which lack Apc, p53, Kras and Smad4 (Li et al., 2014). In the reverse experiment, expression of mutated KRAS and/or TP53 in pancreas organoids resulted in neoplastic transformation in culture and in vivo (Boj et al., 2015). Furthermore, two independent studies have achieved to model the “adeno-carcinoma sequence”, a stepwise activation pattern of oncogenes, with simultaneous inactivation of tumor suppressor genes, introduced in human colon organoids (Drost et al., 2015; Matano et al., 2015). In addition, Crispr-Cas9 was utilized to introduce combinations of CRC driver mutations to generate accurate CRC progression models. Surprisingly, those models showed that growth happens independently from intestinal stem cell niche factors like EGF, WNT, R-spondin and Noggin (Matano et al., 2015). Organoid technology can also be utilized to study cancer-related processes and signaling pathways. Using intestinal organoids, it was shown that mutations in RHOA, a gene involved in gastric cancer, were able to induce resistance to commonly used anticancer drugs (Wang K. et al., 2014). Finally, human colon organoids were used to show that CDX2 loss and BRAF mutations are the driving force in serrated colon carcinoma (Sakamoto et al., 2017). Although cancer organoids hold immense potential as models of patient-specific cancer biology, there are still limitations to be considered. Eliminating the variability which accompanies cancer organoid culture is of vital importance in order to establish reproducible platforms which will speed up the insights into patient care and better clinical outcome. Unfortunately, for many cancers the efficiency of organoid formation and in vitro expansion is as low as 5% (Neal et al., 2018; Dijkstra et al., 2020). In addition, cancer cell interactions with the extracellular matrix (ECM) are very difficult to recapitulate in 3D cultures while the current techniques are not able to accurately represent the physiological condition and the interactions with the ECM. Finally, non-standardized cancer tissue sources, their downstream processing, ill-defined and non-specific medium formulations cause variations in organoid generation which are precluding their clinical implementation (LeSavage et al., 2022).
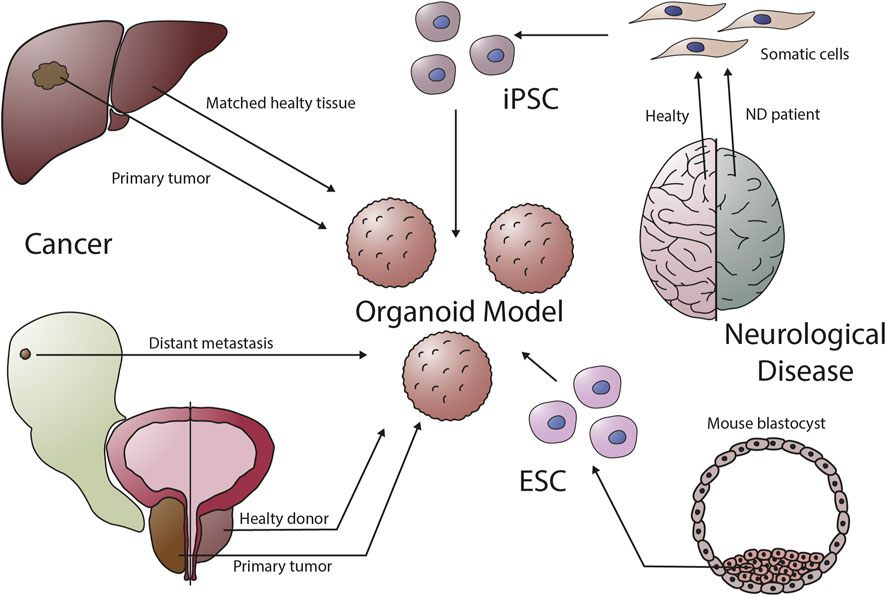
FIGURE 1. Schematic overview of organoid origin. Organoids can be established from various healthy and cancer-derived organs. ESCs isolated from mouse blastocysts are commonly used to generate organoids. iPSCs from patient derived healthy and disease tissue samples are also able to establish organoids.
In this section we primarily focused on organoids and their usage in cancer research. This is not the only disease which leverages on the usage of organoids. In this paragraph we are pointing out disease research which is profiting from the 3D organoid cultures, to highlight organoid’s almost infinite potential in disease modeling. Infectious disease research holds a plethora of organoids as models to understand the underlying disease mechanisms. Brain organoids have been used as models for studying Human Immunodeficiency virus, Herpes Simplex virus, Prion, Zika virus and SARS-CoV-2 (Garcez et al., 2016; Groveman et al., 2019; Jacob et al., 2020; Pellegrini et al., 2020). Of note, skin organoids (Ma et al., 2022), as well as cardiac organoids (Mills et al., 2021), have been used lately to study SARS-CoV-2 as well. Liver organoids have been exploited in research focusing on Hepatitis B, C and Malaria (Baktash et al., 2018; Nie et al., 2018; Arez et al., 2019). Furthermore, reproductive track organoids were utilized to study human papillomavirus (Lõhmussaar et al., 2021). In addition, intestinal organoids are used for studying Human Noroviruses, Salmonella and Vibrio cholera (Yin et al., 2015; Heo et al., 2018) Similarly, other human organoid disease models include cerebral organoids (Lancaster et al., 2013) as models to study Autism and Schizophrenia, optic cup organoids to study glaucoma (Eiraku et al., 2011), inner ear organoids for modeling congenital and early-onset hearing loss (Koehler et al., 2013), as well as skin organoids for systemic sclerosis and inflammatory skin diseases (Kim et al., 2018; Elias et al., 2019). Finally, heart organoids are a useful model to study Barth syndrome, Dilated cardiomyopathy, acute myocardial infarction and Duchenne muscular dystrophy (Wang G. et al., 2014; Hinson et al., 2015; Cashman et al., 2016), while lung organoids model benefit Idiopathic pulmonary fibrosis and Cryptosporidium (Strikoudis et al., 2019; Surolia et al., 2019) research. This small overview pinpoints the diversity of diseases that can be studied in vitro using organoid models.
3 Evolution of organoids–gastruloids and assembloids
Apart from organoids, other more complex 3D models have recently emerged and are gaining a lot of popularity. Treatment of 3D stem cell aggregates with Wnt leads to symmetry break followed by polarized growth with regard to three orthogonal axes (Marikawa et al., 2009; Baillie-Johnson et al., 2015). This process forms elongated 3D structures, the so-called “gastruloids” (van den Brink et al., 2014). Gastruloids display distinct axial organization, clearly defined anterior-posterior, left-right, and dorsal-ventral body axes, and well-organized gene expression domains while also comprising post occipital embryo derivatives of all three germ layers, -endoderm, mesoderm, ectoderm- (Veenvliet et al., 2020). Gastruloids recapitulate key aspects of gastrula-stage embryos and are a powerful tool for studying post-implantation development. Lately, more advanced gastruloid models have been developed that are able to generate brain (Girgin et al., 2021), somite (van den Brink et al., 2020), neural tube (Veenvliet et al., 2020), gut tube and beating heart-like structures (Rossi et al., 2021). Gastruloids can be generated in large numbers, allowing them to be easily used in screens, thus having great potential in clinical applications.
The latest and most complete 3D model that resembles mouse embryos is ETiX-embryoids (Amadei et al., 2022; Lau et al., 2022). ETiX-embryoids are stem cell-based structures which accurately recapitulate post-implantation morphogenesis. ETiX-embryoids form by aggregating trophoblast stem cells, extraembryonic endoderm stem cells and inducible XEN cells without the need for additional external signalling cues (Harrison et al., 2017; Sozen et al., 2018; Girgin et al., 2021). ETiX-embryoids are able to complete gastrulation after 6 days in culture. On day 8 they are forming headfolds with defined forebrain and midbrain regions, a beating heart-like structure, a trunk comprising a neural tube and somites, a tail bud and a gut tube. This almost complete embryo model is developing inside extraembryonic membranes equivalent to amnion and yolk sac. ETiX embryoids offer a powerful and physiologically relevant model of post-implantation embryogenesis in development and disease (Marton and Pașca, 2020).
Assembloids also provide an excellent model for cancer research. For example, spheroids derived from glioblastoma multiforme cancer cells co-cultured with cortical organoids accurately recapitulate the invasion of cancer cells into healthy tissue (da Silva et al., 2018). Furthermore, In recent years, it has become evident that the interaction of tumors with the local cellular and extracellular environment can have a major influence on progression and treatment outcomes (Sun, 2016; Anderson and Simon, 2020). Classical organoid systems, as we have highlighted before, lack a microenvironment that recapitulates these conditions in vitro. In bladder cancer, studies have shown that luminal-cancer-derived organoids shift to a basal subtype in the absence of a stroma (Lee et al., 2018) and therefore diverge from the primary tumor composition. This shift to a basal-like subtype might influence the responsiveness to chemotherapeutic drugs and potentially hamper the outcome of drug screening studies. Kim et al. developed mouse and human bladder assembloids consisting of an inner, multilayered bladder epithelium (urothelium), a stroma, and an outer shell of muscle fibers. In their work, they showed that 7-day-old assembloids display a striking similarity to normal bladder tissue. They also demonstrated that assembloids generated from urothelial carcinomas with matched cancer-associated fibroblasts (CAFs) maintain their luminal subtype, showcasing the importance of the cancer-associated environment (Kim E. et al., 2020). A more extensive review focusing on the current state of assembloids has recently been published by Kanton and Pasca (Kanton and Paşca, 2022).
4 High throughput screening using organoids
The enhanced complexity of organoids has proven to be useful for studying more intricate relationships and processes. However, one of the expectations for organoids was that they might lessen or replace animal models in drug screening. Drug screening assays often rely on high-throughput screening (HTS) approaches to gain meaningful insight within a reasonable amount of time. To highlight the power of organoids in HTS cancer research one can focus on the publication from Vlachogiannis et al. The group generated patient-derived organoid models of metastatic gastrointestinal cancer and used them to predict patient treatment responses (Vlachogiannis et al., 2018). Using a compound library of drugs, they tested organoid sensitivities versus patient responses. The positive predictive value was reported to be 88%, while the negative predictive value was reported to reach an impressive 100%, profoundly demonstrating the ability of organoids to be used for personalized medicine programmes.
To be suitable for HTS, cellular assay protocols must be simple enough so that they can be compatible with liquid-handling robots. Furthermore, production efficacy and inter-organoid homogeneity must be high in order to produce meaningful results (Figure 2). As aforementioned, in many organoid protocols, the organoids are commonly embedded in a drop of Matrigel and grow at a random position within the embedding medium. This makes fast single-plane imaging difficult as the focal plane for each organoid is different. Additionally, compounds of interest in drug screening might interact with the matrix in a difficult-to-predict fashion (Gunasekara et al., 2018). One pillar in the development of HTS organoid assays is the optimization of these culturing methods. For example, Gunasekara et al. introduced a culturing system that can overcome some of the limitations imposed by ECM embedding. Instead of generating drops with embedded organoids, they coated microwell plates with matrigel and then let organoids grow on top of these hydrogels. This setup allowed them to scan organoids using an automated focus scan and to either monitor growth over a time period of 3 days or swelling due to compound exposure over a time period of over 1 h. Although this system allows fast image scanning, it still relies on many manual steps during organoid preparation (Gunasekara et al., 2018).
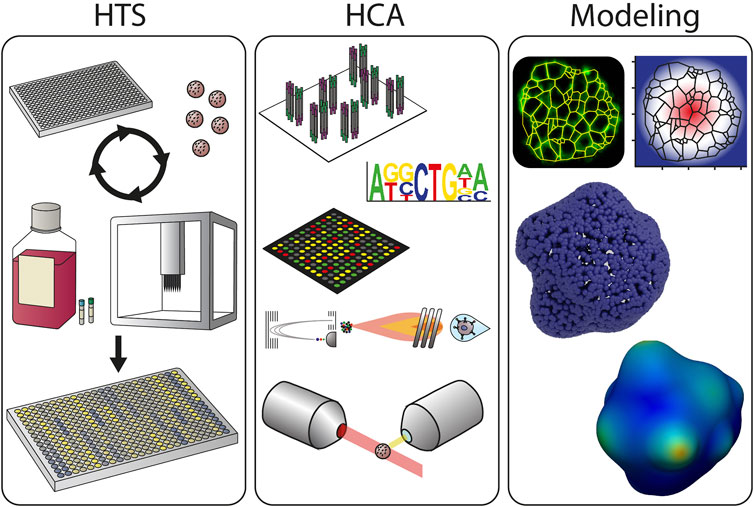
FIGURE 2. Schematic overview of HTS & HCA workflow. HTS relies upon on simple culturing protocols, fully compatible with high count microwell platforms and liquid handling automatons. HTS experimental design allows fast readout and automated data processing. HCA is suitable for obtaining a comprehensive understanding of tissue and cell mechanic, utilizing (single cell) omics and high-resolution imaging tools. Computational models are used to examine mechanobiological processes controlling organoid growth, patterning or for validating results from organoid studies. Measurements and features derived from organoid culture are structuring those models.
For drug screening in 2D cell cultures, 384 or 1,536 well plates are commonly used, which reduces the amount of reagent used per well/assay while allowing testing of a wider range of drugs or drug concentrations within one single plate (An and Tolliday, 2010). This is why these plate formats are also preferred in organoid HTS. Owing to the small dimensions of the wells in these plates, manual handling can be cumbersome and slow. These plate formats are, therefore, more commonly used in combination with liquid handling robots. Complex organoid protocols are not well suited for automatisation using liquid handling robots. In the past 5 years, several microplate-compatible organoid protocols have been developed, for example, for breast cancer (Sachs et al., 2018), kidney organoids (Czerniecki et al., 2018), pancreas (Hou et al., 2018) or colon organoids (Du et al., 2020).
A different approach to make organoid culturing more practical for HTS is the engineering of alternatives to traditional cell culture dishes. An early example of such an alternative, developed by Gracz et al., is a polydimethylsiloxane (PDMS)/polystyrene-based microraft array (MRA). These arrays allowed them to study stem cell niche interaction between intestinal stem cells (ISC) and paneth cells both through imaging over extended time periods and endpoint gene expression analysis on a high-throughput scale (Gracz et al., 2015). Another example of a micro-engineered organoid culturing system, suitable for HTS, is the hydrogel-based microcavities system developed by Brandenberg and colleagues. Using PDMS stamps, they create U-shaped microwells in a poly (ethylene glycol) (PEG) substrate. The size and shape of these wells are such that the cavities act not only as stable traps for the organoids but also improve intra-organoids homogeneity and reduce the amount of Matrigel required (Brandenberg et al., 2020).
Improvement of the culturing conditions is not the only challenge that can be addressed using microengineering. The recently developed JeWell (Beghin et al., 2022) microwell array is an outstanding example of an organoid culturing system that improves the accessibility of organoid systems for fast imaging. On the JeWell chip, each microcavity is flanked with mirrors at a 45° angle. This enables single-objective SPIM imaging of hundreds of organoids within a short amount of time, thus not only increasing the speed but also the information depth that can be obtained from organoids.
HTS methods are currently focusing on simpler organoid systems because higher-order organoids, such as assembloids or gastruloids, often rely on even more elaborate culturing systems. However, there are some examples of how such elaborate systems could be made compatible with HTS. For instance, Mantizou et al. showed that gastruloids could be used for teratogenicity testing in HTS (Mantziou et al., 2021), while Kim et al. established a 3D-bioprinting method for their bladder cancer assembloids which is compatible with a high-throughput approach (Kim E. et al., 2020). The approach developed by Zhu et al. is one of the most recent examples of how to make higher-order organoids systems feasible for HTS. As part of their protocol, they use electrospray-generated microcapsules to pre-grew region-specific brain organoids. These organoids were then permitted to fuse into brain assembloids on a microfluidics chip (Zhu et al., 2023). This strategy makes it possible to produce brain assembloids at a scale that is compatible with HTS and shows once again how microengineering techniques can enhance the integrability of organoid systems to HTS.
5 Endpoint measurements and image-based profiling in HTS
Evidently, scaling up the number of organoids that may be handled within a single experiment is only one side of the coin. Measurable parameters and how they are interpreted are ultimately of main interest. Two of the most fundamental readout parameters are viability and metabolic activity (Czerniecki et al., 2018; Hou et al., 2018; Phan et al., 2019; Du et al., 2020); however, information gained from such measurements is very limited. To increase information depth, these measurements can also be combined with enzyme-linked immunosorbent assays (ELISA) for quantifying biomarkers such as the Kidney injury molecule-1 (KIM-1) (Czerniecki et al., 2018). These types of measurements can be evaluated utilizing assays that are available as kits on the market, many of which are compatible with HTS.
Furthermore, such measurements can be combined with (live-)imaging approaches. The simplest readouts for image-based HTS are alterations in size or shape, either compared to a control group and/or monitored over time and hereafter referred to as image-based profiling (IBP). Datasets for basic IBP can be acquired using epifluorescence and confocal microscopes (Gracz et al., 2015; Czerniecki et al., 2018; Gunasekara et al., 2018; Brandenberg et al., 2020). However, manual evaluation of every single organoid in an HTS essay is not feasible. Therefore, automated pipelines for IBP are needed for the unbiased and fast interpretation of large arrays of imaging data. Such pipelines are capable of performing basic operations, such as foreground and background separation, edge detection and feature extraction, and are often specifically tailored and optimized for the HTS systems they were developed for (Walsh et al., 2014; Gracz et al., 2015; Gunasekara et al., 2018; Brandenberg et al., 2020). Other approaches utilize machine learning to incorporate 3D information for fast phenotypic screening (Kassis et al., 2019). More recently, machine-learning techniques have also been applied in high-content screens, which will be discussed hereafter.
6 High content analysis using omics tools
The biological complexity of organoids that improves fidelity in HTS can also be exploited to study processes and interactions on cellular levels in a more physiological environment using high content analysis (HCA). In HTS the goal is to screen high numbers of organoids within a short amount of time by measuring easily accessible and interpretable markers. In contrast, the focus in HCA is to dissect developmental processes, tissue homeostasis and alteration thereof during disease onset and progression. In order to understand the intricate interactions within cellular networks in organoids, multidimensional datasets are derived utilizing omics and/or multidimensional imaging technologies (Figure 2).
In omic-based approaches, datasets can be obtained either on a population level (organoid population or cell populations from a single organoid) or on a single cell level. Population-based methods are typically less costly, easier to perform and less noisy than single-cell approaches. Therefore they are the method of choice when cellular heterogeneity is not the focus of interest. Whole genome sequencing (WGS), for example, is applied to understand the mutational landscape of organoids and to compare them to their tissue of origin at the genome level (Behjati et al., 2014; Roerink et al., 2018; Kopper et al., 2019). ChIP-seq, ATAC-seq, and methylation arrays can give insights into the epigenome based on chromatin-protein interactions and DNA accessibility, for example, during developmental processes, or to assess epigenetic changes in cancers (Kanton et al., 2019; Kopper et al., 2019). Bulk RNA profiles of organoids are used to gauge differences in gene expression levels in organoids from healthy tissue (Middendorp et al., 2014), cancer (Boj et al., 2015; Roerink et al., 2018; Kopper et al., 2019) or upon environmental changes (Gjorevski et al., 2016). Finally, mass spectrometry (MS) based methods can be employed to get a better understanding of the proteomic landscape (Boj et al., 2015; Gonneaud et al., 2016; Williams et al., 2016; Cristobal et al., 2017) and the metabolome of organoids (Lindeboom et al., 2018). All these methods can be combined to systematically dissect regulatory mechanisms which predestined cell faith, as demonstrated by Lindenboom et al. in their multi-omics study (Lindeboom et al., 2018).
Furthermore, for almost all of these methods, a single cell (sc) equivalent exists nowadays. With the help of these techniques, tissues can be studied at the cellular level to determine their molecular makeup. In Organoids scWGS has, for example, been used to show that the genetic feature of organoids derived from ovarian cancer mirror the original tumor biopsy and maintain their tumor heterogeneity (Kopper et al., 2019). Likewise, scWGS was applied to monitor the genomic heterogeneity and stability of pancreatic cancer organoids (Usman et al., 2022) or investigate intra-tumor heterogeneity and tumor evolution in colorectal cancer organoids (Kester et al., 2022).
Whereas scWGS is useful to investigate the genomic heterogeneity in organoids, scRNA-sequencing can be applied to study cell status and differentiation based on gene expression profiles. This form of benchmarking is particularly important because it has been documented that organoids generated from tumors might not always retain the characteristics of their parental tumors. This has been demonstrated for bladder cancer organoids, which tend to shift from a luminal to a basal subtype in culture (Lee et al., 2018). Such pheromones have major implications for drug screening and personalized medicine, for instance, since tumor composition can affect treatment outcomes.
Wang et al., compared the gene expression signatures of patient-derived colorectal cancer organoids and matched normal organoids to the gene expression signature of the primary tissue. In their study, they were able to show that the tumor organoids retain the gene expression signature of the corresponding tumor, while on the other hand, the organoids derived from normal tissue adopt some tumor-like features (Wang et al., 2022).
Organoids can also be used in conjunction with scRNA-sequencing to study cell populations with low abundance. Employing scRNA sequencing and intestinal organoids, as a mean to obtain a random mixture of intestinal cells free of non-epithelial cells, Grün et al. discovered a rare intestinal cell type in these organoids, and subsequently confirmed the existence of these cell types in cell isolates from mouse intestines (Grün et al., 2015). Similarly, Czernieck et al. explored the maturation heterogeneity of kidney organoids in their HTS platform using scRNA-sequencing and found previously undetected cell compartments in their organoid model (Czerniecki et al., 2018).
In the context of developmental biology, Camp et al. performed scRNA-sequencing on cerebral organoids and were able to show that the gene expression pattern in the cortex-like regions of the organoids is similar to the fetal cerebral cortex (Camp et al., 2015), while Kanton et al. combined scRNA-seq and scATAC-seq to show how chromatin accessibility and gene expression differ between human and chimpanzee cerebral organoid development (Kanton et al., 2019). Similarly, Sridhar et al. compared the cellular composition and gene expression patterns between the human fetal retina and retinal organoids and detailed similarities and differences between the two (Sridhar et al., 2020).
In addition, for scProteomics CyTOF has recently been used to demonstrate that the protein expression pattern in organoid cultures from normal breast tissue is also conserved (Rosenbluth et al., 2020). Furthermore, CyTOF can also be used to investigate cell-specific post-translational modifications on proteins, as demonstrated by Qin and colleagues (Qin et al., 2020). However, many of the above-mentioned works use a mix of different bulk and or sc approaches and demonstrate that a smart combination of culturing methods and sc- and bulk-omic methodologies opens new ways for exploring organoid biology.
7 Imaging and machine learning for HCA
Imaging approaches have played an integral part in the world of organoids. As previously discussed, imaging can be used for feature extraction in HTS. Furthermore, immunohistochemical stainings are commonly used to characterize organoids and validate findings from omics-based HCA screens. Imaging methods are constrained by the number of possible fluorophore combinations. Nevertheless, imaging approaches can be considered HCA, because they allow the studying of biomarkers in a spatiotemporal context within their cellular environment. High-resolution microscopes such as confocal or light sheet microscopes can be utilized to extract 2D and 3D information of immunofluorescent (IF) labeled organoids (Dekkers et al., 2019). Alternatively, approaches like optical metabolic imaging allow for label-free imaging (Walsh et al., 2014), and smFISH can give insights into the spatial expression pattern of RNA molecules in organoids (Omerzu et al., 2019; Borrelli and Moor, 2020). As an alternative to quantifying soluble biomarkers using ELISA McGhee et al. developed an image-based assay to study these biomarkers in situ. The approach is based on immunosorbent beads embedded in matrigel, which allows for spatiotemporal quantification of these biomarkers (McGhee et al., 2022). Furthermore, organoids are accessible for genetic modifications. Stable integration of fluorescently labeled proteins or membrane markers opens the field to 4D imaging and allows, for example, to monitor apoptotic or mitotic events over the time course of several days (Verissimo et al., 2016). Of note, light sheet microscopy has become an important tool in live imaging of organoids, as it allows for fast z-scanning with low phototoxicity over extended periods of time. These properties make light sheet HCA imaging also suitable for HTS (Beghin et al., 2022; de Medeiros et al., 2022). However, the analysis of such multidimensional datasets can be challenging and time-consuming.
Many groups have used machine learning methods to analyze the multidimensional datasets created from organoids. PCA has been utilized to cluster organoids based on their phenotypic profiles (Booij et al., 2017), while random forest classification has been applied to predict the disease state in patient-derived midbrain organoids from patients with Parkinson’s disease (Monzel et al., 2020). Machine learning cannot only be applied to imaging data but can also be used to predict drug responses based on pharmacogenomic data from organoid models (Kong et al., 2020). Recently, deep learning techniques have been increasingly utilized to analyze organoid systems. For instance, OrgaQuant was developed in order to automatically locate and quantify the size of organoids in brightfield images (Kassis et al., 2019), while OrganoidTracker is a convolutional neural network (CNN)-based tool to track nuclei in 4D data sets (Kok et al., 2020). Likewise, Medeiros and Beghin used CNN for data processing in their HTS-HCA organoid platforms (Beghin et al., 2022; de Medeiros et al., 2022). To make machine learning for organoid imaging data more accessible to researchers with limited programming expertise, Gritti et al. developed a machine learning tool called MOrgAna. This software automates image segmentation, quantification and visualization of morphological and fluorescence information of organoids across hundreds of images through a user-friendly interface (Gritti et al., 2021).
8 Computational modeling with organoids
Computational methods play an integral role in the analysis of complex omics and imaging data, and computational simulations have become an important tool in cancer and drug research (Metzcar et al., 2019; Cui et al., 2020; Lin et al., 2020). Likewise, in silico studies of tissue development during embryogenesis are well established (Tanaka, 2015; Gómez et al., 2017; Tosenberger et al., 2019), and powerful simulation environments to simulate patterning and growth in tissues are readily available, both for continuum and cellular domains (Swat et al., 2012; Mirams et al., 2013; Iber et al., 2015; Tanaka et al., 2015; Multerer et al., 2018; Kim H. et al., 2020). The inherent properties of organoid models, such as recapitulating and maintaining biological features of their tissue of origin, accessibility and their comparably small size, make them an ideal tool to inform and/or validate computational simulations (Figure 2). Various approaches in 2D and 3D, such as agent-based models, vertex models, Potts models, and finite element models have been utilized to model cellular interactions and biomechanical properties in organoids (Montes-Olivas et al., 2019; Norfleet et al., 2020; Byrne, 2022). Furthermore, computational modeling has also been used to optimize culture conditions (Goto-Silva et al., 2019).
As organoids can be imaged at cellular resolution, 3D vertex models are frequently used to simulate organoid development at cellular resolution (Misra et al., 2016; Okuda et al., 2018; Yang et al., 2021). As epithelial cells change their neighbors along the apical-basal axis (Gómez et al., 2021; Iber and Vetter, 2022), classical 3D vertex models have been extended to allow for an intermediate vertex along the apical-basal axis (Ioannou et al., 2020). Recently, high-resolution 3D simulation frameworks have become available that represent cells by individual, deformable meshes (Van Liedekerke et al., 2020; Torres-Sánchez et al., 2022). Given their high computational costs, these simulation frameworks are sometimes used in combination with spheroid models to permit the simulation of larger tissues and efficient parameter screens. Further developments will allow for the simulation of larger tissues at higher spatial resolution over longer time frames. In combination with advancements in organoid research, this will open up new ways to understand the molecular principles of tissue development and disease.
9 Summary
In this review, we illustrated the advancements of modern-day organoid protocols that enable HTS and HCA screening. Conventional HTS rely on 2D cell culture assays, where the lack of cell-cell and cell-matrix interaction may confound results. Organoid cultures can circumvent some of these limitations, but classic organoids culturing protocols are not well suited for upscaling. Nevertheless, recent developments in culturing methods and platforms have demonstrated that organoids can be made accessible, for high-throughput drug screening.
The higher complexity of organoids makes them also an appealing tool for HCA. Bulk- and single-cell omics approaches can be used to study tissue interaction, homeostasis, and cell interaction, as well as alterations that are caused by or lead to disease onset and progression. Due to their relatively small size, organoids are furthermore accessible for high-resolution 3D and 4D imaging. Moreover, machine learning has emerged as an integral tool for comprehending high-dimensional datasets, particularly in HCA.
Computational methods play an essential role in analyzing complex omics and imaging data. However, computational approaches are not only of interest for data analysis. In silico studies of mechanobiological properties in tissues can be used to validate findings from organoid research or inform experimental designs. The smaller size and accessibility of organoids can again be advantageous, as using spheroid models for in silico studies instead of larger tissue areas helps to lower computational costs.
The articles we covered in this review represent a small portion of the body of work published on organoid research to date. This illustrates the profound impact that the emergence of modern-day organoid protocols have had on biomedical research. As many groups work on enhancing organoid procedures to address current limitations and make organoids more accessible, it is anticipated that organoid research will become even more significant in the fields of disease, cancer and development.
Author contributions
FL and ND conceived and wrote the manuscript. FL created the figures. DI conceived and edited the manuscript. All authors contributed to the article and approved it for publication.
Funding
Open access funding provided by ETH Zurich.
Acknowledgments
We thank all members of the CoBi group, especially Dr Roman Vetter, for their feedback and discussions.
Conflict of interest
The authors declare that the research was conducted in the absence of any commercial or financial relationships that could be construed as a potential conflict of interest.
Publisher’s note
All claims expressed in this article are solely those of the authors and do not necessarily represent those of their affiliated organizations, or those of the publisher, the editors and the reviewers. Any product that may be evaluated in this article, or claim that may be made by its manufacturer, is not guaranteed or endorsed by the publisher.
References
Amadei, G., Handford, C. E., Qiu, C., De Jonghe, J., Greenfeld, H., Tran, M., et al. (2022). Embryo model completes gastrulation to neurulation and organogenesis. Nature 610, 143–153. doi:10.1038/s41586-022-05246-3
An, W. F., and Tolliday, N. (2010). Cell-based assays for high-throughput screening. Mol. Biotechnol. 45, 180–186. doi:10.1007/s12033-010-9251-z
Anderson, N. M., and Simon, M. C. (2020). The tumor microenvironment. Curr. Biol. 30, R921–R925. doi:10.1016/j.cub.2020.06.081
Arez, F., Rebelo, S. P., Fontinha, D., Simão, D., Martins, T. R., Machado, M., et al. (2019). Flexible 3D cell-based platforms for the discovery and profiling of novel drugs targeting plasmodium hepatic infection. ACS Infect. Dis. 5, 1831–1842. doi:10.1021/acsinfecdis.9b00144
Baillie-Johnson, P., van den Brink, S. C., Balayo, T., Turner, D. A., and Martinez Arias, A. (2015). Generation of aggregates of mouse embryonic stem cells that show symmetry breaking, polarization and emergent collective behaviour in vitro. J. Vis. Exp. JoVE, 53252. doi:10.3791/53252
Baktash, Y., Madhav, A., Coller, K. E., and Randall, G. (2018). Single particle imaging of polarized hepatoma organoids upon hepatitis C virus infection reveals an ordered and sequential entry process. Cell. Host Microbe 23, 382–394.e5. doi:10.1016/j.chom.2018.02.005
Bartfeld, S., Bayram, T., van de Wetering, M., Huch, M., Begthel, H., Kujala, P., et al. (2015). In vitro expansion of human gastric epithelial stem cells and their responses to bacterial infection. Gastroenterology 148, 126–136.e6. doi:10.1053/j.gastro.2014.09.042
Beghin, A., Grenci, G., Sahni, G., Guo, S., Rajendiran, H., Delaire, T., et al. (2022). Automated high-speed 3D imaging of organoid cultures with multi-scale phenotypic quantification. Nat. Methods 19, 881–892. doi:10.1038/s41592-022-01508-0
Behjati, S., Huch, M., van Boxtel, R., Karthaus, W., Wedge, D. C., Tamuri, A. U., et al. (2014). Genome sequencing of normal cells reveals developmental lineages and mutational processes. Nature 513, 422–425. doi:10.1038/nature13448
Birey, F., Andersen, J., Makinson, C. D., Islam, S., Wei, W., Huber, N., et al. (2017). Assembly of functionally integrated human forebrain spheroids. Nature 545, 54–59. doi:10.1038/nature22330
Boj, S. F., Hwang, C.-I., Baker, L. A., Chio, I. I. C., Engle, D. D., Corbo, V., et al. (2015). Organoid models of human and mouse ductal pancreatic cancer. Cell. 160, 324–338. doi:10.1016/j.cell.2014.12.021
Booij, T. H., Bange, H., Leonhard, W. N., Yan, K., Fokkelman, M., Kunnen, S. J., et al. (2017). High-throughput phenotypic screening of kinase inhibitors to identify drug targets for polycystic kidney disease. SLAS Discov. Adv. Sci. Drug Discov. 22, 974–984. doi:10.1177/2472555217716056
Borrelli, C., and Moor, A. E. (2020). “Single-molecule RNA FISH in whole-mount organoids,” in Intestinal stem cells: Methods and protocols. Editor P. Ordóñez-Morán (New York, NY: Springer US), 237–247. Methods in Molecular Biology. doi:10.1007/978-1-0716-0747-3_15
Brandenberg, N., Hoehnel, S., Kuttler, F., Homicsko, K., Ceroni, C., Ringel, T., et al. (2020). High-throughput automated organoid culture via stem-cell aggregation in microcavity arrays. Nat. Biomed. Eng. 4, 863–874. doi:10.1038/s41551-020-0565-2
Breslin, S., and O’Driscoll, L. (2013). Three-dimensional cell culture: The missing link in drug discovery. Drug Discov. Today 18, 240–249. doi:10.1016/j.drudis.2012.10.003
Broutier, L., Mastrogiovanni, G., Verstegen, M. M., Francies, H. E., Gavarró, L. M., Bradshaw, C. R., et al. (2017). Human primary liver cancer–derived organoid cultures for disease modeling and drug screening. Nat. Med. 23, 1424–1435. doi:10.1038/nm.4438
Byrne, H. (2022). In vitro and in silico approaches to engineering three-dimensional biological tissues and organoids. Interface Focus 12, 20220046. doi:10.1098/rsfs.2022.0046
Camp, J. G., Badsha, F., Florio, M., Kanton, S., Gerber, T., Wilsch-Bräuninger, M., et al. (2015). Human cerebral organoids recapitulate gene expression programs of fetal neocortex development. PNAS 112, 15672–15677. doi:10.1073/pnas.1520760112
Cashman, T. J., Josowitz, R., Johnson, B. V., Gelb, B. D., and Costa, K. D. (2016). Human engineered cardiac tissues created using induced pluripotent stem cells reveal functional characteristics of BRAF-mediated hypertrophic cardiomyopathy. PLoS ONE 11, e0146697. doi:10.1371/journal.pone.0146697
Clevers, H. (2016). Modeling development and disease with organoids. Cell. 165, 1586–1597. doi:10.1016/j.cell.2016.05.082
Cristobal, A., van den Toorn, H. W. P., van de Wetering, M., Clevers, H., Heck, A. J. R., and Mohammed, S. (2017). Personalized proteome profiles of healthy and tumor human colon organoids reveal both individual diversity and basic features of colorectal cancer. Cell. Rep. 18, 263–274. doi:10.1016/j.celrep.2016.12.016
Cui, W., Aouidate, A., Wang, S., Yu, Q., Li, Y., and Yuan, S. (2020). Discovering anti-cancer drugs via computational methods. Front. Pharmacol. 11, 733. doi:10.3389/fphar.2020.00733
Czerniecki, S. M., Cruz, N. M., Harder, J. L., Menon, R., Annis, J., Otto, E. A., et al. (2018). High-throughput screening enhances kidney organoid differentiation from human pluripotent stem cells and enables automated multidimensional phenotyping. Cell. Stem Cell. 22, 929–940.e4. doi:10.1016/j.stem.2018.04.022
da Silva, B., Mathew, R. K., Polson, E. S., Williams, J., and Wurdak, H. (2018). Spontaneous glioblastoma spheroid infiltration of early-stage cerebral organoids models brain tumor invasion. SLAS Discov. Adv. Sci. Drug Discov. 23, 862–868. doi:10.1177/2472555218764623
de Medeiros, G., Ortiz, R., Strnad, P., Boni, A., Moos, F., Repina, N., et al. (2022). Multiscale light-sheet organoid imaging framework. Nat. Commun. 13, 4864. doi:10.1038/s41467-022-32465-z
Dekkers, J. F., Alieva, M., Wellens, L. M., Ariese, H. C. R., Jamieson, P. R., Vonk, A. M., et al. (2019). High-resolution 3D imaging of fixed and cleared organoids. Nat. Protoc. 14, 1756–1771. doi:10.1038/s41596-019-0160-8
Dijkstra, K. K., Monkhorst, K., Schipper, L. J., Hartemink, K. J., Smit, E. F., Kaing, S., et al. (2020). Challenges in establishing pure lung cancer organoids limit their utility for personalized medicine. Cell. Rep. 31, 107588. doi:10.1016/j.celrep.2020.107588
Drost, J., Karthaus, W. R., Gao, D., Driehuis, E., Sawyers, C. L., Chen, Y., et al. (2016). Organoid culture systems for prostate epithelial and cancer tissue. Nat. Protoc. 11, 347–358. doi:10.1038/nprot.2016.006
Drost, J., van Jaarsveld, R. H., Ponsioen, B., Zimberlin, C., van Boxtel, R., Buijs, A., et al. (2015). Sequential cancer mutations in cultured human intestinal stem cells. Nature 521, 43–47. doi:10.1038/nature14415
Du, Y., Li, X., Niu, Q., Mo, X., Qui, M., Ma, T., et al. (2020). Development of a miniaturized 3D organoid culture platform for ultra-high-throughput screening. J. Mol. Cell. Biol. 12, 630–643. doi:10.1093/jmcb/mjaa036
Dutta, D., Heo, I., and Clevers, H. (2017). Disease modeling in stem cell-derived 3D organoid systems. Trends Mol. Med. 23, 393–410. doi:10.1016/j.molmed.2017.02.007
Eiraku, M., Takata, N., Ishibashi, H., Kawada, M., Sakakura, E., Okuda, S., et al. (2011). Self-organizing optic-cup morphogenesis in three-dimensional culture. Nature 472, 51–56. doi:10.1038/nature09941
Elias, M. S., Wright, S. C., Nicholson, W. V., Morrison, K. D., Prescott, A. R., Ten Have, S., et al. (2019). Functional and proteomic analysis of a full thickness filaggrin-deficient skin organoid model. Wellcome Open Res. 4, 134. doi:10.12688/wellcomeopenres.15405.2
Fatehullah, A., Tan, S. H., and Barker, N. (2016). Organoids as an in vitro model of human development and disease. Nat. Cell. Biol. 18, 246–254. doi:10.1038/ncb3312
Fujii, M., Shimokawa, M., Date, S., Takano, A., Matano, M., Nanki, K., et al. (2016). A colorectal tumor organoid library demonstrates progressive loss of niche factor requirements during tumorigenesis. Cell. Stem Cell. 18, 827–838. doi:10.1016/j.stem.2016.04.003
Gao, D., Vela, I., Sboner, A., Iaquinta, P. J., Karthaus, W. R., Gopalan, A., et al. (2014). Organoid cultures derived from patients with advanced prostate cancer. Cell. 159, 176–187. doi:10.1016/j.cell.2014.08.016
Garcez, P. P., Loiola, E. C., Madeiro da Costa, R., Higa, L. M., Trindade, P., Delvecchio, R., et al. (2016). Zika virus impairs growth in human neurospheres and brain organoids. Sci. (New York, N.Y.) 352, 816–818. doi:10.1126/science.aaf6116
Girgin, M. U., Broguiere, N., Mattolini, L., and Lutolf, M. P. (2021). Gastruloids generated without exogenous Wnt activation develop anterior neural tissues. Stem Cell. Rep. 16, 1143–1155. doi:10.1016/j.stemcr.2021.03.017
Gjorevski, N., Sachs, N., Manfrin, A., Giger, S., Bragina, M. E., Ordóñez-Morán, P., et al. (2016). Designer matrices for intestinal stem cell and organoid culture. Nature 539, 560–564. doi:10.1038/nature20168
Gómez, H. F., Dumond, M. S., Hodel, L., Vetter, R., and Iber, D. (2021). 3D cell neighbour dynamics in growing pseudostratified epithelia. eLife 10, e68135. doi:10.7554/eLife.68135
Gómez, H. F., Georgieva, L., Michos, O., and Iber, D. (2017). “Image-based in silico models of organogenesis,” in Systems biology (Hoboken, New Jersey, U.S: John Wiley & Sons), 319–340. doi:10.1002/9783527696130.ch12
Gonneaud, A., Jones, C., Turgeon, N., Lévesque, D., Asselin, C., Boudreau, F., et al. (2016). A SILAC-based method for quantitative proteomic analysis of intestinal organoids. Sci. Rep. 6, 38195. doi:10.1038/srep38195
Goto-Silva, L., Ayad, N. M. E., Herzog, I. L., Silva, N. P., Lamien, B., Orlande, H. R. B., et al. (2019). Computational fluid dynamic analysis of physical forces playing a role in brain organoid cultures in two different multiplex platforms. BMC Dev. Biol. 19, 3. doi:10.1186/s12861-019-0183-y
Gracz, A. D., Williamson, I. A., Roche, K. C., Johnston, M. J., Wang, F., Wang, Y., et al. (2015). A high-throughput platform for stem cell niche co-cultures and downstream gene expression analysis. Nat. Cell. Biol. 17, 340–349. doi:10.1038/ncb3104
Gritti, N., Lim, J. L., Anlaş, K., Pandya, M., Aalderink, G., Martínez-Ara, G., et al. (2021). MOrgAna: Accessible quantitative analysis of organoids with machine learning. Development 148, dev199611. doi:10.1242/dev.199611
Groveman, B. R., Foliaki, S. T., Orru, C. D., Zanusso, G., Carroll, J. A., Race, B., et al. (2019). Sporadic Creutzfeldt-Jakob disease prion infection of human cerebral organoids. Acta Neuropathol. Commun. 7, 90. doi:10.1186/s40478-019-0742-2
Grün, D., Lyubimova, A., Kester, L., Wiebrands, K., Basak, O., Sasaki, N., et al. (2015). Single-cell messenger RNA sequencing reveals rare intestinal cell types. Nature 525, 251–255. doi:10.1038/nature14966
Gunasekara, D. B., DiSalvo, M., Wang, Y., Nguyen, D. L., Reed, M. I., Speer, J., et al. (2018). Development of arrayed colonic organoids for screening of secretagogues associated with enterotoxins. Anal. Chem. 90, 1941–1950. doi:10.1021/acs.analchem.7b04032
Harrison, S. E., Sozen, B., Christodoulou, N., Kyprianou, C., and Zernicka-Goetz, M. (2017). Assembly of embryonic and extraembryonic stem cells to mimic embryogenesis in vitro. Sci. (New York, N.Y.) 356, eaal1810. doi:10.1126/science.aal1810
Hautefort, I., Poletti, M., Papp, D., and Korcsmaros, T. (2022). Everything you always wanted to know about organoid-based models (and never dared to ask). Cell. Mol. Gastroenterology Hepatology 14, 311–331. doi:10.1016/j.jcmgh.2022.04.012
Heo, I., Dutta, D., Schaefer, D. A., Iakobachvili, N., Artegiani, B., Sachs, N., et al. (2018). Modelling Cryptosporidium infection in human small intestinal and lung organoids. Nat. Microbiol. 3, 814–823. doi:10.1038/s41564-018-0177-8
Hinson, J. T., Chopra, A., Nafissi, N., Polacheck, W. J., Benson, C. C., Swist, S., et al. (2015). Titin mutations in iPS cells define sarcomere insufficiency as a cause of dilated cardiomyopathy. Sci. (New York, N.Y.) 349, 982–986. doi:10.1126/science.aaa5458
Hofer, M., and Lutolf, M. P. (2021). Engineering organoids. Nat. Rev. Mater. 6, 402–420. doi:10.1038/s41578-021-00279-y
Hou, S., Tiriac, H., Sridharan, B. P., Scampavia, L., Madoux, F., Seldin, J., et al. (2018). Advanced development of primary pancreatic organoid tumor models for high-throughput phenotypic drug screening. SLAS Discov. Adv. Sci. Drug Discov. 23, 574–584. doi:10.1177/2472555218766842
Hua, Z.-Q., Liu, H., Wang, N., and Jin, Z.-B. (2020). “Chapter 7 - towards stem cell-based neuronal regeneration for glaucoma,” in Progress in brain research. Editors G. Bagetta, and C. Nucci (Amsterdam, Netherlands: Elsevier), 99–118. vol. 257 of Glaucoma: A Neurodegenerative Disease of the Retina and Beyond - Part B. doi:10.1016/bs.pbr.2020.05.026
Huch, M., Bonfanti, P., Boj, S. F., Sato, T., Loomans, C. J. M., van de Wetering, M., et al. (2013). Unlimited in vitro expansion of adult bi-potent pancreas progenitors through the Lgr5/R-spondin axis. EMBO J. 32, 2708–2721. doi:10.1038/emboj.2013.204
Iber, D., Tanaka, S., Fried, P., Germann, P., and Menshykau, D. (2015). “Simulating tissue morphogenesis and signaling,” in Tissue morphogenesis: Methods and protocols. Editor C. M. Nelson (New York, NY: Springer), 323–338. Methods in Molecular Biology. doi:10.1007/978-1-4939-1164-6_21
Iber, D., and Vetter, R. (2022). 3D organisation of cells in pseudostratified epithelia. Front. Phys. 10. doi:10.3389/fphy.2022.898160
Ioannou, F., Dawi, M. A., Tetley, R. J., Mao, Y., and Muñoz, J. J. (2020). Development of a new 3D hybrid model for epithelia morphogenesis. Front. Bioeng. Biotechnol. 8, 405. doi:10.3389/fbioe.2020.00405
Jacob, F., Pather, S. R., Huang, W.-K., Zhang, F., Wong, S. Z. H., Zhou, H., et al. (2020). Human pluripotent stem cell-derived neural cells and brain organoids reveal SARS-CoV-2 neurotropism predominates in choroid plexus epithelium. Cell. Stem Cell. 27, 937–950.e9. doi:10.1016/j.stem.2020.09.016
Kanton, S., Boyle, M. J., He, Z., Santel, M., Weigert, A., Sanchís-Calleja, F., et al. (2019). Organoid single-cell genomic atlas uncovers human-specific features of brain development. Nature 574, 418–422. doi:10.1038/s41586-019-1654-9
Kanton, S., and Paşca, S. P. (2022). Human assembloids. Development 149, dev201120. doi:10.1242/dev.201120
Kapałczyńska, M., Kolenda, T., Przybyła, W., Zajączkowska, M., Teresiak, A., Filas, V., et al. (2018). 2D and 3D cell cultures - a comparison of different types of cancer cell cultures. Archives Med. Sci. 14, 910–919. doi:10.5114/aoms.2016.63743
Kassis, T., Hernandez-Gordillo, V., Langer, R., and Griffith, L. G. (2019). OrgaQuant: Human intestinal organoid localization and quantification using deep convolutional neural networks. Sci. Rep. 9, 12479. doi:10.1038/s41598-019-48874-y
Kester, L., de Barbanson, B., Lyubimova, A., Chen, L.-T., van der Schrier, V., Alemany, A., et al. (2022). Integration of multiple lineage measurements from the same cell reconstructs parallel tumor evolution. Cell. Genomics 2, 100096. doi:10.1016/j.xgen.2022.100096
Kim, E., Choi, S., Kang, B., Kong, J., Kim, Y., Yoon, W. H., et al. (2020a). Creation of bladder assembloids mimicking tissue regeneration and cancer. Nature 588, 664–669. doi:10.1038/s41586-020-3034-x
Kim, H., Jin, X., Glass, D. S., and Riedel-Kruse, I. H. (2020b). Engineering and modeling of multicellular morphologies and patterns. Curr. Opin. Genet. Dev. 63, 95–102. doi:10.1016/j.gde.2020.05.039
Kim, J.-W., Kim, Y., Kim, J., Park, M.-J., Kwon, E., Lee, J., et al. (2018). AB0189 3d skin organoid mimicking systemic sclerosis generated by patient-derived induced pluripotent stem cells: ‘disease in a dish’ and development of animal model. Ann. Rheumatic Dis. 77, 1281. doi:10.1136/annrheumdis-2018-eular.4502
Koehler, K. R., Mikosz, A. M., Molosh, A. I., Patel, D., and Hashino, E. (2013). Generation of inner ear sensory epithelia from pluripotent stem cells in 3D culture. Nature 500, 217–221. doi:10.1038/nature12298
Kok, R. N. U., Hebert, L., Huelsz-Prince, G., Goos, Y. J., Zheng, X., Bozek, K., et al. (2020). OrganoidTracker: Efficient cell tracking using machine learning and manual error correction. PLOS ONE 15, e0240802. doi:10.1371/journal.pone.0240802
Kong, J., Lee, H., Kim, D., Han, S. K., Ha, D., Shin, K., et al. (2020). Network-based machine learning in colorectal and bladder organoid models predicts anti-cancer drug efficacy in patients. Nat. Commun. 11, 5485. doi:10.1038/s41467-020-19313-8
Kopper, O., de Witte, C. J., Lõhmussaar, K., Valle-Inclan, J. E., Hami, N., Kester, L., et al. (2019). An organoid platform for ovarian cancer captures intra- and interpatient heterogeneity. Nat. Med. 25, 838–849. doi:10.1038/s41591-019-0422-6
Lancaster, M. A., Renner, M., Martin, C.-A., Wenzel, D., Bicknell, L. S., Hurles, M. E., et al. (2013). Cerebral organoids model human brain development and microcephaly. Nature 501, 373–379. doi:10.1038/nature12517
Lau, K. Y. C., Rubinstein, H., Gantner, C. W., Hadas, R., Amadei, G., Stelzer, Y., et al. (2022). Mouse embryo model derived exclusively from embryonic stem cells undergoes neurulation and heart development. Cell. Stem Cell. 29, 1445–1458.e8. doi:10.1016/j.stem.2022.08.013
Lee, S. H., Hu, W., Matulay, J. T., Silva, M. V., Owczarek, T. B., Kim, K., et al. (2018). Tumor evolution and drug response in patient-derived organoid models of bladder cancer. Cell. 173, 515–528.e17. doi:10.1016/j.cell.2018.03.017
LeSavage, B. L., Suhar, R. A., Broguiere, N., Lutolf, M. P., and Heilshorn, S. C. (2022). Next-generation cancer organoids. Nat. Mater. 21, 143–159. doi:10.1038/s41563-021-01057-5
Li, M. L., Aggeler, J., Farson, D. A., Hatier, C., Hassell, J., and Bissell, M. J. (1987). Influence of a reconstituted basement membrane and its components on casein gene expression and secretion in mouse mammary epithelial cells. Proc. Natl. Acad. Sci. 84, 136–140. doi:10.1073/pnas.84.1.136
Li, X., Nadauld, L., Ootani, A., Corney, D. C., Pai, R. K., Gevaert, O., et al. (2014). Oncogenic transformation of diverse gastrointestinal tissues in primary organoid culture. Nat. Med. 20, 769–777. doi:10.1038/nm.3585
Lin, X., Li, X., and Lin, X. (2020). A review on applications of computational methods in drug screening and design. Molecules 25, 1375. doi:10.3390/molecules25061375
Lindeboom, R. G., van Voorthuijsen, L., Oost, K. C., Rodriguez-Colman, M. J., Luna-Velez, M. V., Furlan, C., et al. (2018). Integrative multi-omics analysis of intestinal organoid differentiation. Mol. Syst. Biol. 14, e8227. doi:10.15252/msb.20188227
Lõhmussaar, K., Oka, R., Espejo Valle-Inclan, J., Smits, M. H. H., Wardak, H., Korving, J., et al. (2021). Patient-derived organoids model cervical tissue dynamics and viral oncogenesis in cervical cancer. Cell. Stem Cell. 28, 1380–1396.e6. doi:10.1016/j.stem.2021.03.012
Ma, J., Liu, J., Gao, D., Li, X., Zhang, Q., Lv, L., et al. (2022). Establishment of human pluripotent stem cell-derived skin organoids enabled pathophysiological model of SARS-CoV-2 infection. Adv. Sci. 9, e2104192. doi:10.1002/advs.202104192
Mantziou, V., Baillie-Benson, P., Jaklin, M., Kustermann, S., Arias, A. M., and Moris, N. (2021). In vitro teratogenicity testing using a 3D, embryo-like gastruloid system. Reprod. Toxicol. 105, 72–90. doi:10.1016/j.reprotox.2021.08.003
Marikawa, Y., Tamashiro, D. A. A., Fujita, T. C., and Alarcón, V. B. (2009). Aggregated P19 mouse embryonal carcinoma cells as a simple in vitro model to study the molecular regulations of mesoderm formation and axial elongation morphogenesis. Genesis 47, 93–106. doi:10.1002/dvg.20473
Marton, R. M., and Pașca, S. P. (2020). Organoid and assembloid technologies for investigating cellular crosstalk in human brain development and disease. Trends Cell. Biol. 30, 133–143. doi:10.1016/j.tcb.2019.11.004
Matano, M., Date, S., Shimokawa, M., Takano, A., Fujii, M., Ohta, Y., et al. (2015). Modeling colorectal cancer using CRISPR-Cas9–mediated engineering of human intestinal organoids. Nat. Med. 21, 256–262. doi:10.1038/nm.3802
McGhee, A. J., McGhee, E. O., Famiglietti, J. E., and Sawyer, W. G. (2022). In situ 3d spatiotemporal measurement of soluble biomarkers in organoid culture. arXiv. doi:10.48550/arXiv.2209.01164
Metzcar, J., Wang, Y., Heiland, R., and Macklin, P. (2019). A review of cell-based computational modeling in cancer biology. JCO Clin. Cancer Inf. 3, 1–13. doi:10.1200/CCI.18.00069
Middendorp, S., Schneeberger, K., Wiegerinck, C. L., Mokry, M., Akkerman, R. D. L., Wijngaarden, S., et al. (2014). Adult stem cells in the small intestine are intrinsically programmed with their location-specific function. Stem Cells 32, 1083–1091. doi:10.1002/stem.1655
Miller, A. J., Dye, B. R., Ferrer-Torres, D., Hill, D. R., Overeem, A. W., Shea, L. D., et al. (2019). Generation of lung organoids from human pluripotent stem cells in vitro. Nat. Protoc. 14, 518–540. doi:10.1038/s41596-018-0104-8
Mills, R. J., Humphrey, S. J., Fortuna, P. R. J., Lor, M., Foster, S. R., Quaife-Ryan, G. A., et al. (2021). BET inhibition blocks inflammation-induced cardiac dysfunction and SARS-CoV-2 infection. Cell. 184, 2167–2182.e22. doi:10.1016/j.cell.2021.03.026
Mirams, G. R., Arthurs, C. J., Bernabeu, M. O., Bordas, R., Cooper, J., Corrias, A., et al. (2013). Chaste: An open source C++ library for computational physiology and biology. PLOS Comput. Biol. 9, e1002970. doi:10.1371/journal.pcbi.1002970
Misra, M., Audoly, B., Kevrekidis, I. G., and Shvartsman, S. Y. (2016). Shape transformations of epithelial shells. Biophysical J. 110, 1670–1678. doi:10.1016/j.bpj.2016.03.009
Montes-Olivas, S., Marucci, L., and Homer, M. (2019). Mathematical models of organoid cultures. Front. Genet. 10, 873. doi:10.3389/fgene.2019.00873
Monzel, A. S., Hemmer, K., Kaoma, T., Smits, L. M., Bolognin, S., Lucarelli, P., et al. (2020). Machine learning-assisted neurotoxicity prediction in human midbrain organoids. Park. Relat. Disord. 75, 105–109. doi:10.1016/j.parkreldis.2020.05.011
Multerer, M. D., Wittwer, L. D., Stopka, A., Barac, D., Lang, C., and Iber, D. (2018). “Simulation of morphogen and tissue dynamics,” in Morphogen gradients: Methods and protocols. Editor J. Dubrulle (New York, NY: Springer), 223–250. Methods in Molecular Biology. doi:10.1007/978-1-4939-8772-6_13
Nanki, K., Toshimitsu, K., Takano, A., Fujii, M., Shimokawa, M., Ohta, Y., et al. (2018). Divergent routes toward Wnt and R-spondin niche independency during human gastric carcinogenesis. Cell. 174, 856–869.e17. doi:10.1016/j.cell.2018.07.027
Neal, J. T., Li, X., Zhu, J., Giangarra, V., Grzeskowiak, C. L., Ju, J., et al. (2018). Organoid modeling of the tumor immune microenvironment. Cell. 175, 1972–1988.e16. doi:10.1016/j.cell.2018.11.021
Nie, Y.-Z., Zheng, Y.-W., Miyakawa, K., Murata, S., Zhang, R.-R., Sekine, K., et al. (2018). Recapitulation of Hepatitis B virus-host interactions in liver organoids from human induced pluripotent stem cells. EBioMedicine 35, 114–123. doi:10.1016/j.ebiom.2018.08.014
Norfleet, D. A., Park, E., and Kemp, M. L. (2020). Computational modeling of organoid development. Curr. Opin. Biomed. Eng. 13, 113–118. doi:10.1016/j.cobme.2019.12.014
Okuda, S., Takata, N., Hasegawa, Y., Kawada, M., Inoue, Y., Adachi, T., et al. (2018). Strain-triggered mechanical feedback in self-organizing optic-cup morphogenesis. Sci. Adv. 4, eaau1354. doi:10.1126/sciadv.aau1354
Omerzu, M., Fenderico, N., de Barbanson, B., Sprangers, J., de Ridder, J., and Maurice, M. M. (2019). Three-dimensional analysis of single molecule FISH in human colon organoids. Biol. Open 8, bio042812. doi:10.1242/bio.042812
Pellegrini, L., Albecka, A., Mallery, D. L., Kellner, M. J., Paul, D., Carter, A. P., et al. (2020). SARS-CoV-2 infects the brain choroid plexus and disrupts the blood-CSF barrier in human brain organoids. Cell. Stem Cell. 27, 951–961.e5. doi:10.1016/j.stem.2020.10.001
Phan, N., Hong, J. J., Tofig, B., Mapua, M., Elashoff, D., Moatamed, N. A., et al. (2019). A simple high-throughput approach identifies actionable drug sensitivities in patient-derived tumor organoids. Commun. Biol. 2, 1–11. doi:10.1038/s42003-019-0305-x
Przepiorski, A., Sander, V., Tran, T., Hollywood, J. A., Sorrenson, B., Shih, J.-H., et al. (2018). A simple bioreactor-based method to generate kidney organoids from pluripotent stem cells. Stem Cell. Rep. 11, 470–484. doi:10.1016/j.stemcr.2018.06.018
Qin, X., Sufi, J., Vlckova, P., Kyriakidou, P., Acton, S. E., Li, V. S. W., et al. (2020). Cell-type-specific signaling networks in heterocellular organoids. Nat. Methods 17, 335–342. doi:10.1038/s41592-020-0737-8
Rheinwatd, J. G., and Green, H. (1975). Seria cultivation of strains of human epidemal keratinocytes: The formation keratinizin colonies from single cell is. Cell. 6, 331–343. doi:10.1016/S0092-8674(75)80001-8
Roerink, S. F., Sasaki, N., Lee-Six, H., Young, M. D., Alexandrov, L. B., Behjati, S., et al. (2018). Intra-tumour diversification in colorectal cancer at the single-cell level. Nature 556, 457–462. doi:10.1038/s41586-018-0024-3
Rosenbluth, J. M., Schackmann, R. C. J., Gray, G. K., Selfors, L. M., Li, C. M.-C., Boedicker, M., et al. (2020). Organoid cultures from normal and cancer-prone human breast tissues preserve complex epithelial lineages. Nat. Commun. 11, 1711. doi:10.1038/s41467-020-15548-7
Rossi, G., Broguiere, N., Miyamoto, M., Boni, A., Guiet, R., Girgin, M., et al. (2021). Capturing cardiogenesis in gastruloids. Cell. Stem Cell. 28, 230–240.e6. doi:10.1016/j.stem.2020.10.013
Sachs, N., de Ligt, J., Kopper, O., Gogola, E., Bounova, G., Weeber, F., et al. (2018). A living biobank of breast cancer organoids captures disease heterogeneity. Cell. 172, 373–386.e10. doi:10.1016/j.cell.2017.11.010
Sakamoto, N., Feng, Y., Stolfi, C., Kurosu, Y., Green, M., Lin, J., et al. (2017). BRAFV600E cooperates with CDX2 inactivation to promote serrated colorectal tumorigenesis. eLife 6, e20331. doi:10.7554/eLife.20331
Sato, T., Stange, D. E., Ferrante, M., Vries, R. G. J., van Es, J. H., van den Brink, S., et al. (2011). Long-term expansion of epithelial organoids from human colon, adenoma, adenocarcinoma, and barrett’s epithelium. Gastroenterology 141, 1762–1772. doi:10.1053/j.gastro.2011.07.050
Sato, T., Vries, R. G., Snippert, H. J., van de Wetering, M., Barker, N., Stange, D. E., et al. (2009). Single Lgr5 stem cells build crypt-villus structures in vitro without a mesenchymal niche. Nature 459, 262–265. doi:10.1038/nature07935
Schneider, H., Muirhead, E. E., and Zydeck, F. A. (1963). Some unusual observations of organoid tissues and blood elements in monolayer cultures. Exp. Cell. Res. 30, 449–459. doi:10.1016/0014-4827(63)90322-7
Seino, T., Kawasaki, S., Shimokawa, M., Tamagawa, H., Toshimitsu, K., Fujii, M., et al. (2018). Human pancreatic tumor organoids reveal loss of stem cell niche factor dependence during disease progression. Cell. Stem Cell. 22, 454–467.e6. doi:10.1016/j.stem.2017.12.009
Sozen, B., Amadei, G., Cox, A., Wang, R., Na, E., Czukiewska, S., et al. (2018). Self-assembly of embryonic and two extra-embryonic stem cell types into gastrulating embryo-like structures. Nat. Cell. Biol. 20, 979–989. doi:10.1038/s41556-018-0147-7
Spence, J. R., Mayhew, C. N., Rankin, S. A., Kuhar, M. F., Vallance, J. E., Tolle, K., et al. (2011). Directed differentiation of human pluripotent stem cells into intestinal tissue in vitro. Nature 470, 105–109. doi:10.1038/nature09691
Sridhar, A., Hoshino, A., Finkbeiner, C. R., Chitsazan, A., Dai, L., Haugan, A. K., et al. (2020). Single-cell transcriptomic comparison of human fetal retina, hPSC-derived retinal organoids, and long-term retinal cultures. Cell. Rep. 30, 1644–1659.e4. doi:10.1016/j.celrep.2020.01.007
Strikoudis, A., Cieślak, A., Loffredo, L., Chen, Y.-W., Patel, N., Saqi, A., et al. (2019). Modeling of fibrotic lung disease using 3D organoids derived from human pluripotent stem cells. Cell. Rep. 27, 3709–3723.e5. doi:10.1016/j.celrep.2019.05.077
Sun, Y. (2016). Tumor microenvironment and cancer therapy resistance. Cancer Lett. 380, 205–215. doi:10.1016/j.canlet.2015.07.044
Surolia, R., Li, F. J., Wang, Z., Li, H., Dsouza, K., Thomas, V., et al. (2019). Vimentin intermediate filament assembly regulates fibroblast invasion in fibrogenic lung injury. JCI insight 4, e123253. doi:10.1172/jci.insight.123253
Swat, M. H., Thomas, G. L., Belmonte, J. M., Shirinifard, A., Hmeljak, D., and Glazier, J. A. (2012). Multi-scale modeling of tissues using CompuCell3D. Methods Cell. Biol. 110, 325–366. doi:10.1016/B978-0-12-388403-9.00013-8
Takasato, M., Er, P. X., Becroft, M., Vanslambrouck, J. M., Stanley, E. G., Elefanty, A. G., et al. (2014). Directing human embryonic stem cell differentiation towards a renal lineage generates a self-organizing kidney. Nat. Cell. Biol. 16, 118–126. doi:10.1038/ncb2894
Takebe, T., Sekine, K., Enomura, M., Koike, H., Kimura, M., Ogaeri, T., et al. (2013). Vascularized and functional human liver from an iPSC-derived organ bud transplant. Nature 499, 481–484. doi:10.1038/nature12271
Tanaka, S., Sichau, D., and Iber, D. (2015). LBIBCell: A cell-based simulation environment for morphogenetic problems. Bioinforma. Oxf. Engl. 31, 2340–2347. doi:10.1093/bioinformatics/btv147
Tanaka, S. (2015). Simulation frameworks for morphogenetic problems. Computation 3, 197–221. doi:10.3390/computation3020197
Tiriac, H., Belleau, P., Engle, D. D., Plenker, D., Deschênes, A., Somerville, T. D. D., et al. (2018). Organoid profiling identifies common responders to chemotherapy in pancreatic cancer. Cancer Discov. 8, 1112–1129. doi:10.1158/2159-8290.CD-18-0349
Torres-Sánchez, A., Winter, M. K., and Salbreux, G. (2022). Interacting active surfaces: A model for three-dimensional cell aggregates. PLOS Comput. Biol. 18, e1010762. doi:10.1371/journal.pcbi.1010762
Tosenberger, A., Gonze, D., Chazaud, C., and Dupont, G. (2019). Computational models for the dynamics of early mouse embryogenesis. Int. J. Dev. Biol. 63, 131–142. doi:10.1387/ijdb.180418gd
Turco, M. Y., Gardner, L., Hughes, J., Cindrova-Davies, T., Gomez, M. J., Farrell, L., et al. (2017). Long-term, hormone-responsive organoid cultures of human endometrium in a chemically defined medium. Nat. Cell. Biol. 19, 568–577. doi:10.1038/ncb3516
Usman, O. H., Zhang, L., Xie, G., Kocher, H. M., Hwang, C.-i., Wang, Y. J., et al. (2022). Genomic heterogeneity in pancreatic cancer organoids and its stability with culture. npj Genomic Med. 7, 1–10. doi:10.1038/s41525-022-00342-9
van de Wetering, M., Francies, H. E., Francis, J. M., Bounova, G., Iorio, F., Pronk, A., et al. (2015). Prospective derivation of a living organoid biobank of colorectal cancer patients. Cell. 161, 933–945. doi:10.1016/j.cell.2015.03.053
van den Brink, S. C., Alemany, A., van Batenburg, V., Moris, N., Blotenburg, M., Vivié, J., et al. (2020). Single-cell and spatial transcriptomics reveal somitogenesis in gastruloids. Nature 582, 405–409. doi:10.1038/s41586-020-2024-3
van den Brink, S. C., Baillie-Johnson, P., Balayo, T., Hadjantonakis, A.-K., Nowotschin, S., Turner, D. A., et al. (2014). Symmetry breaking, germ layer specification and axial organisation in aggregates of mouse embryonic stem cells. Development 141, 4231–4242. doi:10.1242/dev.113001
Van Liedekerke, P., Neitsch, J., Johann, T., Warmt, E., Gonzàlez-Valverde, I., Hoehme, S., et al. (2020). A quantitative high-resolution computational mechanics cell model for growing and regenerating tissues. Biomechanics Model. Mechanobiol. 19, 189–220. doi:10.1007/s10237-019-01204-7
Vasyutin, I., Zerihun, L., Ivan, C., and Atala, A. (2019). Bladder organoids and spheroids: Potential tools for normal and diseased tissue modelling. Anticancer Res. 39, 1105–1118. doi:10.21873/anticanres.13219
Veenvliet, J. V., Bolondi, A., Kretzmer, H., Haut, L., Scholze-Wittler, M., Schifferl, D., et al. (2020). Mouse embryonic stem cells self-organize into trunk-like structures with neural tube and somites. Science 370, eaba4937. doi:10.1126/science.aba4937
Verissimo, C. S., Overmeer, R. M., Ponsioen, B., Drost, J., Mertens, S., Verlaan-Klink, I., et al. (2016). Targeting mutant RAS in patient-derived colorectal cancer organoids by combinatorial drug screening. eLife 5, e18489. doi:10.7554/eLife.18489
Vlachogiannis, G., Hedayat, S., Vatsiou, A., Jamin, Y., Fernández-Mateos, J., Khan, K., et al. (2018). Patient-derived organoids model treatment response of metastatic gastrointestinal cancers. Science 359, 920–926. doi:10.1126/science.aao2774
Wang, K., Yuen, S. T., Xu, J., Lee, S. P., Yan, H. H. N., Shi, S. T., et al. (2014b). Whole-genome sequencing and comprehensive molecular profiling identify new driver mutations in gastric cancer. Nat. Genet. 46, 573–582. doi:10.1038/ng.2983
Walsh, A. J., Cook, R. S., Sanders, M. E., Aurisicchio, L., Ciliberto, G., Arteaga, C. L., et al. (2014). Quantitative optical imaging of primary tumor organoid metabolism predicts drug response in breast cancer. Cancer Res. 74, 5184–5194. doi:10.1158/0008-5472.CAN-14-0663
Wang, G., McCain, M. L., Yang, L., He, A., Pasqualini, F. S., Agarwal, A., et al. (2014a). Modeling the mitochondrial cardiomyopathy of Barth syndrome with induced pluripotent stem cell and heart-on-chip technologies. Nat. Med. 20, 616–623. doi:10.1038/nm.3545
Wang, R., Mao, Y., Wang, W., Zhou, X., Wang, W., Gao, S., et al. (2022). Systematic evaluation of colorectal cancer organoid system by single-cell RNA-Seq analysis. Genome Biol. 23, 106. doi:10.1186/s13059-022-02673-3
Weeber, F., van de Wetering, M., Hoogstraat, M., Dijkstra, K. K., Krijgsman, O., Kuilman, T., et al. (2015). Preserved genetic diversity in organoids cultured from biopsies of human colorectal cancer metastases. Proc. Natl. Acad. Sci. 112, 13308–13311. doi:10.1073/pnas.1516689112
Williams, K. E., Lemieux, G. A., Hassis, M. E., Olshen, A. B., Fisher, S. J., and Werb, Z. (2016). Quantitative proteomic analyses of mammary organoids reveals distinct signatures after exposure to environmental chemicals. PNAS 113, E1343–E1351. doi:10.1073/pnas.1600645113
Yang, Q., Xue, S.-L., Chan, C. J., Rempfler, M., Vischi, D., Maurer-Gutierrez, F., et al. (2021). Cell fate coordinates mechano-osmotic forces in intestinal crypt formation. Nat. Cell. Biol. 23, 733–744. doi:10.1038/s41556-021-00700-2
Yin, Y., Bijvelds, M., Dang, W., Xu, L., van der Eijk, A. A., Knipping, K., et al. (2015). Modeling rotavirus infection and antiviral therapy using primary intestinal organoids. Antivir. Res. 123, 120–131. doi:10.1016/j.antiviral.2015.09.010
Keywords: organoids, high-throughput screening, high-content screening, stem cells, assembloids, cancer, computational modeling, gastruloids
Citation: Lampart FL, Iber D and Doumpas N (2023) Organoids in high-throughput and high-content screenings. Front. Chem. Eng. 5:1120348. doi: 10.3389/fceng.2023.1120348
Received: 09 December 2022; Accepted: 10 March 2023;
Published: 21 March 2023.
Edited by:
Camilla Luni, University of Bologna, ItalyReviewed by:
Mariaceleste Aragona, University of Copenhagen, DenmarkMattia Francesco Maria Gerli, University College London, United Kingdom
Copyright © 2023 Lampart, Iber and Doumpas. This is an open-access article distributed under the terms of the Creative Commons Attribution License (CC BY). The use, distribution or reproduction in other forums is permitted, provided the original author(s) and the copyright owner(s) are credited and that the original publication in this journal is cited, in accordance with accepted academic practice. No use, distribution or reproduction is permitted which does not comply with these terms.
*Correspondence: Nikolaos Doumpas, nikolaos.doumpas@bsse.ethz.ch