- 1Dan F. Smith Department of Chemical and Biomolecular Engineering, Lamar University, Beaumont, TX, United States
- 2Gas and Fuels Research Center, Texas A&M Engineering Experiment Station, College Station, TX, United States
- 3Vishwamitra Research Institute, Crystal Lake, IL, United States
- 4The Dow Chemical Company, Lake Jackson, TX, United States
- 5Emerson Automation Solutions, Baton Rouge, LA, United States
Due to environmental regulations continually reducing emission quantity allowed over time, there is a growing need for adaptable and feasible environmental monitoring, such as emission, wastewater quality, and air pollution monitoring, for the process industry (and surrounding communities). Alternative environmental monitoring and process monitoring technologies based on industrial internet of things (IIoT) and artificial intelligence (AI) enable the process industry to take a proactive approach toward the environment and asset integrity management. The monitoring devices can be deployed in a stationary or dynamic manner. In this study, the emerging trend and various applications of IIoT and advanced data analytics methodologies in environmental monitoring are reviewed. An example showing challenges and research needs in sensor placement is given. Future directions in technology, regulation, and application have been discussed as well.
Introduction
Advances in applications that can utilize big data and analytics are becoming more widespread within the manufacturing sector. New approaches, including information distribution via the industrial internet of things (IIoT) and big data analytic methodologies, allow processing facilities to analyze data in real time and act to correct abnormal operations or mitigate asset failures. In specific, the widespread applications of sensors combined with large data streams and key performance indicator (KPI) dashboards enable streaming collection and visualization of information (analyzed data) that can be utilized for monitoring and taking actions. The data stream management can be used for setting alarms, triggering control systems, activating mitigation actions, monitoring, and reporting for regulatory authorities and internal applications. As a result, the IIoT platform facilitates the integration of sensors, data analytics, networks, real-time monitoring, and informed decision making. Therefore, IIoT serves as an essential component of the emerging digital transformation by providing minimally invasive remote asset operating information.
In recent years, there emerged a new trend of using digital technology to better monitor pollutants as a means of improving overall environmental quality. IIoT devices have been widely applied and many success stories have been reported. Martínez et al. (2020) reported a low cost integrated IIoT system for monitoring nitrate and nitrite in water with a novel ion chromatograph detection method. Zhu et al. (2020) proposed an IIoT-based automation system built on top of the legacy wastewater treatment plant (WWTP) automation system to enable remote monitoring and control. Rezwan et al. (2019) developed a minimalist model for monitoring working conditions of a WWTP, as well as its key performance indicators such as PH, temperature, and turbidity. Edmundo Guerra et al. (2020) developed an unmanned aerial vehicle (UAV) platform to capture samples from the open air basin of a WWTP. In addition to monitoring the quality of water, monitoring and controlling the pollutants in air, such as volatile organic compounds (VOCs) and hazardous air pollutants (HAPs), are also very important for the process industries and surrounding communities. Violation of emission can lead to void of permit for production. The environmental regulations are continually reducing the quantity allowed over time, therefore increasing the demand for monitoring air and water quality proactively, efficiently, and at low cost. In addition, IIoT technologies have been widely used in asset management, even for assets buried underground. For example, undetected corrosion or erosion of the pipelines can lead directly to catastrophic events. Using IIoT technology, Emerson’s pipeline management solutions remotely monitor the real-time progression of corrosion and erosion (Pipeline Integrity & Leak, 2021).
Traditionally, pollutants in air or water are monitored via stationary monitoring stations, or in some instances, infrequent manual sampling. Due to the high cost of sensors as well as maintenance of a monitoring network, sensors can only be installed at a limited number of locations that may lead to sparse spatial and temporal coverage, resulting in delayed and inaccurate measurements of pollutants. As the IIoT technologies remove the constraints of physical connections between sensors and the processing center, it enables the new environmental quality monitoring methodologies based on alternative water pollutant and emission monitoring and dynamic sensing methodologies. Alternative monitoring technologies, such as optical and remote sensing instruments and wireless sensors, can monitor pollutants from a specific equipment/area/unit/plant in a timely manner. Process monitoring technologies based on IIoT can use big data approaches and sophisticated cloud computing to provide solutions for operation and emission management issues.
In this study, we will highlight the emerging trends of applying IIoT in environmental monitoring, specifically in the areas of advanced data analytics and sensor placement. The future directions of such applications, including the methodologies, hardware, and regulations, will be discussed as well.
Advancement in Data Analytic Methodologies
The data analytic methods applied in environmental monitoring are dictated by the diverse data generated from different IIoT sensors and sensor networks. In general, their applications can be classified into two categories:
1) Data processing for alternative emission monitoring technologies, including various optical and remote sensing (ORS) devices and wireless sensor network. The representative ORS technologies include Fourier transform infrared spectroscopy (FTIR), tuneable diode laser, ultraviolet differential optical absorption spectroscopy (UV-DOAS), differential absorption lidar monitoring (DIAL), cavity ring-down spectroscopy, particulate matter LiDAR, thermal infrared camera, solar occultation flux (SOF) monitoring, and hyperspectral imaging. Satellite instruments have also been used to monitor the emissions of O3, NO2, CO, HCHO, SO2, NH3, volatile organic compounds (VOCs), and even greenhouse gases CO2 and CH4 (Handbook, 2018; Kim et al., 2020). To analyze these data of diverse types, such as images and spectrum, deep learning methodologies are employed (Zeng et al., 2016; Kamruzzaman et al., 2018; Mishra et al., 2019). In addition, deep learning methods are also applied to gain improved prediction accuracy for monitoring complicated processes (Yan et al., 2020).
2) “Soft sensors,” that is, mathematical models based on past data for predicting emissions using current process data. These soft sensors are developed via a wide range of machine learning methodologies, including multivariate analysis (Garces and Sbarbaro, 2011), support vector machine (Garces and Sbarbaro, 2011), artificial neural network (O’Connor et al., 2012), and so on. In the area of emission monitoring, one example is ABB-developed predictive emission monitoring systems (PEMSs), which can estimate all the most common pollutants from process industry: NOx, SO2, CO, CO2, flue gas flow, etc., based on process data such as temperature, pressure, and flow rate. It has been successfully applied to complex process units, such as fluid catalytic cracking (FCC) and sulfur recovery units (SRU), in refineries (Bonavita and Ciarlo, 2014; Ciarlo, 2015). A PEMS uses an artificial neural network to build the prediction models. A schematic representation of the ANN is depicted in Figure 1.
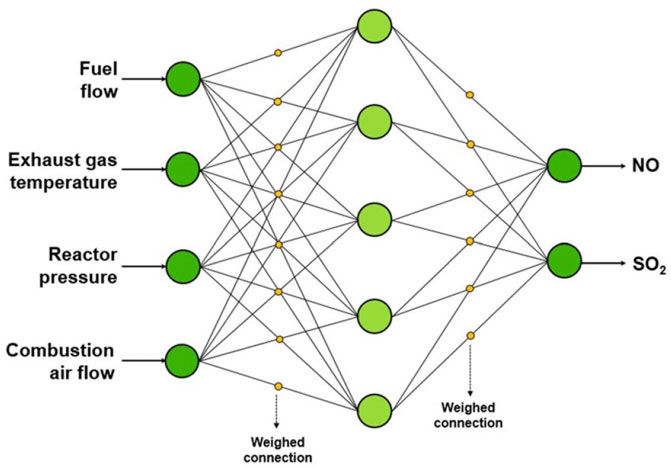
FIGURE 1. Feed forward neural network schematic (Ciarlo, 2015).
Another example is predictive flare control. Industrial flares are used to burn off the unwanted gas during start up, shutdown, emergency, and normal production. If not combusted completely, large quantities of undesired intermediate products or incomplete combustion products, such as radical precursors (e.g., formaldehyde), soot precursors (acetylene, ethylene, benzene), carbon monoxide, and soot, in addition to unburned hydrocarbons. The Environment Protection Agency (EPA) Refinery Sector Rule (RSR) (USEPA, 2020) emphasizes smokeless combustion with combustion efficiency (CE)
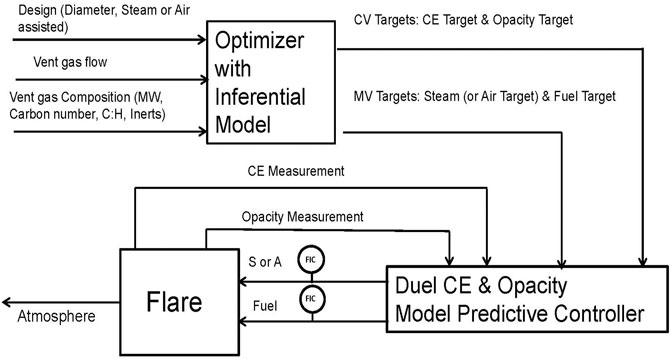
FIGURE 2. Schematic of predictive flare control (Lou et al., 2017).
In the past, the flare operators have the tendency of adding too much assist steam or assist air to suppress smoke at the expense of DRE and CE. Then, in order to comply with the requirement of NHVcz and NHVdil, an excessive amount of supplemental fuel (usually natural gas) has to be added. The addition of steam, assist air, and supplemental fuel increases the flare operating cost. Actually, the value of NHVcz increased with less assist steam. Flaring can meet compliance with less assist steam, assist air, and supplemental fuel. Case studies were conducted using historical data. It was found that operating cost can be saved significantly, with accurate prediction of the flaring performance.
By combining alternative emission monitoring technologies with process monitoring technologies based on IIoT, such as PEMS and the predictive flare control method, the process industry can utilize process data and emission data seamlessly to reduce emissions, minimize potential risks, and achieve operational excellence.
The “connected plants” that use sensors and digital assets to monitor and control process for product quality and process safety already generate significant amount of valuable data. The data can be used for plant optimization and asset utilization. The integration of additional sensors for environmental monitoring is complementary for the data pool. Also, the process knowledge and simulations can transform the data to actionable insight for pervasive monitoring. For instance, emission monitoring for a stack of a refinery kiln can be tied in with fuel gas flow rate, other burner parameters and data that would be used by data reconciliation algorithms for predictive maintenance, and any troubleshooting for reducing the emission or burner maintenance (e.g., decoking).
Advancement in Dynamic Sensing
In addition to alternative emission monitoring, IIoT can be easily integrated with dynamic sensing, a new monitoring technique that adjusts the locations of portable sensors in real time to measure the dynamic changes in air and water quality. Unlike stationary monitoring stations, real-time sensor placement optimization algorithm will allow the assessment of spatial–temporal variability of pollution. While the number and emission intensity of sources determine the volume of the chemical species, it is the flow, temperature, humidity, sunlight, etc., that determines the fate and transport of the species. The resulting concentrations of species change spatially and temporally because of these physical and chemical processes. Therefore, dynamic sensing is not only more economic but also more efficient for environment monitoring.
Automobiles and drones are typical platforms carrying portable sensors. Recently, the US government approved regulations for use of commercial drones. With the advancement of drone technologies, it is possible to measure the dynamic changes in air or water quality with portable sensors, which change positions in real time (de Almeida Oliveira and Godoy, 2016; Diwekar and Mukherjee, 2017; Tmušić et al., 2020). Theories and framework developed in sensor placement have been widely applied for monitoring water bodies, water security networks, advanced power systems, and other infrastructure issues such as security of the power grid, pollutant release from process industries, and so on.
Sensor placement and route planning frameworks are important to fully realize the potential of IIoT platform with mobile sensor systems. It typically consists of a model predicting the spatiotemporal distribution of pollutants and an optimizer identifying optimal sensor locations/routes. The AERMOD developed at US EPA can predict the fate and transport of the pollutants (Fox, 2021). An advanced version of AREMOD, stoAERMOD, was developed at Vishwamitra Research Institute (VRI), which can model the distribution of pollutants by incorporating stochastic inputs in terms of both sources as well as meteorological data, for example, wind speed and temperature. Spatiotemporal Gaussian processes have also been employed to model the environmental field (Sun et al., 2019). Due to the tremendous computational time required, algorithms developed for real-time monitoring of the fate and transport of chemical species have limited success. Prof. Diwekar and her team have developed an efficient and robust method for solving the dynamic sensor placement problem using Better Optimization of Nonlinear Uncertain Systems (BONUS) algorithm (Sahin and Diwekar, 2004). Nguyen et al. (2018) proposed a practical and feasible polynomial algorithm for sensor placement based on Gaussian processes.
An example of using dynamic sensing framework for monitoring the pollutant distribution as well as its exposure to human population in the city of Atlanta has been reported by Mukherjee et al. (2020). In this example, two mobile sensors are employed for this task and their locations are planned in real time according to the changes of factors such as diurnal fluctuation of the number of automobiles, wind speed, and temperature. The objective is to determine the optimal locations of a network of sensors so that maximum observability of the pollutants can be achieved. The observability of the sensor networks is measured with Fisher information (FI), which is a probabilistic function. (Lee and Diwekar, 2012). The spatiotemporal sensor positions in real time can be solved as an optimization problem, solved for different time periods through maximizing FI subject to the fate and transport models of the pollutants under the weather, as well as traffic uncertainty.
The dynamic location of the sensors at five different time of the day are shown in Figure 3.
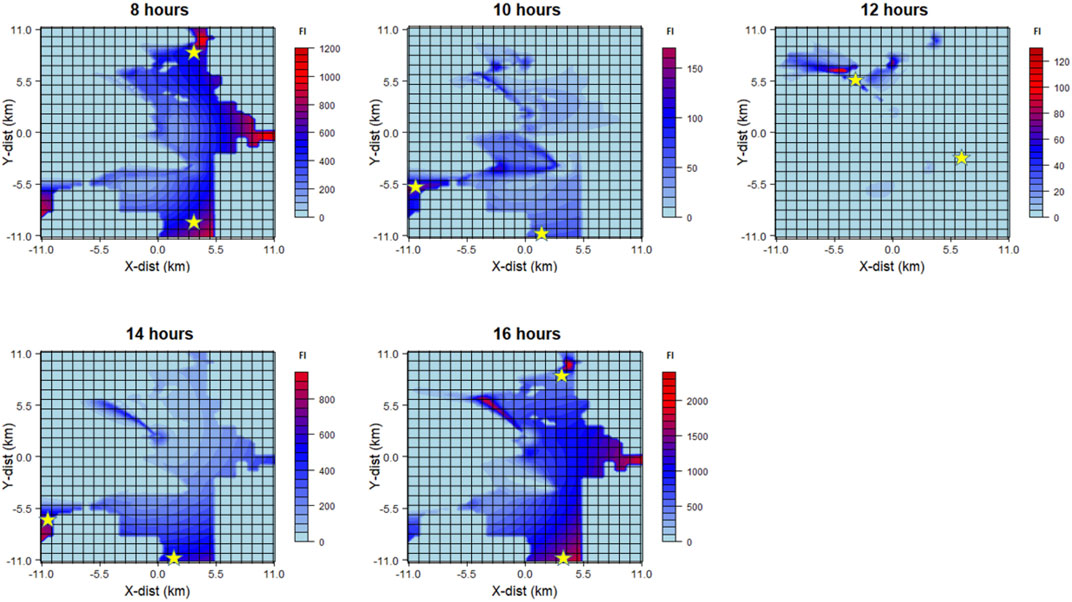
FIGURE 3. Optimal sensor placement for Atlanta, Georgia, using Fisher information (FI) based on particulate matter (PM) concentration, at different hours of the day. The x and y coordinates represent the distance from the center of the city and the color represents the intensity of FI.
Algorithm development for real-time spatiotemporal sensor placement for environmental monitoring is a challenging task. Several factors caused by the properties of the environment that include weather uncertainties and the sensing phenomena need to be considered (Schoellhammer et al., 2006). The uncertainty associated with the system demands a stochastic optimization method. Portable low-cost sensors for dynamic sensing is a new area of research for environmental monitoring. Drone-based monitoring is the future of dynamic monitoring of the environment. In case of a relatively small controlled system, dynamic environmental monitoring has also been proposed using intelligent tendril robots (Nahar, 2017). Traditionally, optimal sensor location is determined based on pollutant concentration. Further investigations can be made for the development of sensor placement algorithms in applications such as target tracking and identification. Also, algorithm for exposure assessment needs to be developed for actual impact assessment. Presently, optimal sensor placement for environmental monitoring is limited to two dimensional analysis. Potential research in the future may include taking into consideration three dimensional analysis. Three dimensional spatial analysis can potentially improve the accuracy of prediction. Sophisticated machine learning algorithms that optimize sensor position in an intelligent manner is a promising area of research that needs attention in the future (Nahar, 2017; Semaan, 2017; Wang et al., 2019).
Future Direction
In addition to the recent progress in data analysis and sensor placement for IIoT in environmental monitoring, there are some new directions worth highlighting in terms of technology, application, and regulation.
As more and more IIoT sensors can collect audio, image, video, text, and even social media data for predictive maintenance, risk reduction, trend prediction, etc., the variety of the data sources needs to be addressed. Therefore, sensor fusion or information fusion methodologies are applied on top of the data analytic methodologies for individual data schemes to combine multi-rate, multi-resolution, and heterogeneous data together and improve the robustness of inference. Wang and Chiang (2019) proposed a judicious fusion framework for combining multi-rate sensor data with different qualities to monitor chemical plant performance. Qin et al. (2012) developed a sensor fusion–based monitoring system consisting of ultraviolet–visible spectroscopy UV/VIS spectrometer and turbidimeter to monitor wastewater quality. A sensor fusion application combining images and process sensor data for monitoring toxin level in WWTP has been reported (Strelet et al., 2021). Schneider et al. (2017) achieved accurate near real-time air quality monitoring from multiple low-cost sensors using geostatistic-based sensor fusion methodology that merges sensor data and predictions of urban-scale air quality data. ABB has also reported a fault-resilient PEMS framework to identify and reconcile the faulty sensor readings for emission monitoring (Angelosante et al., 2018).
The increasing number of sensors also casts higher demand for data transferring and processing. Edge computing is a distributed information technology (IT) architecture in which client data are processed at the periphery of the network, as close to the originating source as possible. Introducing edge computing in IIoT can significantly reduce the decision-making latency, save bandwidth resources, and to some extent, protect privacy. Applications include, but are not limited to, smart grids, manufacturing, workplace safety, intelligent connected vehicles (ICV), and smart logistics. (Qiu et al., 2020). Many cloud computing providers provide edge computing platforms. For example, AWS FreeRTOs (FreeAvailable at: h, 2022) is an open source, real-time operating system for microcontrollers used in IIoT.
Regarding the innovations in hardware, microcontrollers have been used to make small, low power edge devices, which are easy to program, deploy, secure, connect, and manage. For example, Andino Systems provides innovative solutions for the usage of Raspberry Pi PCs in industrial environments.
Cyber security is another area that attracts research interest. To widely apply IIoT system, it is essential to address the concern about information security, the consequences it led to, forgery, and leaked information. Recently, blockchain technology that enables trusted record transactions has been applied to environmental monitoring with IIoT system to encrypt the collected and transferred information (Han et al., 2019). With the development of information security, such concerns from the process industry will be mitigated. The increasing acceptance of cloud computing services in the process industry adds more optimism to the future of IIoT in environmental monitoring. The former faced the concern of information security as well but addressed the concern with advancement in security technologies. A similar process can be expected in the adoption of IIoT.
In addition to the technological progress, regulations and economic incentives are even more influential factors dictating the application of IIoT in environmental monitoring. This study has been focused on monitoring sensors (stationary and dynamic), data collection, data analytics, and the IIoT transfer of data and information to databases and KPI dashboards. What has not been covered is what action or regulations should be considered from this new insight into water and air pollutants. Any regulation should have sufficient data and analysis to the extent of the problem and the regulations to be applied to mitigate harmful pollutants from the environment must be considered. In addition, any regulations would drive future research and development into lower cost and dynamic sensors for added flexibility to monitor multiple areas.
One good practice to highlight is the “US EPA Citizen Science Program” that collaborates with the public and EPA to connect on environmental science and protection (Han et al., 2019). The program utilizes a large amount of inexpensive sensors to identify research questions, collect and analyze data, interpret results and suggest recommended actions, make new discoveries, and develop technologies and applications—all to understand better and solve environmental challenges.
The pace of industry embracing the IIoT technologies depends on how big the incentives are. One of the common concerns is the return to investment ratio, which is common in all artificial intelligence–related applications in the process industry. As forecasted by Gartner, 50% of the AI investment will be measured by return on investment (Goasduff, 2022). Some promising potential applications would be the processes facing strict environmental regulations. With the real-time, precise, and robust measurements from the IIoT system and timely corrective actions enabled, such processes can be operated in regimes closer to the regulation limit but with higher profitable margin. The lower cost and easier installation of the IIoT system compared to the wired system are also incentives for its application. Another potential area for application is safety. One example is H2S monitoring within a processing facility and along the fence line to ensure this deadly toxic gas is identified and alerted before harming anyone (Flame and Gas Detection, 2022).
Conclusion
The new monitoring technologies on IIoT are now available and feasible to capture environmental and process data to perform analysis and examine corrective actions if warranted or regulated. They enable real-time data and analysis to provide valuable information about environmental issues, when they occur and where, and for how long. The new trend of applying IIoT for asset management and environmental monitoring is critical to the process industry, surrounding communities, and regulatory agencies.
With the advancement in wireless sensors, microcontrollers, 5G or 6G in the future, and data analytic methodologies to handle different data types, new frontiers beyond the process industry of applying IIoT techniques will continue to emerge.
Author Contributions
All authors listed have made a substantial, direct, and intellectual contribution to the work and approved it for publication.
Funding
This research was partially supported by Lamar University Center for Midstream Management and Science (CMMS).
Conflict of Interest
Author ZW was employed by the company The Dow Chemical Company. Author TO was employed by the company Emerson Automation Solutions.
The remaining authors declare that the research was conducted in the absence of any commercial or financial relationships that could be construed as a potential conflict of interest.
Publisher’s Note
All claims expressed in this article are solely those of the authors and do not necessarily represent those of their affiliated organizations, or those of the publisher, the editors and the reviewers. Any product that may be evaluated in this article, or claim that may be made by its manufacturer, is not guaranteed or endorsed by the publisher.
References
Angelosante, D., Guerriero, M., Ciarlo, G., and Bonavita, N. (2018). “A Sensor Fault-Resilient Framework for Predictive Emission Monitoring Systems,” in 2018 21st International Conference on Information Fusion (FUSION), 10 - 13 July 2018. (Cambridge, UK: IEEE), 557–564.
Bonavita, N., and Ciarlo, G. (2014). Inferential Sensors for Emission Monitoring: an Industrial Perspective. Front. Environ. Eng. (Fiee) 3.
Ciarlo, G. C. F. (2015). Predictive emission Monit. Syst. (Pems) Deploying software-based emission Monit. Syst. refining Process. Available at: https://library.e.abb.com/public/310e4b7c45a742578722e61894999b4e/AT_ANALYTICAL_029-EN_A.pdf (Accessed February 28, 2022).
de Almeida Oliveira, T., and Godoy, E. P. (2016). ZigBee Wireless Dynamic Sensor Networks: Feasibility Analysis and Implementation Guide. IEEE Sensors J. 16 (11), 4614–4621. doi:10.1109/jsen.2016.2542063
Diwekar, U., and Mukherjee, R. (2017). Optimizing Spatiotemporal Sensors Placement for Nutrient Monitoring: a Stochastic Optimization Framework. Clean. Techn Environ. Pol. 19 (9), 2305–2316. doi:10.1007/s10098-017-1420-3
Flame and Gas Detection (2022) Available at: https://www.emerson.com/en-us/automation/measurement-instrumentation/flame-gas-detection (accessed 02 11, 2022).
Fox, T. 2021 EPA White Papers on Planned Updates to AERMOD Modeling System Available at: https://www.epa.gov/scram/aermod-modeling-system-development (accessed 12/16/2021).
FreeRTOS. Available at: https://aws.amazon.com/freertos/ 2022 (accessed 02/11/2022).
Garces, H., and Sbarbaro, D. (2011). Outliers Detection in Environmental Monitoring Databases. Eng. Appl. Artif. Intelligence 24 (2), 341–349. doi:10.1016/j.engappai.2010.10.018
Goasduff, L. 3 Barriers to AI Adoption.2022 Available at: https://www.gartner.com/smarterwithgartner/3-barriers-to-ai-adoption (accessed 01/29/2022).
Guerra, E., Bolea, Y., Gamiz, J., and Grau, A. (2020). Design and Implementation of a Virtual Sensor Network for Smart Waste Water Monitoring. Sensors 20 (2), 358. doi:10.3390/s20020358
Han, Y., Park, B., and Jeong, J. (2019). A Novel Architecture of Air Pollution Measurement Platform Using 5G and Blockchain for Industrial IoT Applications. Proced. Comput. Sci. 155, 728–733. doi:10.1016/j.procs.2019.08.105
Handbook, E. (2018). Optical and Remote Sensing for Measurement and Monitoring of Emissions Flux of Gases and Particulate Matter. US Environ. Prot. Agency, Off. Air Qual. Plann. Stand. Air Qual. Assess. Division: Res. Triangle Park, NC, USA. Available at: https://www.epa.gov/sites/default/files/2018-08/documents/gd-52v.2.pdf (Acessed February 28, 2022).
Kamruzzaman, J., Wang, G., Karmakar, G., Ahmad, I., and Bhuiyan, M. Z. A. (2018). Acoustic Sensor Networks in the Internet of Things Applications. Future Generation Comput. Syst. 86, 1167–1169. doi:10.1016/j.future.2018.05.019
Kim, J., Jeong, U., Ahn, M.-H., Kim, J. H., Park, R. J., Lee, H., et al. (2020). New era of Air Quality Monitoring from Space: Geostationary Environment Monitoring Spectrometer (GEMS). Bull. Am. Meteorol. Soc. 101 (1), E1–E22. doi:10.1175/bams-d-18-0013.1
Lee, A. J., and Diwekar, U. M. (2012). Optimal Sensor Placement in Integrated Gasification Combined Cycle Power Systems. Appl. Energ. 99, 255–264. doi:10.1016/j.apenergy.2012.04.027
Lou, H., Gai, H., and Smith, D. F. (2019). “Emission Monitoring and Plant Integrity Enhancement,” in 2019 AIChE annual meeting, Orlando, FL.
Lou, H. H. W. A., Chen, D., Li, X. C., Martin, C., Alphones, A., and Damodara, V. (2017). “In Optimization for Flare Operation,” in 2017 AIChE Spring Meeting and 13th Global Congress on Process Safety, San Antonio, TX, March 28, 2017.
Martínez, R., Vela, N., el Aatik, A., Murray, E., Roche, P., and Navarro, J. M. (2020). On the Use of an IoT Integrated System for Water Quality Monitoring and Management in Wastewater Treatment Plants. Water 12 (4), 1096. doi:10.3390/w12041096
Mishra, B. K., Thakker, D., Mazumdar, S., Simpson, S., and Neagu, D. (2019). “Using Deep Learning for IoT-Enabled Camera: A Use Case of Flood Monitoring,” in 2019 10th International Conference on Dependable Systems, Services and Technologies (DESSERT, Leeds, UK, 5-7 June 2019 (IEEE), 235–240.
Mukherjee, R., Diwekar, U. M., and Kumar, N. (2020). Real-time Optimal Spatiotemporal Sensor Placement for Monitoring Air Pollutants. Clean. Techn Environ. Pol. 22 (10), 2091–2105. doi:10.1007/s10098-020-01959-z
Nahar, D. S. (2017). Dynamic Environmental Monitoring Using Intelligent Tendril Robots. Clemson: Clemson University.
Nguyen, L. V., Hu, G., and Spanos, C. J. (2018). Efficient Sensor Deployments for Spatio-Temporal Environmental Monitoring. IEEE Trans. Syst. Man, Cybernetics: Syst. 50 (12), 5306–5316.
O’Connor, E., Smeaton, A. F., O’Connor, N. E., and Regan, F. (2012). A Neural Network Approach to Smarter Sensor Networks for Water Quality Monitoring. Sensors 12 (4), 4605–4632.
Pipeline Integrity & Leak Detection.2021 Available at: https://www.emerson.com/en-us/industries/automation/oil-gas/pipeline-integrity-leak-detection (accessed 12/16/2021).
Qin, X., Gao, F., and Chen, G. (2012). Wastewater Quality Monitoring System Using Sensor Fusion and Machine Learning Techniques. Water Res. 46 (4), 1133–1144. doi:10.1016/j.watres.2011.12.005
Qiu, T., Chi, J., Zhou, X., Ning, Z., Atiquzzaman., M., and Wu, D. O. (2020). Edge Computing in Industrial Internet of Things: Architecture, Advances and Challenges. IEEE Commun. Surv. Tutorials 22 (4), 2462–2488.
Rezwan, S., Ishtiak, T., Rahman, R., Rahman, H. A., Akter, M., Ratul, H. A., et al. (2019). “A Minimalist Model of IoT Based Sensor System for Sewage Treatment Plant Monitoring,” in 2019 IEEE 10th Annual Information Technology, Electronics and Mobile Communication Conference (IEMCON), Vancouver, BC, Canada, 17-19 Oct. 2019 (IEEE), 0939–0945.
Sahin, K. H., and Diwekar, U. M. (2004). Better Optimization of Nonlinear Uncertain Systems (BONUS): A New Algorithm for Stochastic Programming Using Reweighting through Kernel Density Estimation. Ann. Operations Res. 132 (1), 47–68. doi:10.1023/b:anor.0000045276.18995.c8
Schneider, P., Castell, N., Vogt, M., Dauge, F. R., Lahoz, W. A., and Bartonova, A. (2017). Mapping Urban Air Quality in Near Real-Time Using Observations from Low-Cost Sensors and Model Information. Environ. Int. 106, 234–247. doi:10.1016/j.envint.2017.05.005
Schoellhammer, T., Wong, J., Hansen, M., Potkonjak, M., and Estrin, D. (2006). Sensor Deployment Using Interleaved Experimentation. modeling, optimization.
Semaan, R. (2017). Optimal Sensor Placement Using Machine Learning. Comput. Fluids 159, 167–176. doi:10.1016/j.compfluid.2017.10.002
Strelet, E., Wang, Z., Peng, Y., Castillo, I., Rendall, R., Braun, B., et al. (2021). Multi-source Heterogeneous Data Fusion for Toxin Level Quantification. IFAC-PapersOnLine 54 (3), 67–72. doi:10.1016/j.ifacol.2021.08.220
Sun, C., Yu, Y., Li, V. O. K., and Lam, J. C. K. (2019). Multi-type Sensor Placements in Gaussian Spatial fields for Environmental Monitoring. Sensors 19 (1), 189. doi:10.3390/s19010189
Tmušić, G., Manfreda, S., Aasen, H., James, M. R., Gonçalves, G., Ben-Dor, E., et al. (2020). Current Practices in UAS-Based Environmental Monitoring. Remote Sensing 12 (6), 1001.
USEPA, (2020). Petroleum Refinery Sector Risk and Technology Review and New Source Performance Standards. 40 CFR, 63.670. Available at: https://www.govinfo.gov/content/pkg/FR-2020-02-04/pdf/2020-01108.pdf (Accessed February 28, 2022).
Wang, Z., Li, H. X., and Chen, C. (2019). Reinforcement Learning-Based Optimal Sensor Placement for Spatiotemporal Modeling. IEEE Trans. Cybern 50 (6), 2861–2871. doi:10.1109/TCYB.2019.2901897
Wang, Z., and Chiang, L. (2019). Monitoring Chemical Processes Using Judicious Fusion of Multi-Rate Sensor Data. Sensors 19 (10), 2240. doi:10.3390/s19102240
Yan, W., Xu, R., Wang, K., Di, T., and Jiang, Z. (2020). Soft Sensor Modeling Method Based on Semisupervised Deep Learning and its Application to Wastewater Treatment Plant. Ind. Eng. Chem. Res. 59 (10), 4589–4601. doi:10.1021/acs.iecr.9b05087
Zeng, Y., Morris, J., and Dombrowski, M. (2016). Multi-spectral Infrared Imaging System for Flare Combustion Efficiency Monitoring. Google Patents.
Keywords: industrial internet of things (IIoT), advanced manufacturing (AM), emerging technologies, environmental monitoring, artificial intelligence
Citation: Lou HH, Mukherjee R, Wang Z, Olsen T, Diwekar U and Lin S (2022) A New Area of Utilizing Industrial Internet of Things in Environmental Monitoring. Front. Chem. Eng. 4:842514. doi: 10.3389/fceng.2022.842514
Received: 23 December 2021; Accepted: 15 February 2022;
Published: 10 March 2022.
Edited by:
Qinghua He, Auburn University, United StatesReviewed by:
Rajagopalan Srinivasan, Indian Institute of Technology Madras, IndiaCopyright © 2022 Lou, Mukherjee, Wang, Olsen, Diwekar and Lin. This is an open-access article distributed under the terms of the Creative Commons Attribution License (CC BY). The use, distribution or reproduction in other forums is permitted, provided the original author(s) and the copyright owner(s) are credited and that the original publication in this journal is cited, in accordance with accepted academic practice. No use, distribution or reproduction is permitted which does not comply with these terms.
*Correspondence: Zhenyu Wang, endhbmcxMkBkb3cuY29t; Tim Olsen, VGltLk9sc2VuQEVtZXJzb24uY29t; Helen H. Lou, aGVsZW4ubG91QGxhbWFyLmVkdQ==