- Process and Systems Engineering Research Center (PROSYS), Department of Chemical and Biochemical Engineering, Technical University of Denmark, Kongens Lyngby, Denmark
This manuscript describes the conceptual process design of an integrated xylitol biorefinery with value-added co-products. Based on an existing three-step framework, the main product of a second-generation integrated biorefinery is chosen in the first stage. Based upon this, other decisions as the feedstock and value-added co-products are made. All relevant unit operations for the process are introduced. An initial superstructure with all potential process alternatives is composed of all introduced models. In the second step of the framework, a global sensitivity analysis is performed, firstly with coarse sampling to determine all viable flowsheet options and secondly with fine sampling to determine the most sensitive operational variables. As a result of the sensitivity analysis, most of the flowsheet options in the initial superstructure are not feasible. Based on these results, flowsheet sampling with the five most sensitive operational variables is performed to create surrogate models. In the scope of this work, three types of surrogate models are benchmarked against each other. Regarding the results of the superstructure optimization, firstly, it becomes apparent that the production of biokerosene does not contribute significantly to the net present value of the biorefinery. Furthermore, reducing the number of unit operations in the downstream processing leads to lower capital expenditures, but it lowers the product yield. Lastly, most flowsheets are economically feasible, indicated by a positive net present value. Based on this result, the most promising candidate process topology is subjected to the third step of the framework, including uncertainty in capital expenditure and operational expenses according to their estimations and uncertainties in the product prices. As a result, the net present value of the flowsheet turns negative, indicating that the high uncertainties for the expenditure and the expenses do not allow for an economically feasible operation. Lastly, the analysis of conceptually designed process flowsheets based on Monte Carlo sampling shows failure rates, with the NPV falling below the break-even point, of around 60% probability or higher. Based on these results, an economically feasible construction and operation of a xylitol biorefinery seems unlikely. Further ways to improve the metrics are elucidated.
Introduction
Biorefineries are considered an essential part of a strategy towards more sustainable production patterns of fuels and chemicals as demanded by the 2030 Sustainability Agenda of the United Nations (United Nations, 2015). In general, a biorefinery is a production concept for the conversion of biomass into different products, e.g., xylitol (Cherubini, 2010). In this regard, a multitude of realizations of this biorefinery concept have been investigated and published in the past decades. As diverse as the realizations are, the classification of biorefinery setups is not standardized and varies depending on the type and generation of utilized feedstock, the utilized conversion technologies, the obtained product type and number, and the integration level (Cherubini, 2010; Bastidas-Oyanedel and Schmidt, 2019; Ubando et al., 2020). Nonetheless, all of the elaborated concepts have in common that their sustainability potential is compelling, but their economic viability is generally described as challenging to achieve (Ubando et al., 2020).
Xylitol as a product has gained attraction throughout research for several decades. Xylitol is an excellent sugar substitute with many beneficial health properties, as around 40% fewer calories than sucrose, anticariogenic properties, and a low glycemic index, making it perfectly suitable for diabetic nutrition (Da Silva and Chandel, 2012). Furthermore, several studies indicate a potential use as building bock chemical (Hernández-Pérez et al., 2019; Delgado Arcaño et al., 2020). Due to the high interest and potentially high product prices, the US Department of Energy declared xylitol one of the top 12 chemicals to be produced in a biorefinery already in 2004 (Werpy and Petersen, 2004).
To this end, all major xylitol producers employ a chemical production process with the hemicellulosic fraction of lignocellulosic biomass as feedstock. According to Delgado Arcaño et al. (2020), the production process consists of four steps, namely 1) the biomass pretreatment, 2) the purification of the obtained xylose, 3) the chemical conversion of xylose to xylitol, and 4) the purification of the produced xylitol. Both the purification steps and the temperature and pressure conditions required for the conversion process induce high costs, which explains the comparatively high product price of xylitol (Hernández-Pérez et al., 2019).
An alternative production route for xylitol is the fermentation with suitable organisms in a biotechnological process. A significant number of research publications on the biotechnological production with either wild-type or genetically modified microorganisms exist. However, all of them do point out that there is no consensus on any economically viable full-scale production process, and further conceptual research needs to be performed on this, primarily focusing on an efficient pretreatment technology, robust cell factories, and an optimized downstream process (Albuquerque et al., 2014; Rao et al., 2016; Dasgupta et al., 2017; Hernández-Pérez et al., 2019). Both the chemical production route and the biotechnological production route are found on similar pretreatment unit operations, given the fact that also in the biotechnological process, the feedstock is lignocellulosic biomass. However, a significant advantage of the biotechnological route is the resilience towards impurities, reducing the effort to purify the hemicellulosic hydrolysate from the pretreatment (Hernández-Pérez et al., 2019).
Due to these considerations, this work focuses on a multi-product second-generation integrated biorefinery, referring to lignocellulosic biomass as second-generation feedstock. Both the approach of producing multiple products from biomass to utilize the feedstock to a maximum amount and the optimization of heat and mass integration are prone to augment the economic viability, which makes this concept most promising both regarding the sustainability and the economic aspect (Bastidas-Oyanedel and Schmidt, 2019; Ubando et al., 2020). Nonetheless, the question of which products to co-produce in the biorefinery setup, which feedstock to utilize, and how to introduce efficient ways to integrate the process remains challenging. Hence, this demands a conceptual design approach to ensure an optimal process design of the biorefinery to answer the conceptional underlying question regarding the economic feasibility of such biorefinery concepts under given technological and economic conditions.
Concerning conceptual process design, there exist several schools of thinking. In the S3O framework, a synergistic approach is followed to best integrate expert knowledge, optimization approaches, and simulation-based strategies to leverage synergies for the conceptual design of bioprocesses in three steps (Vollmer et al., 2021a). The framework is applied for the case study of designing a xylitol biorefinery with value-added co-products in this manuscript. In the first step, the main product (xylitol) and value-added co-products (succinic acid, biokerosene, heat) are selected after thorough reasoning, together with the potential feedstock (wheat straw) and potential unit operations that are suitable for the biotechnological production of xylitol. In the second step, key performance indicators, e.g., the net present value (NPV) of the plant, are used as the objective function to maximize the economic potential of the plant by finding the most suitable operational conditions and process configuration. Lastly, in the third step, the found process and operational conditions are optimized under uncertainty to consolidate the process design. Based on the results of the second and third step of the framework, a techno-economic analysis (TEA) is performed. The TEA involves Monte Carlo-based uncertainty analysis to quantify the influence of different economic and operational factors on the economic feasibility of the process. The major novelty of this work in comparison to similar studies is the integration of mechanistic models via the synergistic optimization-based framework for the conceptual process design and the use of detailed data for the equipment to obtain a realistic estimate of the capital expenditures and operational expenses and detailed market prices for the techno-economic analysis of the biotechnological production of xylitol (Franceschin et al., 2011; Mountraki et al., 2017; Giuliano et al., 2018).
The remainder of the paper is structured as follows: theoretical background is provided about the product xylitol and its production process in a potential biorefinery setup, followed by an in-depth description of all unit operations and their respective mechanistic model, which is used in the process design framework. Afterward, the structure and functionalities of the framework are elucidated in detail and provide additional considerations regarding the costing and sizing of equipment. Lastly, the specific calculations regarding the techno-economic and the sustainability analysis are introduced. In Results, the results are presented. In Conclusions and Future Research, conclusions are drawn, and an outlook to future research is given.
Materials and Methods
Feedstock and Products
Feedstock
In general, lignocellulosic biomass consists of three main fractions, namely hemicellulose, cellulose, and lignin. All three possess a polymer structure and are composed of typical monomers: Hemicellulose consists mainly of pentose sugars, mostly xylose, and smaller amounts of arabinose (Vollmer et al., 2021c). Cellulose consists mainly of glucose monomers, while lignin is a mostly heterogeneous macromolecule consisting of the three monolignols p-coumaryl alcohol, coniferyl alcohol, and sinapyl alcohol. Their fractions largely depend on the type of feedstock, and theoretically, all of them can be converted to different products. Wheat straw, as the considered feedstock in this case study, has a comparatively high fraction of hemicellulose sugars, which indicates a potentially high yield of xylose monomers.
Xylitol
Xylitol (C5H12O5) is the sugar alcohol of xylose, an aldopentose, and naturally occurs in woods and other crop plants (Albuquerque et al., 2014). It is highly soluble in water and has a negative heat of solution. As mentioned in Introduction, the increasing interest in xylitol is also reflected by the growing market size of xylitol. With a market size of 670 Mio USD at 161.5 mio MT produced in 2013, this is supposed to grow to a volume of 1.15 Bio USD at 266.5 mio MT in around 2023 at a market price of 4.5–8.1 USD/kg (Hernández-Pérez et al., 2019; Delgado Arcaño et al., 2020). Main producers for the global market are Danisco DuPont (DK, FI), Futaste (CN), Cargill (US) and Mitsubishi Corporation (JP) amongst others (Hernández-Pérez et al., 2019).
In the chemical production, as mentioned in section 1, acid hydrolysis is performed for the biomass pretreatment, which mainly fractionates and depolymerizes the hemicellulosic fraction of the lignocellulosic biomass. The obtained hydrolysate consists primarily of xylose monomers, as they constitute the major part of the hemicellulose. The following xylose purification step is necessary due to the formation of various inhibitory substances, e.g., 5-hydroxymethylfurfural and furfural, which otherwise would lead to rapid deactivation of the catalyst in the conversion process. Subsequently, the xylose is converted to xylitol under the presence of hydrogen on a metallic catalyst, classically Raney nickel, with high yields and conversion rates. Lastly, the produced xylitol is purified in several steps (Delgado Arcaño et al., 2020).
In the biotechnological production, for the employed cell factories in the fermentation, a plethora of different microorganisms has been studied regarding their potential of producing xylitol at a favorable yield, productivity, and titer. Yeast strains are primarily in focus due to their natural ability to assimilate xylose and convert it into xylitol. In particular, Candida species, Debaromyces hansenii, and Kluyveromyces marxianus are suitable cell factories with yields of xylitol from xylose around, volumetric productivities of
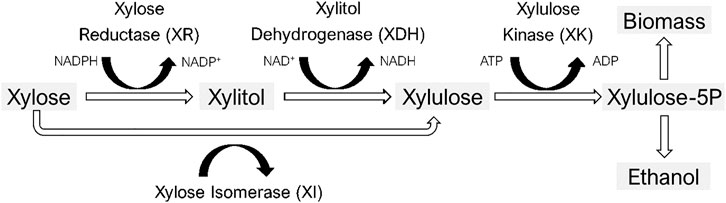
FIGURE 1. Xylose assimilation pathway in yeasts (Albuquerque et al., 2014).
As illustrated, xylose is assimilated through the cell wall and converted in the first step to xylitol. Xylitol is then further converted and ultimately ends up in the anabolism to form biomass or converted via the pentose phosphate pathway into metabolites participating in the glycolysis and ending up as pyruvate (Albuquerque et al., 2014). In order to maximize the operational yield for xylitol in a fermentation process, the conditions of the process have to be adjusted accordingly to prevent the consumption of xylose for further process steps. A key parameter here is the aeration of the process: several studies have shown that aerobic conditions with a low oxygen availability are advantageous with regards to the availability of co-factors, as shown in Figure 1 (Albuquerque et al., 2014; Rao et al., 2016). Furthermore, the availability of co-substrate as, e.g., glucose at lower concentrations is beneficial for the productivity of the cell factory (Rao et al., 2016; Hernández-Pérez et al., 2019).
Those and other prominent candidates as Saccharomyces cerevisiae have been subjected to metabolic and genetic engineering strategies to increase productivity and titer for xylitol production (Rao et al., 2016; Dasgupta et al., 2017; Hernández-Pérez et al., 2019). The main targets are the overexpression of xylose reductase, the suppression of xylitol dehydrogenase, the knockout of the genes for xylose isomerase and xylitol dehydrogenase, as well as more advanced engineering strategies to up- and downregulate the availability or completely shifting co-factors (Rao et al., 2016; Dasgupta et al., 2017; Hernández-Pérez et al., 2019). Furthermore, evolution techniques have been successfully applied to increase the tolerance to inhibitory components (Hernández-Pérez et al., 2019). The performance metrics of these engineered cell factories are yields of xylitol from xylose around
Value-Added Co-Products
Succinic Acid
Succinic acid, also identified as one of the top 12 biobased chemicals by the US Department of Energy, has potential use as a platform chemical (Werpy and Petersen, 2004). What makes the biotechnological production particularly attractive from a sustainability point of view is the possibility of CO2 capture in the process, as the process stoichiometry requires a net CO2 uptake (Mancini et al., 2020). Succinate is a metabolite in the citric acid cycle and naturally occurs in most microorganisms. The production of succinic acid with cell factories has been investigated thoroughly: the most promising cell factories for production are, amongst others, Actinobacillus succinogenes and Mannheimia succiniciproducens (Mancini et al., 2020). Also, engineered cell factories like Escherichia coli show favorable yield, productivity, and titer (Mancini et al., 2020). A problem with the biotechnological production is the formation of other organic acids and the induced product inhibition, which can impair the performance of a fermenter and a complex downstream process (Mancini et al., 2020).
Biokerosene
As lignin consists of a vast mixture of differently polymerized aromatic compounds, several products can be obtained from it, e.g., carbon fibers, thermoplastic and elastomeric polymers, and other fuels and chemicals (Ragauskas et al., 2014). Out of this product palette, the research on the production of fuels and chemicals has yielded several potential production strategies with an acceptable technology readiness level that allows for a potential commercial production (Ragauskas et al., 2014). The aviation industry currently focuses heavily on improving the sustainability in commercial aviation, of which one approach is the substitution of commercial kerosene with biokerosene from renewable resources (Chiaramonti et al., 2014). Fuels produced from lignin or lignocellulosic biomass in general naturally contain a high amount of aromatic components, which is particularly important for the properties of aviation fuel (Chiaramonti et al., 2014). Hence the production of biokerosene from lignin is a potentially suitable valorization strategy (Chiaramonti et al., 2014).
Heat
Alternatively, the lignin fraction can also be valorized by targeting its high energy content. By combusting the lignin directly, heat can be created, which can be converted into other forms of energy, e.g., steam or electricity (Ragauskas et al., 2014).
Unit Operations
The following section describes each unit operation that is employed in a possible xylitol biorefinery setup for the production of xylitol and value-added co-products. Furthermore, the respectively developed mechanistic model describing the underlying physical, chemical, and biological phenomena is elucidated for each unit operation. For all model files, the reader is referred to the GitHub repository of this manuscript, where all model and simulation files are provided (Vollmer, 2021).
All models described in this section are implemented in MATLAB. For the evaporation, the models implemented in ASPEN Plus are used and interfaced with MATLAB through a COM interface. The S3O framework is also implemented in MATLAB. The mechanistic models are all fitted to either proprietary experimental data or data retrieved from literature. The models are all validated within their boundaries for their mass and energy balances, kinetics, and thermodynamic assumptions. They are implemented in the S3O framework and assessed regarding their prediction robustness through an uncertainty and sensitivity analysis. Further information regarding the models and their implementation can be found in the original manuscript describing the S3O framework (Vollmer et al., 2021a).
Pretreatment
For both the chemical and the biotechnological production process of xylitol, the biomass pretreatment as the first unit operation has vital importance for the whole process. With xylitol as the main product, the priority for the pretreatment lies in the fractionation and depolymerization of the hemicellulosic fraction. The most prominent pretreatment technology for this task are dilute acid, autothermal hydrolysis, and steam explosion pretreatment (Mussatto and Dragone, 2016). Previous studies have shown the excellent metrics of dilute acid pretreatment on wheat straw in terms of high monomer yield, good fractionation, and acceptably low inhibitor formation (Vollmer et al., 2021c).
Vollmer et al. (2021b) developed a mechanistic model describing the pretreatment with mass and energy balances for all major occurring components. The kinetics of the pretreatment are described with first-order reaction equations. For a detailed overview of the work and the specific model equations, the reader is referred to the manuscript and the supplementary material for the model equations and the parameter values (Vollmer et al., 2021c). In this manuscript, the pretreatment unit is abbreviated as PT.
Enzymatic Hydrolysis
The residue from the pretreatment containing the cellulosic and lignin fraction needs to be processed further to fractionate and depolymerize the cellulosic fraction into glucose monomers. Amongst possibly employable technologies, most biorefinery concepts rely on an enzymatic hydrolysis process for this task (Kadam et al., 2004). The utilized enzymes are a cocktail of different glucanases, glucosidases, and hydrolases, which efficiently break down the cellulose into cellobiose and finally into glucose but can be inhibited by present sugars like glucose and xylose (Prunescu and Sin, 2013; Novozymes, 2017).
Kadam et al. (2004) proposed a mechanistic model describing the enzymatic hydrolysis with mass and energy balances for cellulose, cellobiose, glucose, and xylose; the kinetics of the enzymatic hydrolysis are described with Michaelis-Menten kinetics (Kadam et al., 2004). Prunescu and Sin (2013) reviewed the model parameters and updated them accordingly to new commercially available enzymes (Prunescu and Sin, 2013). For a detailed overview of the work and the specific model equations, the reader is referred to both manuscripts and the supplementary material for the model equations and the parameter values. In this manuscript, the enzymatic hydrolysis unit is abbreviated as EH.
Fermentation
Both the xylose-rich stream of the pretreatment and the glucose-rich stream of the enzymatic hydrolysis serve as the substrate for both fermentation steps. Given the elaborations in Sections 2.1.2 and 2.1.3, the employed cell factory for the fermentation of xylitol is a Candida mogii strain, and the employed cell factory for the fermentation of succinic acid is a Mannheimia succiniciproducens strain.
The developed mechanistic models are both black-box models with mass and energy balances for all primary components, and the kinetics are set up as described by Heijnen and van Gulik (2009) with substrate uptake rates, Herbert-Pirt distribution relations, and product formation rates (Heijnen and van Gulik, 2009). The used data for the parameter estimation of the xylitol fermentation model derives from a paper published by Tochampa et al. (2005). The used data for the parameter estimation of the succinic acid fermentation model derives from a paper published by Song et al. (2008). Both models allow for the simulation of batch and fed-batch processes. In this manuscript, the fermentation unit for the production of xylitol is abbreviated as FX, the unit for the production of succinic acid is abbreviated as FS.
Evaporation
After the pretreatment, after the enzymatic hydrolysis, and after the fermentations, the process streams contain a certain concentration of the product of interest. For all cases, it can be of interest to increase the concentrations of the stream to achieve higher titers in the fermentation and to remove certain inhibitory compounds. Potential technologies for this unit operation are either evaporation units in various forms or membrane units, with the former ones being widely commercially available and thus easier to implement but having very high energy demands (Kiss et al., 2016). Mechanistic models for simulating evaporation processes are readily available in different commercial process simulators, e.g., ASPEN Plus. In this manuscript, the upconcentration unit for the hemicellulose hydrolyzate is abbreviated as UH. The unit for the cellulose hydrolyzate is abbreviated as UC, the evaporation unit for the downstream processing of xylitol is abbreviated as EX, the unit for the downstream processing of succinic acid is abbreviated as ES.
Crystallization
Xylitol and succinic acid are soluble in water and solid at room temperature. Hence, crystallization is a suitable unit operation to separate and purify those two substances from aqueous streams. Crystallization is performed as either heating, cooling, pH, or antisolvent crystallization (Kirwan and Orella, 2002). For the xylitol, both a cooling crystallization and an antisolvent crystallization with ethanol are considered, as the high xylitol solubility in water decreases in the presence of ethanol (Martínez et al., 2008). For succinic acid, a cooling crystallization is considered.
The developed mechanistic model is based on the work of (Giulietti et al., 2001; Öner et al., 2018). The crystallization is described with mass and energy balances. The kinetics are described with a population balance, describing the nucleation and growth of crystals. The population balance is solved with the method of moments. The solubility and kinetic data for the xylitol crystallization is obtained from experiments, the kinetic data for the succinic acid crystallization is obtained from the literature (Mullin and Whiting, 1980; Qiu and Rasmuson, 1990; Qiu and Rasmuson, 1991; Qiu and Rasmuson, 1994). For a detailed overview of the work and the specific model equations, the reader is referred to both manuscripts and the supplementary material for the model equations and the parameter values. In this manuscript, the crystallization units for the downstream processing of xylitol are abbreviated as CXi, the units for the downstream processing of succinic acid are abbreviated as CSi.
Lignin Pyrolysis
After the fractionation and depolymerization of the cellulosic fraction in the enzymatic hydrolysis, the remaining solid fraction consists primarily of lignin. As described in section 2.1.3, the lignin fraction also consists of monomers, which are supposed to be processed furtherly to sustainable aviation fuel. The first unit operation for this process is a pyrolysis step to break down the lignin’s macromolecular structure and create bio-oil as a precursor for the fuel (Wang and Tao, 2016). Fast pyrolysis with time ranges between
The used mechanistic model is based on the work of (Debiagi et al., 2018). The mechanistic model is based on mass and energy balances. First-order reaction equations describe the kinetic behavior. The model describes the degradation of three lignin monomer structures commonly present in different fractions in the biomass into over 50 different products. All parameters for the model are provided in the original publication (Debiagi et al., 2018). For a detailed overview of the work and the specific model equations, the reader is referred to the original manuscript and the supplementary material for the model equations and the parameter values. In this manuscript, the lignin pyrolysis unit is abbreviated as LP.
Lignin Hydrotreatment
The produced bio-oil in the lignin pyrolysis classically contains a comparatively high amount of oxygen and is thus not directly usable as aviation fuel (Wang and Tao, 2016). In order to reduce the oxygen amount, several options have been investigated in research, of which catalytic hydrotreatment is one of the most promising ones (Zacher et al., 2014). The occurring reactions are quite complex, which is why a lumped kinetic network is introduced that involves eight components (Cordero-Lanzac et al., 2020). All the components which are formed during the pyrolysis are assigned to one of the lumped components of the hydrotreatment. The reaction itself occurs over a zeolite catalyst and the addition of hydrogen.
The employed and adapted mechanistic model is based on (Cordero-Lanzac et al., 2020), involving mass and energy balances. Reaction-convection-diffusion equations describe the kinetic behavior. All parameters for the model were reestimated based on the kinetic data. For the parameters and a detailed model description, the reader is referred to the original manuscript and the supplementary material. In this manuscript, the lignin hydrotreatment unit is abbreviated as LH.
Lignin Fractionation
Lastly, after the hydrotreatment, the present fractions in the stream must be separated. Besides the two products, these are the fraction with gaseous components, phenolics, aromatics, and water. In a two-step separation with two flash drums, first, the gaseous components are removed, and subsequently, the phenolics and aromatics are separated from the water. Depending on the created fractions, a fractionated distillation can also be used as a unit operation. Mechanistic models for simulating fractionation processes are readily available in different commercial process simulators, e.g., ASPEN Plus. In this manuscript, the lignin fractionation unit is abbreviated as LF.
Auxiliary Unit Operations
Besides all the listed primary unit operations, the biorefinery setup requires several auxiliary unit operations for full functionality. These are listed shortly in the following section:
• Feedstock Processing: To process the lignocellulosic biomass, it has to be transported into the process through conveyor belts, milled, and stored before actually entering the pretreatment unit operation. In this manuscript, the feedstock processing unit is abbreviated as Feed.
• Product Storage: In order to process the produced xylitol, the value-added co-products, and other intermediates, storage capacity is installed to buffer 7 days of production, involving solid storages for the xylitol and succinic acid, and liquid storage for the kerosene and storage for all other chemicals and intermediate steps involved in the process. In this manuscript, the storage units are abbreviated as Store.
• Wastewater treatment: In order to treat all aqueous effluents from the process, a wastewater treatment plant is considered to be installed to comply with legislative constraints and also to regenerate freshwater for the process in the form of material flows for unit operations or in the form of material flows as cooling water. In this manuscript, the wastewater processing unit is abbreviated as WWT.
• Combustion, power, and steam generation: Lastly, to recover the organic residues of the process, these gaseous and solid streams are combusted to generate steam and power in a turbine. This process has a significant impact on the overall economics by increasing the biorefinery integration and lowering operational expenditures at the cost of capital investment. In this manuscript, the steam generation unit is abbreviated as Steam.
All the listed auxiliary unit operations are not mechanistically modeled. Instead, based on a report of the National Renewable Energy Laboratory (NREL) on the process design of an ethanol biorefinery, all the ingoing mass and energy streams in the xylitol biorefinery are scaled to the ethanol biorefinery in the report to estimate the outgoing mass and energy streams and the costs (Humbird et al., 2011). This induces uncertainty in the following analyses, which is why these decisions will be evaluated later on through uncertainty and sensitivity analysis in the techno-economic analysis.
Furthermore, from a practical point of view, additional unit operations in the process can be required. This is related to the feedstock and the production of inhibitory compounds in the pretreatment. Besides the considered major inhibitory compounds, further components are formed in minor amounts that can require, e.g., an adsorption process for their removal (Bhatia et al., 2020). Also, the lignin itself imposes challenges to the process due to the potential of clogging of equipment (Pienihäkkinen et al., 2021). This possible requirement of additional unit operation imposes another source of uncertainty, which will be equally addressed in the techno-economic analysis. A detailed description of the calculations can be found in the Supplementary Material.
Process Design
Process Synthesis and Design Framework
As described by Vollmer et al. (2021a), the framework consists of three sequential steps: 1) the selection of products, feedstock, and processes, 2) superstructure optimization, and 3) simulation-based optimization. The overall procedure is established with the idea of “having the end in mind.” Hence, in the first step, based on expert knowledge, a meaningful selection of products is made and a feedstock from which these products are supposed to be produced. Subsequently, potential process steps are evaluated and composed to a process superstructure in a bottom-up fashion. This aims at keeping the potential number of process realizations low. In the second step, this superstructure is optimized through mathematical optimization to obtain several candidate process topologies. In order to include the different mechanistic models in the superstructure, surrogate models are used based on different machine learning technologies. For a successful application of these surrogate models, a proper benchmark of different alternatives is vital (Vollmer et al., 2021b). After determining the reduced set of candidate process topologies, in the third step, all of these are subjected to simulation-based optimization to consolidate the process design and find a truly optimal solution (Vollmer et al., 2021a).
Sizing of Equipment
For using the mechanistic models for process design, the overall plant capacity used for the design calculation and the corresponding mass and energy in- and outflows entail a specific capacity for each unit operation. In general, the volumetric capacity of a unit operation
with the hourly capacity
Capital Expenditures and Operational Expenses
Based on each unit operation’s volumetric or mass-based capacity, the fixed capital investment for all unit operations in the plant can be calculated. Whenever possible, costing is based on the NREL Report on “Process design and economics for conversion of lignocellulosic biomass to ethanol,” as the report is based on actual quotations from different equipment manufacturers with a high level of accuracy and detailedness (Humbird et al., 2011). As both biorefinery setups differ to a certain extent, all fixed capital investment, which is not available in the NREL report, is estimated through a cost estimation tool (Peters et al., 2002). All costs retrieved are extrapolated to the capacity of the planned biorefinery by the plant capacity ratio method as follows:
With
With
Being 7.88% higher as the sum of capital costs for all unit operations. The total indirect costs are determined as follows:
Amounting to 60% of the total direct costs. The fixed capital investment is defined as the sum of total direct and indirect costs:
And lastly, the total capital investment corresponds to:
Being 5.47% higher than the fixed capital investment and defining the capital expenditures of the biorefinery. The operational expenses of the biorefinery are defined by the total production costs
Being the sum of both operational costs. Lastly, the sales of the products are determined by summing up all individual sales of each product:
With
Techno-Economic Analysis
Key Performance Indicators
Ultimately, the economic feasibility of a biorefinery can be evaluated based on capital expenditure and operational expenses by calculating different key performance indicators (KPI). According to Peters et al. (2002), they are calculated as follows: The first KPI considered in this study is the return on investment
The
With
With
Lastly, the calculation of the
On a side note, only one variable can be left free to be optimized to keep this equation solvable. In this case, for xylitol as the primary product, the product price of xylitol is chosen instead of, e.g., a multiplier for all product prices. (Peters et al., 2002).
Economic Risk Analysis
As mentioned earlier, the design of any biotechnological process inherently incorporates uncertainty of various sources. These potentially can be assumptions about yields and productivities, estimates about capacity and capital investment, factors that influence the scale-up of a process, or external factors like price fluctuations. To assess the impact of uncertainties on the economic feasibility, Monte Carlo-Based Methods are a suitable option. In this case, assessing the effects of the uncertainties on the predicted key performance indicators, an uncertainty analysis is performed as described in (Sin et al., 2009).
Results
Selection of Products, Feedstock, and Process Unit Operations
The main product for the biorefinery concept in this study is xylitol. In light of the explanations in Introduction and Feedstock and Products, an integrated xylitol biorefinery with lignocellulosic biomass as feedstock will utilize the hemicellulosic fraction of the lignocellulosic biomass for the production of xylitol. This leaves the cellulosic and the lignin fraction as possible substrates for value-added co-products in a multi-product biorefinery. The cellulosic fraction consists mainly of glucose monomers, whereas the lignin fraction is an amorphous macromolecule with different aromatic monomers. As discussed in section 2.1.3, potential value-added co-products, in this case, can be succinic acid, biokerosene, or heat, with the prior one being a product for the cellulosic fraction and the latter two a product for the lignin fraction.
Regarding a potential feedstock, all three fractions should be represented to a sufficient amount to produce all four products possibly. With xylitol being the product with the highest product price, the potential feedstock favorably has a high hemicellulosic fraction. This is the case for most agricultural residues, e.g., wheat straw, which is hence selected for this case study. As plant capacity, an amount of
Regarding the processing units, all relevant unit operations are described in Unit Operations, considering only possibilities with a high technology readiness level to retrieve a realistic process design for the xylitol biorefinery. All possible process routes, which form the superstructure for step 2 of the framework, are displayed in Figure 2.
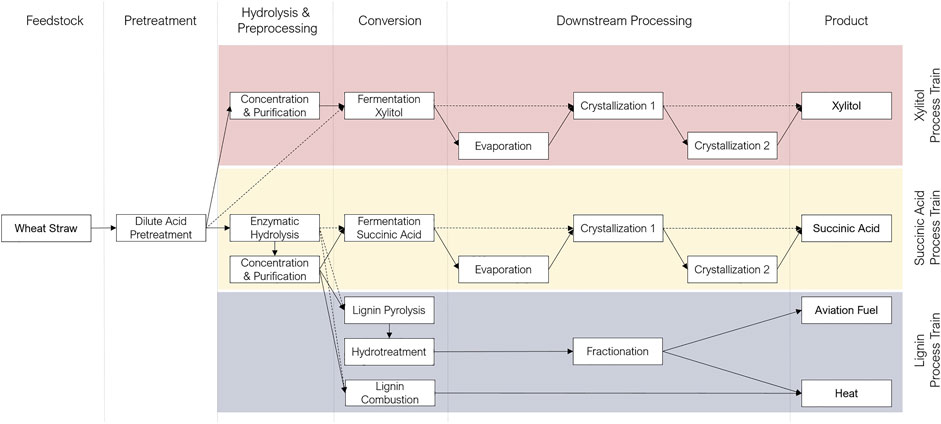
FIGURE 2. Overview over the postulated superstructure for the xylitol biorefinery in the S3O framework.
As can be seen, the potential options in the superstructure involve the inclusion or exclusion of an upconcentration unit, an evaporation unit, and a second crystallization unit for the xylitol process train, based on previously obtained results (Vollmer et al., 2020). The same superstructure is assumed for the succinic acid process train, also agreeing with other proposed process designs (Jansen and van Gulik, 2014). In the lignin process train, the two possible options are to convert the lignin to biofuel and use the residues of this and other process streams for combustion or to use the lignin entirely for combustion to increase the potential for heat integration in the process.
Design Space Exploration
The outcome of the design space exploration in the scope of this case study is twofold. Firstly, it is used to reduce the size of the superstructure and exclude options that a priori are infeasible due to nontrivial design constraints. It serves to determine the sensitivity of the operational variables to prioritize them for the SSO.
The design space exploration itself is performed in two stages. In the first stage, coarse sampling with
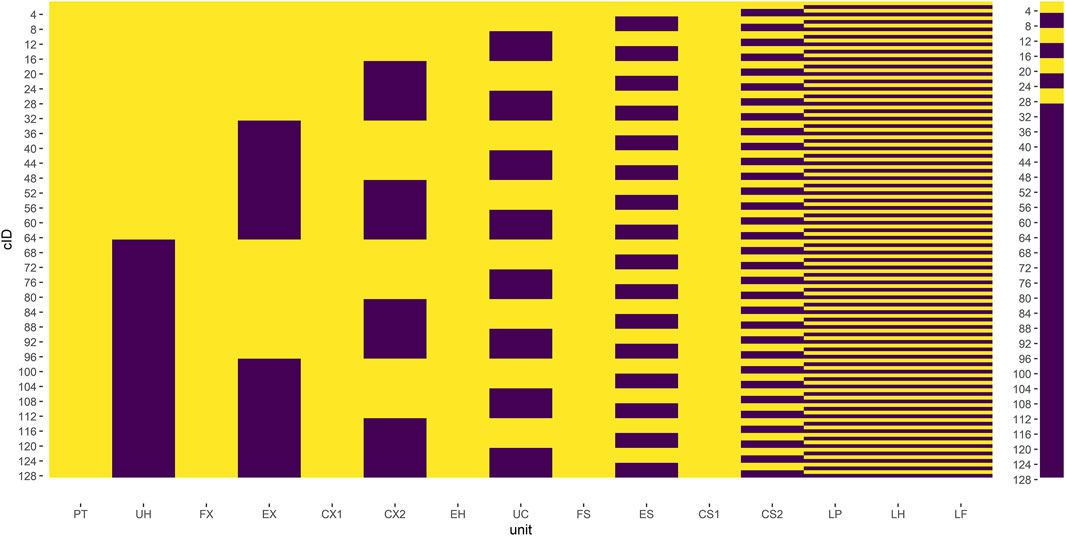
FIGURE 3. Feasibility matrix of the coarse sampling, displaying the existence (yellow) or absence (blue) of each unit operation in each configuration ID, with the last column indicating the feasibility (yellow) or infeasibility (blue) of the flowsheet.
As illustrated, most flowsheet options actually do not allow for the production of xylitol and succinic acid as all sample points yield infeasible solutions. More precisely, all flowsheet options that do not include either the upconcentration step for the hemicellulose process train (UH) or the evaporation step of the hemicellulose train (EX), or the evaporation step of the cellulose train (ES). In reverse, this indicates that these unit operations are seemingly compulsory for any xylitol biorefinery setup. The cause behind this is the necessary substrate concentration for the xylitol fermentation and the high dilution of the fermentation broth, paired with the high solubility of xylitol. Consequently, this already indicates a high necessary amount of heat in the downstream process, which will impact the operational expenses of the process.
In the second stage of the design space exploration, fine sampling with
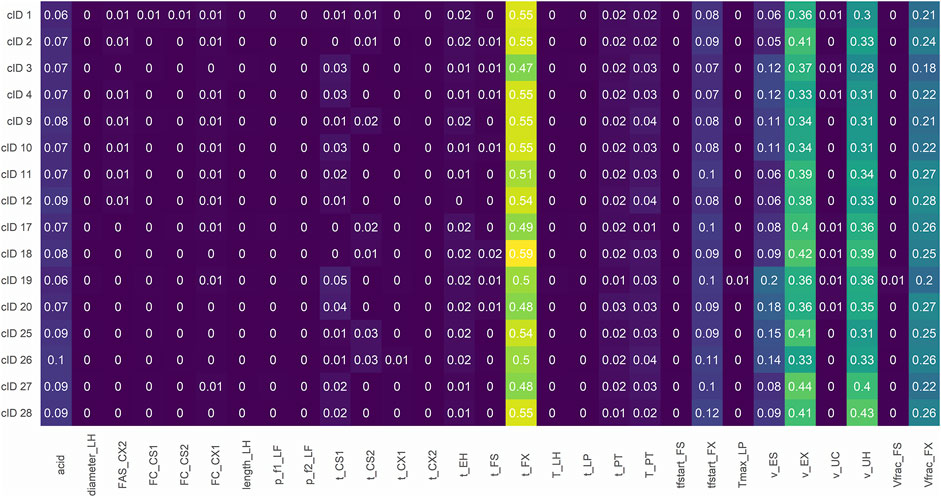
FIGURE 4. Heatmap of the total sensitivity indices for each flowsheet option and each operational variable (nonexistent variables are indicated with 0).
It is clearly visible that for most flowsheets, particularly variables that influence the xylitol fermentation step show the highest sensitivities. This is explainable since the microorganism can also consume xylitol as a metabolite in the fermentation, indicating an optimum for the xylitol production, which must be met. By meeting this optimum and maximizing the production of xylitol, key performances as the NPV are potentially improved due to the high selling price of the xylitol. In conclusion, the five most sensitive variables for each flowsheet option, as indicated in Figure 4, are used as input variables in the superstructure optimization problem. All other variables are fixed to their set point.
Superstructure Optimization
As the last operation in step 2) of the S3O framework, SSO is performed to determine candidate process topologies consolidated in step 3) of the framework. For this, flowsheet simulations with a reduced input space are performed for all feasible configuration IDs. The reduced input space consists of the five most sensitive input variables concerning the NPV, as presented in Figure 4. For each configuration ID,
Similar to the results of (Vollmer et al., 2021a; Vollmer et al., 2021b), the DTR surrogate shows the weakest validation metrics for the test dataset, whereas the GPR surrogate overall excels with respect to the resulting metrics. The ANN surrogate equally performs overall well with slightly weaker metrics than the GPR surrogate. All three models are used in the following SSO. All metrics are also listed in the Supplementary Material.
For the SSO, the optimization problem is formulated as follows:
In the optimization problem, the objective function is the NPV, which is supposed to be maximized. Constraints are imposed on the mass of yearly produced xylitol, which has to be higher than 5% of the global annual production capacity, and on the mass of yearly produced succinic acid, which has to be higher than 20% of the global annual production capacity. Lastly, the required electrical power for the operation of the plant cannot be higher as 0.05% of the electrical power produced through wind energy in Denmark. With the DTR surrogates, the given optimization problem turns into a mixed-integer linear program, which is solved with the GUROBI solver. With the GPR and the ANN surrogate, the given optimization problem turns into a series of nonlinear programs, which are solved with the fmincon solver and a multi-start setup. The results of all optimization runs are presented in the Supplementary Material.
The first result displayed in Figure 6 is that all configuration IDs (1, 3, 9, 11, 17, 19, 25, 27) that involve lignin production show no significantly higher NPV than their respective counterparts without lignin production. Furthermore, certain missing unit operations, e.g., the upconcentration unit for the cellulose stream, decrease the amounts of xylitol and succinic acid drastically, leading to an infringement of the applied boundary conditions. With respect to the prediction quality of the surrogate models, it is to point out that the DTR surrogate predicted all feasible solutions correctly. However, configuration IDs 3, 4, 19, 20, and 25 were predicted infeasible despite the other surrogates correctly predicting their feasibility. On the other hand, both the GPR and the ANN surrogate overpredicted the amount of produced xylitol for several flowsheets, yielding infeasible solutions. The constraint that most GPR and ANN solutions infringe is the constraint on the minimum xylitol production. For generally infeasible solutions, the constraint on the minimum xylitol production and the maximum electricity consumption are critical. The minimum succinic acid production constraint is surpassed in all found solutions. In conclusion, the same behavior for the surrogate models as discussed in Vollmer et al. (2021a) is visible in this case, namely the missing extrapolation ability of the DTR surrogate model and the weak prediction abilities of the GPR and the ANN surrogate. Therefore, selecting an appropriate surrogate model is highly context-specific and should be based on a benchmark after performing the optimization problem instead of relying on the results from the cross-validation of the models before the optimization (Vollmer et al., 2021a).
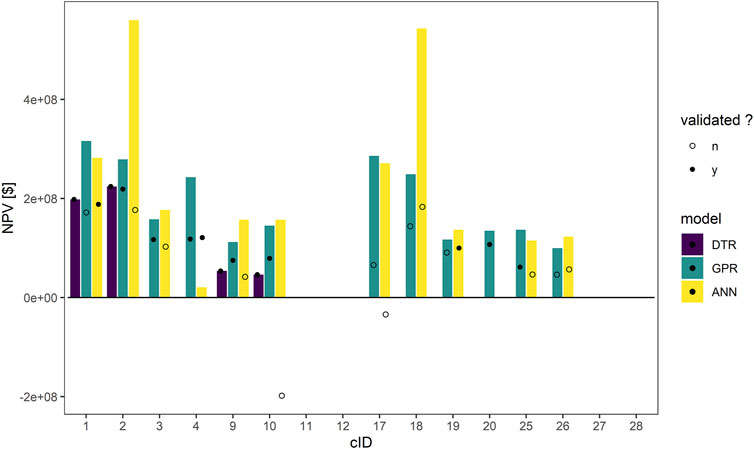
FIGURE 6. Results of the SSO for all three surrogate model types and the corresponding validation simulation.
In conclusion, configuration ID 2 predicted the highest NPV. Hence it is considered a candidate for step 3) of the framework. For comparative reasons, configuration ID 2 and configuration IDs 4 and 10 are selected for the risk assessment in Techno-Economic Analysis. Despite the infeasibility of configuration ID 18, it is selected as the fourth candidate, as the NPV is also comparatively high and the infringement of the boundary constraint is minimal. The operational variables are optimized again by subjecting it to step 3) of the framework, potentially leading to improved production, as shown in Vollmer et al. (2021a), which renders this configuration ID also feasible. The detailed formulation of the SSO and all results from the SSO are also listed in the Supplementary Material.
Simulation-Based Optimization
For the third step of the framework, the set of candidate process topologies as found in Superstructure Optimization is now subjected to simulation-based optimization under uncertainty. The objective for this optimization setup remains to be the NPV of the plant, and the optimizable variables remain the five most sensitive operational variables as found in Design Space Exploration. Regarding the uncertainties, a variation in the CAPEX, represented by the FCI, and the OPEX, represented by the TPC, is considered. The FCI is assumed to be up to 50% lower or 100% higher than its originally calculated value, following a triangular distribution. Equally, the TPC is assumed to be up to 20% lower or 50% higher than its original value, following a triangular distribution. Furthermore, the product prices for xylitol and succinic acid are considered within a specific range, based on historical price data between 2016 and 2021. The range for xylitol is assumed to be within 4.29 $/kg and 4.81$/kg, with a mean value of 4.57 $/kg, and the succinic acid between 3.18 $/kg and 3.20 $/kg, with a mean value of 3.19 $/kg, both assumed to follow a uniform distribution (Orion Market Research, 2020; IMARC, 2021). The MOSKopt solver is set up to run with
The MOSKopt solver hedges against the constraints by the mean value of the realizations of the uncertainty. The chosen infill criterion is the mcFEI criterion, using a particle swarm optimizer as infill solver.
The results from k = 100 iterations are shown in Figure 7. Firstly, it is visible that the predicted NPV under uncertainty is significantly lower than predicted in the SSO, and the mean value lies below the break-even point. Despite the optimizer being able to optimize the operational conditions, the uncertainties affect the objective to such an extent that the optimization to values above the break-even point is not feasible.
Techno-Economic Analysis
Analysis of Capital Expenditure and Operational Expenses
For a detailed insight into the techno-economic analysis results, Figure 8 shows the detailed composition of both the FCI and the TPC.
It is prominently visible that the FCI is dominated by around 50% through the investment in the wastewater treatment facilities, and the TPC is dominated mainly by the required steam and naturally also through the feedstock. These results again emphasize two prominent issues with (second-generation) biorefineries in particular. As mentioned in Introduction, a big issue for these processes is the costs in the downstream processing. By choosing evaporation units in the downstream processing, the requirement for steam is naturally high. This is intertwined with the results for the FCI, as the process runs on an aqueous basis and low concentrations induce both high costs for the downstream processing to remove the liquid but also high costs for the wastewater treatment capacity, as the aqueous streams, being very high, need to be treated before being recycled or released to the environment.
Capacity, Yield, and Size Analysis of the Plant
For the process design with cID 2 and a given annual feedstock capacity of
Risk-Based Economic Evaluation
For the risk analysis, the consolidated process design of configuration IDs 2,4, 10, and 18 are chosen as a basis with the optimized operational conditions as determined in Simulation-Based Optimization. The considered uncertainties are the same as for the simulation-based optimization. To quantify the uncertainty in the market data of both products, different distributions are analyzed concerning their quality of fit to historical price data for xylitol and succinic acid. The investigated distributions are a normal distribution, a lognormal distribution, a nonparametric distribution, an extreme-value distribution, and a uniform distribution. The results are summarized in Figure 9. On the x-axis, the price
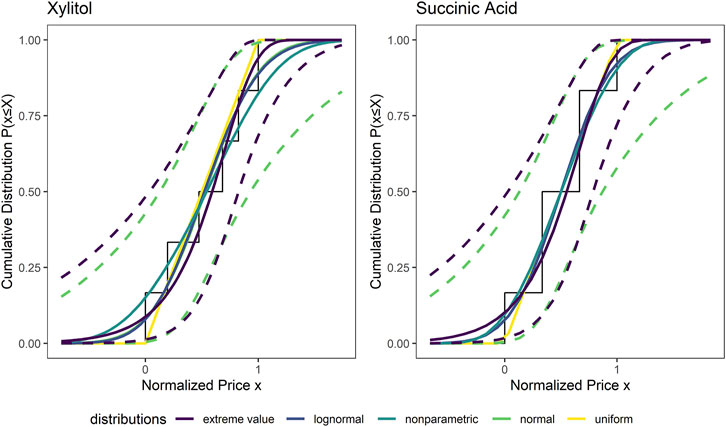
FIGURE 9. Cumulative density functions of all investigated distributions for the xylitol and the succinic acid price data.
It becomes apparent that all investigated distributions fit equally well, which is also confirmed by the coefficient of determination and the root mean squared error, both listed in the Supplementary Material. This implies that the historical data is not conclusive enough to identify the actual probability distribution function that describes the price uncertainties. Hence, a heuristic approach is employed by selecting a normal distribution and an extreme-value distribution as a thin-tailed and fat-tailed distribution. The parameters and metrics of all distributions are listed in the Supplementary Material. The Monte Carlo analysis is performed with
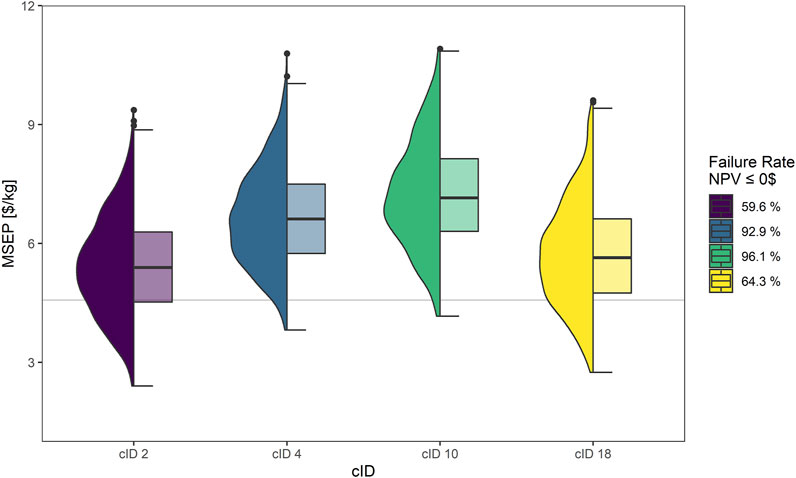
FIGURE 10. Violin plots of the MSEP of xylitol for N = 1,000 Monte Carlo samples for the risk analysis of the consolidated process design.
For all four evaluated configuration IDs, it becomes apparent that their economic feasibility is highly uncertain. The average selling price for xylitol of 2021, indicated at
Given this picture, a profitable construction and operation of a xylitol biorefinery is a high-risk venture. As the uncertainties in the product prices are determined through the development of their respective markets, which do not show significant volatilities over the past 5 years, a profitable operation seems highly unlikely. Furthermore, due to the low volatility in prices, the large overall uncertainty bounds are highly attributable to the uncertainties in the CAPEX and OPEX of the plant. Further investigation and a detailed design are crucial to minimize these and perform the risk analysis with updated costs to provide a confident conclusion on the economic viability.
Conclusion and Future Research
As the overarching goal in this manuscript, the process design of a biorefinery with xylitol as the main product was investigated to find a biotechnological process alternative to the existing chemical production process for xylitol. As several questions arise, like the decision on potential value-added co-products and considerations regarding which feedstock to use and how to best integrate the process, a conceptual design approach was required. For this, the S3O framework from a previous publication of the authors was utilized. Compared to other studies, mechanistic models were used, and detailed data regarding the costing of the equipment and current market prices of the product.
In a three-stage approach, products, feedstock, and process units are selected, and mechanistic unit operation models are built. Subsequently, an initial superstructure is formulated, and its design space is evaluated by both a coarse and a global sensitivity analysis. The coarse sampling serves the investigation on the feasibility of the flowsheets. As the first result in this study, only 16 out of 128 initial process flowsheet options are considered feasible. The global sensitivity analysis was used to investigate which operational variables are the most sensitive ones regarding the net present value as a key performance indicator of the plant. For all feasible flowsheets, the most sensitive variables influence the operation unit of the xylitol fermentation. This is explained by the fact that xylitol can be metabolized by the cell factory again, indicating a defined global optimum for the production of xylitol regarding the operational conditions.
Flowsheet sampling with the five most sensitive operational variables is performed for all feasible flowsheets. Three different surrogate model types are fitted to it (DTR, GPR, ANN) and benchmarked against each other regarding their validation metrics (coefficient of determination, root mean squared error). The results agree with those found earlier in Vollmer et al. (2021a), showing that the DTR surrogates have worse performance metrics than the GPR and the ANN. However, utilizing them in the surrogate-assisted superstructure optimization, the DTR models perform equally well as the other two. The results indicate, also in agreement with (Vollmer et al., 2021a), that the DTR lacks the possibility of interpolating highly nonlinear functional relationships with an insufficient amount of data points, which leads to suboptimal solutions. In contrast, the GPR and the ANN surrogates tend to overpredict the objective function value and constraint function values, resulting in infeasible solutions. From a process point of view, the conclusion prevails that the utilization of lignin for the production of biokerosene does not involve any economic advantage over using it for combustion and the generation of steam and electricity. Furthermore, excluding downstream process operations like the additional crystallization units or the upconcentration units before the fermentation units reduces the CAPEX of the potential process design, but this does not result in an increased NPV as the amount of recovered product is reduced significantly.
Based on these conclusions, the process configuration that involves all unit operations and utilizes the lignin for combustion (cID 2) is selected for simulation-based optimization in the third step. The considered uncertainties are both in the CAPEX and OPEX, expressed by a variation in the FCI and the TPC according to the used class 5 estimates. In addition, the product prices are considered uncertain within a range according to their global market prices between 2016 and 2021. The results indicate that when hedging the uncertainties against the mean value of the predictions, the operational conditions are further optimized. However, the uncertainties impact the objective stronger than the improvement of the objective, which decreases the NPV even below the break-even point. An additional Monte Carlo analysis with 1,000 points based on the operational conditions found by the SSO and the SBO shows a failure rate of almost 50% for the configuration ID 2, and–in comparison–failure rates of 55–90% for other potential candidates from the SSO. Similar results are seen throughout the literature for other realized biorefinery setups:
Mountraki et al. (2017) obtain similar results, stating that the chemical production of xylitol is more profitable than their biorefinery setup (Mountraki et al., 2017). On the other hand, Franceschin et al. (2011) state that their xylitol biorefinery setup can be feasible, but only with estimated capital investment data and a significantly higher xylitol market price (Franceschin et al., 2011). Giuliano et al. (2018) do not conclude a clear answer regarding the profitability but rather indicate the potential MSEP of ethanol for an integrated production with xylitol in a biorefinery setup is lower than the production of ethanol alone (Giuliano et al., 2018). While numerous companies, e.g., Dupont, hold patents for the biotechnological production of xylitol, all these companies still rely on its chemical production of it (Ubando et al., 2020). Regarding biotechnological succinic acid production, earlier joint ventures of different companies failed in the past years. However, the market for biotechnologically produced succinic acid from sugar directly–and not from lignocellulosic biomass–is supposed to grow in the future (Jansen and van Gulik, 2014; Mancini et al., 2020; Orion Market Research, 2020).
Given these considerations, the economic feasibility is not overall given and highly depends on the uncertainties in CAPEX and OPEX. Only a detailed design with concrete values for CAPEX and OPEX would allow for a clear decision for or against an investment as the uncertainty in the decision decreases. This comprises both more detailed sizing and costing of the considered equipment and potential additional equipment regarding the inhibitory compounds and lignin, as mentioned in section 2.2.9. With those uncertainties reduced, a stable market situation, as a projection based on historical price data, can potentially lead to an economically feasible xylitol biorefinery. Ultimately, the global price for xylitol is dictated by global trends towards healthier nutrition or the use of bioplastics, so an increase in the price in the future could be realistic (IMARC, 2021). However, the price for succinic acid as a product that is already produced biotechnologically depends much more on the global price for fossil oil, which has–against expectations–not significantly increased over the past 10 years, which has been the primary reason for economic infeasibilities of other biorefinery projects in the past (Mancini et al., 2020; Orion Market Research, 2020; Ubando et al., 2020). A comprehensive analysis of these and further factors would go beyond the scope of this thesis, hence, but is concisely discussed in a report by, e.g., McKinsey about “the future of second-generation biomass” (Alfano et al., 2016).
Putting these findings in a future perspective, further research on several aspects could lead to improvements in the xylitol biorefinery process itself and, subsequently, on the economic feasibility. Firstly, the utilization of the economies of scale is a considerable possibility to improve the economic performance of the plant, as the CAPEX does not scale linearly with the plant size and hence can lead to higher KPIs for larger plant capacities (Vollmer et al., 2022a).
Secondly, both fermentation units currently utilize wild-type cell factories. As presented in sections 2.1.2 and 2.1.3.1, the average product yields for both products range between 50% and 60%. Using engineered or optimized cell factories can significantly increase this value, together with the achievable productivities and titers for the fermentation (Vollmer et al., 2022b). The optimization of this has an immediate effect on the KPIs of the plant. Further research needs to investigate the impacts of engineered cell factories on the downstream processing and other requirements. Potential modifications of the downstream process can either increase or decrease the CAPEX and OPEX for the biorefinery, which ultimately determines the full effect of engineered/optimized cell factories. Lastly, the aspect of sustainability has not been investigated in the scope of this work. However, the quantitative assessment of the sustainability impact of a new biorefinery, not only focusing on the reduced emissions of CO2 but also other environmental aspects, is necessary to fully assess the potential of these biorefineries and their contribution to more sustainable value chains and production patterns for the future.
Data Availability Statement
The original contributions presented in the study are included in the article/Supplementary Material, further inquiries can be directed to the corresponding author.
Author Contributions
NV: writing the manuscript, simulations, completing results; KG: general idea, analyzing results, internal review. GS: genreral idea, analyzing results, interal review, general guidance.
Funding
The research project is part of the Fermentation-Based Biomanufacturing Initiative funded by the Novo Nordisk Foundation (Grant no. NNF17SA0031362).
Conflict of Interest
The authors declare that the research was conducted in the absence of any commercial or financial relationships that could be construed as a potential conflict of interest.
Publisher’s Note
All claims expressed in this article are solely those of the authors and do not necessarily represent those of their affiliated organizations, or those of the publisher, the editors and the reviewers. Any product that may be evaluated in this article, or claim that may be made by its manufacturer, is not guaranteed or endorsed by the publisher.
Supplementary Material
The Supplementary Material for this article can be found online at: https://www.frontiersin.org/articles/10.3389/fceng.2022.838478/full#supplementary-material
References
Albuquerque, T. L. D., Da Silva, I. J., De MacEdo, G. R., and Rocha, M. V. P. (2014). Biotechnological Production of Xylitol from Lignocellulosic Wastes: A Review. Process Biochem. 49, 1779–1789. doi:10.1016/j.procbio.2014.07.010
Alfano, S., Berruti, F., Denis, N., and Santagostino, A. (2016). The Future of Second-Generation Biomass. Available at: https://www.mckinsey.com/business-functions/sustainability/our-insights/the-future-of-second-generation-biomass (Accessed Febrauary 2, 2022).
J.-R. Bastidas-Oyanedel, and J. E. Schmidt (Editors) (2019). Biorefinery: Integrated Sustainable Processes for Biomass Conversion to Biomaterials, Biofuels and Fertilizers (Cham: Springer). doi:10.1007/978-3-030-10961-5
Bhatia, S. K., Jagtap, S. S., Bedekar, A. A., Bhatia, R. K., Patel, A. K., Pant, D., et al. (2020). Recent Developments in Pretreatment Technologies on Lignocellulosic Biomass: Effect of Key Parameters, Technological Improvements, and Challenges. Bioresour. Technol. 300, 122724. doi:10.1016/J.BIORTECH.2019.122724
Cherubini, F. (2010). The Biorefinery Concept: Using Biomass Instead of Oil for Producing Energy and Chemicals. Energ. Convers. Manage. 51, 1412–1421. doi:10.1016/j.enconman.2010.01.015
Chiaramonti, D., Prussi, M., Buffi, M., and Tacconi, D. (2014). Sustainable Bio Kerosene: Process Routes and Industrial Demonstration Activities in Aviation Biofuels. Appl. Energ. 136, 767–774. doi:10.1016/j.apenergy.2014.08.065
Cordero-Lanzac, T., Hita, I., García-Mateos, F. J., Castaño, P., Rodríguez-Mirasol, J., Cordero, T., et al. (2020). Adaptable Kinetic Model for the Transient and Pseudo-steady States in the Hydrodeoxygenation of Raw Bio-Oil. Chem. Eng. J. 400, 124679. doi:10.1016/j.cej.2020.124679
Da Silva, S. S., and Chandel, A. K. (2012). D-Xylitol: Fermentative Production, Application and Commercialization. Berlin, Heidelberg: Springer-Verlag Berlin Heidelberg. doi:10.1007/978-3-642-31887-0
Dasgupta, D., Bandhu, S., Adhikari, D. K., and Ghosh, D. (2017). Challenges and Prospects of Xylitol Production with Whole Cell Bio-Catalysis: A Review. Microbiol. Res. 197, 9–21. doi:10.1016/j.micres.2016.12.012
Debiagi, P., Gentile, G., Cuoci, A., Frassoldati, A., Ranzi, E., and Faravelli, T. (2018). A Predictive Model of Biochar Formation and Characterization. J. Anal. Appl. Pyrolysis 134, 326–335. doi:10.1016/j.jaap.2018.06.022
Delgado Arcaño, Y., Valmaña García, O. D., Mandelli, D., Carvalho, W. A., and Magalhães Pontes, L. A. (2020). Xylitol: A Review on the Progress and Challenges of its Production by Chemical Route. Catal. Today 344, 2–14. doi:10.1016/j.cattod.2018.07.060
DuPont (2012). XIVIATM Xylitol White Paper. Available at: http://www.danisco.com/fileadmin/user_upload/danisco/documents/products/2e_XIVIA_White_Paper.pdf (Accessed Febrauary 2, 2022).
Franceschin, G., Sudiro, M., Ingram, T., Smirnova, I., Brunner, G., and Bertucco, A. (2011). Conversion of rye Straw into Fuel and Xylitol: A Technical and Economical Assessment Based on Experimental Data. Chem. Eng. Res. Des. 89, 631–640. doi:10.1016/j.cherd.2010.11.001
Giuliano, A., Barletta, D., De Bari, I., and Poletto, M. (2018). Techno-Economic Assessment of a Lignocellulosic Biorefinery Co-Producing Ethanol and Xylitol or Furfural. Comput. Aided Chem. Eng. 43, 585–590. doi:10.1016/B978-0-444-64235-6.50105-4
Giulietti, M., Seckler, M. M., Derenzo, S., Ré, M. I., and Cekinski, E. (2001). Industrial Crystallization and Precipitation from Solutions: State of the Technique. Braz. J. Chem. Eng. 18, 423–440. doi:10.1590/S0104-66322001000400007
Heijnen, J. J., and van Gulik, W. M. (2009). “Section II - Balances and Reaction Models,” in The Metabolic Pathway Engineering Handbook: Fundamentals. Editor C. D. Smolke (Boca Raton: CRC Press). II-1-11–20.
Hernández-Pérez, A. F., de Arruda, P. V., Sene, L., da Silva, S. S., Kumar Chandel, A., and de Almeida Felipedas, M. D. G. G. (2019). Xylitol Bioproduction: State-Of-The-Art, Industrial Paradigm Shift, and Opportunities for Integrated Biorefineries. Crit. Rev. Biotechnol. 39, 924–943. doi:10.1080/07388551.2019.1640658
Humbird, D., Davis, R., Tao, L., Kinchin, C., Hsu, D., Aden, A., et al. (2011). Process Design and Economics for Conversion of Lignocellulosic Biomass to Ethanol. Available at: http://www.nrel.gov/docs/fy11osti/51400.pdf%5Cnpapers2://publication/uuid/49A5007E-9A58-4E2B-AB4E-4A4428F6EA66 (Accessed Febrauary 2, 2022).
IMARC (2021). Xylitol Market: Global Industry Trends, Share, Size, Growth, Opportunity and Forecast 2021-2026. Noida, Uttar Pradesh: IMARCS Group.
Jansen, M. L., and van Gulik, W. M. (2014). Towards Large Scale Fermentative Production of Succinic Acid. Curr. Opin. Biotechnol. 30, 190–197. doi:10.1016/j.copbio.2014.07.003
Kadam, K. L., Rydholm, E. C., and McMillan, J. D. (2004). Development and Validation of a Kinetic Model for Enzymatic Saccharification of Lignocellulosic Biomass. Biotechnol. Prog. 20, 698–705. doi:10.1021/bp034316x
Kirwan, D. J., and Orella, C. J. (2002). “Crystallization in the Pharmaceutical and Bioprocessing Industries,” in Handbook of Industrial Crystallization (Oxford: Elsevier), 249–266. doi:10.1016/b978-075067012-8/50013-6
Kiss, A. A., Lange, J.-P., Schuur, B., Brilman, D. W. F., van der Ham, A. G. J., and Kersten, S. R. A. (2016). Separation Technology-Making a Difference in Biorefineries. Biomass and Bioenergy 95, 296–309. doi:10.1016/j.biombioe.2016.05.021
Mancini, E., Mansouri, S. S., Gernaey, K. V., Luo, J., and Pinelo, M. (2020). From Second Generation Feed-Stocks to Innovative Fermentation and Downstream Techniques for Succinic Acid Production. Crit. Rev. Environ. Sci. Technol. 50, 1829–1873. doi:10.1080/10643389.2019.1670530
Martínez, E. A., Giulietti, M., de Almeida e Silva, J. B., and Derenzo, S. (2008). Kinetics of the Xylitol Crystallization in Hydro-Alcoholic Solution. Chem. Eng. Process. Process Intensification 47, 2157–2162. doi:10.1016/j.cep.2007.11.004
Mountraki, A. D., Koutsospyros, K. R., Mlayah, B. B., and Kokossis, A. C. (2017). Selection of Biorefinery Routes: The Case of Xylitol and its Integration with an Organosolv Process. Waste Biomass Valor. 8, 2283–2300. doi:10.1007/s12649-016-9814-8
Mullin, J. W., and Whiting, M. J. L. (1980). Succinic Acid Crystal Growth Rates in Aqueous Solution. Ind. Eng. Chem. Fund. 19, 117–121. doi:10.1021/i160073a020
Mussatto, S. I., and Dragone, G. M. (2016). “Biomass Pretreatment, Biorefineries, and Potential Products for a Bioeconomy Development,” in Biomass Fractionation Technologies for a Lignocellulosic Feedstock Based Biorefinery (Amsterdam: Elsevier), 1–22. doi:10.1016/B978-0-12-802323-5.00001-3
Novozymes (2017). Novozymes Cellic® CTec3 HS Application Sheet - Secure Your Plant’s Lowest Cost. Bagsværd, Denmark: Novozymes A/S.
Öner, M., Bach, C., Tajsoleiman, T., Molla, G. S., Freitag, M. F., Stocks, S. M., et al. (2018). “Scale-up Modeling of a Pharmaceutical Crystallization Process via Compartmentalization Approach,” in Computer Aided Chemical Engineering, 181–186. doi:10.1016/B978-0-444-64241-7.50025-2
Orion Market Research (2020). Global Succinic Acid Market Forecast. Indore, Madhya Pradesh: Orion Market Research Pvt Ltd, 2016–2026.
Özdenkçi, K., De Blasio, C., Muddassar, H. R., Melin, K., Oinas, P., Koskinen, J., et al. (2017). A Novel Biorefinery Integration Concept for Lignocellulosic Biomass. Energ. Convers. Manage. 149, 974–987. doi:10.1016/J.ENCONMAN.2017.04.034
Peters, M., Timmerhaus, K., and Peters, M. (2002). Plant Design and Economics for Chemical Engineers. Max: 0639785503897: Amazon.com: Books. 5th ed. New York, NY: McGraw-Hill Education. Available at: https://www.amazon.com/Plant-Design-Economics-Chemical-Engineers/dp/0072392665 (Accessed November 11, 2021).
Pienihäkkinen, E., Lindfors, C., Ohra-Aho, T., Lehtonen, J., Granström, T., Yamamoto, M., et al. (2021). Fast Pyrolysis of Hydrolysis Lignin in Fluidized Bed Reactors. Energy Fuels 35, 14758–14769. doi:10.1021/ACS.ENERGYFUELS.1C01719
Prunescu, R. M., and Sin, G. (2013). Dynamic Modeling and Validation of a Lignocellulosic Enzymatic Hydrolysis Process - A Demonstration Scale Study. Bioresour. Technol. 150, 393–403. doi:10.1016/j.biortech.2013.10.029
Qiu, Y., and Rasmuson, Å. C. (1990). Growth and Dissolution of Succinic Acid Crystals in a Batch Stirred Crystallizer. Aiche J. 36, 665–676. doi:10.1002/aic.690360504
Qiu, Y., and Rasmuson, Å. C. (1991). Nucleation and Growth of Succinic Acid in a Batch Cooling Crystallizer. Aiche J. 37, 1293–1304. doi:10.1002/aic.690370903
Qiu, Y., and Rasmuson, Å. C. (1994). Estimation of Crystallization Kinetics from Batch Cooling Experiments. Aiche J. 40, 799–812. doi:10.1002/aic.690400507
Ragauskas, A. J., Beckham, G. T., Biddy, M. J., Chandra, R., Chen, F., Davis, M. F., et al. (2014). Lignin Valorization: Improving Lignin Processing in the Biorefinery. Science 344, 61851246843. doi:10.1126/science.1246843
Rao, L. V., Goli, J. K., Gentela, J., and Koti, S. (2016). Bioconversion of Lignocellulosic Biomass to Xylitol: An Overview. Bioresour. Technol. 213, 299–310. doi:10.1016/j.biortech.2016.04.092
Sin, G., Gernaey, K. V., and Lantz, A. E. (2009). Good Modeling Practice for PAT Applications: Propagation of Input Uncertainty and Sensitivity Analysis. Biotechnol. Prog. 25, 1043–1053. doi:10.1002/btpr.166
Song, H., Jang, S. H., Park, J. M., and Lee, S. Y. (2008). Modeling of Batch Fermentation Kinetics for Succinic Acid Production by Mannheimia Succiniciproducens. Biochem. Eng. J. 40, 107–115. doi:10.1016/j.bej.2007.11.021
Tochampa, W., Sirisansaneeyakul, S., Vanichsriratana, W., Srinophakun, P., Bakker, H. H. C., and Chisti, Y. (2005). A Model of Xylitol Production by the Yeast Candida Mogii. Bioproc. Biosyst. Eng. 28, 175–183. doi:10.1007/s00449-005-0025-0
Ubando, A. T., Felix, C. B., and Chen, W.-H. (2020). Biorefineries in Circular Bioeconomy: A Comprehensive Review. Bioresour. Technol. 299, 122585. doi:10.1016/j.biortech.2019.122585
United Nations (2015). Transforming Our World: The 2030 Agenda for Sustainable Development. Available at: https://sdgs.un.org/sites/default/files/publications/21252030 Agenda for Sustainable Development web.pdf (Accessed March 1, 2021).
Vollmer, N. I., Gernaey, K. V., Mussatto, S. I., and Sin, G. (2020). Surrogate Modelling Based Uncertainty and Sensitivity Analysis for the Downstream Process Design of a Xylitol Biorefinery. Comput. Aided Chem. Eng. 48, 1663–1668. doi:10.1016/B978-0-12-823377-1.50278-0
Vollmer, N. I., Al, R., Gernaey, K. V., and Sin, G. (2021a). Synergistic Optimization Framework for the Process Synthesis and Design of Biorefineries. Front. Chem. Sci. Eng. 16, 251–273. doi:10.1007/s11705-021-2071-9
Vollmer, N. I., Al, R., and Sin, G. (2021b). Benchmarking of Surrogate Models for the Conceptual Process Design of Biorefineries. Comput. Aided Chem. Eng. 50, 475–480. doi:10.1016/B978-0-323-88506-5.50075-9
Vollmer, N. I., Driessen, J. L. S. P., Yamakawa, C. K., Gernaey, K. V., Mussatto, S. I., and Sin, G. (2021c). Model Development for the Optimization of Operational Conditions of the Pretreatment of Wheat Straw. Chem. Eng. J. 430, 133106. doi:10.1016/j.cej.2021.133106
Vollmer, N. I., Gernaey, K. V., and Sin, G. (2022a). Value Chain Optimization of a Xylitol Biorefinery with Delaunay Triangulation Regression Models. Comput. Aided Chem. Eng. Epub ahead of print.
Vollmer, N. I., Gernaey, K. V., and Sin, G. (2022b). Sensitivity Analysis and Risk Assessment for the In-Silico Design and Use of Optimized Cell Factories in a Xylitol Biorefinery. Comput. Aided Chem. Eng. Epub ahead of print
Vollmer, N. I. (2021). Xylitol Biorefinery. Available at: https://github.com/NikolausVollmer/Xylitol-Biorefinery (Accessed Febrauary 2, 2022).
Wang, W.-C., and Tao, L. (2016). Bio-Jet Fuel Conversion Technologies. Renew. Sustain. Energ. Rev. 53, 801–822. doi:10.1016/j.rser.2015.09.016
Werpy, T., and Petersen, G. (2004). Top Value Added Chemicals from Biomass Volume I. Golden, CO: National Renewable Energy Lab (NREL). doi:10.2172/15008859
Zacher, A. H., Olarte, M. V., Santosa, D. M., Elliott, D. C., and Jones, S. B. (2014). A Review and Perspective of Recent Bio-Oil Hydrotreating Research. Green. Chem. 16, 491–515. doi:10.1039/c3gc41382a
Keywords: biorefinery, xylitol, succinic acid, process design, superstructure optimization, simulation-based optimization, techno-economic analysis, sustainable production
Citation: Vollmer NI, Gernaey KV and Sin G (2022) Conceptual Process Design of an Integrated Xylitol Biorefinery With Value-Added Co-Products. Front. Chem. Eng. 4:838478. doi: 10.3389/fceng.2022.838478
Received: 17 December 2021; Accepted: 26 January 2022;
Published: 10 February 2022.
Edited by:
Aristide Giuliano, ENEA—Centro Ricerche Trisaia, ItalyReviewed by:
Juan Gabriel Segovia Hernandez, University of Guanajuato, MexicoVille Alopaeus, Aalto University, Finland
Copyright © 2022 Vollmer, Gernaey and Sin. This is an open-access article distributed under the terms of the Creative Commons Attribution License (CC BY). The use, distribution or reproduction in other forums is permitted, provided the original author(s) and the copyright owner(s) are credited and that the original publication in this journal is cited, in accordance with accepted academic practice. No use, distribution or reproduction is permitted which does not comply with these terms.
*Correspondence: Gürkan Sin, Z3NpQGt0LmR0dS5kaw==