- 1Graduate School of Pharmaceutical Sciences, The University of Tokyo, Tokyo, Japan
- 2Live Cell Diagnosis, Ltd., Saitama, Japan
- 3Department of Applied Physics and Physico-informatics, Faculty of Science and Technology, Keio University, Yokohama, Kanagawa, Japan
- 4Institute for Protein Research, Osaka University, Osaka, Japan
- 5Graduate School of Science, Osaka University, Osaka, Japan
- 6Department of Applied Genomics, Kazusa DNA Research Institute, Chiba, Japan
- 7Research Center for Advanced Science and Technology, The University of Tokyo, Tokyo, Japan
Heterogeneity in the cellular microenvironment in vivo affects the variability of reactivity among immune cells. Individual-specific microenvironmental differences play a crucial role in determining macroscopic outcomes, such as the efficacy of immunotherapy and disease progression. The microenvironment is also featured by cytokines released from cells, significantly regulating immune cell function. However, the overall understanding, at single-cell resolution, of how cytokines shape the microenvironment and promote paracrine signaling remains unclear. In this manuscript, we propose a methodology that addresses both the microenvironment itself and the response to the microenvironment to comprehend microenvironment behavior at the single-cell level. Our objective is to contribute to the basic understanding of the interplay between immune cells and their microenvironment, with particular relevance to implications for immunotherapy and disease progression.
1 Introduction
The functions of individual cells are known to exhibit significant variability; however, to maintain a healthy body, it is essential for these cellular functions to be expressed at the appropriate times. The living body possesses mechanisms to ensure the appropriate expression of these cellular functions, one of which involves regulation through the microenvironment formed by the cells themselves (Iwasaki and Medzhitov, 2015; Li et al., 2021). The microenvironment refers to the immediate environment surrounding a particular cell and is formed through the production of signaling molecules such as cytokines and chemokines from the cell. These signaling molecules act on nearby cells and tissues, inducing structural changes such as extracellular matrix remodeling and angiogenesis, as well as attracting additional cells. While the formation of a suitable microenvironment supports the maintenance of health, an unsuitable microenvironment can be detrimental to health. This is especially relevant in immunotherapies that leverage immune cells, where controlling the microenvironment is a key focus for minimizing variability in the target immune cell functions and eliciting specific responses. However, because signaling molecules are produced in limited quantities and diffuse freely, they are difficult to detect. As a result, the characteristics of the formation process and the dynamics of microenvironments remain poorly understood, making the development of strategies for microenvironment control challenging (Wang et al., 2023; Rosenberg et al., 2008).
In recent years, a range of advanced analytical techniques that retain spatial information have been developed considering the importance of cellular microenvironment. Spatial omics analysis is a method that comprehensively detects gene or protein expression from individual cells within fixed tissue sections (Alexandrov, Saez-Rodriguez, and Saka, 2023). These advancements have been facilitated by the development of various detection technologies, both in situ and ex situ, as well as by improvements in methods for analyzing spatially structured data, enabling the detection of cell populations with spatially distinctive gene expression patterns (Cui et al., 2024; Singhal et al., 2024). These methods may provide insights into unique cell populations defined by their microenvironment. However, these approaches have limitations as they cannot directly observe the microenvironment itself and only provide snapshot data. This makes it challenging to capture dynamic information, such as changes and developments within the microenvironment.
Flow cytometry analysis using intracellular staining is commonly employed to detect signaling molecules potentially produced by individual cells (Mair and Tosevski, 2014). However, as this method does not retain spatial information, it is inadequate for verifying the microenvironment created by these cells or the microenvironment having influenced these cells, even though it can confirm the presence of specific cytokine-producing cells within a population. Additionally, immunoassays for single-cell analysis, such as ELISPOT and FluoroSpot, are used to detect signaling molecules produced by individual cells (Axelsson, 2022). Nevertheless, these methods do not allow for the simultaneous observation of cells and the signaling molecules they produce, making it challenging to verify whether the produced signaling molecules influence neighboring cells. A method that retains spatial information involves using cells engineered with fluorescent reporter genes for monitoring cytokine expression, which can be effective in imaging studies in vivo, tissue sections and in vitro model systems (Hwang et al., 2013; Parekh et al., 2024; Scheu, Dresing, and Locksley, 2008). However, this method restricts the types of cytokines that can be analyzed. Additionally, as it detects just the initiation of translation, it does not allow for the observation of cytokine release kinetics. More specifically, it does not necessarily confirm that the cytokines have indeed been released.
This paper focuses on the microenvironment generated when macrophages produce interferon-beta (IFN-β). While the role of IFN-β has been extensively studied in the context of viral infection, it is also recognized for its role in forming critical microenvironments during bacterial infections and cancer immunity (Bogdan, Mattner, and Schleicher, 2004; Decker, Müller, and Stockinger, 2005; Qing and Liu, 2023; Vigo et al., 2019; Cheon, Borden, and Stark, 2014; Yu, Zhu, and Chen, 2022). IFN-β activates innate immune cells, including macrophages, natural killer (NK) cells, and dendritic cells, and promotes the production of chemokines, thereby recruiting other immune cells and amplifying the inflammatory response. Thus, IFN-β is considered a key factor in triggering, sustaining and advancing immune responses. At the single-cell level, however, there is significant variability in the expression of Ifnb1, the gene encoding IFN-β, a phenomenon attributed to the stochastic nature of gene expression (Rand et al., 2012; Zhao et al., 2012). Moreover, several single-cell studies on the response of infected cells have shown that, despite other responses to pathogens, many infected cells do not express Ifnb1 (Shalek et al., 2014; Patil et al., 2015). The proportion of Ifnb1-expressing cells suggests that IFN-β-secreting cells are a minority within the total cell population. This implies the existence of strategies that enable a small number of cells to exert systemic effects (Nandakumar, Windross, and Paludan, 2019; Ji, Shi, and Yin, 2024).
To tackle the challenge of elucidating the precise mechanisms underlying the formation of microenvironments at the single-cell level, we propose an innovative methodology for examining the spatial distribution of IFN-β, encompassing both its presence and effects. We employed an integrated FluoroSpot and RNA-fluorescence in situ hybridization (FISH) hybrid assay to visualize IFN-β protein secretion and the response of recipient cells within a population of RAW264.7 mouse macrophage cells stimulated with lipopolysaccharide (LPS). We analyzed microenvironment dynamics using both experimental and simulation data and introduced a novel real-time imaging method, Live Cell Imaging of Secretion Activity (LCI-S) (Shirasaki et al., 2014), to facilitate spatiotemporal evaluation.
2 Materials and methods
2.1 Cell culture
The murine macrophage cell line RAW 264.7 (ATCC TIB-71) was obtained from the American Type Culture Collection (Manassas, VA, United States) and was maintained in DMEM (08458-45, Nacalai Tesque, Kyoto, Japan) supplemented with 100 U/mL penicillin, 100 μg/mL streptomycin (26253-84, Nacalai Tesque), and 10% heat-inactivated fetal bovine serum (10437028, Gibco/Thermo Fisher Scientific, Waltham, MA, United States) at 37°C in a humid atmosphere containing 5% CO2. Cells from passages 5 to 12, following the thawing of the cryostock, were used. In the experiment validating the simulation results, 3 ml of cell suspension was added to each well of a 6-well plate (140675, Nunc/Thermo Fisher Scientific) at the following cell concentrations: Dense: 1.3 × 106 cells/ml, Medium: 1.3 × 105 cells/ml, and Sparse: 1.3 × 104 cells/ml.
2.2 FluoroSpot/RNA-FISH assay
Microscope-grade coverslips were plasma-treated (SEDE-PFA, Meiwafosis) and aminated with Vectabond Reagent (SP-1800, Vector Laboratories). The aminated coverslip was bonded by self-absorption to a polydimethylsiloxane (PDMS) block (Sylgard184, Daw Corning Co.) with a 15-mm-diameter through-hole that served as a cell culture chamber. The glass surface in the chamber was modified with anti-mouse IFN-β antibody (clone# 7F-D3, 7891, Yamasa Corp., Chiba, Japan) using a crosslinker (dimethyl pimelimidate, 21667, Thermo Fisher Scientific) and blocked with monoethanolamine (0.1 M, pH 8.2) and 0.1% Lipidure BL-802 (NOF Corp., Tokyo, Japan). RAW 264.7 cells in a culture dish were retrieved and diluted in medium at 2.0 × 105 cells/mL. A 400-μL aliquot of the cell suspension was introduced into the chamber (=4.5 × 104 cells/cm2) immediately after administration of 100 ng/mL LPS (Sigma, L6529). Cells in the chamber were incubated for 4 h in a CO2 incubator at 37°C. After incubation, the cells were washed three times with prewarmed fresh medium and then the F(ab’)2 fragment of anti-mouse IFN-β antibody (0.25 μg/mL in medium, 32401-1, Pestka Biomedical Laboratories, Inc., Piscataway, NJ, United States) was added. The F(ab’)2 fragment was prepared using a Pierce F(ab’)2 Preparation Kit (44988, Thermo Fisher Scientific). After incubation at 23°C for 20 min, the cells were washed three times with prewarmed fresh medium and stained with Cy3-labeled anti-mouse IgG (30 μg/mL, 115-165-146, Jackson ImmunoResearch Inc., West Grove, PA, United States). After incubation at 23°C for 20 min, the cells were washed three times with prewarmed fresh medium and fixed in 4% paraformaldehyde (15710, Electron Microscopy Sciences, Hatfield, PA, United States). The fixed cells were subjected to RNA-FISH using the QuantiGene ViewRNA ISH Cell Assay (QVC0001, Affymetrix/Thermo Fisher Scientific) with mouse Cxcl10-specific Type 6 probe (Affymetrix ViewRNA probe set, VB6-10663-06) and mouse Gapdh-specific Type 4 probe (VB4-10414-06), following the manufacturer’s instruction.
Bright-field images and fluorescence images of the cells were acquired with an inverted microscope (ECLIPSE Ti-E, Nikon, Tokyo, Japan) equipped with a ×40 objective (S Fluor ×40/0.90 NA, Nikon), an EM-CCD camera (ImagEM, C9100-13, Hamamatsu Photonics K.K., Shizuoka, Japan), and a high-pressure xenon lamp. In total, 400 areas were scanned for bright-field and three fluorescence images (Cxcl10-Alexa 647, Gapdh-Alexa 488, and DAPI).
2.3 Image analysis for FluoroSpot/RNA-FISH assay
Images were analyzed using ImageJ (National Institutes of Health). Briefly, background signal obtained by averaging all images followed by median filtering in each color channel was subtracted from every image in each channel. Cell areas were determined by applying edge detection to the bright field, creating binary images, and were segmented by marker-controlled watershed segmentation using DAPI marker images. In Cxcl10 FISH images with the background subtracted as described above, the mean fluorescence intensity within each cell area was measured and defined as the Cxcl10 mRNA expression level. The mean fluorescence intensity around a cell in background-subtracted IFN-β FluoroSpot images was measured as the concentration of IFN-β. The area around the cell was defined as an area where other cells did not overlap in the external 10 pixels (=∼3 μm) of each cell area.
2.4 Mathematical model
2.4.1 Secretion and diffusion of IFN-β
We considered the situation where N cells start secreting IFN-β at some time point within 4 h of LPS stimulation, and the cells are randomly dispersed on a square area with sides of 20 mm at the bottom of the culture dish (z = 0). The secreted IFN-β molecules follow Fick’s law of diffusion and degrade, with a half-life of 5 h. The spatial dynamics of IFN-β concentration can then be modeled by the following reaction-diffusion equation:
where
where (xi,yi, zi) is the position of the i-th cell, and
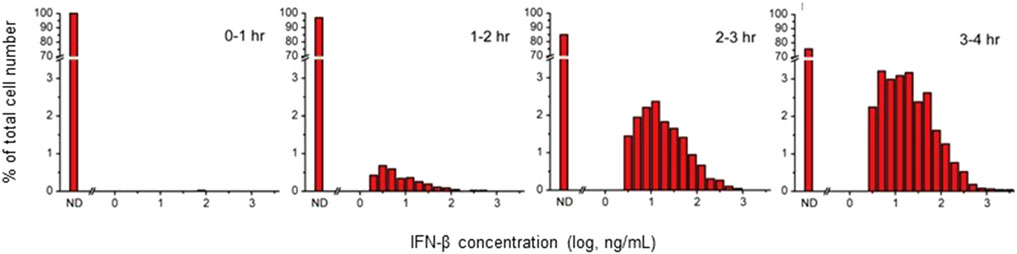
Figure 1. Distribution of IFN-β Secretion from Individual RAW 264.7 Cells Measured Using the Microengraving Method. RAW 264.7 cells cultured at 4.2 × 105 cells/cm2 were stimulated with 100 ng/mL of LPS and recovered at 0, 1, 2, or 3 h after stimulation for introduction into Microengraving chips. The secreted IFN-β from the cells was then captured onto detection slides for an additional hour. The horizontal axis of the histogram represents the amount of secreted IFN-β on a logarithmic scale.
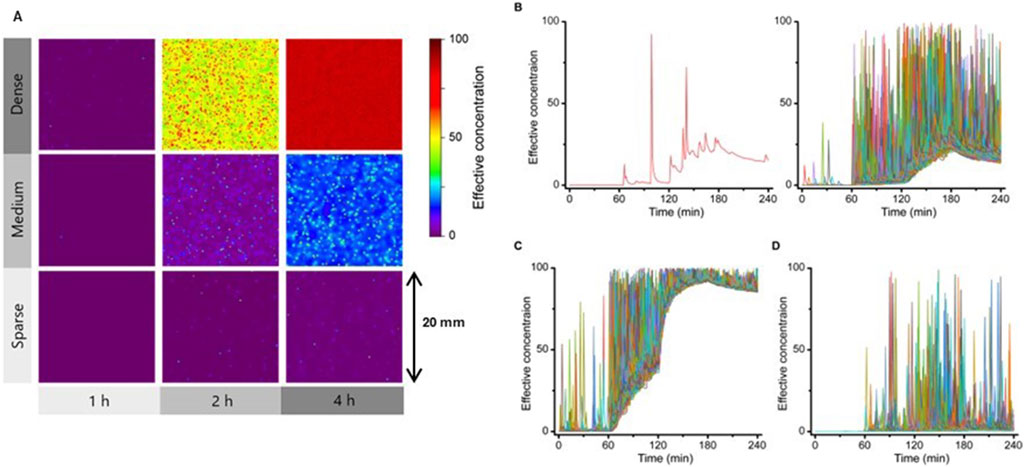
Figure 2. Dynamic time course of the IFN-β concentration field formed by each cell. (A) Simulated results of the time course of the concentration distribution of IFN-β secreted from points placed at high (Dense), medium (Medium) and low (Sparse) density in a 20 mm x 20 mm area. IFN-β distributions at 1, 2 and 4 h after cellular response triggering are shown; IFN-β concentrations are shown in color scale as effective concentrations. (B) Time Course change in IFN-β concentration at an arbitrary point within the simulated region under the Medium condition shown in (A), with a representative example at one point (left) and an overlay of 500 points (right). (C) Overlay from the same 500 points as in (B) under Dense (left) or Sparse (right) conditions.
2.4.2 Stochastic model of Cxcl10 transcription
We produced a simplified stochastic model that simulates the reception of IFN-β signal and the downstream pathway of Cxcl10 mRNA transcription. In the model, we reduced the reaction pathways to two steps, as shown in Figure 3. Intermediate molecules between the reception of IFN-β and Cxcl10 mRNA were reduced to one entity called “Intermediate.” The following stochastic model was obtained based on the chemical master equation (McQuarrie, 1967), which allows for capturing the intrinsic noise due to the low-copy nature of molecules:
where i is the index of reaction specified in Figure 4A, and
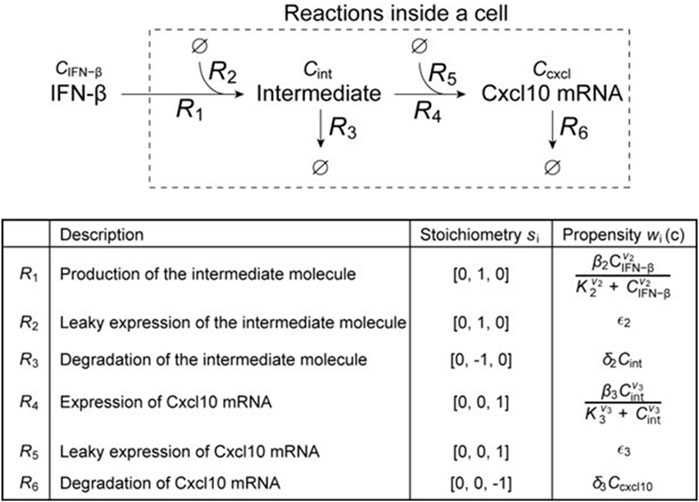
Figure 3. Schematic diagram of a simplified model of intracellular signal transduction after the reception of IFN-β, and details of the reactions defined for each route.
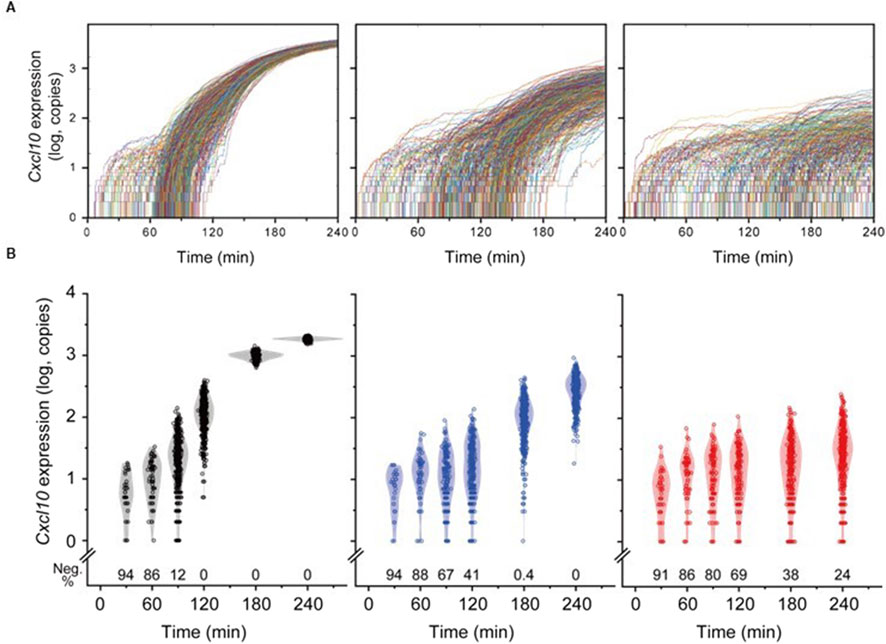
Figure 4. Temporal Variation in Individual Cell Responses to the IFN-β Field Generated by Cells. (A) Temporal changes in Cxcl10 expression copy numbers in individual cells. From left to right, corresponding to Dense, Medium, and Sparse conditions, each trace represents data from a single cell. For each cell density condition, traces from 500 cells were overlaid. (B) Distribution of responses from all cells in Dense (black), Medium (blue), and Sparse (red). Each dot represents the response level of a single cell, overlaid with a kernel smoothing curve. The bottom of each graph shows the percentage of non-responding cells (Neg.%) at each time point.
2.4.3 Parameter Fitting
To determine the parameters, we used least square fitting to the experimental result of single-cell quantitative real-time polymerase chain reaction (qRT-PCR) for recombinant mouse IFN-β (Figures 5, 6). Specifically, as the Ct value for amplification from one copy was around 26 (data not shown), mean normalized Ct values of the positive samples were converted to the copy number of Cxcl10 mRNA molecules by
where
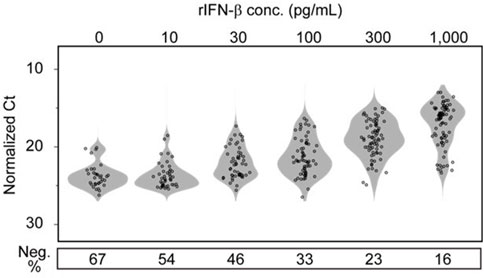
Figure 5. Dose-dependent responses of individual RAW 264.7 cells in terms of Cxcl10 expression to exogenous IFN-β stimulation. RAW 264.7 cells in wells of a 6-well plate in Dense state were stimulated for 1 h by adding recombinant IFN-β to the culture medium at the concentration shown on the upper part of the graph. The response of individual cells to IFN-β was quantitatively evaluated by Cxcl10 expression level in each cell analyzed using sc-qRT-PCR. Cells that did not show signal amplification with qRT-PCR were counted as response negative cells (Neg.) and the ratio is shown in the lower part of the graph.
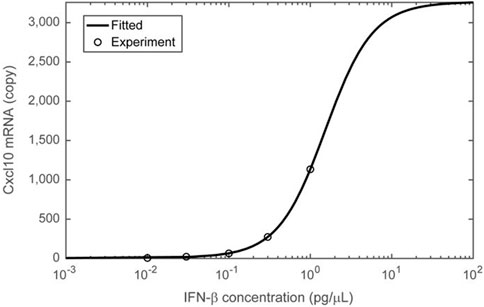
Figure 6. Nonlinear (ultrasensitive) Cxcl10 expression in response to IFN-β stimulation concentrations. The Cxcl10 mRNA copy number, estimated from the results presented in Figure 5, is plotted on the vertical axis, and the concentration of recombinant IFN-β in the medium added during the same experiment is plotted on the horizontal axis. The average values of Cxcl10 mRNA copy number at each concentration are represented by open circles. The Cxcl10 expression response to stimulation at different IFN-β concentrations was fitted using a two-step Hill equation (solid curve), assuming intracellular signaling as depicted in the schematic of Figure 3. Details of the fitting are provided in the Materials and Methods section, and the parameters obtained from the fitting are listed in Table 2.
2.5 Live cell imaging of secretion activity (LCI-S)
In this study, cells were observed using the method outlined at Transducers 2017 (Tanaka et al., 2017). A custom-made waveguide chip, produced by Live Cell Diagnosis Ltd., was employed to monitor secretion activity during cell culture. The chip has a PDMS cell containment chamber on the top surface of the glass optimized for waveguide optics, and the glass surface was aminated. A mixture of capture antibodies (final concentration of 100 μg/mL) and dimethyl pimelimidate-2HCl (final concentration of 7 mg/mL) was loaded into the chamber to fix the capture antibodies on the bottom surface. The remaining reaction groups were blocked with monoethanolamine (0.1 M, pH 8.2). The chip with immobilized antibodies was stored in phosphate-buffered saline supplemented with Lipidure BL802 reagent (0.2% w/v) at 4°C until further use.
The biotin-labeled detection antibody was coupled with either CF660R-labeled streptavidin or Cy3-labeled streptavidin at 1:10 molar ratio in the dark for 2 h. Unoccupied sites on streptavidin were blocked with excess dPEG4-biotin acid (10199; Quanta BioDesign, Ltd., Powell, OH, United States). Unconjugated streptavidin and dPEG4-biotin were removed using ultrafiltration (Amicon Ultra-0.5, 100 kDa; Merck Millipore, Billerica, MA, United States). The detection media containing the prepared CF660R and Cy3-labeled detection antibodies for each antigen were used at a final concentration of 30 nM each.
An aliquot of RAW 264.7 cells was introduced into a chamber of a chip at the frequency of 1.2 × 105/cm2. The culture supernatant was replaced with a freshly prepared detection medium containing 2′3 ′-Cyclic GMP-AMP (cGAMP) at a final concentration of 50 μM immediately before observation. The entire area of the chip chamber was scanned every 20 min on the fully automated inverted fluorescence microscopy (Ti2-E, Nikon) with a high numerical (NA=0.95) ×20 objective lens (CFI Apochromat LWD ×20 WI λS, Nikon), a high-resolution CMOS camera (ORCA-Flash 4.0 v2, Hamamatsu Photonics) and a stage top incubator system (INUBG2TF-WSKM, Tokai Hit), controlled using NIS-Elements software (Nikon).
2.6 Temporal signal deconvolution through image conversion in LCI-S data analysis
The subsequent image analysis method served as a simplified method to estimate secretion activity at a specific time point. Given the frame number at a particular time point as “n,” the average of five consecutive images from “n” to “n+4” was subtracted from the average of five consecutive images from “n-1” to “n-5.” It is important to acknowledge that this method retains a historical context contingent upon the binding speed of the detection antibody.
3 Results
3.1 Visualization of the microenvironment formed by IFN-β utilizing the FluoroSpot/RNA-FISH assay
This study aims to characterize the spatial propagation of functional IFN-β in a model where a specific subset of cells secretes IFN-β within a larger cell population, contributing to microenvironment formation. During the planning stage, we hypothesized that the frequency of IFN-β-producing cells in LPS-stimulated RAW264.7 cells would be moderately low. This assumption was based on the notion that IFN-β production is significantly influenced by the MyD88-dependent pathway. Specifically, the MyD88-independent pathway involved in IFN-β production is activated subsequent to the establishment of the MyD88-dependent pathway, and the IFN-β response is further suppressed by the upregulation of MyD88 (Takeda and Akira, 2004; Kagan et al., 2008; Fitzgerald et al., 2003; Saikh, 2021). Microengraving experiments (Love et al., 2006), outlined in Figure 1, confirmed this hypothesis, showing IFN-β secretion in only a small percentage of cells, reaching only 25% even after 4 h of stimulation.
To assess the microenvironment shaped by IFN-β, we utilized the FluoroSpot/RNA-FISH assay, simultaneously imaging secreted IFN-β and Cxcl10 mRNA expression. Cxcl10 is a gene encoding the chemotactic protein interferon γ-induced protein 10 kDa (IP-10), which is known to be induced by IFN. These two elements constitute a minimal model for microenvironment formation, wherein IFN-β released from cells ultimately recruits various immune cells to the local area. This approach demonstrated that highly Cxcl10 mRNA-expressing cells were frequently observed around substantially IFN-β-secreting cells (Figure 7A). The region where IFN-β was clearly detected was within 50 μm of secreting cells. Apart from this, a few isolated cells sometimes exhibited elevated Cxcl10 expression despite low pericellular IFN-β concentrations, suggesting two possible mechanisms: a low-probability induction by background-level IFN-β or direct induction through the MyD88-dependent pathway.
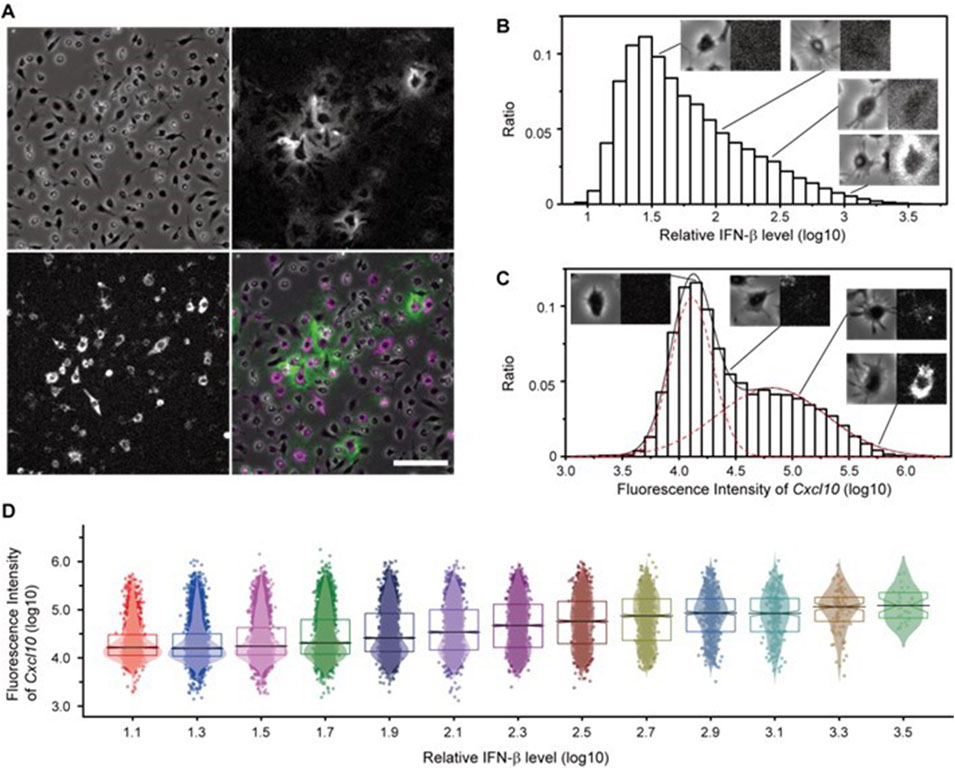
Figure 7. Simultaneous Observation of Secreted IFN-β Proteins and Induced Cellular Responses Using the FluoroSpot/RNA-FISH Assay. (A) Secreted IFN-β protein (green) visualized by the FluoroSpot assay and Cxcl10 mRNA expression (magenta) visualized by RNA-FISH after 4 h of stimulation with LPS. Top left: brightfield image; top right: IFN-β; bottom left: Cxcl10 mRNA; bottom right: merged image. Scale bar = 100 µm. (B) Distribution of pericellular IFN-β intensity measured from FluoroSpot/RNA-FISH images. Each set of microscopic images (left: bright field; right: IFN-β signal) is shown as a representative cell image at the indicated IFN-β levels. (C) Distribution of intracellular Cxcl10 mRNA intensity measured from FluoroSpot/RNA-FISH images. Each set of microscopic images (left: bright field, right: Cxcl10 mRNA signal) is shown as a representative cell image at the indicated Cxcl10 levels. (D) Distribution of cellular response per IFN-β level around the cell. Each dot represents the single-cell response level (Cxcl10 gene expression level), overlaid with a kernel smoothing curve. The black horizontal line represents the median at each IFN-β level, the notch represents the 95% confidence interval around the median, and the boxes represent quartiles.
For quantitative evaluation, we measured pericellular IFN-β and intracellular Cxcl10 signal intensities for each cell, revealing large variability in both (Figures 7B, C). The intensity of the IFN-β signal around cells showed a continuous distribution, indicating not only the presence of a strong IFN-β signal within 50 μm of the secreting cells but also a theoretically weak, continuous distribution extending indefinitely. In contrast, plotting Cxcl10 levels on a logarithmic scale revealed two distinct Gaussian peaks, suggesting the coexistence of cells with signals below the detection limit and a diverse range of expression levels. To determine whether the pericellular IFN-β signal influences Cxcl10 expression cells were stratified based on the intensity of the pericellular IFN-β the bimodal pattern of Cxcl10 expression in each stratum exhibited a tendency toward increased median expression as the IFN-β signal intensity rose. Furthermore, the proportion of cells with undetectable Cxcl10 expression decreased, while the proportion of cells with measurable Cxcl10 expression increased in regions with higher IFN-β signal intensity (Figure 7D). These findings indicate that the quantified IFN-β signal intensity correlates with the ability of cells to sense IFN-β. Specifically, cells with high peripheral IFN-β signal intensity were predominantly those secreting IFN-β or in close proximity to such cells, and the majority of these cells expressed Cxcl10. These results suggest that the Cxcl10 mRNA response is effectively induced in the microenvironment neighboring IFN-β-secreting cells.
3.2 Simulation of spatial propagation of secreted IFN-β
The FluoroSpot/RNA-FISH system may overestimate the localization of IFN-β concentrations and underestimate baseline elevations because of immobilization of secreted IFN-β. Additionally, the need to detect IFN-β secretion signals around individual cells imposes an upper limit on the permissible cell density. To address these limitations, simulations were employed to investigate whether a microenvironment could form under conditions where IFN-β is allowed to diffuse freely. A mathematical model was created to assess spatial distribution and cell responses. The reaction-diffusion model, simulating IFN-β secretion and diffusion based on Fick’s law, utilized parameters from measured data. The simulation results were illustrated as heat maps of IFN-β concentration over time on a 20 mm × 20 mm open plane (Figure 2A). The secretory cell density in “Medium” condition was set to match that in the FluoroSpot experiment. Additionally, simulations were conducted with a “Dense” condition, representing a 10-fold higher cell density, and a “Sparse” condition, representing a 10-fold lower cell density. Results indicated an overall increase in IFN-β levels in both the “Medium” and “Dense” conditions, with a clear local increase in IFN-β concentration observed near the secreting cells across all density conditions.
To further analyze these dynamics, time-dependent changes in IFN-β concentration at specific spatial points were plotted (Figure 2B). A representative point (left) and superimposed plots from 500 randomly selected points (right) revealed transient peaks in IFN-β concentration, likely resulting from direct secretion events, as well as dynamic changes in the baseline level. Under high-density conditions, the baseline IFN-β level increased significantly (Figure 2C), whereas under low-density conditions, the baseline elevation was minimal (Figure 2D). Collectively, these findings suggest that even when IFN-β diffuses freely, transient local increases in concentration occur depending on secretion rates and distances from secreting cells. Moreover, the baseline IFN-β concentration rises as cell density increases, amplifying the microenvironment's overall signaling capacity. In contrast, at lower cell densities, the baseline increase is minimal, and the frequency of these peaks is notably reduced.
A stochastic model simulated cell responses in the IFN-β field described above, revealing a slower response and a wider distribution of maximum response intensity reached at the 4-h time point under the Sparse condition (Figure 4). While all cells in the Medium condition eventually responded, their maxima remained lower and more diverse compared to Dense conditions. The fact that the microenvironment under Sparse or Medium conditions initiated responses, but could not achieve full activity, suggests the necessity of prolonged exposure situations seen in the Dense condition, such as the baseline increase in IFN-β concentration or high frequency of transient exposure, to elicit full activity. In other words, even when the proportion of IFN-β-producing cells is low, accumulating these cells and increasing their local density can serve as an effective strategy to amplify their impact on surrounding cells. The simulation model suggested that although microenvironments can initiate a response, a single transient exposure to IFN-β may not elicit strong individual cell responses. In the Sparse condition, 76% of cells initiated a response after 4 h. Assuming a correlation between the distance from the secretion point and cellular reactivity, it is suggested that the proximity of IFN-β-secretory cells within a radius of 142 μm, where the area inside the circle centered on the secretion point accounts for 76% of the space, was necessary to initiate a response. Furthermore, given that secretory cells constitute 25% of the total cell population, the average distance between cells was approximately 14 μm under Dense conditions, where a uniform and robust response was observed in all cells. In contrast, under Medium conditions, where all responsive cells exhibited a reaction but with low uniformity, the average distance between cells was approximately 46 μm.
3.3 Comparison of simulation and actual measurements
The subsequent experiment aimed to validate the simulation results of the microenvironment formed by freely diffusing IFN-β (Figure 8). RAW 264.7 cells at different densities (Dense, Medium, and Sparse) were stimulated with LPS for 4 h in conventional cell culture plates, and Cxcl10 gene expression was quantified by sc-qRT-PCR. Normalizing target gene expression to Gapdh, all cells in the Dense condition exhibited high Cxcl10 expression (60th percentile range 2.54). In the Sparse condition, 27.9% of cells did not express Cxcl10, and the Ct value was higher than in the Dense condition (60th percentile range 5.35) indicating a lower expression level. The Medium condition showed intermediate results (60th percentile range 4.18, 15.9% of cells), consistent with simulated outcomes. This confirmed that increasing cell density induces higher and more uniform Cxcl10 expression through the paracrine effect of IFN-β.
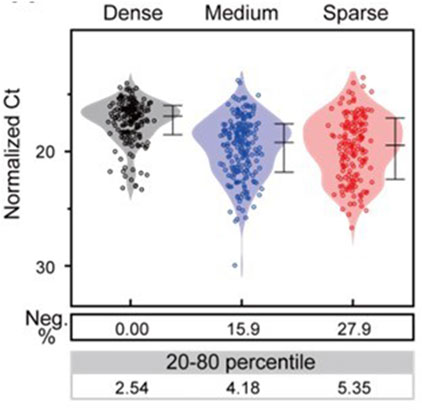
Figure 8. Comparison of the distribution of cellular responses to stimulation at different cell densities. Each dot represents the response level (expression level of the Cxcl10 gene in the normalized Ct value) in each cell, and the kernel smoothing curve was superimposed. Below the dot graph, the negative percentage (Neg. %) and the variation in the expression level (%CV in the linear axis) under each condition are shown.
On the other hand, we did not observe differences in expression levels between Medium and Sparse conditions, which did not fully align with the simulation results. The presence of localized cell aggregation, even seen under Sparse condition, suggests that cellular behaviors such as motility may render the microenvironment created by IFN-β-secreting cells more effective compared to simulated scenarios. This contrasts with the simulations, which assumed completely random resting points for cells. Nevertheless, it is essential to consider the contribution of the MyD88-dependent pathway, which directly induces Cxcl10 expression in LPS-stimulated RAW264.7 cells. To investigate these hypotheses, we are currently endeavoring to conduct time-lapse imaging studies, as detailed in the following section.
3.4 Visualizing the IFN-β microenvironment with LCI-S
We have the belief that spatiotemporal analysis of cellular responses can provide enhanced insights into the cellular microenvironment. Presently, our investigation is centered on determining whether a time series of responses can be revealed through LCI-S—a real-time secretion visualization method that combines FluoroSpot and total internal reflection fluorescence (TIRF) microscopy. LCI-S employs TIRF microscopy to visualize the FluoroSpot signal of IFN-β to differentiate it from excessive fluorescent antibody without washing processes. This approach allows simultaneous detection of IFN-β release while observing cellular dynamics such as movement and enzymatic activity over time, facilitating the analysis of their relationship. In addition, as a parameter for assessing the impact of the IFN-β microenvironment on cells, the secretion of IP-10, the translation product of Cxcl10, was visualized concurrently with the secreted IFN-β. Here, we observed the secretory response of RAW264.7 cells stimulated with cGAMP to avoid complications arising from IFN-β-independent IP-10 secretion via the MyD88-dependent pathway induced by LPS stimulation (see Figure 9 for an example). Following stimulation, IFN-β secretion commenced from certain cells, and subsequently, IP-10 secretion was observed in the surrounding cells. Considering the slow dissociation of the immune complex at the bottom surface, it is reasonable to assume that the observed signal represents cumulative secretory activity.
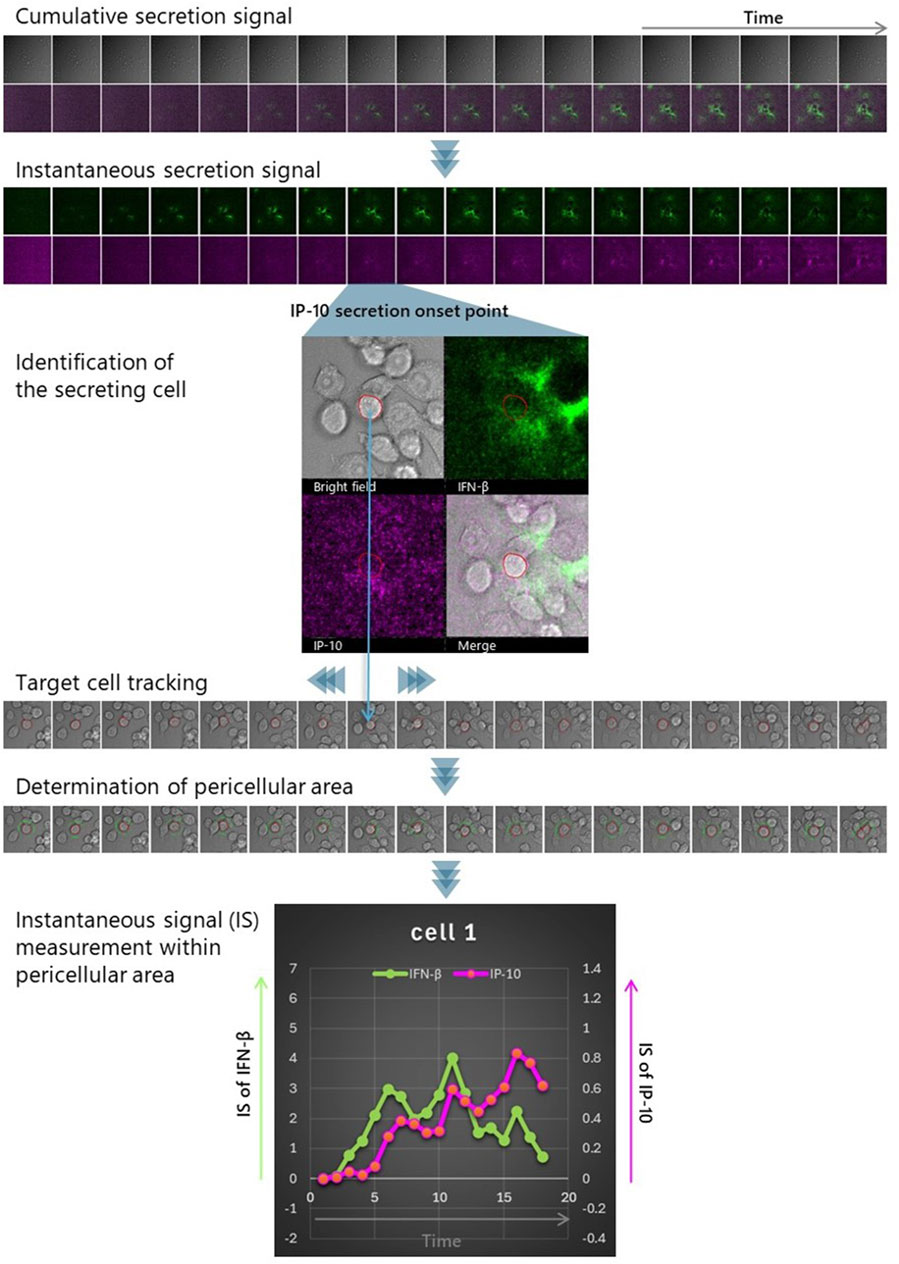
Figure 9. Strategies for dynamically detecting interactions with the microenvironment. LCI-S measurements were conducted on RAW264.7 cells cultured at a density of 1 × 105 cells/cm2 on a TIRF chip. Immediately before starting observation, the culture medium was supplemented with 50 μM cGAMP and detection antibodies for IFN-β and IP-10, labeled with distinct dyes. Time-lapse imaging was performed every 20 min, capturing bright-field images along with IFN-β (green) and IP-10 (magenta) signals (Cumulative secretion signal). Subsequently, after reducing noise by rolling average for 5 frames, difference images were generated from each pair of consecutive images in the time series (Instantaneous secretion signal). Based on these difference images, cells that initiated IP-10 secretion were detected (Identification of the secreting cell), and their movement was tracked using the bright-field images before and after (Target cell tracking). The outlines of the tracked cells at each time point were extracted, and an extended region 3 μm beyond the cell perimeter was defined as the pericellular area (Determination of pericellular area). By measuring changes in the intensity of IFN-β and IP-10 signals within this pericellular area, the exposure history of IP-10-secreting cells to IFN-β can be determined. This allows for the evaluation of the IFN-β microenvironment’s influence on IP-10 secretion, including the intensity and timing of the response (Instantaneous signal (IS) measurement within the pericellular area).
Since the cumulative secretory activity signal represents IFN-β rendered non-functional due to immobilization, we chose to apply basic image processing techniques to isolate the moment of secretion, when functional IFN-β is presumed to be most abundant, and interpret this isolated signal as the functional IFN-β microenvironment (see Methods for details).
The correlation between IP-10 secretion and exposure to active IFN-β was assessed by overlaying the positional history of IP-10-secreting cells onto the calculated IFN-β instantaneous secretion images. We quantified and graphically represented the pericellular IFN-β and IP-10 instantaneous secretion of IP-10 secreting cells, respectively (see Figure 9). This analysis was replicated for the five cells presented, and the resultant changes in pericellular IFN-β and IP-10 signal intensity are illustrated in Figure 10. Notably, in four out of the five cells, a substantial increase in IFN-β signal was observed, preceding the rise in IP-10 secretory signal.
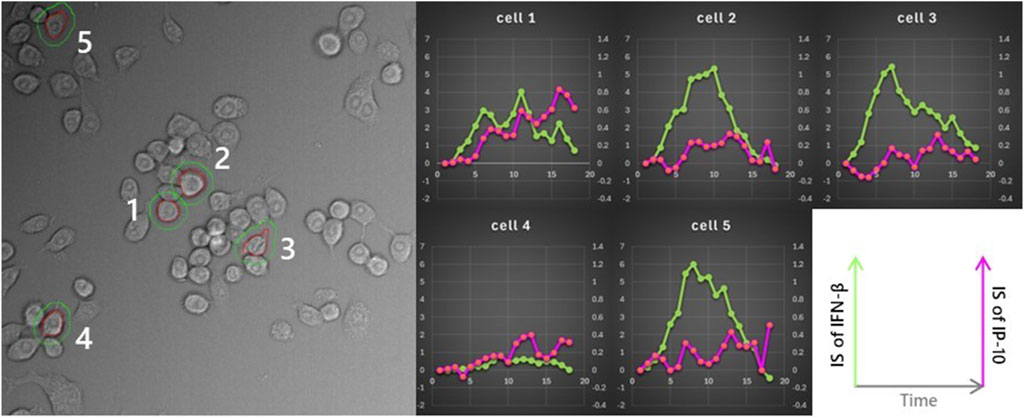
Figure 10. Association between the IFN-β microenvironment perceived by cells and IP-10 secretion. The same analysis was applied to five cells in the same field of view, following the strategy outlined in Figure 9. All cells, except for cell 4, were exposed to high concentrations of IFN-β. However, cell 4 did not exhibit any clear interaction with the microenvironment.
Thus, time-course measurements using LCI-S present a unique method for monitoring dynamic cell-cell interactions mediated by cytokines and elucidating causal relationships. We propose this as an innovative approach to understand the dynamic nature of cellular microenvironments.
4 Discussion
In this paper, we have examined methodologies aimed at elucidating both the microenvironment itself and cellular responses to it, with the goal of advancing the understanding of cellular microenvironments. The analytical approach that preserves spatial information aligns with the principles of spatial omics analysis (Alexandrov, Saez-Rodriguez, and Saka, 2023); however, it differs in that it enables the spatial discussion of causality between locally distinctive cell populations and microenvironment-forming cytokines that may drive the formation of such populations.
First, we underscored the significance of analyses that retain spatial context, exemplified by the FluoroSpot/RNA-FISH assay. Under the conditions applied in this study, the microenvironment created by an individual cell was detectable within a radius of approximately 50 μm. While the Cxcl10 expression response was pronounced within this range, its efficiency declined at greater distances. These findings do not suggest that the minority of IFN-β-producing cells enhanced the activity of the whole cells in the population. This observation is likely attributable to a combination of factors: the decrease in IFN-β concentration as it diffuses three-dimensionally away from the secreting cell, and the ultrasensitivity of cells to IFN-β. However, due to the immobilization of antibodies in the FluoroSpot setup for capturing target signal molecules, there was a potential risk of underestimating the diffusion range and impact of these molecules on cells.
To address this, we integrated single-cell measurements with numerical simulations to gain insights into the microenvironment formed by IFN-β-producing cells. Simulations offer robust solutions to compensate for the compromises made when visualizing certain physical quantities. Nonetheless, it is crucial to recognize that simulations can potentially overestimate or underestimate biological parameters that are not considered, necessitating the acquisition and comparison of real measurements under conditions that closely replicate the simulated setup. Experiments using simulations and actual cell cultures at three different densities demonstrated that when cells were cultured at high density, Cxcl10 mRNA expression was uniformly induced across the cell population, with consistently high expression levels and minimal variability. The strong and uniform response was attributed to either an increased peak frequency, a higher background level, or a combination of both. This suggests that even without an increase in the proportion of IFN-β-secreting cells, the IFN-β microenvironment can effectively influence the entire cell population through localized accumulation.
We subsequently presented a live-cell imaging approach to elucidate the dynamic changes in the microenvironment induced by active cells movements. This method involves spatiotemporal analysis, offering a comprehensive examination that considers cellular secretory functions, motility, and other dynamic properties.
A crucial aspect of the proposed method is the conversion of cumulative signals of secretory activity into instantaneous signals. Simulation of cytokine kinetics motivated us to develop the method. An image analysis method for this conversion, based on the law of mass action, is currently under development and will be presented shortly.
In conclusion, by visualizing the cellular microenvironment using fluorescence live-cell imaging and validating these findings through simulations, we have uncovered how a small subset of cells secreting signaling molecules can create a microenvironment capable of influencing the entire cell population. Our model demonstrates that while signaling molecules secreted by individual cells create a highly localized microenvironment, increasing the frequency of signaling cells through localized cell accumulation effectively activates the entire population. It is well established that various chemokines promote cell localization (Weninger et al., 2014; Natsuaki et al., 2014; Brewitz et al., 2017; Kienle et al., 2021; Khazen et al., 2022). Additionally, recent single-cell secretion analyses have revealed that, in many cell types, only a small fraction of the population exhibits the expected secretion activity (Bucheli et al., 2021; Yamagishi et al., 2024). This low frequency of secretion may be advantageous for the body, as it can serve as a safety mechanism to prevent excessive signal transduction when combined with processes such as chemotaxis and cell aggregation.
Advances in single-cell biology have enabled the acquisition of detailed information at the single-cell level. The future challenge lies in elucidating the principles governing the interactions between the microenvironment and individual cells at the single-cell level. This kind of dynamic analysis including simulations and time-lapse measurements will identify new parameters regulating microenvironment efficacy, which promises to contribute to future medical advancements, such as improvements in cell-based therapies and immunotherapy.
Data availability statement
The raw data supporting the conclusions of this article will be made available by the authors, without undue reservation.
Ethics statement
Ethical approval was not required for the studies on animals in accordance with the local legislation and institutional requirements because only commercially available established cell lines were used.
Author contributions
MY: Conceptualization, Data curation, Formal Analysis, Funding acquisition, Investigation, Methodology, Validation, Visualization, Writing–original draft, Writing–review and editing. YuH: Data curation, Formal Analysis, Investigation, Methodology, Software, Visualization, Writing–original draft, Writing–review and editing. NS: Investigation, Writing–review and editing. YP: Investigation, Writing–review and editing. YoH: Project administration, Writing–review and editing. TF: Project administration, Writing–review and editing. OO: Project administration, Supervision, Writing–review and editing. YS: Conceptualization, Funding acquisition, Methodology, Project administration, Supervision, Writing–review and editing.
Funding
The author(s) declare that financial support was received for the research, authorship, and/or publication of this article. This work was supported by the Naito Foundation (to MY) and partially by Japan Science and Technology Agency (JST), Precursory Research for Embryonic Science and Technology (PRESTO) (Grant Number JP17940748), and JST Moonshot R&D (Grant Number JPMJMS2217-4-2) (to YS).
Acknowledgments
The authors thank the FACS Lab in RCAI (now IMS) of RIKEN for technical assistance on FACS experiments. We thank Tomohiko Okazaki for incisive comments.
Conflict of interest
Authors MY and NS were employed by Live Cell Diagnosis, Ltd.
The remaining authors declare that the research was conducted in the absence of any commercial or financial relationships that could be construed as a potential conflict of interest.
Publisher’s note
All claims expressed in this article are solely those of the authors and do not necessarily represent those of their affiliated organizations, or those of the publisher, the editors and the reviewers. Any product that may be evaluated in this article, or claim that may be made by its manufacturer, is not guaranteed or endorsed by the publisher.
References
Alexandrov, T., Saez Rodriguez, J., and Saka, S. K. (2023). Enablers and challenges of spatial omics, a melting pot of technologies. Mol. Syst. Biol. 19 (11), e10571. doi:10.15252/msb.202110571
Axelsson, B. (2022). Detection and enumeration of cytokine-secreting cells by FluoroSpot. Methods Mol. Biol. 2386, 81–99. doi:10.1007/978-1-0716-1771-7_6
Brewitz, A., Eickhoff, S., Dähling, S., Quast, T., Bedoui, S., Kroczek, R. A., et al. (2017). CD8+ T cells orchestrate PDC-XCR1+ dendritic cell spatial and functional cooperativity to optimize priming. Immunity 46 (2), 205–19. doi:10.1016/J.IMMUNI.2017.01.003/ATTACHMENT/4CEDBF7B-22CC-47F2-8B69-28BFAED3D53B/MMC10.PDF
Bogdan, C., Mattner, J., and Schleicher, U. (2004). The role of type I interferons in non-viral infections. Immunol. Rev. 202 (1), 33–48. doi:10.1111/J.0105-2896.2004.00207.X
Bucheli, O. T. M., Sigvaldadóttir, I., and Eyer, K. (2021). Measuring single-cell protein secretion in immunology: technologies, advances, and applications. Eur. J. Immunol. 51 (6), 1334–47. doi:10.1002/EJI.202048976
Cheon, H., Borden, E. C., and Stark, G. R. (2014). Interferons and their stimulated genes in the tumor microenvironment. Seminars Oncol. 41 (2), 156–173. doi:10.1053/j.seminoncol.2014.02.002
Cui, T., Li, Y. Y., Li, B.-L., Zhang, H., Yu, T.-T., Zhang, J.-N., et al. (2024). SpatialRef: a reference of spatial omics with known spot annotation. Nucleic Acids Res. 1 (1256879), gkae892–14. doi:10.1093/NAR/GKAE892
Decker, T., Müller, M., and Stockinger, S. (2005). The Yin and yang of type I interferon activity in bacterial infection. Nat. Rev. Immunol. 5 (9), 675–687. doi:10.1038/nri1684
Fitzgerald, K. A., Rowe, D. C., Barnes, B. J., Caffrey, D. R., Visintin, A., Latz, E., et al. (2003). LPS-TLR4 signaling to IRF-3/7 and NF-κb involves the toll adapters TRAM and TRIF. J. Exp. Med. 198 (7), 1043–1055. doi:10.1084/JEM.20031023
Gillespie, D. T. (1976). A general method for numerically simulating the stochastic time evolution of coupled chemical reactions. J. Comput. Phys. 22 (4), 403–434. doi:10.1016/0021-9991(76)90041-3
Hwang, S.-Y., Hur, K.-Y., Kim, J.-R., Cho, K.-H., Kim, S.-H., and Yoo, J.-Y. (2013). Biphasic RLR–IFN-β response controls the balance between antiviral immunity and cell damage. J. Immunol. 190 (3), 1192–1200. doi:10.4049/JIMMUNOL.1202326
Iwasaki, A., and Medzhitov, R. (2015). Control of adaptive immunity by the innate immune system. Nat. Immunol. 16 (4), 343–353. doi:10.1038/ni.3123
Ji, S., Shi, Y., and Yin, B (2024). Macrophage barrier in the tumor microenvironment and potential clinical applications. Cell. Commun. Signal. 22 (1), 74–14. doi:10.1186/S12964-023-01424-6
Kagan, J. C., Su, T., Horng, T., Chow, A., Akira, S., and Medzhitov, R. (2008). TRAM couples endocytosis of Toll-like receptor 4 to the induction of interferon-β. Nat. Immunol. 9, 361–368. doi:10.1038/ni1569
Khazen, R., Corre, B., Garcia, Z., Lemaître, F., Bachellier-Bassi, S., d’Enfert, C., et al. (2022). “Spatiotemporal Dynamics of Calcium Signals during Neutrophil Cluster Formation”, in Proceedings of the National Academy of Sciences of the United States of America 119 (29), e2203855119. doi:10.1073/PNAS.2203855119/SUPPL_FILE/PNAS.2203855119.SM11.AVI
Kienle, K., Glaser, K. M., Eickhoff, S., Mihlan, M., Knöpper, K., Reátegui, E., et al. (2021). Neutrophils self-limit swarming to contain bacterial growth in vivo. Science 372 (6548). doi:10.1126/SCIENCE.ABE7729/SUPPL_FILE/ABE7729_KIENLE_SM.PDF
Li, X., Shao, M., Zeng, X., Qian, P., and Huang, H. (2021). Signaling pathways in the regulation of cytokine release syndrome in human diseases and intervention therapy. Signal Transduct. Target. Ther. 6, 367. doi:10.1038/s41392-021-00764-4
Love, J. C., Ronan, J. L., Grotenbreg, G. M., Van Der Veen, A. G., and Ploegh, H. L. (2006). A microengraving method for rapid selection of single cells producing antigen-specific antibodies. Nat. Biotechnol. 24 (6), 703–707. doi:10.1038/nbt1210
Mair, F., and Tosevski, V. (2014). Intracellular staining for cytokines and transcription factors. Methods Mol. Biol. 1193, 39–49. doi:10.1007/978-1-4939-1212-4_5
McQuarrie, D. A. (1967). Stochastic approach to chemical kinetics. J. Appl. Probab. 4 (3), 413–478. doi:10.2307/3212214
Nandakumar, R., Windross, S. J., and Paludan, S. R. (2019). Intercellular communication in the innate immune system through the CGAS-STING pathway. Methods Enzym. 625, 1–11. doi:10.1016/bs.mie.2019.07.007
Natsuaki, Y., Egawa, G., Nakamizo, S., Ono, S., Hanakawa, S., Okada, T., et al. (2014). Perivascular leukocyte clusters are essential for efficient activation of effector t cells in the skin. Nat. Immunol. 15 (11), 1064–69. doi:10.1038/ni.2992
Parekh, N. J., Winship, D., Van Dis, E., and Stetson, D. B. (2024). Regulation and dynamics of IFN-β expression revealed with a knockin reporter mouse. J. Immunol, ji2400227. doi:10.4049/JIMMUNOL.2400227
Patil, S., Fribourg, M., Ge, Y., Batish, M., Tyagi, S., Hayot, F., et al. (2015). Single-cell analysis shows that paracrine signaling by first responder cells shapes the interferon-β response to viral infection. Sci. Signal. 8 (363), ra16. doi:10.1126/scisignal.2005728
Qing, F., and Liu, Z. (2023). Interferon regulatory factor 7 in inflammation, cancer and infection. Front. Immunol. 14, 1190841. doi:10.3389/fimmu.2023.1190841
Rand, U., Rinas, M., Schwerk, J., Nöhren, G., Linnes, M., Kröger, A., et al. (2012). Multi-layered stochasticity and paracrine signal propagation shape the type-I interferon response. Mol. Syst. Biol. 8 (May), 584. doi:10.1038/msb.2012.17
Rosenberg, S. A., Restifo, N. P., Yang, J. C., Morgan, R. A., and Dudley, M. E. (2008). Adoptive cell transfer: a clinical path to effective cancer immunotherapy. Nat. Rev. Cancer 8, 299–308. doi:10.1038/nrc2355
Saikh, K. U. (2021). MyD88 and beyond: a perspective on MyD88-targeted therapeutic approach for modulation of host immunity. Immunol. Res. 69 (2), 117–128. doi:10.1007/s12026-021-09188-2
Scheu, S., Dresing, P., and Locksley, R. M. (2008). Visualization of IFNβ production by plasmacytoid versus conventional dendritic cells under specific stimulation conditions in vivo. Proc. Natl. Acad. Sci. 105 (51), 20416–20421. doi:10.1073/pnas.0808537105
Shalek, A. K., Satija, R., Shuga, J., Trombetta, J. J., Gennert, D., Lu, D., et al. (2014). Single-cell RNA-seq reveals dynamic paracrine control of cellular variation. Nature 510, 363–369. doi:10.1038/nature13437
Shirasaki, Y., Yamagishi, M., Suzuki, N., Izawa, K., Nakahara, A., Mizuno, J., et al. (2014). Real-time single-cell imaging of protein secretion. Sci. Rep. 4 (1), 4736. doi:10.1038/srep04736
Singhal, V., Chou, N., Lee, J., Yue, Y., Liu, J., Chock, W. K., et al. (2024). BANKSY unifies cell typing and tissue domain segmentation for scalable spatial omics data analysis. Nat. Genet. 56, 431–441. doi:10.1038/s41588-024-01664-3
Swaminathan, R., Hoang, C. P., and Verkman, A. S. (1997). Photobleaching recovery and anisotropy decay of green fluorescent protein GFP-S65T in solution and cells: cytoplasmic viscosity probed by green fluorescent protein translational and rotational diffusion. Biophysical J. 72 (4), 1900–1907. doi:10.1016/S0006-3495(97)78835-0
Takeda, K., and Akira, S. (2004). TLR signaling pathways. Seminars Immunol. 16 (1), 3–9. doi:10.1016/j.smim.2003.10.003
Tanaka, Y., Suzuki, N., Mora, K., Mizuno, J., Shoji, S., Uemura, S., et al. (2017). “Widefield real-time single-cell secretion imaging with optical waveguide technique,” in Transducers 2017 - 19th international conference on solid-state sensors, actuators and microsystems. doi:10.1109/TRANSDUCERS.2017.7994363
Terada, N., Tadakuma, H., Ishihama, Y., Yamagishi, M., Zako, T., and Funatsu, T. (2005). Analysis of nuclear microenvironments by translational diffusion of GFP using fluorescence correlation spectroscopy. Bioimages 13, 1–10. doi:10.11169/bioimages.13.1
Terry, B. R., Matthews, E. K., and Haseloff, J. (1995). Molecular characterization of recombinant green fluorescent protein by fluorescence correlation microscopy. Biochem. Biophysical Res. Commun. 217 (1), 21–27. doi:10.1006/BBRC.1995.2740
Vigo, T., La Rocca, C., Faicchia, D., Procaccini, C., Ruggieri, M., Salvetti, M., et al. (2019). IFNβ enhances mesenchymal stromal (Stem) cells immunomodulatory function through STAT1-3 activation and mTOR-associated promotion of glucose metabolism. Cell. Death and Dis. 10 (2), 85. doi:10.1038/s41419-019-1336-4
Wang, Q., Shao, X., Zhang, Y., Zhu, M., Wang, F. X. C., Mu, J., et al. (2023). Role of tumor microenvironment in cancer progression and therapeutic strategy. Cancer Med. 12, 11149–11165. doi:10.1002/cam4.5698
Weninger, W., Biro, M., and Jain, R. (2014). Leukocyte migration in the interstitial space of non-lymphoid organs. Nat. Rev. Immunol. 14 (4), 232–46. doi:10.1038/nri3641
Yamagishi, M., Miyata, K., Kamatani, T., Kabata, H., Baba, R., Tanaka, Y., et al. (2024). Quantitative live-cell imaging of secretion activity reveals dynamic immune responses. IScience 27 (6). doi:10.1016/j.isci.2024.109840
Yu, R., Zhu, B., and Chen, D. (2022). Type I interferon-mediated tumor immunity and its role in immunotherapy. Cell. Mol. Life Sci. 79, 191. doi:10.1007/s00018-022-04219-z
Keywords: cytokine, microenvironment, live-cell imaging, heterogeneity, immune response, IFN-β, simulation
Citation: Yamagishi M, Hori Y, Suzuki N, Peng Y, Harada Y, Funatsu T, Ohara O and Shirasaki Y (2025) Evaluation of the microenvironment formed by interferon-β. Front. Chem. Biol 3:1425571. doi: 10.3389/fchbi.2024.1425571
Received: 30 April 2024; Accepted: 18 November 2024;
Published: 21 January 2025.
Edited by:
Sung Bae Kim, National Institute of Advanced Industrial Science and Technology (AIST), JapanReviewed by:
Melissa Sprachman, Draper Laboratory, United StatesJyothi Sistla, Stony Brook University, United States
Copyright © 2025 Yamagishi, Hori, Suzuki, Peng, Harada, Funatsu, Ohara and Shirasaki. This is an open-access article distributed under the terms of the Creative Commons Attribution License (CC BY). The use, distribution or reproduction in other forums is permitted, provided the original author(s) and the copyright owner(s) are credited and that the original publication in this journal is cited, in accordance with accepted academic practice. No use, distribution or reproduction is permitted which does not comply with these terms.
*Correspondence: Mai Yamagishi, bXlhbWFnaXNoaUBsY2QuY28uanA=; Yoshitaka Shirasaki, c2hpcmFzYWtpQGcuZWNjLnUtdG9reW8uYWMuanA=