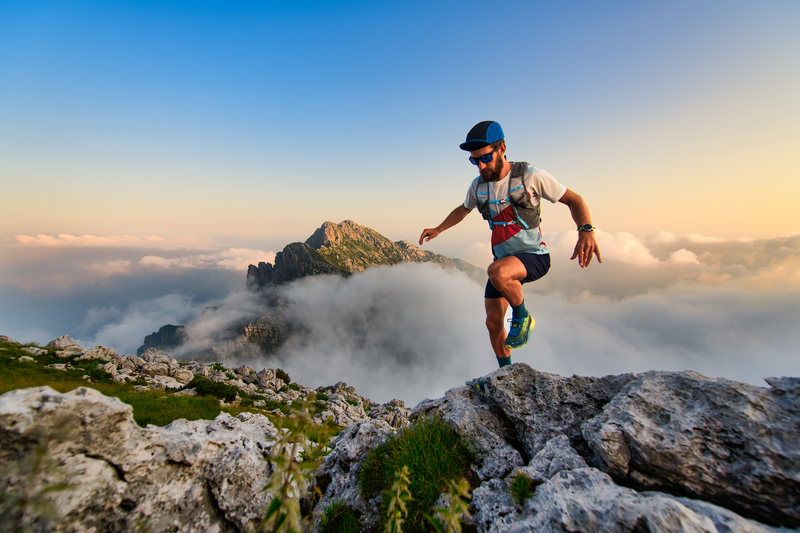
94% of researchers rate our articles as excellent or good
Learn more about the work of our research integrity team to safeguard the quality of each article we publish.
Find out more
MINI REVIEW article
Front. Chem. Biol. , 16 January 2024
Sec. Quantitative and Analytical Techniques
Volume 3 - 2024 | https://doi.org/10.3389/fchbi.2024.1352676
This article is part of the Research Topic Advancements and Challenges in Quantitative and Analytical Techniques: A Comprehensive Overview View all articles
The development of multiplexing technologies for proteomics has enabled the quantification of proteins on a global scale across samples with high confidence. In the covalent ligand discovery pipeline, quantitative proteomics can be used to establish selectivity profiles and provide critical mechanistic insight into the action of lead compounds. Current multiplexing systems allow for the analysis of up to eighteen samples in a single run, allowing proteomic analyses to match the pace of high-throughput covalent ligand discovery workflows. This review discusses several quantitative proteomic techniques and their applications in the field of covalent ligand discovery.
Mass spectrometry-based proteomics has emerged as the established method for protein identification and quantitation in complex biological samples, representing the gold standard in the field. In the realm of covalent drug discovery, chemoproteomics has become an integral component, as it enables the mapping of chemical modifications induced by covalent ligands through proteomics approaches (Meissner et al., 2022). The success of these techniques has revolutionized modern drug-screening workflows by enabling high-throughput and quantitative analysis. This review focuses on elucidating the principles and methodology of various quantitative proteomics techniques, including label-free quantitation, iTRAQ (isobaric tagging for relative and absolute quantification), and TMT (tandem mass tags) labeling. Additionally, we explore the application of these tools in quantitative chemoproteomics, demonstrating their utility in the discovery of covalent ligands.
In global protein identification using mass spectrometry (MS), two main approaches are employed: “top-down” analysis (Siuti and Kelleher, 2007) of intact proteins and “bottom-up” analysis of enzymatic peptide digests. In this review, we will focus on bottom-up approaches. The general workflow (Figure 1A) for sample preparation is as follows (Pappireddi et al., 2019): protein extracts are denatured using urea, followed by reduction of disulfide bridges using a reducing agent like dithiothreitol (DTT) or Tris (2-carboxyethyl) phosphine (TCEP), and alkylation of the resulting sulfhydryl groups with reagents such as iodoacetamide (IA) to prevent bridge reformation. Subsequently, the samples are digested with specific proteases such as trypsin, which cleaves at the C-terminal end of lysine and arginine residues, or other proteases like LysC, ArgC, and GluC, which cleave C-terminal to lysine, arginine, and glutamate residues, respectively. The resulting mixture of peptides can be optionally fractionated prior to sample injection to maximize proteome coverage during the analysis (Manadas et al., 2010).
FIGURE 1. Principles of shotgun proteomics and an overview of isobaric labeling techniques. (A) Upper: The bottom-up proteomics workflow. Flowing sample lysis, protein extracts are processed and subjected to proteolytic digestion. Peptide mixtures are then analyzed by LC-MS/MS. Lower: Sample multiplexing increases throughput. (B) Data-dependent acquisition (DDA) and data-independent acquisition (DIA). (C) Nonisobaric and isobaric labeling approaches. In nonisobaric labeling, sample-encoded peptides appear as distinct peaks in the MS1 spectrum, enabling relative quantification. In isobaric labeling, sample-encoded peptides appear as a single MS1 peak, resulting in no additional MS1 complexity. (D) 4-plex iTRAQ tags. A slash indicates the MS2 fragmentation site that liberates the mass reporter. (E) Upper: Selected structure of tag 128 from the 6-plex TMT set. Lower: Selected structure of tag 129C from the TMT 16/18-plex set. A slash indicates the MS2 fragmentation site that liberates the mass reporter.
Proteomics data is typically obtained using LC-MS/MS (liquid chromatography-tandem mass spectrometry). After chromatographic separation, peptides enter the mass spectrometer, where they undergo ionization into the gas phase through electrospray ionization (ESI). These newly formed “precursor ions” then proceed into the mass analyzer, where they are filtered based on their mass-to-charge ratio (m/z) and subsequently detected. The distribution of m/z values for all precursors at a given time is referred to as the MS1 spectrum, where the height of each peak is proportional to the number of detected ions. Selected peptide ions can then undergo further fragmentation. This step produces the MS2 spectrum, which consists of peptide fragment ions. Analyzing the data from the MS2 spectrum allows for the identification of peptides and enables the mapping of post-translational modifications (Han et al., 2008). To reduce ambiguity in peptide identification, MS2 ions can undergo additional fragmentation, generating MS3 spectra (Olsen and Mann, 2004).
There are two paradigms in bottom-up MS2 data acquisition: data-dependent acquisition (DDA) and data-independent acquisition (DIA) (Figure 1B) (Schubert et al., 2017). In data-dependent acquisition (DDA), precursor ions are selected for further fragmentation based on a “top N” approach. After acquiring a full-scan MS1 spectrum, MS2 scans are obtained for the most abundant precursor ions. DDA provides unbiased coverage of the proteome since the selection process is stochastic. However, this strategy introduces a “missing value problem” because the same precursors may not be fragmented consistently across runs. This limitation becomes problematic in high-throughput applications like fragment-based screening, where numerous samples need to be compared. The data-independent acquisition (DIA) workflow, on the other hand, can mitigate this problem (Aebersold and Mann, 2016). In DIA methods such as SWATH-MS (Messner et al., 2021), precursor ions within preset m/z ranges are fragmented simultaneously, ensuring full and unbiased coverage of the MS1 spectrum. By acquiring all precursor fragmentation data, DIA can largely overcome the missing value problem. However, the resulting MS2 spectra are more complex as they are the superposition of the fragmentation patterns for several precursors. To extract information from DIA spectra, data deconvolution with sophisticated informatics frameworks is necessary (Tsugawa et al., 2015).
Advancements in quantitative multiplexing technologies including label-free, iTRAQ, and TMT approaches have enabled the relative quantification of proteins across multiple samples within a single experiment. These technologies have made MS-based proteomics more compatible with high-throughput drug discovery workflows. Relative abundance information facilitates the comparison of protein levels between different samples, even though precise quantification at an absolute level remains challenging (Ankney et al., 2018). A brief overview of these techniques is presented in Table 1.
TABLE 1. Overview of several quantitative proteomics techniques, including label-free, iTRAQ, and TMT approaches.
Label-free quantification (LFQ) enables the comparison of protein levels between samples without the need for labeling during sample preparation. One approach for LFQ is spectral counting, as the number of acquired MS/MS spectra tends to increase with the relative abundance of a given peptide (Megger et al., 2013; Lee et al., 2019). To establish the relative abundance of peptides with reasonable confidence, various statistical frameworks are available which account for spectral counts, protein length, fragment ion intensities, and the number of unique peptides for each protein. A second strategy for label-free quantification involves utilizing MS1-based quantification of precursor ion intensities (Megger et al., 2013; Smith and Tostengard, 2020). Integrating the intensity of the m/z peak associated with a specific peptide over its chromatographic retention time gives the extracted ion chromatogram (XIC), with the area under the curve representing the ion count for the peptide. Ion count analysis enables the establishment of relative abundance ratios for a particular protein across multiple samples (Cox et al., 2014). Label-free quantification eliminates the need for expensive isotopic tags and additional steps in sample preparation, although it is less accurate than label-based quantification techniques. It provides a straightforward analytical workflow, albeit with relatively lower precision between replicates. Label-free quantification requires consecutive sample runs, resulting in lower throughput. Nevertheless, this method enables the comparison of an unlimited number of samples post-acquisition (Moulder et al., 2016), offering broad utility in large-cohort clinical (Dytfeld et al., 2016) or longitudinal studies (Geyer et al., 2021; Vu et al., 2023).
The introduction of isobaric tags marked a significant breakthrough in quantitative proteomics, primarily due to their enhanced multiplexing capabilities and high quantitative accuracy (Sivanich et al., 2022). Isobaric labeling strategies, including widely available iTRAQ and TMT reagents, involve chemical modification of peptides with isotope-coded tags of the same nominal mass post-digestion, enabling quantitation (Figure 1C). Each isobaric label contains a reporter group, a cleavable linker, a mass balancing group, and a protein reactive group. The unique distribution of isotopes within each tag enables the identification of samples in the MS2 or MS3 spectrum (Chen et al., 2021). Unlike other isotopic labeling techniques, peptides labeled with isobaric tags exhibit a single peak in the MS1 spectrum, allowing for extensive multiplexing without additional MS1 complexity. Fragmentation of the labile linker site at the MS2 or MS3 level generates sample-encoded low m/z reporter ions, along with complementary reporter ions that consist of the mass balancing group attached to the peptide or peptide fragment. Both sets of ions can be utilized for relative quantification, as their masses are unique to each channel within the multiplexed sample (Pappireddi et al., 2019).
Since the labeling step occurs after digestion, isobaric tagging is susceptible to variability from sample handling. Despite the multiplexing limit and the cost associated with commercial tags, this method exhibits high throughput, and sample multiplexing effectively eliminates the missing value problem. MS2-based quantification offers excellent reproducibility and quantitative accuracy. However, this approach is also prone to interference effects caused by contaminating peptides (Ankney et al., 2018). In tandem mass spectrometry, the precursor of interest is often co-fragmented with several near-isobaric peptides, reducing sensitivity between samples to low-abundance peptides. These interference effects can be addressed through post-acquisition computational corrections or experimentally by using MS3-based quantification. By employing a narrow MS3 acquisition window, target reporter ions can be selectively isolated while filtering out unwanted interfering ions (Ting et al., 2011). Additionally, interfacing ion mobility instrumentation to the MS/MS setup can reduce interference by improving m/z separation (Pfammatter et al., 2018).
iTRAQ is available in two variants: a 4-plex and an 8-plex isobaric chemical labeling system. Both systems use a peptide reactive N-hydroxysuccinimide (NHS) ester group, which covalently modifies N-terminal amines and free amino groups of lysine side chains (Figure 1D) (Evans et al., 2012). The 4-plex system generates reporter ions across the m/z range of 114–117, while the 8-plex system generates reporter ions at m/z 113-119 and 121. iTRAQ is compatible with a wide range of samples since the tagging step occurs after digestion. The amine reactivity of the iTRAQ reagent allows for broad peptide labeling. By labeling all sample peptides, the iTRAQ method enables comprehensive proteome coverage and facilitates high-confidence peptide identification, while still retaining valuable post-translational modification (PTM) information. Like all post-digestion labeling methods, iTRAQ is susceptible to loss of precision between replicates resulting from sample handling. However, iTRAQ is broadly compatible with diverse MS platforms, including QToF, ion-trap, and Orbitrap instrumentation (Evans et al., 2012).
TMT (Tandem Mass Tag) is a post-digestion chemical labeling method that can multiplex up to eighteen samples and is commercially available as a 2-plex, 6-plex, 10/11-plex, or 16/18-plex system. The 6-plex and 10/11-plex TMT systems utilize a dimethyl piperidine-based reporter group, which is attached to an amine-reactive NHS-ester group through a labile linker (Figure 1E, upper). The differential distribution of five 13C and 15N atoms within each tag results in reporter ions spanning the m/z range of 126–131. By substituting one 15N atom with 13C, the system can be expanded to 10-plex configuration. In this case, each channel from m/z 127-130 is split by 6 mDa between an N or C variant. The additional 11th channel, labeled with only 13C atoms, completes the 11-plex set, represented by the 131-C variant. For higher multiplexing capabilities, the 16/18-plex TMT systems utilize an isobutyl proline reporter group and a longer linker (Li et al., 2020; Li et al., 2021). These systems accommodate nine differentially distributed isotopes, generating reporter ions from m/z 126-134 upon fragmentation (Figure 1E, lower). While the TMT labeling system is more expensive, it offers a significant advantage in its high multiplexing capacity, which minimizes missing values in large sample datasets. This capability enhances quantitative accuracy and precision without compromising coverage and efficiency in peptide identification. The narrow mass shift between channels in the 18-plex configuration necessitates high-resolution scanning during data acquisition (Pappireddi et al., 2019). This makes TMT labeling less compatible with older instruments that may not be able to achieve adequate resolution for high-multiplex sample deconvolution (Erdjument-Bromage et al., 2018). Nevertheless the robustness and efficiency of the TMT methodology make it particularly appealing for high-volume drug discovery workflows.
High-throughput screening has emerged as a crucial approach in modern drug discovery, facilitating the rapid identification of lead compounds from large small-molecule libraries (Blay et al., 2020). The advancement of quantitative multiplexing technologies has enhanced the utility of proteomics workflows within the drug discovery pipeline. The recent approvals of covalent inhibitors targeting BTK (Pan et al., 2007; Byrd et al., 2013) and the previously considered “undruggable” KRAS-G12C (Ostrem et al., 2013; Kim et al., 2020) have sparked significant interest in drug discovery programs, redirecting attention towards the rational design of covalent drugs. Advanced chemoproteomic methods, such as activity-based protein profiling (ABPP), have been instrumental in identifying numerous reactive cysteines within the ligandable cysteinome (Weerapana et al., 2010; Backus et al., 2016; Vinogradova et al., 2020; Spradlin et al., 2021). The discovery and evaluation of potent and selective covalent ligands using quantitative proteomics techniques have the potential to unlock previously challenging targets and expand the druggable proteome, opening up new avenues in drug discovery (Moellering and Cravatt, 2012).
Gaining insights into the on- and off-target profiles of cysteine-targeting covalent drugs is crucial for understanding their clinical activity and safety. Quantitative proteomics provides an effective platform for this purpose, as it allows for the quantitative and site-specific mapping of protein interactions with the candidate compounds within native biological systems. In a study focused on ibrutinib (Lanning et al., 2014), an FDA-approved covalent inhibitor of Bruton’s tyrosine kinase (BTK) targeting BTK C481 (Pan et al., 2007), an alkynylated probe of ibrutinib was synthesized. This probe was employed for chemical proteomic target profiling in A431 and Ramos cells. Comparison between cells treated with the alkynylated ibrutinib probe and vehicle-treated cells revealed enrichment of BTK, BLK, TEC, ERBB2 kinases, as well as several non-kinase proteins. To confirm the binding and engagement of ibrutinib on the identified proteins, a subsequent competitive proteomic experiment was conducted. In this experiment, the cells were initially pretreated with ibrutinib, followed by treatment with the alkynylated ibrutinib probe. This experiment confirms the ibrutinib’s interaction with the proteins of interest and provided additional evidence for its engagement on these targets. In another study (Ye et al., 2021) focusing on MM2-48, an analog of ibrutinib that exhibits similar BTK inhibition but displays higher cytotoxicity in BTK-dependent cancer cells, alkynylated probes of both ibrutinib and MM2-48 were generated. Quantitative proteomics was utilized to measure the protein targets engaged by these compounds. The results revealed that, in addition to the expected target BTK, MM2-48 engaged C141 on BRCA2 and CDKN1A-interacting protein (BCCIP), which were not bound by ibrutinib. BCCIP is a protein recognized for its significant involvement in cell cycle regulation and DNA double-strand break-induced homologous recombinational repair (Lu et al., 2005). The authors demonstrated that MM2-48, by specifically targeting C141 of BCCIP, displayed a more potent inhibitory effect on homologous recombinational repair compared to ibrutinib. This finding highlights the distinct and enhanced inhibitory properties of MM2-48 in modulating this critical DNA repair mechanism when compared to ibrutinib.
Quantitative proteomics has also been used to evaluate the proteome-wide selectivity of KRAS-G12C inhibitors. KRAS is an oncogenic protein belonging to the small GTPase family and is found to harbor gain-of-function mutations in approximately 30% of human cancers (Simanshu et al., 2017). Historically, RAS proteins have been considered “undruggable” due to their lack of suitable allosteric regulatory sites and their high affinity for binding Guanosine Triphosphate/Guanosine Diphosphate (GTP/GDP). Nevertheless, a unique opportunity arises with the KRas-G12C mutation, which occurs in around 13% of lung adenocarcinomas, opening the door for the development of covalent inhibitors. The Shokat lab embarked on this endeavor by creating an electrophilic small molecule that targets a novel pocket beneath the Switch II loop region of KRas-G12C, specifically in its GDP-bound state, thereby obstructing Son of Sevenless (SOS)-mediated GDP/GTP exchange (Ostrem et al., 2013). In another study, Patricelli and others employed a competitive ABPP approach to quantitatively measure compound engagement on KRAS-G12C within cells. This approach simultaneously enabled the proteome-wide profiling of off-target interactions (Patricelli et al., 2016). Through their work, a compound named ARS-853 emerged, exhibiting potent engagement with the acquired cysteine in KRAS-G12C in NCI-H358 cells, with only two additional targets, FAM213A and RTN4, being identified (Patricelli et al., 2016). Subsequently, an in vivo active KRAS-G12C inhibitor, ARS-1620, was developed and confirmed through the competitive ABPP approach to significantly engage KRAS-G12C within the NCI-H358 proteome (Janes et al., 2018). FAM213A and AHR, two commonly observed targets of covalent compounds bearing an acrylamide warhead, were identified as off-targets of ARS-1620, as well as targets of the inactive ARS-1620 R-atropisomer (Janes et al., 2018). Notably, sotorasib (also known as AMG510) recently became the first FDA-approved KRAS-G12C inhibitor for the treatment of KRAS-G12C-expressing metastatic non-small cell lung cancer (NSCLC) (Nakajima et al., 2022). Importantly, utilizing competitive ABPP, it was found that the acquired cysteine of KRAS-G12C was the sole cysteine substantially engaged by sotorasib within the NCI-H358 proteome (Canon et al., 2019).
The advancement of quantitative multiplexing technologies has revolutionized the field of proteomics, enabling the simultaneous quantification of thousands of proteins across multiple samples with high accuracy and confidence. Sample multiplexing has streamlined data acquisition, facilitating high-throughput analysis while addressing critical challenges such as the missing value problem and ratio distortion. The availability of diverse labeling systems ensures compatibility with various sample types and workflows, making multiplexing a versatile tool in proteomic research. Label-free methodology offers a cost-effective approach for relative quantification between large numbers of samples, which is extremely valuable for clinical proteomic analyses. Isobaric labeling technologies, such as iTRAQ and TMT, allow for a range of sample multiplexing capabilities for high-accuracy relative quantification. In recent years, isobaric labeling techniques have emerged as an attractive strategy in quantitative proteomics. These techniques allow for the encoding of up to 18 samples in a single experiment, offering exceptional precision, accuracy, and dynamic range for quantitative analysis.
In the realm of drug discovery, where high-throughput screening workflows are prevalent, proteomic datasets play a vital role in establishing selectivity and interaction profiles of compounds or providing mechanistic insights into potential hit molecules. Particularly in the domain of covalent ligand discovery, quantitative chemical proteomics has expedited the identification of new ligands, expanding the repertoire of druggable targets. Looking ahead, the continuous evolution of quantitative methodologies will further integrate quantitative proteomics into the standard drug discovery workflow. By leveraging the power of quantitative proteomics, researchers can accelerate the development of groundbreaking therapeutics, leading to more effective treatments and improved patient outcomes.
AB: Writing–original draft, Writing–review and editing. XZ: Writing–original draft, Writing–review and editing.
The author(s) declare financial support was received for the research, authorship, and/or publication of this article. We gratefully acknowledge the support of the NIH (R00 CA248715, T32 GM105538), Damon Runyon Cancer Research Foundation (DFS-53-22), the Ono Pharma Foundation, and the Dr. Ralph and Marian Falk Medical Research Trust.
The authors declare that the research was conducted in the absence of any commercial or financial relationships that could be construed as a potential conflict of interest.
All claims expressed in this article are solely those of the authors and do not necessarily represent those of their affiliated organizations, or those of the publisher, the editors and the reviewers. Any product that may be evaluated in this article, or claim that may be made by its manufacturer, is not guaranteed or endorsed by the publisher.
Aebersold, R., and Mann, M. (2016). Mass-spectrometric exploration of proteome structure and function. Nature 537 (7620), 347–355. doi:10.1038/nature19949
Ankney, J. A., Muneer, A., and Chen, X. (2018). Relative and absolute quantitation in mass spectrometry-based proteomics. Annu. Rev. Anal. Chem. 11 (1), 49–77. doi:10.1146/annurev-anchem-061516-045357
Backus, K. M., Correia, B. E., Lum, K. M., Forli, S., Horning, B. D., Gonzalez-Paez, G. E., et al. (2016). Proteome-wide covalent ligand discovery in native biological systems. Nature 534 (7608), 570–574. doi:10.1038/nature18002
Blay, V., Tolani, B., Ho, S. P., and Arkin, M. R. (2020). High-Throughput Screening: today's biochemical and cell-based approaches. Drug Discov. Today 25 (10), 1807–1821. doi:10.1016/j.drudis.2020.07.024
Byrd, J. C., Furman, R. R., Coutre, S. E., Flinn, I. W., Burger, J. A., Blum, K. A., et al. (2013). Targeting BTK with ibrutinib in relapsed chronic lymphocytic leukemia. N. Engl. J. Med. 369 (1), 32–42. doi:10.1056/nejmoa1215637
Canon, J., Rex, K., Saiki, A. Y., Mohr, C., Cooke, K., Bagal, D., et al. (2019). The clinical KRAS(G12C) inhibitor AMG 510 drives anti-tumour immunity. Nature 575 (7781), 217–223. doi:10.1038/s41586-019-1694-1
Chen, X., Sun, Y., Zhang, T., Shu, L., Roepstorff, P., and Yang, F. (2021). Quantitative proteomics using isobaric labeling: a practical guide. Genomics Proteomics Bioinforma. 19 (5), 689–706. doi:10.1016/j.gpb.2021.08.012
Cox, J., Hein, M. Y., Luber, C. A., Paron, I., Nagaraj, N., and Mann, M. (2014). Accurate proteome-wide label-free quantification by delayed normalization and maximal peptide ratio extraction, termed MaxLFQ. Mol. Cell. Proteomics 13 (9), 2513–2526. doi:10.1074/mcp.m113.031591
Dytfeld, D., Luczak, M., Szczepaniak, T., Przybylowicz-Chalecka, A., Ratajczak, B., Czerwinska-Rybak, J., et al. (2016). Comparative proteomic profiling of sera from patients with refractory multiple myeloma reveals pathways and biomarkers predicting response to bortezomib-based therapy. Blood 128 (22), 2092. doi:10.1182/blood.v128.22.2092.2092
Erdjument-Bromage, H., Huang, F. K., and Neubert, T. A. (2018). Sample preparation for relative quantitation of proteins using tandem mass tags (TMT) and mass spectrometry (MS). Methods Mol. Biol. 1741, 135–149. doi:10.1007/978-1-4939-7659-1_11
Evans, C., Noirel, J., Ow, S. Y., Salim, M., Pereira-Medrano, A. G., Couto, N., et al. (2012). An insight into iTRAQ: where do we stand now? Anal. Bioanal. Chem. 404 (4), 1011–1027. doi:10.1007/s00216-012-5918-6
Geyer, P. E., Arend, F. M., Doll, S., Louiset, M. L., Virreira Winter, S., Müller-Reif, J. B., et al. (2021). High-resolution serum proteome trajectories in COVID-19 reveal patient-specific seroconversion. EMBO Mol. Med. 13 (8), e14167. doi:10.15252/emmm.202114167
Han, X., Aslanian, A., and Yates, J. R. (2008). Mass spectrometry for proteomics. Curr. Opin. Chem. Biol. 12 (5), 483–490. doi:10.1016/j.cbpa.2008.07.024
Janes, M. R., Zhang, J. C., Li, L. S., Hansen, R., Peters, U., Guo, X., et al. (2018). Targeting KRAS mutant cancers with a covalent G12C-specific inhibitor. Cell 172 (3), 578–589.e17. doi:10.1016/j.cell.2018.01.006
Kim, D., Xue, J. Y., and Lito, P. (2020). Targeting KRAS(G12C): from inhibitory mechanism to modulation of antitumor effects in patients. Cell 183 (4), 850–859. doi:10.1016/j.cell.2020.09.044
Lanning, B. R., Whitby, L. R., Dix, M. M., Douhan, J., Gilbert, A. M., Hett, E. C., et al. (2014). A road map to evaluate the proteome-wide selectivity of covalent kinase inhibitors. Nat. Chem. Biol. 10 (9), 760–767. doi:10.1038/nchembio.1582
Lee, H. Y., Kim, E. G., Jung, H. R., Jung, J. W., Kim, H. B., Cho, J. W., et al. (2019). Refinements of LC-MS/MS spectral counting statistics improve quantification of low abundance proteins. Sci. Rep. 9 (1), 13653. doi:10.1038/s41598-019-49665-1
Li, J., Cai, Z., Bomgarden, R. D., Pike, I., Kuhn, K., Rogers, J. C., et al. (2021). TMTpro-18plex: the expanded and complete set of TMTpro reagents for sample multiplexing. J. Proteome Res. 20 (5), 2964–2972. doi:10.1021/acs.jproteome.1c00168
Li, J., Van Vranken, J. G., Pontano Vaites, L., Schweppe, D. K., Huttlin, E. L., Etienne, C., et al. (2020). TMTpro reagents: a set of isobaric labeling mass tags enables simultaneous proteome-wide measurements across 16 samples. Nat. Methods 17 (4), 399–404. doi:10.1038/s41592-020-0781-4
Lu, H., Guo, X., Meng, X., Liu, J., Allen, C., Wray, J., et al. (2005). The BRCA2-interacting protein BCCIP functions in RAD51 and BRCA2 focus formation and homologous recombinational repair. Mol. Cell. Biol. 25 (5), 1949–1957. doi:10.1128/mcb.25.5.1949-1957.2005
Manadas, B., Mendes, V. M., English, J., and Dunn, M. J. (2010). Peptide fractionation in proteomics approaches. Expert Rev. Proteomics 7 (5), 655–663. doi:10.1586/epr.10.46
Megger, D. A., Bracht, T., Meyer, H. E., and Sitek, B. (2013). Label-free quantification in clinical proteomics. Biochimica Biophysica Acta (BBA) - Proteins Proteomics 1834 (8), 1581–1590. doi:10.1016/j.bbapap.2013.04.001
Meissner, F., Geddes-McAlister, J., Mann, M., and Bantscheff, M. (2022). The emerging role of mass spectrometry-based proteomics in drug discovery. Nat. Rev. Drug Discov. 21 (9), 637–654. doi:10.1038/s41573-022-00409-3
Messner, C. B., Demichev, V., Bloomfield, N., Yu, J. S. L., White, M., Kreidl, M., et al. (2021). Ultra-fast proteomics with scanning SWATH. Nat. Biotechnol. 39 (7), 846–854. doi:10.1038/s41587-021-00860-4
Moellering, R. E., and Cravatt, B. F. (2012). How chemoproteomics can enable drug discovery and development. Chem. Biol. 19 (1), 11–22. doi:10.1016/j.chembiol.2012.01.001
Moulder, R., Goo, Y. A., and Goodlett, D. R. (2016). Label-free quantitation for clinical proteomics. Methods Mol. Biol. 1410, 65–76. doi:10.1007/978-1-4939-3524-6_4
Nakajima, E. C., Drezner, N., Li, X. X., Mishra-Kalyani, P. S., Liu, Y. J., Zhao, H., et al. (2022). FDA approval summary: sotorasib for KRAS G12C-mutated metastatic NSCLC. Clin. Cancer Res. 28 (8), 1482–1486. doi:10.1158/1078-0432.ccr-21-3074
Olsen, J. V., and Mann, M. (2004). Improved peptide identification in proteomics by two consecutive stages of mass spectrometric fragmentation. Proc. Natl. Acad. Sci. U. S. A. 101 (37), 13417–13422. doi:10.1073/pnas.0405549101
Ostrem, J. M., Peters, U., Sos, M. L., Wells, J. A., and Shokat, K. M. (2013). K-Ras(G12C) inhibitors allosterically control GTP affinity and effector interactions. Nature 503 (7477), 548–551. doi:10.1038/nature12796
Pan, Z., Scheerens, H., Li, S. J., Schultz, B. E., Sprengeler, P. A., Burrill, L. C., et al. (2007). Discovery of selective irreversible inhibitors for Bruton's tyrosine kinase. ChemMedChem 2 (1), 58–61. doi:10.1002/cmdc.200600221
Pappireddi, N., Martin, L., and Wühr, M. (2019). A review on quantitative multiplexed proteomics. Chembiochem 20 (10), 1210–1224. doi:10.1002/cbic.201800650
Patricelli, M. P., Janes, M. R., Li, L. S., Hansen, R., Peters, U., Kessler, L. V., et al. (2016). Selective inhibition of oncogenic KRAS output with small molecules targeting the inactive state. Cancer Discov. 6 (3), 316–329. doi:10.1158/2159-8290.cd-15-1105
Pfammatter, S., Bonneil, E., McManus, F. P., Prasad, S., Bailey, D. J., Belford, M., et al. (2018). A novel differential ion mobility device expands the depth of proteome coverage and the sensitivity of multiplex proteomic measurements. Mol. Cell. Proteomics 17 (10), 2051–2067. doi:10.1074/mcp.tir118.000862
Schubert, O. T., Röst, H. L., Collins, B. C., Rosenberger, G., and Aebersold, R. (2017). Quantitative proteomics: challenges and opportunities in basic and applied research. Nat. Protoc. 12 (7), 1289–1294. doi:10.1038/nprot.2017.040
Simanshu, D. K., Nissley, D. V., and McCormick, F. (2017). RAS proteins and their regulators in human disease. Cell 170 (1), 17–33. doi:10.1016/j.cell.2017.06.009
Siuti, N., and Kelleher, N. L. (2007). Decoding protein modifications using top-down mass spectrometry. Nat. Methods 4 (10), 817–821. doi:10.1038/nmeth1097
Sivanich, M. K., Gu, T.-J., Tabang, D. N., and Li, L. (2022). Recent advances in isobaric labeling and applications in quantitative proteomics. Proteomics 22 (19-20), 2100256. doi:10.1002/pmic.202100256
Smith, R., and Tostengard, A. R. (2020). Quantitative evaluation of ion chromatogram extraction algorithms. J. Proteome Res. 19 (5), 1953–1964. doi:10.1021/acs.jproteome.9b00768
Spradlin, J. N., Zhang, E., and Nomura, D. K. (2021). Reimagining druggability using chemoproteomic platforms. Acc. Chem. Res. 54 (7), 1801–1813. doi:10.1021/acs.accounts.1c00065
Ting, L., Rad, R., Gygi, S. P., and Haas, W. (2011). MS3 eliminates ratio distortion in isobaric multiplexed quantitative proteomics. Nat. Methods 8 (11), 937–940. doi:10.1038/nmeth.1714
Tsugawa, H., Cajka, T., Kind, T., Ma, Y., Higgins, B., Ikeda, K., et al. (2015). MS-DIAL: data-independent MS/MS deconvolution for comprehensive metabolome analysis. Nat. Methods 12 (6), 523–526. doi:10.1038/nmeth.3393
Vinogradova, E. V., Zhang, X., Remillard, D., Lazar, D. C., Suciu, R. M., Wang, Y., et al. (2020). An activity-guided map of electrophile-cysteine interactions in primary human T cells. Cell 182 (4), 1009–1026.e29. doi:10.1016/j.cell.2020.07.001
Vu, L., Garcia-Mansfield, K., Pompeiano, A., An, J., David-Dirgo, V., Sharma, R., et al. (2023). Proteomics and mathematical modeling of longitudinal CSF differentiates fast versus slow ALS progression. Ann. Clin. Transl. Neurol. 10 (11), 2025–2042. doi:10.1002/acn3.51890
Weerapana, E., Wang, C., Simon, G. M., Richter, F., Khare, S., Dillon, M. B., et al. (2010). Quantitative reactivity profiling predicts functional cysteines in proteomes. Nature 468 (7325), 790–795. doi:10.1038/nature09472
Keywords: proteomics, TMT, label-free quantification (LFQ), iTRAQ, covalent ligand
Citation: Basu AA and Zhang X (2024) Quantitative proteomics and applications in covalent ligand discovery. Front. Chem. Biol 3:1352676. doi: 10.3389/fchbi.2024.1352676
Received: 08 December 2023; Accepted: 08 January 2024;
Published: 16 January 2024.
Edited by:
Rohit Mahar, Hemwati Nandan Bahuguna Garhwal University, IndiaReviewed by:
Parbeen Singh, University of Connecticut, United StatesCopyright © 2024 Basu and Zhang. This is an open-access article distributed under the terms of the Creative Commons Attribution License (CC BY). The use, distribution or reproduction in other forums is permitted, provided the original author(s) and the copyright owner(s) are credited and that the original publication in this journal is cited, in accordance with accepted academic practice. No use, distribution or reproduction is permitted which does not comply with these terms.
*Correspondence: Xiaoyu Zhang, emhhbmdAbm9ydGh3ZXN0ZXJuLmVkdQ==
Disclaimer: All claims expressed in this article are solely those of the authors and do not necessarily represent those of their affiliated organizations, or those of the publisher, the editors and the reviewers. Any product that may be evaluated in this article or claim that may be made by its manufacturer is not guaranteed or endorsed by the publisher.
Research integrity at Frontiers
Learn more about the work of our research integrity team to safeguard the quality of each article we publish.