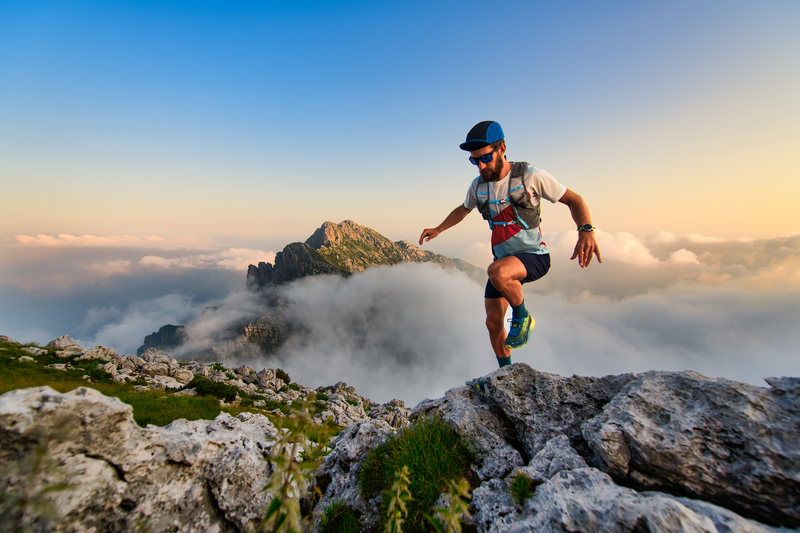
94% of researchers rate our articles as excellent or good
Learn more about the work of our research integrity team to safeguard the quality of each article we publish.
Find out more
ORIGINAL RESEARCH article
Front. Cell. Neurosci.
Sec. Cellular Neurophysiology
Volume 19 - 2025 | doi: 10.3389/fncel.2025.1534839
This article is part of the Research Topic Stress, Mental Health, and the Synapse: Investigating the Mechanisms and Implications View all articles
The final, formatted version of the article will be published soon.
You have multiple emails registered with Frontiers:
Please enter your email address:
If you already have an account, please login
You don't have a Frontiers account ? You can register here
Acute stress results from sudden short-term events, and individuals need to quickly adjust their physiological and psychological to re-establish balance. Chronic stress, on the other hand, results in long-term physiological and psychological burdens due to the continued existence of stressors, making it difficult for individuals to recover and prone to pathological symptoms. Both types of stress can affect working memory and change cognitive function. In this study, we explored the impact of acute and chronic stress on synaptic modulation using a biologically inspired, data-driven rodent prefrontal neural network model. The model consists of a specific number of excitatory and inhibitory neurons that are connected through AMPA, NMDA, and GABA synapses. The study used a short-term recall to simulate working memory tasks and assess the ability of neuronal populations to maintain information over time. The results showed that acute stress can enhance working memory information retention by enhancing AMPA and NMDA synaptic currents. In contrast, chronic stress reduces dendritic spine density and weakens the regulatory effect of GABA currents on working memory tasks. In addition, this structural damage can be complemented by strong connections between excitatory neurons with the same selectivity. These findings provide a reference scheme for understanding the neural basis of working memory under different stress conditions.
Keywords: Short-term (working) memory, short-term plasticity, acute stress, chronic stress, rat, SNN
Received: 28 Nov 2024; Accepted: 25 Feb 2025.
Copyright: © 2025 Du, Sun, Wang, Zhang and Zeng. This is an open-access article distributed under the terms of the Creative Commons Attribution License (CC BY). The use, distribution or reproduction in other forums is permitted, provided the original author(s) or licensor are credited and that the original publication in this journal is cited, in accordance with accepted academic practice. No use, distribution or reproduction is permitted which does not comply with these terms.
* Correspondence:
Chengcheng Du, Chinese Academy of Sciences (CAS), Beijing, China
Disclaimer: All claims expressed in this article are solely those of the authors and do not necessarily represent those of their affiliated organizations, or those of the publisher, the editors and the reviewers. Any product that may be evaluated in this article or claim that may be made by its manufacturer is not guaranteed or endorsed by the publisher.
Research integrity at Frontiers
Learn more about the work of our research integrity team to safeguard the quality of each article we publish.