- 1Department of Molecular Biology and Biochemistry, Simon Fraser University, Burnaby, BC, Canada
- 2Centre for Cell Biology, Development, and Disease, Simon Fraser University, Burnaby, BC, Canada
- 3Institute for Neuroscience and Neurotechnology, Simon Fraser University, Burnaby, BC, Canada
- 4Department of Biological Sciences, Simon Fraser University, Burnaby, BC, Canada
- 5Department of Biomedical Physiology and Kinesiology, Simon Fraser University, Burnaby, BC, Canada
- 6Rotman Research Institute, Baycrest Health Sciences, University of Toronto, Toronto, ON, Canada
Precision, or personalized, medicine aims to stratify patients based on variable pathogenic signatures to optimize the effectiveness of disease prevention and treatment. This approach is favorable in the context of brain disorders, which are often heterogeneous in their pathophysiological features, patterns of disease progression and treatment response, resulting in limited therapeutic standard-of-care. Here we highlight the transformative role that human induced pluripotent stem cell (hiPSC)-derived neural models are poised to play in advancing precision medicine for brain disorders, particularly emerging innovations that improve the relevance of hiPSC models to human physiology. hiPSCs derived from accessible patient somatic cells can produce various neural cell types and tissues; current efforts to increase the complexity of these models, incorporating region-specific neural tissues and non-neural cell types of the brain microenvironment, are providing increasingly relevant insights into human-specific neurobiology. Continued advances in tissue engineering combined with innovations in genomics, high-throughput screening and imaging strengthen the physiological relevance of hiPSC models and thus their ability to uncover disease mechanisms, therapeutic vulnerabilities, and tissue and fluid-based biomarkers that will have real impact on neurological disease treatment. True physiological understanding, however, necessitates integration of hiPSC-neural models with patient biophysical data, including quantitative neuroimaging representations. We discuss recent innovations in cellular neuroscience that can provide these direct connections through generative AI modeling. Our focus is to highlight the great potential of synergy between these emerging innovations to pave the way for personalized medicine becoming a viable option for patients suffering from neuropathologies, particularly rare epileptic and neurodegenerative disorders.
1 Introduction
Precision medicine is a clinical approach that strives to develop targeted treatment paradigms for individual or specific groups of patients based on their unique genetic, molecular, physiological, environmental, and behavioral signatures (Kosorok and Laber, 2019). The promise of human induced pluripotent stem cells (hiPSCs) (Takahashi and Yamanaka, 2006; Takahashi et al., 2007) to play a central role in precision medicine approaches for the diagnosis and treatment of human diseases has been evident since their initial discovery, given that they reflect the full genomic complement of the patient from which they were derived (Agarwal et al., 2008; Gunaseeli et al., 2010; Marchetto et al., 2010; Chun et al., 2011). The synergistic emergence of advanced genome, tissue engineering, and high-throughput analytical approaches, combined with increased clinical accessibility and depth of genome sequencing technologies, is helping to make the promise of hiPSCs as central agents of precision medicine a reality. These advancing technologies allow researchers to model complex neurodevelopmental processes in vitro at cellular and molecular levels and to identify potential pathogenic mechanisms, biomarkers and therapeutic vulnerabilities, all in a patient-specific context. In under two decades (Takahashi and Yamanaka, 2006; Takahashi et al., 2007), hiPSCs have transformed our understanding of human development in both normal and disease contexts and new advances continue to mount at a rapid rate.
hiPSC-based technologies have been particularly impactful for the brain. Given the inaccessibility and scarcity of ex vivo human brain tissues available for study, and a relative lack of congruence between humans and animal models (Jucker, 2010; Knock and Julian, 2021), mechanistic investigation of the human brain has historically been challenging. hiPSCs now provide researchers with an invaluable tool to simply produce human neural cells and ever more complex tissues “in a dish.” Produced from the nuclear reprogramming of highly accessible sources of somatic cells, hiPSCs can be derived from any individual and strategies to establish these cells are becoming increasingly accessible (Liu et al., 2020). The initial somatic cell source used for hiPSC derivation was dermal fibroblasts obtained by punch biopsy of the donor’s skin; however, blood plasma and even urine samples are now more commonly used, making the process simpler and less invasive for donors and clinicians (Sohn et al., 2012). The reprogramming of somatic cells involves the introduction into the donor cells of four transcription factors–OCT4, SOX2, KLF4, and c-MYC–by either nucleofection or, more commonly due to increased efficiency, transduction with viral vectors (Takahashi et al., 2007). As hiPSCs have the same genotype as their somatic cell source obtained from the human donor, they typically carry disease-associated mutations of interest as well as other genetic variants specific to that individual. Isogenic hiPSC lines produced by genomic engineering to repair a disease-causing variant, or hiPSCs obtained from family members with a similar, though typically not identical, germline signature, serve as valuable controls for mechanistic investigations (An et al., 2012; Fujimori et al., 2018; Laperle et al., 2020). These standard methods allow researchers to study the unique impacts of inherited patient-specific mutations in the multiple cell types that can be differentiated from hiPSCs. Although cells and tissues derived from human stem cells cannot, on their own, provide information across all physiological scales, they can illuminate the genetic and molecular features of neurological disorders. With ongoing advances, such as the ability to produce a myriad of neural cell types, more complex region-specific three-dimensional (3D) organoid tissues, and non-neural cell types that are important contributors in the neural niche (Muffat et al., 2016; Abud et al., 2017; Centeno et al., 2018; Wörsdörfer et al., 2019), the mechanisms and causative factors identified will become more likely to serve as valid biomarkers or therapeutic targets. Moreover, the subtle differences in genetic make-up of these cultures makes them the perfect tool to characterize the development and progression of neurological disorders for each patient or specific classes of patients (such as those that share a common rare disease diagnosis).
A wide variety of protocols to generate neural cells and 3D tissues from hiPSCs have been developed (Muratore et al., 2014; Galiakberova and Dashinimaev, 2020; Mayhew and Singhania, 2023), and these vary in their complexity and therefore their degree of fidelity to the molecular, structural and functional heterogeneity of in vivo human brain tissue. These engineered models of the human brain are now routinely used to investigate molecular processes that underly key stages of brain development including stem cell maintenance and fate decisions, neuro- and glio-genesis, and neuronal network formation and synaptic properties (Liu and Zhang, 2011). hiPSC models are also increasingly used to elucidate mechanisms of not only neurodevelopmental but also aging-related diseases (Yagi et al., 2011; Stern et al., 2018; Knock and Julian, 2021). In parallel, methods to precisely modify the genome of hiPSCs, for instance to insert or repair disease-relevant mutations or lineage-tracing markers, and to produce neural cells and tissues in vitro that reflect the complexity of the native environment, have become increasingly sophisticated. Continued innovation in these areas will greatly enrich our ability to uncover both common mechanisms of disease between distinct disorders and to then determine how each patient population, or individuals within these populations, are unique–a central requirement to achieve precision-level medical care.
As summarized in Figure 1, this review will highlight emerging technologies that together have great potential to center hiPSCs as powerful tools for physiological-level modeling of patient- and disease-specific pathological trajectories, and as agents for drug discovery, in neurological disorders. Efforts to consistently improve the depth and physiological relevance of high-throughput screening, neurophysiological analysis approaches, and the region-specific brain organoids and co-culture models (e.g., multi-region neural organoid models or those incorporating non-neural cell types) that can be established will have a particularly profound impact. We posit that a future exists in which hiPSCs are centered as agents to adequately inform clinical understanding and likely treatment trajectories for patients with genetic neurological disorders. However, to realize this goal the increasing repertoire of iPSC-derived human neural tissues, which elevate our ability to model the human brain with high complexity and fidelity, must continue to develop. Additionally, their integration with patient-derived biophysical data is needed. Personalization that spans cells to neural systems, achieved by integrating high resolution hiPSC-derived phenotyping data with multiscale biophysical models established from patient neuroimaging representations, can deepen our understanding of the underlying features of a patient’s disease and clarify complex mechanisms. Measuring cellular phenotypes across timescales, particularly after integration and validation with neurocognitive physiological parameters, may also uncover tissue and fluid-based biomarkers of bona fide disease trajectories and potential treatment approaches. These powerful emerging approaches and the critical advances that will follow from their integration, will transform the ability of researchers and clinicians to unravel the complexities of brain function and dysfunction and pave the way for personalized medicine becoming a viable and effective option for patients suffering from genetic neuropathologies.
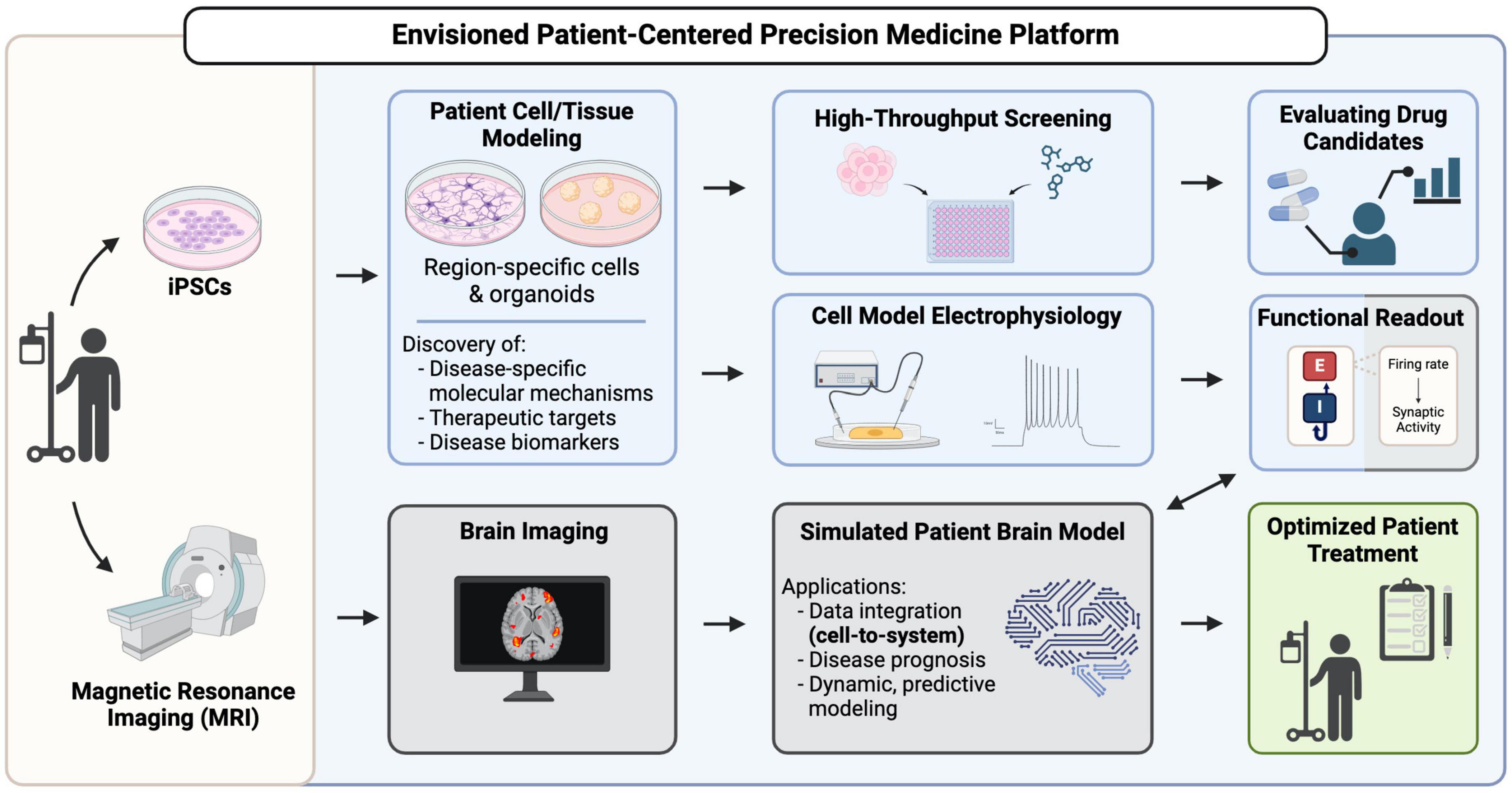
Figure 1. A vision for hiPSCs as key contributors in patient-centered precision medicine platforms. Patient-derived iPSCs are generated from patient somatic cells and are differentiated into brain region-specific cells and organoids for profiling, uncovering disease mechanisms and potential therapeutic targets. High-throughput screening using these patient-derived cells can reveal drug candidates that can go on for further evaluation of their efficacy. Functional readouts from electrophysiological assays, such as excitatory/inhibitory (E/I) balance in different neuronal populations can be integrated with brain imaging data in a simulated patient brain model to ultimately generate a predictive model to inform decision-making in patient diagnosis, prognosis and treatment.
2 A need for increased complexity to improve physiological relevance of hiPSC-based disease models
Human pluripotent stem cell-derived neuronal cultures and organoids have now been used to model numerous neurological disorders with a genetic basis. Early studies were largely focused on common diseases that impact development and aging trajectories like schizophrenia, bipolar disorder, autism syndromes, Alzheimer’s disease (AD) and Parkinson’s disease (PD) (Murai et al., 2016; Ochalek et al., 2017; Lewis et al., 2019; Bame et al., 2020; Laperle et al., 2020). Though more recently, rare genetic diseases have been increasingly represented in hiPSC disease modeling efforts and this focus promises to be especially impactful, both for our understanding of neuropathology in general and for our ability to effectively treat marginalized patient populations (Hosoya et al., 2017; Saito et al., 2018). Rare diseases frequently impact the brain, are typically genetic, and individually affect a small proportion of the human population (each fewer than 1 in 2000 live births) (Umair and Waqas, 2023). Given the large number of rare diseases that are thought to exist (an estimated ∼8000) (Somanadhan et al., 2023) and the small number of patients available for study in each case, there is currently little to no mechanistic understanding for most rare conditions. This reality precludes accurate diagnoses, limits options for effective treatment, and results in a lack of fundamental knowledge about how many genetic disorders impact the form and function of our brains. Though rapid advances in genetic testing are increasingly improving the odds of identifying causative genetic variants for distinct rare conditions, which can then lead to cell and molecular-level mechanistic understanding, this is not always successful. Furthermore, there is often a lack of adequate functional information to validate candidate mutations or determine which gene variant, sometimes among multiple potential candidates, is causing a patient’s disease.
Patient-derived hiPSC-neural models (paired with isogenic or familial controls) have already revealed important mechanistic details, and in some cases have elucidated therapeutic vulnerabilities, for many genetic disorders (Paşca et al., 2011; Liu et al., 2013; Barak et al., 2022). Few, however, have led to true clinical translation, and for most rare diseases hiPSC-derived models have yet to be developed at all. hiPSC approaches to model neural diseases have so far been fragmented, where investigations of pathology from individual groups typically emphasize specific aspects of a disease–such as cellular, physiological, cognitive, or clinical presentation–but lack an integrated view across multiple scales. Additionally, hiPSC-based studies typically assess individual cell types or a single brain region at a time, even though many neurological diseases are multifactorial involving contributions from different cell types and brain regions (Allen and Lyons, 2018; Fessel, 2023). This piecemeal strategy is inadequate to fully model and interrogate genetic brain diseases, as they are typically complex and impact multiple scales of brain form and function.
Establishing stem cell-based models for every rare genetic condition of the brain is a tall order. Though easing the burden, it has been promising to see as more studies as reported that specific pathogenic mechanisms often overlap between different genetic conditions. Furthermore, these overlapping mechanisms are often also observed in more common disorders for which mechanistic understanding is more abundant, and center on organelle dysfunction, altered proteostasis, and changes in the balance of excitatory and inhibitory (E/I) neurophysiological parameters (Reddy, 2009; Maestú et al., 2021; Pradhan and Bellingham, 2021; Wingo et al., 2022). Precision medicine approaches harnessing hiPSC technologies may therefore be accelerated by efforts to first group patients together who share similar disease signatures, even if they span distinct conditions. After this initial stratification, unique disease- or patient-specific phenotypic differences can then be more easily identified. This approach could help to put many patients on a promising diagnostic or treatment path more quickly and could greatly streamline mechanistic and therapeutic discovery endeavors, including identification of patient groups for which clinically approved drugs can be repurposed.
Before hiPSC-based technologies can reliably support disease diagnosis, trajectory predictions and treatment validation in the clinic (Figure 1), new paths to increase the throughput, fidelity, complexity, and physiological relevance of the neural cells and tissues they can produce must continue to emerge. Efforts to consistently improve the depth and accuracy of high-throughput screening and neurophysiological analysis approaches, and the region-specific brain organoids and co-culture models (e.g., multi-region neural organoid models or those incorporating non-neural cell types) that can be established from hiPSCs, will have a particularly profound impact. Technical strategies to analyze the impact of gene variants on cell fate decisions and molecular phenotypes, particularly those that are amenable to fluorescent-based imaging (Murai et al., 2016; Ochalek et al., 2017; Yang et al., 2017), are now quite robust. However, neurophysiological understanding at the cellular level requires quantification of the synaptic activity inherent in these cultures. Direct integration of cellular-level data with patient-derived neuroimaging representations (Figure 1) can be achieved by pairing datasets whose E/I balance parameters are closely matched (Figure 1; Schirner et al., 2018). Thus, we will focus our discussion on integrative cells-to-neural systems modeling of neurological disorders that are characterized by epilepsy and neurodegeneration, which consistently display measurable alterations in synaptic E/I balance. Notably, many neurological conditions–both developmental and aging-related–are marked by epileptic activity or neural tissue degeneration and their underlying mechanisms have been extensively investigated in animal and hiPSC disease models (Solodkin et al., 2011; Falcon et al., 2016; Bavassano et al., 2017; Jirsa et al., 2017; Rousseaux et al., 2018; Hashemi et al., 2020; Hommersom et al., 2022; Schirner et al., 2022; Jirsa et al., 2023). Given the prominence of epilepsy and neurodegeneration among neurological conditions, we therefore predict that the integrated patient-centered modeling platform we envision (Figure 1) will ultimately be amenable to most genetic brain conditions.
2.1 Advances in patient-centered modeling of neurodegenerative disorders
Neurodegenerative diseases (NDDs) are a diverse group of complex conditions characterized by progressive and irreversible dysfunction of the brain due to the continuous loss of specific neuron populations that are susceptible to degeneration (Forman et al., 2004). Animal models of NDDs have enhanced our understanding of the molecular pathogenesis of diseases such as AD, PD, and Huntington’s disease (HD) (Savitt et al., 2006; Yang et al., 2017; Dawson et al., 2018), with the hope of facilitating the discovery of multiple points of therapeutic intervention (Zeiss, 2017). However, findings in model organisms are not always translatable as some NDD pathologies are unique to humans. For example, because AD does not occur naturally in rodents, mouse models require genetic modification to cause the overexpression of human amyloid precursor protein (APP) and presenilin genes associated with familial AD (Sasaguri et al., 2017). The disease also manifests differently than in humans, where although the mice will develop amyloid plaques like those found in patients, they do not exhibit tauopathy or neurodegeneration (Selkoe, 2001). Due to the complexity and heterogeneity of NDDs in humans, animal models cannot fully recapitulate all physiological aspects of these disorders. Thus, there is a significant need for the development of advanced model systems that can effectively address the limitations of current animal models to enhance our mechanistic understanding of neurodegenerative diseases.
To address these concerns, many researchers are increasingly using human-based neuronal models to study NDDs. The synergy of novel hiPSC technologies with developments in genome-editing and sequencing has allowed for the investigation of cellular mechanisms during NDD pathogenesis. hiPSCs can also allow for the study of neurological diseases that don’t have underlying mutations yet identified, as patient-derived hiPSCs retain the full genetic information of any germline and sometimes somatic mutations, providing researchers with a tool to also study sporadic diseases. In the case of Amyotrophic Lateral Sclerosis (ALS), a heterogenous motor neuron disease which causes the progressive degeneration of motor neurons in the spinal cord and brain (Cluskey and Ramsden, 2001), 90–95% of cases are sporadic (sALS) (Kiernan et al., 2011), meaning they are not inherited unlike familial ALS (fALS). Before the development of hiPSCs, it was difficult to establish useful models of sALS. Now, not only are researchers able to establish human cellular models of sALS, but they have also used them to identify a therapeutic agent, ropinirole, which showed protective effects in both their fALS and sALS cell models (Fujimori et al., 2018). This discovery led to phase 1/2a clinical trials in patients with sALS testing ropinirole (Morimoto et al., 2023), where ropinirole was shown to slow disease progression, resulting in slower functional decline when patients were treated with the drug earlier. While further efforts are required to uncover the precise mechanism of action for ropinirole in ALS, this body of work demonstrates that hiPSC culture models have the capability to be used in precision medicine pipelines to identify drug candidates and predict the responsiveness of patients to treatments (Farkhondeh et al., 2019; Okano and Morimoto, 2022).
The bulk of hiPSC-neural modeling studies to date have centered on models of the forebrain. However, it is well known that in vivo, NDDs often involve heterogenous representations across multiple brain regions. The forebrain may be involved in some pathological process, but it is not always the primary tissue region affected with other brain regions often displaying the first signs of the degeneration cascade. This means that many existing human cell models of NDD do not address the appropriate cell and tissue types. Recent innovations that are transforming our ability to produce region-specific brain organoids, particularly the cerebellum, are starting to fill this critical gap (Silva et al., 2020; Atamian et al., 2024). For example, the cerebellum is an important contributor in neural atrophy disorders such as Familial Ataxia Syndromes (Silva et al., 2020), Multiple System Atrophy (MSA) (Ciolli et al., 2014), and Creutzfeldt-Jakob Disease (CJD) (Zerr and Parchi, 2018). New findings suggest that cerebellar gray matter atrophy is also involved in many conditions previously attributed to the degeneration of the cerebral cortex such as AD, PD and Frontotemporal Dementia (FTD) (Guo et al., 2016; Gellersen et al., 2017; Seidel et al., 2017), suggesting that a more thorough investigation of the cerebellum can be insightful for human NDD modeling.
One such example of cerebellar atrophy disorders are the spinocerebellar ataxias (SCAs), a group of over 40 rare hereditary progressive movement disorders that primarily affect neurons in the hindbrain and cerebellum, with involvement in some forms in the spinal cord and cerebral cortex (Klockgether et al., 2019; Chirino-Pérez et al., 2021; Guo et al., 2023; Pilotto et al., 2023). To study this class of NDDs in a human model, researchers can use hiPSCs derived from both healthy individuals and SCA patients to generate cerebellar organoids, which can consistently reproduce multiple types of functional cerebellar neurons (Atamian et al., 2024). This very recent establishment of a functional human model of the cerebellum is an integral step to improving our understanding of human neurophysiology and pathology, as studies have shown that the human cerebellum differs drastically from that of rodents. For example, in contrast to mouse, the human cerebellum has more outer radial glia in an outer subventricular zone during development that drives human cerebellar expansion and gyrification (Nowakowski et al., 2016). Other differences in human cerebellum development are in rhombic lip (RL) morphology and the existence of substructure zones such as ventricular (RLVZ) and subventricular zones (RLSVZ), as well as internalization of the RL into the posterior lobule to form a tightly packed pool of cells (Haldipur et al., 2019). Differences in development ultimately translate to a different mature tissue, highlighting the importance of a human-based model to capture all aspects of neurophysiology and pathology in the cerebellum. While it can be argued that because hiPSC models elucidate mechanisms of disease in an earlier developmental model they might not be well suited for modeling diseases that arise later in adulthood, there is evidence to suggest that events during early prodromal stages in these developmental models can shed light on the acquisition of late-stage syndromic phenotypes (Caldwell et al., 2015; Tremlett and Marrie, 2021). This prodromal window may also be a more suitable therapeutic window to recover brain homeostasis.
The recent development of brainstem organoids (Eura et al., 2020; Lui et al., 2023), an area of extensive atrophy in many patients with SCA, will help to further develop appropriately complex models for this class of NDDs. Incorporating multiple brain region-specific organoids that are relevant to a given NDD–for example forebrain, cerebellum and brainstem–can permit analysis of degenerative mechanisms both within and between distinct affected regions. The rapid advance of brain organoid assembloid technologies (further detailed below), where unique types of organoids are physically connected to permit observation and assessment of neuronal network formation across brain regions, is substantially supporting efforts to improve physiological relevance in neurological disease models.
2.2 Advances in patient-centered epilepsy modeling
Epilepsy, a condition characterized by persistent predisposition to experiencing seizures, is thought to affect a staggering ∼1% of the population, or about 80 million people worldwide (Beghi, 2020). Epilepsy can arise due to one of many genetically defined conditions or, more often, as a secondary feature of other neuropathological conditions or from unknown causes. The exact mechanisms driving the underlying neural network dysfunction in these disorders largely remain to be uncovered (Perucca and Perucca, 2019), but in vitro modeling of inherited epilepsy disorders with hiPSCs has opened new doors for mechanistic investigations and drug discovery. The added value emerging from hiPSC-derived neural epilepsy models again highlights the limitations of existing animal models to capture certain human-specific features, which are critical to the establishment of altered brain architecture and synaptic networks that underlie these syndromes (Blair et al., 2018; Knock and Julian, 2021; Eichmuller et al., 2022). As with NDDs, in many epilepsies a genetic cause is presumed but the gene variant is unknown. hiPSC approaches to model brain development and neural network function from epileptic patients can therefore inform pathogenic mechanisms both for patients with well-defined mutations and for those whose genetic cause is not yet identified. In the latter case, the presence of epileptic phenotypes in hiPSC neural models derived from patients, compared to those from familial controls, can help to diagnose individuals by establishing if their syndrome does indeed have a genetic basis.
Many genetic epilepsies exist, but we will focus on a select few for which hiPSC models have provided significant advances–Dravet syndrome, a severe myoclonic epilepsy characterized by febrile seizures that begin in infancy, and the class of disorders termed malformations of cortical development (MCDs) (Chen et al., 2014; Costa et al., 2016; Sun and Dolmetsch, 2018; Majolo et al., 2019; Klofas et al., 2020). MCD describes numerous disorders that are characterized by altered brain development causing architectural and neuronal network abnormalities, which almost invariably lead to altered cognition and severe epilepsy that are typically treatment resistant (Oegema et al., 2020). Tuberous sclerosis, focal cortical dysplasia, and megalencephaly are among the most commonly studied MCDs, and hiPSC-derived in vitro models for these conditions continue to improve in their fidelity and capacity to model disease-relevant features (Li et al., 2017; Avansini et al., 2022; Eichmuller et al., 2022).
Given the relative ease of electrophysiological analyses in pure neuronal cultures, hiPSC-based models of epilepsy have predominantly focused on the derivation and functional assessment of 2D cultures of neurons, sometimes also including astrocytes. Typically, these are telencephalic forebrain neurons given the propensity of basic neuronal differentiation protocols to yield forebrain cells (Muratore et al., 2014). Efforts to model Dravet syndrome with patient-derived hiPSCs, which carry a deleterious mutation in the Nav1.1 sodium channel, indicate that cultures of telencephalic interneurons carrying this variant have reduced sodium current density and action potential output; however, no phenotypic change was observed in excitatory neurons (Sun et al., 2016). These results are in line with previous findings in a mouse model of Dravet syndrome (Yu et al., 2006) and highlight differences in the contribution of distinct neuronal subtypes to the epileptic state in Dravet patients. Although only in 2D culture models, these results showcase the potential of hiPSCs to translate cellular-level phenotypes to clinical presentation, and further suggest the potential to evaluate the efficacy of potential therapeutic compounds at the cellular level. hiPSC models have similarly been used to shed light on the antiepileptic properties of cannabidiol (CBD) in Dravet syndrome and to study its mechanism of action. Researchers administered CBD to telencephalic neurons produced from patient-derived hiPSCs, which increased the excitability of inhibitory neurons and decreased the excitability of excitatory neurons, without altering sodium channel currents in these cell types (Sun and Dolmetsch, 2018). These observations indicate that the effect of CBD is also targeted to specific neuronal subtypes and is independent of sodium channel activity.
MCDs stand as the leading cause of medically refractory epilepsy in children (Oegema et al., 2020). In adults, the MCD Focal Cortical Dysplasia ranks as the third most common cause of medically intractable seizures (Kabat and Król, 2012). Molecular analysis of neurons from patients with Focal Cortical Dysplasia Type II identified dysregulation of several genes associated with neuronal migration during neurogenesis and embryonic neural progenitor cell differentiation, which is a key factor that underlies development of the malformed cortical tissue within the brains of these patients that underlies their epileptic activity (Majolo et al., 2019). hiPSCs carrying loss-of-function mutations in DEPDC5, encoding a GATOR1 complex member, show overactivation of the mammalian target of rapamycin complex 1 (mTORC1) signaling node, resulting in epileptic episodes which can be alleviated by administering the mTORC1 inhibitor rapamycin (Klofas et al., 2020). A growing number of studies indicate that mTORC1 plays a significant role in many neurological diseases due to its involvement in autophagy, proteostasis, cell proliferation and migration, and its interactions with multiple signaling pathways that modify the cell’s energetic state, neurotransmitters, and growth factors (Cuyàs et al., 2014; Guo et al., 2018).
mTORC1 disruption is heavily implicated in multiple forms of MCDs, including megalencephaly disorders and tuberous sclerosis (TS) which is caused by mutations in the TSC1 or TSC2 genes (Hernandez et al., 2007; Kashii et al., 2023), leading to persistent mTORC1 hyperactivation. Disruption of the mTORC1 pathway leads to formation of benign tumors and generalized tissue malformations in multiple organs including the brain (Delaney et al., 2014; Crino, 2020; Delaney et al., 2020; Dhaliwal et al., 2024). Epilepsy manifests in up to 90% of TS patients (Rocktäschel et al., 2019), which is due to the presence of these low grade architecturally abnormal tumors or “cortical tubers” (Delaney et al., 2014; Frost and Hulbert, 2015). Patients with TS inherit a mutation in one allele of TSC1 or TSC2 and subsequent loss of heterozygosity in a subset of cells gives rise to focal regions of malformed brain tissue. Complete loss of TSC1 or TSC2 is therefore required to observe the full spectrum of disease-relevant phenotypes. Mouse models are not fully sufficient to investigate the molecular mechanisms of tuber formation, since homozygous germline TSC1 or TSC2 mutants are embryonic lethal due to failure of neural tube closure (Kobayashi et al., 1999; Møller et al., 2016), and various conditional knockouts fail to recapitulate the architecture of cortical tubers. The approach of differentiating neurons and glia from human pluripotent stem cells has proven to be an ideal and unique tool to investigate how TSC1 or TSC2 loss impacts the development of neural precursors, neurons, and glia, and the neural networks that they form, in the human brain (Nadadhur et al., 2019; Delaney et al., 2020). Reports on hiPSC-derived neuronal cultures have shown that deletion of TSC2 leads to structural abnormalities in neuroectodermal rosettes, reminiscent of the defective neural tube closure observed in vivo (Costa et al., 2016; Winden et al., 2019). More directly relevant to epileptic phenotypes, molecular, cellular, and electrophysiological characteristics of dysplastic neurons from cortical tubers induced by deletion of TSC2 have been routinely measured in forebrain neuronal cultures and rescued by the mTORC1 inhibitor rapamycin, a derivative of the leading pharmaceutical treatment for TS (Costa et al., 2016; Nadadhur et al., 2019; Winden et al., 2019; Delaney et al., 2020). Despite these advances in 2D neuronal cultures, TS is a profound example of the importance of 3D organoid modeling to more accurately reflect human disease phenotypes, as only in cerebral organoids has the architecture of cortical tubers been recapitulated (Blair et al., 2018; Eichmuller et al., 2022). The synergy of these hiPSC-derived neural cultures with advances in the electrophysiological assays discussed in the following section make them an ideal tool to study the impacts of potential drug therapeutics on epilepsy phenotypes in vitro.
3 Advancing hiPSC neural circuitry measurements with region-specific brain organoids
Our understanding of the early stages of neural circuitry development in humans has long been impeded by a lack of access to fetal and neonatal brain tissue. Obvious ethical concerns surrounding the use of such tissues to directly observe and manipulate developing neural circuitry have precipitated the use of alternative animal models to uncover key insights into human brain development. Rodents are widely used due to their genetic tractability and well-characterized neuroanatomy (Glowinski and Iversen, 1966). Non-human primate models are also used, albeit more sparingly, as they are closer to humans in terms of brain complexity and behavior (Feng et al., 2020). Additionally, zebrafish have transparent embryos that allow visualization of live early neural development (Sakai et al., 2018), while fruit flies and C. elegans have a simple nervous system, providing a more manageable model for exploring neural circuits (Sengupta and Samuel, 2009; Bellen et al., 2010). While the use of animal models has been essential to understanding the basic nature of brain disorders that impact humans and continue to be an invaluable tool, they also come with their own disadvantages. Confounding factors such as captivity and housing conditions, which can cause stress and impacts on animal welfare, can negatively impact the validity and reproducibility of experimental results. It is also important to note that despite some similarities between humans and animal models, there is a lack of congruence to many features of the developing and adult human brain, which is thought to largely underlie the substantial issue of translational failure as promising therapeutic strategies are moved from experimental models to the clinical domain (Marshall et al., 2023).
The introduction of hiPSC-derived neural models, superseded by human embryonic stem cells (hESCs), in the last 20 years (Takahashi and Yamanaka, 2006; Marchetto et al., 2010; Cheung et al., 2011) has provided a profound tool to study neural circuitry in early human development, when in the past we have mostly been limited to postmortem tissues and neuroimaging data (Manzini et al., 2021). As a result, researchers are now able to use electrophysiological tools to assess the functional attributes of neurons in culture, including: (1) regular and high-density multi-electrode arrays (MEA) for prolonged, non-destructive recordings of electrical activity and network dynamics (Negri et al., 2020; Habibey et al., 2022); (2) patch clamping for direct measurement of neuronal activity (Bardy et al., 2016); (3) calcium imaging using fluorescent calcium indicators to monitor changes in intracellular calcium levels, serving as a proxy of neuronal activity (Estévez-Priego et al., 2023); (4) voltage-sensitive dye imaging using fluorescent dyes that change their fluorescent properties in response to changes in membrane potential (Glover et al., 2008); and (5) optogenetics, harnessing genetically engineered neurons that express light-sensitive proteins called opsins, permitting selective activation or inhibition of neurons by light stimulation to manipulate neuronal activity in vitro (Deisseroth et al., 2006). It is important to note that these 2D models lack native tissue architecture and complex intercellular interactions, which poses a challenge to studying neurodevelopment in vitro (Adlakha, 2023). The development of the 3D neural organoid culture method has provided a platform for the investigation of brain development in a more complex tissue environment. Many of the previously mentioned electrophysiological assays are now being applied to investigate neuronal function in 3D brain organoid models (Birey et al., 2017; Xiang et al., 2017; Fair et al., 2020; Tasnim and Liu, 2022; Yang et al., 2024). The cellular diversity of brain organoids allows researchers to study the diverse interactions that contribute to neural circuit function. Additionally, as these organoids can be maintained in culture for extended periods, including recent advances with organoid transplantation into mouse brain tissues to permit more advanced human neuronal cell and electrophysiological development (Revah et al., 2022; Kelley et al., 2024) researchers can study the development and maturation of neural circuit network dynamics over time (Fair et al., 2020). These 3D systems have been used to model disorders with known electrophysiological abnormalities, such as epilepsy (Hirose et al., 2020; Nieto-Estévez and Hsieh, 2020; Saberi et al., 2022), and can be further applied to substantiate therapeutic testing via high throughput drug screening applications.
To study the interactions between cells from different brain regions, researchers have developed co-culture methods to combine brain-region specific organoids into a single integrated model (Figure 2A; Bagley et al., 2017; Xiang et al., 2017). Since the introduction of these methods, there have been many multi-region organoid co-culture models generated to enable human neural circuitry studies in a dish to investigate complex neuropathophysiologies. The combination of these various neural organoids has allowed researchers to study key developmental processes in a more physiologically relevant model, such as interneuron migration and various neural circuitry projections. Table 1 lists some of these models that have been developed in recent years.
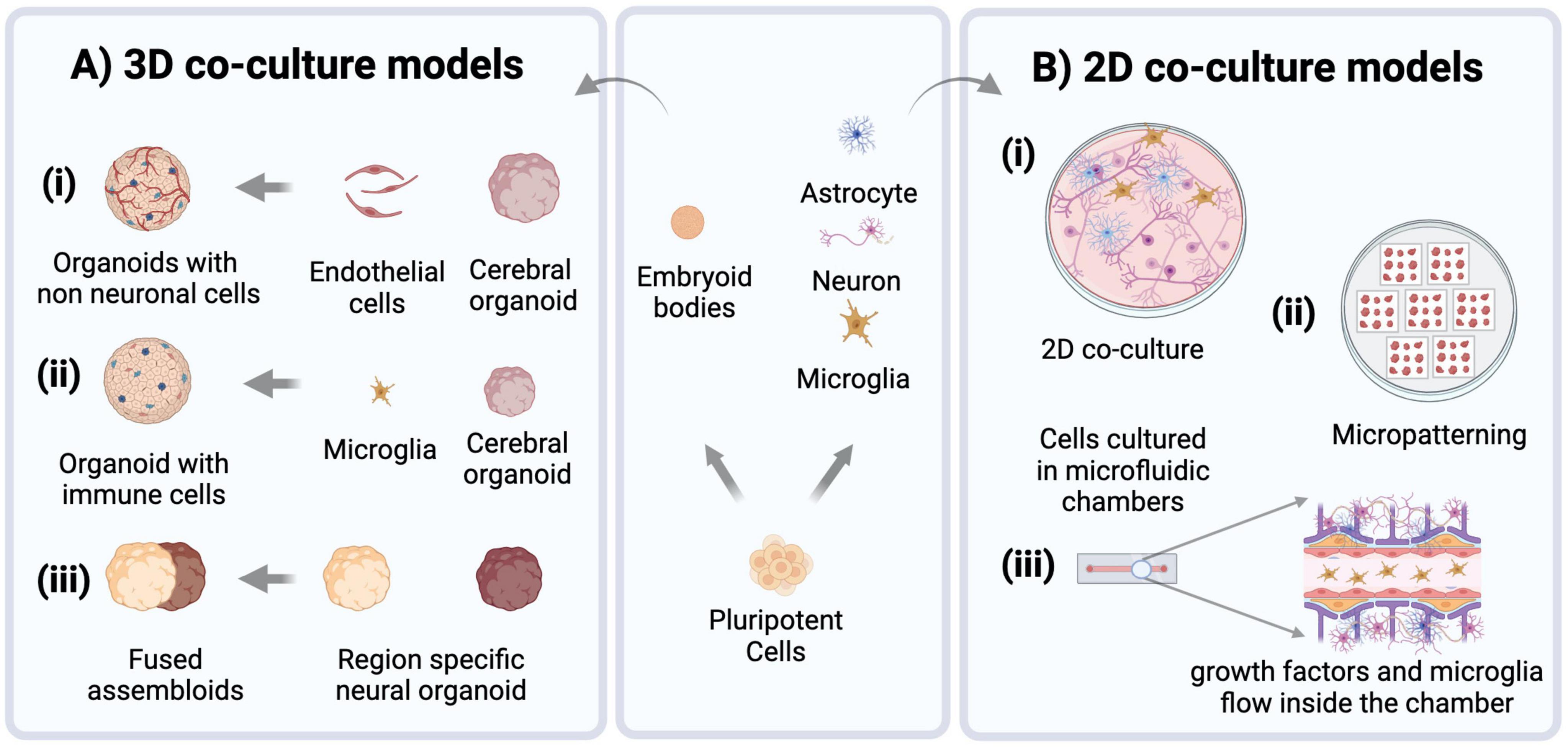
Figure 2. Modeling approaches for brain disorders using hiPSCs. Pluripotent stem cells are routinely used to derive embryoid bodies for heterogenous 3D organoid production, or cultures of neural and non-neural (e.g., microglia) cells that can then be combined to establish co-cultures of specified cell types (center panel). (A) 3D brain organoid models increasingly use co-culture approaches to enhance their physiological relevance by incorporating non-neural cell types such as (i) endothelial cells (ii) microglia, and (iii) generating region-specific organoids which can be fused to create assembloids. (B) 2D co-culture models: (i) culturing neurons, microglia, and astrocytes in a dish. (ii) Micropatterns, Cells are patterned into arrays of specific shapes and sizes to control their spatial organization. (iii) Microfluidic models of brain tissue are created to control the flow of nutrients and incorporate non-neural cell types through small scale channels.
The electrophysiology data collected from these multi-region assembloid models allows for a detailed assessment of neural cell and circuit interactions between different brain regions, particularly as inhibitory interneurons integrate within excitatory neuron networks in the dorsal and ventral forebrain organoid co-culture model, a phenomenon that is highly reflective of neural circuit development in the human brain.
4 Advancing understanding of the brain microenvironment with co-culture of non-neural cell types
In vitro research on the human brain has primarily centered around neural cell types, including neurons, astrocytes and oligodendrocytes. However, when aiming to establish platforms to facilitate effective precision medicine strategies, which should mirror the in vivo condition as closely as possible, it is crucial to acknowledge the critical role that non-neural cells play in brain physiology and pathology. These cells can include pericytes and endothelial cells, which constitute the blood-brain-barrier. One of the most prominent non-neural cell types in the brain, which have emerged as particularly impactful regulatory cells across the lifespan, are brain-specific immune cells called microglia (Colonna and Butovsky, 2017; Mehl et al., 2022). During human brain development, microglia originate from primitive myeloid progenitors derived from the yolk sac. These progenitors migrate into the brain early during development, where they then differentiate into functional microglial cells. Microglia constitute approximately 5–16% of the total cell population in a fully developed brain and are maintained throughout life through self-renewing divisions (Masuda et al., 2020). As brain resident immune cells, microglia play a significant role in normal brain development and homeostasis. They are found to accumulate near dead neurons and are involved in phagocytosis of cell debris and degenerating axons. This role is more evident in a state of neural injury or stroke (Colonna and Butovsky, 2017). Microglia have also been associated with the stem cell niche in the brain at the subventricular zone and neocortex, where they regulate the differentiation of neural stem cells by selective cell engulfment (Xu et al., 2021). Moreover, they regulate the formation of neural circuits and maintain a balance of excitatory and inhibitory neurons by affecting inhibitory interneuron migration (Bohlen et al., 2019; Mehl et al., 2022). In addition to these widespread roles, microglia affect neuronal function through their crosstalk with other glial cells (Thion et al., 2018).
Microglia are morphologically dynamic, ranging from thin, ramified structures under normal physiological conditions and transitioning to an activated state and hypertrophic morphology in response to certain stimuli (such as injury, infection, or neuroinflammation). In the active state, they show increased proliferation while secreting inflammatory factors such as chemokines and cytokines, which profoundly affect brain function [reviewed in Bohlen et al. (2019)]. Their activation, therefore, impacts brain physiology at many levels including neural circuits, cell populations, synapses, and neurotransmitter signaling (Bohlen et al., 2019; Mehl et al., 2022). Considering the many key functions they carry out, it is no surprise that microglia have a significant role in brain disorders spanning psychiatric conditions, such as schizophrenia, bipolar disorder, and autism, to neurodegenerative diseases like AD, PD and ataxias (Willis et al., 2020; Silvin et al., 2022; Tagliatti et al., 2024). In vitro models that lack microglia often present clear limitations in their ability to fully elucidate the mechanisms underlying neurological disorders. Thus, integrating immune cells like microglia into human neural cell models is paramount, and overlooking their contribution can result in significant gaps in our understanding of complex regulatory mechanisms in brain development and disease pathogenesis (Ronaldson and Davis, 2020; du Chatinier et al., 2023).
For ex vivo studies, researchers typically isolate primary microglia from postmortem human, rodent or non-human primate brain tissue (Roqué and Costa, 2017). The use of primary microglia comes with certain limitations, including inconsistent phenotypes due to the positive disease status of the donor, altered activation states during the process of isolation, and, in the case of humans, lack of access to high-quality human postmortem tissue (Timmerman et al., 2018). Immortalized microglial cell lines, such as BV2 (derived from mouse), HMC3 (derived from human embryo), and CHME-5 (derived from adult human tissue) are also available (Dello Russo et al., 2018; Tian et al., 2019). While convenient, these cell lines show heterogeneity, genetic instability, and differences in differentiation state, which may impact their relevance to in vivo conditions (Das et al., 2016; Aktories et al., 2022). In addition to primary and immortalized microglia, protocols have been designed to differentiate or “induce” microglia (iMG) from embryonic or induced pluripotent stem cells (Speicher et al., 2019). iMG generated by these protocols resemble in vivo microglia in the context of their ability to phagocytose exogenous substances and respond to immune stimulation (Abud et al., 2017).
Microglia exhibit distinct responses to their microenvironment when cultured in 3D as opposed to traditional 2D cultures. In 3D environments, microglia display altered morphology, enhanced motility, and differential gene expression profiles compared to their 2D counterparts, reflecting the influence of spatial cues and cell-cell interactions on microglia behavior (Luchena et al., 2022). Therefore, 3D cultures with microglia (Figure 2A) can better recapitulate aspects of tissue architecture and cellular organization, providing a more accurate model for studying microglia behavior and function in vitro and their impacts on the developing brain. iMGs have been shown to incorporate into brain organoid tissue when co-cultured with them and show functional relevance to in vivo microglia post-integration (Abud et al., 2017). Xu et al. (2021) showed that iMG integrated into cerebral organoids engulf neural progenitor cells (NPCs), apoptotic cells, and neuronal synapses. iMGs when co-cultured with brain-specific organoids (i.e., with dorsal and ventral forebrain organoids), displayed different migration ability, intracellular Ca2 + signaling, and responses to pro-inflammatory stimuli (with higher expression of TNF-α and TREM2 in the ventral organoid-microglia group) (Song et al., 2019). Additionally, the transcriptome profile of iMGs cultured with dorsal brain organoids differs from that of ventral brain organoids, demonstrating that they are capable of responding to different neural niches in vitro (Hasselmann et al., 2019).
Microglia are also essential for proper development and function of the retina, and thus play a crucial role in the pathogenesis and progression of retinal disorders. The native state of retinal organoids is not captured accurately if microglia are absent. Therefore, to precisely represent the native retina, hiPSC-derived retinal organoids containing microglia are developed either by direct co-culture or by differentiating microglia in parallel within the organoids (Bartalska et al., 2022; Gao et al., 2022; Usui-Ouchi et al., 2023).
Microglia have also been incorporated into more defined engineered models to study their interaction with neural cells more precisely (Figure 2). These include microfluidic devices that allow dynamic control over microenvironmental factors and replication of in vivo nutrient gradients. Organ-on-a-chip models, which incorporate microfluidics to mimic physiological conditions of the brain and involve more intricate modeling of brain tissue, for example components of neural tissue juxtaposed with cells of the blood-brain-barrier (BBB) (Amirifar et al., 2022). Tissue micropatterning harnesses the power of growth restriction to pattern cells into self-assembled structures that reflect in vivo tissue organization, and interestingly microglia show structural diversity when grown on different micropatterned surfaces (Amadio et al., 2013). This approach can help to correlate the structural differences of microglia with their distinct functional identities. Co-culturing microglia with micropatterned neural tissue can reveal how microglia respond to different patterns of neural organization, providing insights into various subpopulations and their roles in development (Figure 2B). Simulation models further complement these approaches by enabling the computational study of microglial functions, such as their response to stress or inflammation and integrating that information into predictive models of brain function and disease. Ao et al. (2021) developed an organoid-on-chip model by synthesizing a device in a tubular shape where EBs are cultured to form a tubular organoid with an inner channel for the flow of nutrients and non-neural cells such as microglia, thus offering comprehensive insights into their functions and cellular interactions. Similarly, Pediaditakis et al. (2022) synthesized a microfluidic model to culture neurons, microglia, astrocytes and pericytes together, creating a neurovascular unit to study neuron and glia interactions in the brain microenvironment. Amadio et al. (2013) showed that microglia can move through microfluidic channels and travel up to 55 μm within 12 h. Culturing microglia on micropatterned surfaces and restricting their growth spatially can help to obtain more reproducible phenotypes. It has been shown that microglia possess distinct morphologies when cultured on different biomimetic micropatterned surfaces with different shapes correlating to diverse functional identities (Amadio et al., 2013). Finally, to achieve an integrated understanding of biological processes, computational models are also employed [as reviewed in Anderson and Vadigepalli (2016)]. Modeling studies demonstrate a strong correlation between microglial morphology and their function. The introduction of microglia and cytokine signaling into brain simulation models can further the knowledge of their plasticity and variability and the impact of different microglia states on brain form and function (Anderson and Vadigepalli, 2016). The neuroinflammatory environment can be created by co-culturing microglia with neurons and astrocytes, but to capture physiologically relevant microglial phenotypes more robust models must be created. These models should involve use of vasculature, endothelial cells and spatial cues to understand role of microglia in health and disease (Figure 2).
5 hiPSC-derived models as tools to identify disease-associated biomarkers
Disease-specific biomarkers have a crucial role in precision medicine, as they can help to identify specific characteristics that indicate the presence of progression of a particular disease, allowing clinicians to make accurate diagnoses, categorize patients into subtypes based on their biomarker signature and ultimately develop targeted interventions and treatments. Biomarkers can exist in the form of specific proteins, metabolites, genetic mutations, physiological parameters (e.g., blood pressure) or even brain imaging patterns that indicate a patient’s prognosis, in addition to the stage of disease pathogenesis and progression (Chen et al., 2011). Although the use of biomarkers in precision medicine has been mostly applied in oncological indications, such as estrogen receptor, progesterone receptor, and human epidermal growth factor receptor 2 (ER/PR/HER2) for breast cancer (Gamble et al., 2021), applications to a variety of target organs - including the brain - do exist.
Common neurodegenerative diseases such as AD affect millions of people worldwide and are especially prevalent clinically in the aging population. To make an accurate and differential diagnosis, a combination of structural and functional imaging, genetic, blood-based and cerebrospinal fluid (CSF) biomarkers are used by clinicians. In the case of AD, increased CSF levels of total-tau and phosphorylated-tau protein along with decreased levels of CSF Aβ1−42 create the typical AD biomarker profile found in most AD patients (Bouwman et al., 2022). While these CSF biomarkers represent the gold standard for the molecular characterization of neurodegenerative diseases, collecting CSF from patients for long-term observation is not feasible due to its invasive nature (Koníěková et al., 2022). While blood-based biomarkers exist for these neurological diseases, accurately measuring them poses a challenge as these brain-derived biomarkers are present at low concentrations in this fluid due to the blood-brain barrier (Zetterberg and Burnham, 2019). Additionally, certain biomarkers for AD may have potential interference from heterophilic antibodies and can be at risk of proteolytic degradation by proteases in blood plasma (Zetterberg and Burnham, 2019). Due to these considerations, an alternative source for brain-derived biomarkers is needed to enable further discovery and characterization for patients with rare neuropathophysiologies (Zetterberg and Burnham, 2019).
A recent technology describing the generation of human barrier-forming choroid plexus (ChP) neural organoids capable of producing CSF-like fluid (Pellegrini et al., 2020) may present a potential solution to the challenges with CSF-based biomarkers. Organoids are defined as in vitro cellular models formed via self-organization, including multiple organ-specific cell types that demonstrate functional and cytoarchitectural traits associated with a specific organ (Paşca et al., 2022). Fitting this definition, ChP organoids demonstrate key features of the human ChP, forming tight barriers that selectively regulate the entry of small molecules, and secrete a fluid akin to human CSF that contains proteins and known molecular biomarkers in self-contained cystic structures (Pellegrini et al., 2020). The generation of these organoids from hiPSC lines can present an alternative source for patient-derived CSF samples, limiting the need for invasive procedures such as lumbar punctures to collect this fluid. There is evidence to suggest that ChP organoids at day 60 of development and beyond can demonstrate functionality of adult ChP, with the production of a mature hiPSC-derived in vitro cerebral spinal fluid (iCSF) by 100 days (Pellegrini et al., 2020). iCSF can be harvested at multiple timepoints to track changes of the presence and levels of proteins and inflammatory cytokines found within the iCSF, providing insight to the progression of disease. For example, mass spectrometry is a commonly used technique to perform intensive proteomic survey of human CSF (Macron et al., 2019; Núńez Galindo et al., 2019), and can be done on iCSF as well to further investigate the iCSF proteome of patients with rare neurological diseases. Proteomic data from iCSF samples can be compared to existing spectral libraries of human CSF (hCSF) proteomes from patients (Schilde et al., 2020), to confirm that iCSF is a valid alternative to hCSF for biomarker research and discovery. As shown in Figure 3, in addition to proteomic analysis, metabolomic (Yan et al., 2021) and lipidomic (Byeon et al., 2021) analyses can be performed on this fluid to provide a comprehensive characterization of the composition of patient-derived CSF, with the aim of detecting unique disease biomarker signatures compared to CSF from healthy patients. The signatures found using this in vitro model can also be integrated into generative computer modeling platforms to inform clinicians of patient disease progression, creating a translational bridge by turning these observations into informed patient interventions.
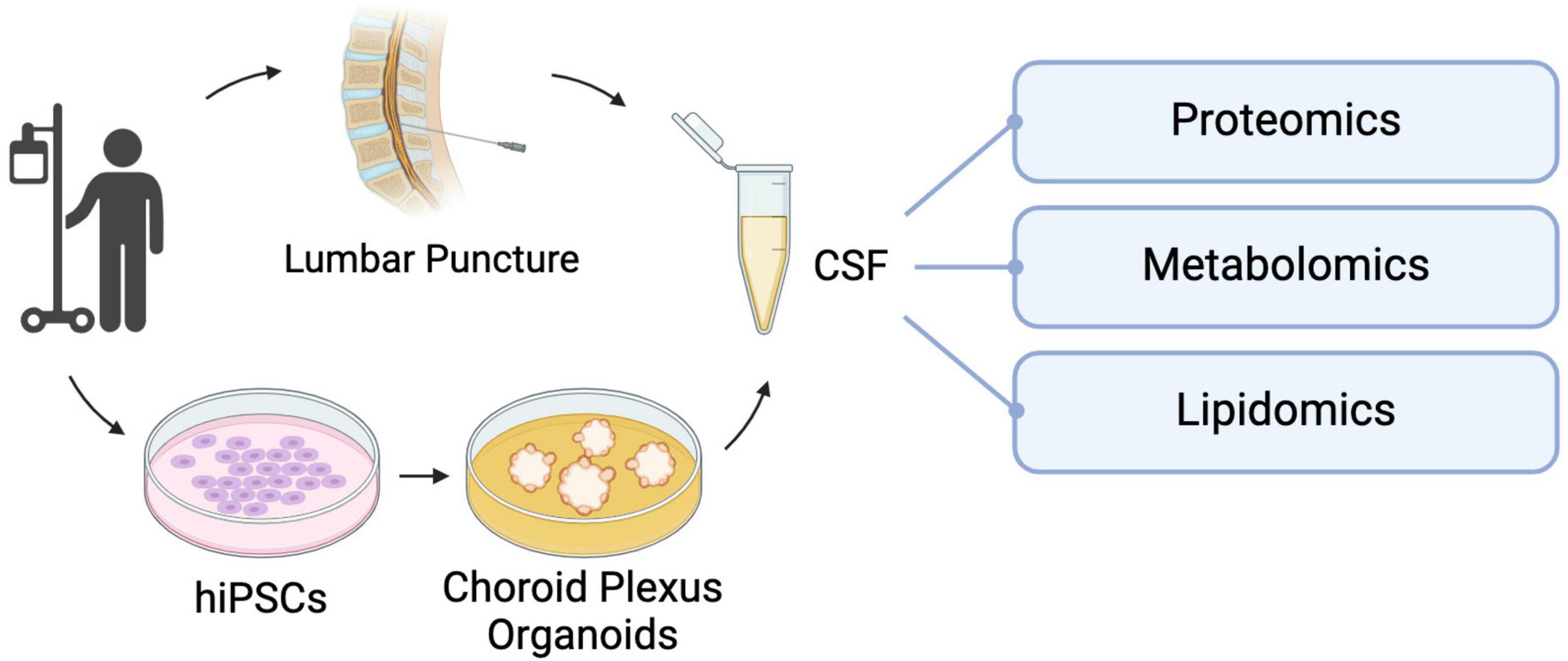
Figure 3. hiPSC-derived choroid plexus organoids present an alternative method of collecting CSF for biomarker analysis without the need for invasive lumbar punctures. This in vitro CSF-like fluid can be used for targeted investigation of factors of interest or unbiased analyses like proteomic, metabolomic and lipidomic screening to uncover unique molecular signatures that can be used to make differential diagnoses and track disease progression and prognosis.
However, while hiPSC-derived neural organoid models provide a promising platform to study the molecular and cellular mechanisms underlying neurological diseases in human cells, they have their limitations. These models are isolated systems that generally do not give rise to cell types of non-neural lineages due to the promotion of neural induction during differentiation, have limited maturation of their existing cell types and possess an atypical physiology that cannot fully recapitulate the complex environment of the human brain (Andrews and Kriegstein, 2022). To address this limitation, researchers have been co-culturing 2D and 3D neural tissues with non-neural cell types (Figure 2A). Neural organoids also lack vascularization, limiting the nutrient and metabolite exchange from the innermost regions of these structures, resulting in cell death in those regions (Lancaster and Knoblich, 2014). In the case of ChP organoids, lack of vascularization also means that this system cannot reproduce one of the key functions of the ChP in vivo, the formation of the blood-cerebrospinal fluid barrier (B-CSF) (Solár et al., 2020). There are groups who have vascularized organoids (Solár et al., 2020), and while these approaches create space for passive media flow, it still doesn’t fully replicate blood flow. Despite these limitations, the ChP organoid model has the potential to provide a platform to allow further work on the identification and validation of biomarkers for rare neurodegenerative and neurodevelopmental diseases, with the aim of contributing to accurate and differential diagnoses and improved patient outcomes.
6 High-throughput approaches to disease-specific drug discovery
Clinical drug trials notoriously report poor outcomes, in part because drug discovery, development, and pre-clinical research have typically relied on non-mammalian model systems that lack important genetic and physiological features that are unique to the human brain. An important part of the challenge is that human primary cells are challenging to procure and propagate in vitro. With the emergence of hiPSCs and the ongoing collective efforts of the scientific community, directed differentiation methods can produce specific cell types which is enabling targeted pharmacological research into different neurodevelopmental and neurodegenerative diseases in which specific cell types are affected (i.e., dopaminergic neurons in PD, GABAergic neurons in Huntington’s disease) (HD iPSC Consortium, 2012; Doi et al., 2020). Also possible is the investigation of interactions between cell types [i.e., microglia and motor neurons in amyotrophic lateral sclerosis (ALS)] (Sances et al., 2016; Vahsen et al., 2022). Combined with CRISPR-Cas9 technology, the use of hiPSCs has revolutionized drug discovery by allowing the generation of in vitro models with much improved relevance to human brain development and cell type fidelity, and by providing a route to gain deeper understanding of the molecular mechanisms that drive human genetic diseases (Takahashi et al., 2007). In this regard, the ability to capture subcellular events in response to pharmacological interventions has been a boon to drug discovery.
High-throughput screening (HTS) is a technique to rapidly assay a range of variables in an automated way that inform cellular phenotypes in relation to genetic mutations or environmental conditions, and is particularly useful as a drug discovery tool. This application was driven by a growing need to find more effective and highly specific drug candidates in a diverse chemical space. In a typical HTS screen, small molecules are tested in parallel against biological targets (e.g., cells or simple tissues) to identify “hit” compounds that can restore normal phenotypes. HTS has been enabled by a confluence of several scientific and technological advances including: (1) the development of large, diverse, and individually characterized compound libraries; (2) improvements to cell-based assays that are cost-effective, involve straightforward protocols, and can probe intra- and inter-cellular events; (3) miniaturization of assays to reduce the cost-prohibitive nature of large screens; (4) advances in engineering of automation robotics to reduce repetitive manual tasks; and (5) development of bioinformatic tools for large dataset management and analysis (Broach and Thorner, 1996; Sittampalam et al., 1997; Fernandes, 1998; Silverman et al., 1998). Each of these fields remains highly active, and ongoing advancements continue to improve HTS and drive its widespread application. Here we will highlight selected areas that could advance drug discovery by harnessing the combined strength of hiPSCs and HTS.
One major advantage of hiPSCs is the continuous and unlimited generation of cells and tissues of interest. However, given the sheer volume of cells required for large screens, which can include 100,000 compounds or more, the manual labor required to culture hiPSCs and downstream cell types is not feasible for most laboratories. Though recently, innovations in automative engineering are addressing this issue by developing automated cell culture systems that entail strictly hands-off and scalable workflows. Liquid handlers and robotic arms can now perform tasks related to tissue culture vessel handling, media exchanges, subculturing, and differentiation protocols (Terstegge et al., 2007; Thomas et al., 2009; Valamehr et al., 2012; Conway et al., 2015; Tristan et al., 2021; Deng et al., 2023). Well-optimized automation can be reliably used to implement standard operating procedures, efficiently manage cell quality control, and reduce random batch-to-batch variability associated with human error. This is important because drug screens are costly assays, and as such it is common practice to have few or no technical or biological replicates. Thus, consistency of cellular and experimental parameters is a key concern as the few (or single) replicate measurements must accurately represent the phenotype(s) of interest.
Due to individual genetic diversity, effective precision medicine platforms will require patient-specific cell lines to appropriately classify both disease-specific cohorts and heterogeneity among patients with a given condition. Thus, scaling up automated cell culture technologies is critical to make this a reality. Efforts have been made to develop automated pipelines for the creation of hiPSC lines from fibroblasts (Paull et al., 2015). In the context of drug discovery, this will improve healthcare efficacy as top drug candidates can be identified before they are administered, thereby minimizing the deleterious side effects of medication optimization.
Despite the current advancements in hiPSC disease modeling, a unique challenge in neuroactive drug discovery using in vitro models is the absence of a BBB. The BBB is a layer of endothelial, mural, astrocytic, and immune cells surrounded by a basement membrane. It has a critical role in tightly regulating the transfer of substances between the circulatory system and the central nervous system, and serves to prevent exposure of the brain to harmful substances (Daneman and Prat, 2015). However, because the permeability of the BBB is very selective, most drug candidates are not effectively transported to the brain and only drugs below 400 Da are able to cross via lipid-mediated diffusion (Pardridge, 2012). Strategies have been developed that enhance the ability of drugs to penetrate the BBB, including increasing drug lipid solubility, use of carrier-mediated transport, or concurrent use of another drug that disrupts BBB permeability (Mikitsh and Chacko, 2014). Thus, consideration and inclusion of models to investigate permeability through the BBB is a critical aspect of in vitro drug discovery that needs to be increasingly considered when making predictions for drug efficacy.
Co-culture models that combine distinct hiPSC-derived cell types to replicate key aspects of the BBB exhibit physiologically appropriate characteristics including tube formation, organized tight junctions with acceptable transendothelial electrical resistance (TEER), expression of active transporters, and uptake of low-density lipoprotein (Lippmann et al., 2012; Aday et al., 2016; Qian et al., 2017). This stem-cell derived model, either as a standalone organoid or combined with organ-on-a-chip technology, shows great promise in enhancing drug discovery research by simulating a more physiologically accurate representation of drug delivery (Nzou et al., 2018; Choi et al., 2024). However, the low-volume nature of producing these models presents a challenge in translatability to HTS. Preliminary efforts have started scale up of the system through innovations in multi-chamber chip fabrication, which allows for parallel drug testing, but this technology has yet to be explored with HTS (Fan et al., 2016; Wevers et al., 2018).
Leveraging hiPSC technologies combined with HTS workflows is a cornerstone of translational medicine because it provides a powerful tool to elucidate disease pathways and identify potential pharmacological targets. However, limitations still exist. As described above, the benefits of organoid and assembloid modeling are evident, but incorporating these advances into a HTS workflow remains a barrier due to scalability limitations of 3D tissue models. Additionally, 3D models are typically heterogeneous in composition and size, which introduces high levels of error and thus are intrinsically challenging to characterize with currently available tools (Carragher et al., 2018; Booij et al., 2019). Additionally, compound hit identification relies primarily on a still limited range of fluorescent protein markers. This means that these assays have limited potential for multiplexity due to overlap in excitation and emission wavelengths. Thus, there is an ongoing demand for standardization of 3D tissue models (to produce tissues of reproducible size and architecture) and improved imaging probes, particularly for quantification of functional disease phenotypes and the short- and long-term intra- and inter-cellular responses with drug treatment.
Increasingly advanced hiPSC models have provided a greater understanding of disease pathogenesis, but to improve pharmacological interventions there exists a need for paradigm shifts in strategies for drug discovery. Historically, drug discoveries depended on observations of phenotypic changes of a single known target. To prevent off-target effects, pharmaceutical research aims to develop compounds with low promiscuity. However, this strategy has poor efficacy for complex diseases such as schizophrenia, multiple sclerosis (MS), and cancer. The problem arises because those diseases are polygenic or involve more complex interactions between different cell types or multiple molecular pathways. More recently, research has revealed the benefits of highly specific multi-target compounds in treating such multifactorial diseases, likely due to synergistic effects through secondary off-targets (Peters, 2013; Anighoro et al., 2014; Makhoba et al., 2020). Now coined as polypharmacology, this new approach to drug discovery aims to elucidate new applications of existing and theoretical compounds (Anighoro et al., 2014).
The forefront of drug discovery is moving toward incorporation of deep learning and artificial intelligence (AI) to aid in identifying drug candidates in silico. AI-assisted tools are continuously improving predictions and characterizations of cell protein folding and structure, identifying druggable proteomes, and simulating protein-ligand binding behavior (Cichońska et al., 2024). Combined with ultra-large virtual libraries, some with over 116 billion molecules that cover all possible structures within the chemical space, the combination of these powerful resources can generate an unprecedented amount of data for a targeted approach to polypharmacology (Ruddigkeit et al., 2012; Reymond, 2015). Well-characterized mechanisms of action can be invaluable for clinicians making critical treatment decisions and can greatly inform drug design and synthesis. Integration of AI-informed data with hiPSC platforms presents an exciting new frontier of disease modeling and pharmaceutical interventions. In parallel, the advance of high-throughput screening methods, empowered by the scalability of hiPSC-derived systems, holds the promise of expediting identification and validation of novel therapeutic compounds. Thus, with the right applications, hiPSCs are positioned to be particularly powerful clinical tools with outstanding patient-specific precision.
7 Integrating hiPSC-derived cellular data into AI-driven patient biophysical models
Early applications of AI toward hiPSC-derived models centered on image analyses to classify cells and hiPSC colonies based on morphological features, allowing for accurate classifications without human bias and enhancing precision and scalability by automating manual assessments (Vo et al., 2024). This laid the groundwork for the use of machine learning to automate the analysis of more complex data sets, such as RNA sequencing data, morphological and molecular assessment of disease-specific cell types, and cell function monitoring. In the context of disease-modeling, AI algorithms can become trained on large data sets to report alterations in cell morphology (Teles et al., 2021), fluorescent readouts of cell state and function (Nishino et al., 2021) to understand and identify differences in cell differentiation in hiPSCs, ultimately allowing it to predict differentiation outcomes. These predictions can be used to facilitate the high-throughput screening of drug candidates by analyzing cellular responses to treatments, providing insights to drug efficacy and toxicity (Kusumoto et al., 2022). Label-free drug screening systems can be especially useful in cases where no effective molecular markers for a given phenotype are known.
We envision that these achievements in AI around hiPSC-derived brain models can be further expanded to permit integration of meaningful cellular data with other types of patient-derived information that represent brain function or dysfunction on a larger scale. For example, rapid advancements in deep learning and generative AI have also led to the establishment of synthetic replicas of biophysical entities based on patient-derived neuroimaging data, coined “digital twins” (DT). In its most general sense, DT technology allows for the integration of large volumes of data to simulate a physical system in a digital space and to make predictive models. This concept has been harnessed for a myriad of applications spanning spacecraft navigation simulation (Grieves and Vickers, 2017) to urban planning (Bolton et al., 2018). In the context of biomedical research and precision medicine, there are promising new efforts that leverage the DT technology to integrate subject-derived data to form brain simulations, ultimately with the goal of using this predictive model to inform decision-making in patient diagnosis, prognosis, and therapeutics. For example, consolidation of longitudinal magnetic resonance imaging (MRI) to produce reference data on normal aging can be used to create an algorithm to identify premature development of brain atrophy and predict and monitor disease progression of MS (Voigt et al., 2021; Cen et al., 2023). Electroencephalography (EEG) theta and delta activity can be used as biomarker candidates to classify stroke patients to guide prevention and post-stroke care (Hussain and Park, 2021).
Previous work on a platform built on generative biophysical modeling, The Virtual Brain (TVB), has accurately generated high-fidelity patient brain models that can map trajectories for normative aging (Lavanga et al., 2023) and neurological conditions like neurodegeneration (Zimmermann et al., 2018) idiopathic epilepsy (Jirsa et al., 2014), stroke (Falcon et al., 2016) and cancer (Aerts et al., 2018). TVB has also shown that the excitatory/inhibitory (E/I) balance in different neuronal populations can provide a strong link between cellular and neuroimaging models (Deco et al., 2014; Proix et al., 2017; Schirner et al., 2018; Figure 1). This suggests that electrophysiological modeling can be used as a parameter to determine differences between patient and control iPSC-derived neural cultures. In the context of epilepsy disorders, changes in E/I balance can be used to determine the efficacy of drugs being tested on iPSC-derived neural tissue for treatment of the disease. As most patients have subtle differences in their disease-causing mutations, testing drugs on cultures derived from their own hiPSCs provides the most direct, translatable link if a drug candidate is identified. In further applications, the electrophysiology data collected from the aforementioned multi-region assembloid models allows for a detailed assessment of neural cell and circuit interactions between different brain regions, particularly as inhibitory interneurons integrate within excitatory neuron networks.
The integration of electrophysiological data from hiPSC-derived models into these AI-modeling platforms has the clinical potential to predict how cellular processes and their manipulation can impact the whole brain, providing estimates of brain function across multiple scales–individual cells, and single or interconnected neural networks–on an individualized basis. This integration can allow for the development of a fully personalized platform for rare diseases that can span population-level and patient-specific mechanisms, informing clinical strategies and cross-validated biomarkers. This approach can allow the rapid development of disease-specific diagnostics and individualized treatment plans for patients with rare neurological disorders.
In summary, as hiPSC-derived neural disease models evolve to more accurately reflect the complexity of human neurobiology in vitro, their potential to uncover disease mechanisms, identify biomarkers and therapeutic targets will only grow. The integration of hiPSC-derived neural models with advanced tools such as genome engineering, high-throughput screening and AI-modeling platforms offers a promising pathway toward the realization of precision medicine for brain disorders. Together, these innovations hold the promise of transforming the management of complex and rare neurological disorders to hopefully offer more effective and tailored therapeutic approaches.
Author contributions
NF: Conceptualization, Writing – original draft, Writing – review and editing. LL: Writing – original draft, Writing – review and editing, Conceptualization. SN: Writing – original draft, Writing – review and editing, Conceptualization. AN: Writing – original draft, Conceptualization. LR: Writing – original draft. AM: Conceptualization, Writing – original draft, Writing – review and editing. LJ: Conceptualization, Funding acquisition, Writing – original draft, Writing – review and editing.
Funding
The author(s) declare that financial support was received for the research, authorship, and/or publication of this article. LJ is a Tier II Canada Research Chair and a Michael Smith Foundation for Health Research/Parkinson Society BC Scholar. LL is funded by MITACS and the NSERC Alliance program, SN by SFU and Phyllis Carter Burr Graduate Fellowships, and AN by the CIHR Canada Graduate Scholarship-M program. We also acknowledge relevant funding support from Ataxia Canada, Epilepsy Canada, the Cancer Research Society (JULIAN, L-CRS 25551), the Natural Sciences and Engineering Research Council of Canada (JULIAN, L-RGPIN-03965), and AM is funded by the NSERC Discovery Grant RGPIN-2018-04457 and Canadian Institutes of Health Research (CIHR) Project Grant PJT-168980.
Conflict of interest
The authors declare that the research was conducted in the absence of any commercial or financial relationships that could be construed as a potential conflict of interest.
Publisher’s note
All claims expressed in this article are solely those of the authors and do not necessarily represent those of their affiliated organizations, or those of the publisher, the editors and the reviewers. Any product that may be evaluated in this article, or claim that may be made by its manufacturer, is not guaranteed or endorsed by the publisher.
References
Abud, E. M., Ramirez, R. N., Martinez, E. S., Healy, L. M., Nguyen, C. H. H., Newman, S. A., et al. (2017). iPSC-derived human microglia-like cells to study neurological diseases. Neuron 94, 278–293.e9. doi: 10.1016/j.neuron.2017.03.042
Aday, S., Cecchelli, R., Hallier-Vanuxeem, D., Dehouck, M. P., and Ferreira, L. (2016). Stem cell-based human blood–brain barrier models for drug discovery and delivery. Trends Biotechnol. 34:382. doi: 10.1016/j.tibtech.2016.01.001
Adlakha, Y. K. (2023). Human 3D brain organoids: steering the demolecularization of brain and neurological diseases. Cell Death Discov. 9:221. doi: 10.1038/s41420-023-01523-w
Aerts, H., Schirner, M., Jeurissen, B., Van Roost, D., Achten, E., Ritter, P., et al. (2018). Modeling brain dynamics in brain tumor patients using the virtual brain. eNeuro 5, ENEURO.0083-18.2018. doi: 10.1523/ENEURO.0083-18.2018
Agarwal, S., Holton, K. L., and Lanza, R. (2008). Efficient differentiation of functional hepatocytes from human embryonic stem cells. Stem Cells 26, 1117–1127. doi: 10.1634/stemcells.2007-1102
Aktories, P., Petry, P., and Kierdorf, K. (2022). Microglia in a dish-which techniques are on the menu for functional studies? Front. Cell Neurosci. 16:908315. doi: 10.3389/fncel.2022.908315
Allen, N. J., and Lyons, D. A. (2018). Glia as architects of central nervous system formation and function. Science 362, 181–185. doi: 10.1126/science.aat0473
Amadio, S., De Ninno, A., Montilli, C., Businaro, L., Gerardino, A., Volonté, C., et al. (2013). Plasticity of primary microglia on micropatterned geometries and spontaneous long-distance migration in microfluidic channels. BMC Neurosci. 14:121. doi: 10.1186/1471-2202-14-121
Amirifar, L., Shamloo, A., Nasiri, R., de Barros, N. R., Wang, Z. Z., Unluturk, B. D., et al. (2022). Brain-on-a-chip: recent advances in design and techniques for microfluidic models of the brain in health and disease. Biomaterials 285:121531. doi: 10.1016/j.biomaterials.2022.121531
An, M. C., Zhang, N., Scott, G., Montoro, D., Wittkop, T., Mooney, S., et al. (2012). Genetic correction of Huntington’s disease phenotypes in induced pluripotent stem cells. Cell Stem Cell 11, 253–263. doi: 10.1016/j.stem.2012.04.026
Andersen, J., Revah, O., Miura, Y., Thom, N., Amin, N. D., Kelley, K. W., et al. (2020). Generation of functional human 3D cortico-motor assembloids. Cell 183, 1913–1929.e26. doi: 10.1016/j.cell.2020.11.017
Anderson, W. D., and Vadigepalli, R. (2016). Modeling cytokine regulatory network dynamics driving neuroinflammation in central nervous system disorders. Drug Discov. Today Dis. Models 19, 59–67. doi: 10.1016/j.ddmod.2017.01.003
Andrews, M. G., and Kriegstein, A. R. (2022). Challenges of organoid research. Annu. Rev. Neurosci. 45, 23–39. doi: 10.1146/annurev-neuro-111020-090812
Angulo Salavarria, M. M., Dell’Amico, C., D’Agostino, A., Conti, L., and Onorati, M. (2023). Cortico-thalamic development and disease: from cells, to circuits, to schizophrenia. Front. Neuroanat. 17:1130797. doi: 10.3389/fnana.2023.1130797
Anighoro, A., Bajorath, J., and Rastelli, G. (2014). Polypharmacology: challenges and opportunities in drug discovery. J. Med. Chem. 57, 7874–7887. doi: 10.1021/jm5006463
Ao, Z., Cai, H., Wu, Z., Song, S., Karahan, H., Kim, B., et al. (2021). Tubular human brain organoids to model microglia-mediated neuroinflammation. Lab. Chip 21, 2751–2762. doi: 10.1039/d1lc00030f
Atamian, A., Birtele, M., Hosseini, N., Nguyen, T., Seth, A., Del Dosso, A., et al. (2024). Human cerebellar organoids with functional Purkinje cells. Cell Stem Cell 31, 39–51.e6. doi: 10.1016/j.stem.2023.11.013
Avansini, S. H., Puppo, F., Adams, J. W., Vieira, A. S., Coan, A. C., Rogerio, F., et al. (2022). Junctional instability in neuroepithelium and network hyperexcitability in a focal cortical dysplasia human model. Brain 145, 1962–1977. doi: 10.1093/brain/awab479
Bagley, J. A., Reumann, D., Bian, S., Lévi-Strauss, J., and Knoblich, J. A. (2017). Fused cerebral organoids model interactions between brain regions. Nat. Methods 14, 743–751. doi: 10.1038/nmeth.4304
Bame, M., McInnis, M. G., and O’Shea, K. S. (2020). MicroRNA alterations in induced pluripotent stem cell-derived neurons from bipolar disorder patients: pathways involved in neuronal differentiation, axon guidance, and plasticity. Stem Cells Dev. 29, 1145–1159. doi: 10.1089/scd.2020.0046
Barak, M., Fedorova, V., Pospisilova, V., Raska, J., Vochyanova, S., Sedmik, J., et al. (2022). Human iPSC-derived neural models for studying Alzheimer’s disease: from neural stem cells to cerebral organoids. Stem Cell Rev. Rep. 18, 792–820. doi: 10.1007/s12015-021-10254-3
Bardy, C., van den Hurk, M., Kakaradov, B., Erwin, J. A., Jaeger, B. N., Hernandez, R. V., et al. (2016). Predicting the functional states of human iPSC-derived neurons with single-cell RNA-seq and electrophysiology. Mol. Psychiatry 21, 1573–1588. doi: 10.1038/mp.2016.158
Bartalska, K., Hübschmann, V., Korkut-Demirbaş, M., Cubero, R. J. A., Venturino, A., Rössler, K., et al. (2022). A systematic characterization of microglia-like cell occurrence during retinal organoid differentiation. iScience 25:104580. doi: 10.1016/j.isci.2022.104580
Bavassano, C., Eigentler, A., Stanika, R., Obermair, G. J., Boesch, S., Dechant, G., et al. (2017). Bicistronic CACNA1A gene expression in neurons derived from spinocerebellar ataxia type 6 patient-induced pluripotent stem cells. Stem Cells Dev. 26, 1612–1625. doi: 10.1089/scd.2017.0085
Beghi, E. (2020). The epidemiology of epilepsy. Neuroepidemiology 54, 185–191. doi: 10.1159/000503831
Bellen, H. J., Tong, C., and Tsuda, H. (2010). 100 years of Drosophila research and its impact on vertebrate neuroscience: a history lesson for the future. Nat. Rev. Neurosci. 11, 514–522. doi: 10.1038/nrn2839
Birey, F., Andersen, J., Makinson, C. D., Islam, S., Wei, W., Huber, N., et al. (2017). Assembly of functionally integrated human forebrain spheroids. Nature 545, 54–59. doi: 10.1038/nature22330
Blair, J. D., Hockemeyer, D., and Bateup, H. S. (2018). Genetically engineered human cortical spheroid models of tuberous sclerosis. Nat. Med. 24, 1568–1578. doi: 10.1038/s41591-018-0139-y
Bohlen, C. J., Friedman, B. A., Dejanovic, B., and Sheng, M. (2019). Microglia in brain development, homeostasis, and neurodegeneration. Annu. Rev. Genet. 53, 263–288. doi: 10.1146/annurev-genet-112618-043515
Bolton, A., Butler, L., Dabson, I., Enzer, M., Evans, M., Fenemore, T., et al. (2018). Gemini principles. CDBB. doi: 10.17863/CAM.32260
Booij, T. H., Price, L. S., and Danen, E. H. J. (2019). 3D Cell-based assays for drug screens: challenges in imaging, image analysis, and high-content analysis. SLAS Discov. 24:615. doi: 10.1177/2472555219830087
Bouwman, F. H., Frisoni, G. B., Johnson, S. C., Chen, X., Engelborghs, S., Ikeuchi, T., et al. (2022). Clinical application of CSF biomarkers for Alzheimer’s disease: from rationale to ratios. Alzheimers Dement. (Amst) 14, e12314. doi: 10.1002/dad2.12314
Byeon, S. K., Madugundu, A. K., Jain, A. P., Bhat, F. A., Jung, J. H., Renuse, S., et al. (2021). Cerebrospinal fluid lipidomics for biomarkers of Alzheimer’s disease. Mol. Omics 17, 454–463. doi: 10.1039/d0mo00186d
Caldwell, C. C., Yao, J., and Brinton, R. D. (2015). Targeting the prodromal stage of Alzheimer’s disease: bioenergetic and mitochondrial opportunities. Neurotherapeutics 12, 66–80. doi: 10.1007/s13311-014-0324-8
Carragher, N., Piccinini, F., Tesei, A., Trask, O. J., Bickle, M., Horvath, P., et al. (2018). Concerns, challenges and promises of high-content analysis of 3D cellular models. Nat. Rev. Drug Discov. 17:606. doi: 10.1038/nrd.2018.99
Cen, S., Gebregziabher, M., Moazami, S., Azevedo, C., and Pelletier, D. (2023). Toward precision medicine using a “Digital Twin” approach: modeling the onset of disease-specific brain atrophy in individuals with multiple sclerosis. Res Sq 13:16279. doi: 10.21203/rs.3.rs-2833532/v1
Centeno, E. G. Z., Cimarosti, H., and Bithell, A. (2018). 2D versus 3D human induced pluripotent stem cell-derived cultures for neurodegenerative disease modelling. Mol. Neurodegener. 13:27. doi: 10.1186/s13024-018-0258-4
Chen, W., Liu, J., Zhang, L., Xu, H., Guo, X., Deng, S., et al. (2014). Generation of the SCN1A epilepsy mutation in hiPS cells using the TALEN technique. Sci. Rep. 4:5404. doi: 10.1038/srep05404
Chen, X. H., Huang, S., and Kerr, D. (2011). Biomarkers in clinical medicine. IARC Sci. Publ. 163, 303–322.
Cheung, A. Y., Horvath, L. M., Grafodatskaya, D., Pasceri, P., Weksberg, R., Hotta, A., et al. (2011). Isolation of MECP2-null Rett Syndrome patient hiPS cells and isogenic controls through X-chromosome inactivation. Hum. Mol. Genet. 20, 2103–2115. doi: 10.1093/hmg/ddr093
Chirino-Pérez, A., Vaca-Palomares, I., Torres, D. L., Hernandez-Castillo, C. R., Diaz, R., Ramirez-Garcia, G., et al. (2021). Cognitive impairments in spinocerebellar ataxia type 10 and their relation to cortical thickness. Mov. Disord. 36, 2910–2921. doi: 10.1002/mds.28728
Choi, G., Yang, H.-Y., Cho, S., Kwon, D., Kim, D.-W., Ko, S., et al. (2024). Brain-On-A-chip based on human pluripotent stem cell-derived neurons and astrocytes for neurotoxicity testing: Communicative Astrocyte–Neuron DYnamics (CANDY) Chip. Adv. Mater. Technol. 9:2400107. doi: 10.1002/admt.202400107
Chun, Y. S., Byun, K., and Lee, B. (2011). Induced pluripotent stem cells and personalized medicine: current progress and future perspectives. Anat. Cell Biol. 44, 245–255. doi: 10.5115/acb.2011.44.4.245
Cichońska, A., Ravikumar, B., and Rahman, R. (2024). AI for targeted polypharmacology: the next frontier in drug discovery. Curr. Opin. Struct. Biol. 84:102771. doi: 10.1016/j.sbi.2023.102771
Ciolli, L., Krismer, F., Nicoletti, F., and Wenning, G. K. (2014). An update on the cerebellar subtype of multiple system atrophy. Cerebellum Ataxias 1:14. doi: 10.1186/s40673-014-0014-7
Cluskey, S., and Ramsden, D. B. (2001). Mechanisms of neurodegeneration in amyotrophic lateral sclerosis. Mol. Pathol. 54, 386–392.
Colonna, M., and Butovsky, O. (2017). Microglia function in the central nervous system during health and neurodegeneration. Annu. Rev. Immunol. 35, 441–468. doi: 10.1146/annurev-immunol-051116-052358
Conway, M. K., Gerger, M. J., Balay, E. E., O’Connell, R., Hanson, S., Daily, N. J., et al. (2015). Scalable 96-well plate based iPSC culture and production using a robotic liquid handling system. J. Vis. Exp. 99:e52755. doi: 10.3791/52755
Costa, V., Aigner, S., Vukcevic, M., Sauter, E., Behr, K., Ebeling, M., et al. (2016). mTORC1 inhibition corrects neurodevelopmental and synaptic alterations in a human stem cell model of tuberous sclerosis. Cell Rep. 15, 86–95. doi: 10.1016/j.celrep.2016.02.090
Crino, P. B. (2020). mTORopathies: a road well-traveled. Epilepsy Curr. 20, 64S–66S. doi: 10.1177/1535759720959320
Cuyàs, E., Corominas-Faja, B., Joven, J., and Menendez, J. A. (2014). Cell cycle regulation by the nutrient-sensing mammalian target of rapamycin (mTOR) pathway. Methods Mol. Biol. 1170, 113–144. doi: 10.1007/978-1-4939-0888-2_7
Daneman, R., and Prat, A. (2015). The blood–brain barrier. Cold Spring Harb. Perspect. Biol. 7:a020412. doi: 10.1101/cshperspect.a020412
Das, A., Kim, S. H., Arifuzzaman, S., Yoon, T., Chai, J. C., Lee, Y. S., et al. (2016). Transcriptome sequencing reveals that LPS-triggered transcriptional responses in established microglia BV2 cell lines are poorly representative of primary microglia. J. Neuroinflamm. 13:182. doi: 10.1186/s12974-016-0644-1
Dawson, T. M., Golde, T. E., and Lagier-Tourenne, C. (2018). Animal models of neurodegenerative diseases. Nat. Neurosci. 21, 1370–1379. doi: 10.1038/s41593-018-0236-8
Deco, G., Ponce-Alvarez, A., Hagmann, P., Romani, G. L., Mantini, D., Corbetta, M., et al. (2014). How local excitation-inhibition ratio impacts the whole brain dynamics. J. Neurosci. 34, 7886–7898. doi: 10.1523/JNEUROSCI.5068-13.2014
Deisseroth, K., Feng, G., Majewska, A. K., Miesenböck, G., Ting, A., Schnitzer, M. J., et al. (2006). Next-generation optical technologies for illuminating genetically targeted brain circuits. J. Neurosci. 26, 10380–10386. doi: 10.1523/JNEUROSCI.3863-06.2006
Delaney, S. P., Julian, L. M., and Stanford, W. L. (2014). The neural crest lineage as a driver of disease heterogeneity in tuberous sclerosis complex and lymphangioleiomyomatosis. Front. Cell Dev. Biol. 2:69. doi: 10.3389/fcell.2014.00069
Delaney, S. P., Julian, L. M., Pietrobon, A., Yockell-Lelièvre, J., Doré, C., Wang, T. T., et al. (2020). Stem cell models identify lineage-specific catabolic signaling, neoplastic mechanisms and therapeutic vulnerabilities in tuberous sclerosis Running title: lineage-specific catabolic signaling in Tuberous Sclerosis. bioRxiv [Preprint] doi: 10.1101/683359
Dello Russo, C., Cappoli, N., Coletta, I., Mezzogori, D., Paciello, F., Pozzoli, G., et al. (2018). The human microglial HMC3 cell line: where do we stand? A systematic literature review. J. Neuroinflamm. 15:259. doi: 10.1186/s12974-018-1288-0
Deng, T., Jovanovic, V. M., Tristan, C. A., Weber, C., Chu, P. H., Inman, J., et al. (2023). Scalable generation of sensory neurons from human pluripotent stem cells. Stem Cell Rep. 18:1030. doi: 10.1016/j.stemcr.2023.03.006
Dhaliwal, N. K., Weng, O. Y., Dong, X., Bhattacharya, A., Ahmed, M., Nishimura, H., et al. (2024). Synergistic hyperactivation of both mTORC1 and mTORC2 underlies the neural abnormalities of PTEN-deficient human neurons and cortical organoids. Cell Rep. 43:114173. doi: 10.1016/j.celrep.2024.114173
Doi, D., Magotani, H., Kikuchi, T., Ikeda, M., Hiramatsu, S., Yoshida, K., et al. (2020). Pre-clinical study of induced pluripotent stem cell-derived dopaminergic progenitor cells for Parkinson’s disease. Nat. Commun. 11:3369. doi: 10.1038/s41467-020-17165-w
du Chatinier, A., Velilla, I. Q., Meel, M. H., Hoving, E. W., Hulleman, E., Metselaar, D. S., et al. (2023). Microglia in pediatric brain tumors: the missing link to successful immunotherapy. Cell Rep. Med. 4:101246. doi: 10.1016/j.xcrm.2023.101246
Eichmuller, O. L., Corsini, N. S., Vértesy, Á, Morassut, I., Scholl, T., Gruber, V. E., et al. (2022). Amplification of human interneuron progenitors promotes brain tumors and neurological defects. Science 375:eabf5546. doi: 10.1126/science.abf5546
Estévez-Priego, E., Moreno-Fina, M., Monni, E., Kokaia, Z., Soriano, J., Tornero, D., et al. (2023). Long-term calcium imaging reveals functional development in hiPSC-derived cultures comparable to human but not rat primary cultures. Stem Cell Rep. 18, 205–219. doi: 10.1016/j.stemcr.2022.11.014
Eura, N., Matsui, T. K., Luginbühl, J., Matsubayashi, M., Nanaura, H., Shiota, T., et al. (2020). Brainstem organoids from human pluripotent stem cells. Front. Neurosci. 14:538. doi: 10.3389/fnins.2020.00538
Fair, S. R., Julian, D., Hartlaub, A. M., Pusuluri, S. T., Malik, G., Summerfied, T. L., et al. (2020). Electrophysiological maturation of cerebral organoids correlates with dynamic morphological and cellular development. Stem Cell Rep. 15, 855–868. doi: 10.1016/j.stemcr.2020.08.017
Falcon, M. I., Riley, J. D., Jirsa, V., McIntosh, A. R., Chen, E. E., Solodkin, A., et al. (2016). Functional mechanisms of recovery after chronic stroke: modeling with the virtual brain. eNeuro 3:ENEURO.158–ENEURO.115. doi: 10.1523/ENEURO.0158-15.2016
Fan, Y., Nguyen, D. T., Akay, Y., Xu, F., and Akay, M. (2016). Engineering a brain cancer chip for high-throughput drug screening. Sci. Rep. 6:25062. doi: 10.1038/srep25062
Farkhondeh, A., Li, R., Gorshkov, K., Chen, K. G., Might, M., Rodems, S., et al. (2019). Induced pluripotent stem cells for neural drug discovery. Drug Discov. Today 24, 992–999. doi: 10.1016/j.drudis.2019.01.007
Feng, G., Jensen, F. E., Greely, H. T., Okano, H., Treue, S., Roberts, A. C., et al. (2020). Opportunities and limitations of genetically modified nonhuman primate models for neuroscience research. Proc. Natl. Acad. Sci. U.S.A. 117, 24022–24031. doi: 10.1073/pnas.2006515117
Fernandes, P. B. (1998). Technological advances in high-throughput screening. Curr. Opin. Chem. Biol. 2:597. doi: 10.1016/s1367-5931(98)80089-6
Fessel, J. (2023). Cure of Alzheimer’s dementia requires addressing all of the affected brain cell types. J. Clin. Med. 12:2049. doi: 10.3390/jcm12052049
Fligor, C. M., Lavekar, S. S., Harkin, J., Shields, P. K., VanderWall, K. B., Huang, K. C., et al. (2021). Extension of retinofugal projections in an assembled model of human pluripotent stem cell-derived organoids. Stem Cell Rep. 16, 2228–2241. doi: 10.1016/j.stemcr.2021.05.009
Forman, M. S., Trojanowski, J. Q., and Lee, V. M. (2004). Neurodegenerative diseases: a decade of discoveries paves the way for therapeutic breakthroughs. Nat. Med. 10, 1055–1063. doi: 10.1038/nm1113
Frost, M., and Hulbert, J. (2015). Clinical management of tuberous sclerosis complex over the lifetime of a patient. Pediatr. Health Med. Ther. 6, 139–146. doi: 10.2147/PHMT.S67342
Fujimori, K., Ishikawa, M., Otomo, A., Atsuta, N., Nakamura, R., Akiyama, T., et al. (2018). Modeling sporadic ALS in iPSC-derived motor neurons identifies a potential therapeutic agent. Nat. Med. 24, 1579–1589. doi: 10.1038/s41591-018-0140-5
Galiakberova, A. A., and Dashinimaev, E. B. (2020). Neural stem cells and methods for their generation from induced pluripotent stem cells. Front. Cell Dev. Biol. 8:815. doi: 10.3389/fcell.2020.00815
Gamble, P., Jaroensri, R., Wang, H., Tan, F., Moran, M., Brown, T., et al. (2021). Determining breast cancer biomarker status and associated morphological features using deep learning. Commun. Med. (Lond) 1:14. doi: 10.1038/s43856-021-00013-3
Gao, M. L., Zhang, X., Han, F., Xu, J., Yu, S. J., Jin, K., et al. (2022). Functional microglia derived from human pluripotent stem cells empower retinal organ. Sci. China Life Sci. 65, 1057–1071. doi: 10.1007/s11427-021-2086-0
Gellersen, H. M., Guo, C. C., O’Callaghan, C., Tan, R. H., Sami, S., Hornberger, M., et al. (2017). Cerebellar atrophy in neurodegeneration-a meta-analysis. J. Neurol. Neurosurg. Psychiatry 88, 780–788. doi: 10.1136/jnnp-2017-315607
Glover, J. C., Sato, K., and Momose-Sato, Y. (2008). Using voltage-sensitive dye recording to image the functional development of neuronal circuits in vertebrate embryos. Dev. Neurobiol. 68, 804–816. doi: 10.1002/dneu.20629
Glowinski, J., and Iversen, L. L. (1966). Regional studies of catecholamines in the rat brain. I. The disposition of [3H]norepinephrine, [3H]dopamine and [3H]dopa in various regions of the brain. J. Neurochem. 13, 655–669. doi: 10.1111/j.1471-4159.1966.tb09873.x
Grieves, M., and Vickers, J. (2017). “Digital twin: mitigating unpredictable, undesirable emergent behavior in complex systems,” in Transdisciplinary Perspectives on Complex Systems, eds J. Kahlen, S. Flumerfelt, and A. Alves (Cham: Springer).
Gunaseeli, I., Doss, M. X., Antzelevitch, C., Hescheler, J., and Sachinidis, A. (2010). Induced pluripotent stem cells as a model for accelerated patient- and disease-specific drug discovery. Curr. Med. Chem. 17, 759–766. doi: 10.2174/092986710790514480
Guo, C. C., Tan, R., Hodges, J. R., Hu, X., Sami, S., Hornberger, M., et al. (2016). Network-selective vulnerability of the human cerebellum to Alzheimer’s disease and frontotemporal dementia. Brain 139, 1527–1538. doi: 10.1093/brain/aww003
Guo, F., Liu, X., Cai, H., and Le, W. (2018). Autophagy in neurodegenerative diseases: pathogenesis and therapy. Brain Pathol. 28, 3–13. doi: 10.1111/bpa.12545
Guo, J., Jiang, Z., Liu, X., Li, H., Biswal, B. B., Zhou, B., et al. (2023). Cerebello-cerebral resting-state functional connectivity in spinocerebellar ataxia type 3. Hum. Brain Mapp. 44, 927–936. doi: 10.1002/hbm.26113
Habibey, R., Striebel, J., Schmieder, F., Czarske, J., and Busskamp, V. (2022). Long-term morphological and functional dynamics of human stem cell-derived neuronal networks on high-density micro-electrode arrays. Front. Neurosci. 16:951964. doi: 10.3389/fnins.2022.951964
Haldipur, P., Aldinger, K. A., Bernardo, S., Deng, M., Timms, A. E., Overman, L. M., et al. (2019). Spatiotemporal expansion of primary progenitor zones in the developing human cerebellum. Science 366, 454–460. doi: 10.1126/science.aax7526
Hashemi, M., Vattikonda, A. N., Sip, V., Guye, M., Bartolomei, F., Woodman, M. M., et al. (2020). The Bayesian virtual epileptic patient: a probabilistic framework designed to infer the spatial map of epileptogenicity in a personalized large-scale brain model of epilepsy spread. Neuroimage 217:116839. doi: 10.1016/j.neuroimage.2020.116839
Hasselmann, J., Coburn, M. A., England, W., Figueroa Velez, D. X., Kiani Shabestari, S., Tu, C. H., et al. (2019). Development of a chimeric model to study and manipulate human microglia in vivo. Neuron 103, 1016–1033.e10. doi: 10.1016/j.neuron.2019.07.002
HD iPSC Consortium (2012). Induced Pluripotent Stem Cells from Patients with Huntington’s Disease Show CAG-Repeat-Expansion-Associated Phenotypes. Cell Stem Cell 11, 264–278. doi: 10.1016/j.stem.2012.04.027
Hernandez, O., Way, S., McKenna, J., and Gambello, M. J. (2007). Generation of a conditional disruption of the Tsc2 gene. Genesis 45, 101–106. doi: 10.1002/dvg.20271
Hirose, S., Tanaka, Y., Shibata, M., Kimura, Y., Ishikawa, M., Higurashi, N., et al. (2020). Application of induced pluripotent stem cells in epilepsy. Mol. Cell Neurosci. 108:103535. doi: 10.1016/j.mcn.2020.103535
Hommersom, M. P., Buijsen, R. A. M., van Roon-Mom, W. M. C., van de Warrenburg, B. P. C., and van Bokhoven, H. (2022). Human induced pluripotent stem cell-based modelling of spinocerebellar ataxias. Stem Cell Rev. Rep. 18, 441–456. doi: 10.1007/s12015-021-10184-0
Hosoya, M., Fujioka, M., Sone, T., Okamoto, S., Akamatsu, W., Ukai, H., et al. (2017). Cochlear cell modeling using disease-specific iPSCs unveils a degenerative phenotype and suggests treatments for congenital progressive hearing loss. Cell Rep. 18, 68–81. doi: 10.1016/j.celrep.2016.12.020
Hussain, I., and Park, S. -J. (2021). Prediction of myoelectric biomarkers in post-stroke gait. Sensors 21:5334. doi: 10.3390/s21165334
Jirsa, V. K., Proix, T., Perdikis, D., Woodman, M. M., Wang, H., Gonzalez-Martinez, J., et al. (2017). The virtual epileptic patient: individualized whole-brain models of epilepsy spread. Neuroimage 145, 377–388. doi: 10.1016/j.neuroimage.2016.04.049
Jirsa, V. K., Stacey, W. C., Quilichini, P. P., Ivanov, A. I., and Bernard, C. (2014). On the nature of seizure dynamics. Brain 137, 2210–2230. doi: 10.1093/brain/awu133
Jirsa, V., Wang, H., Triebkorn, P., Hashemi, M., Jha, J., Gonzalez-Martinez, J., et al. (2023). Personalised virtual brain models in epilepsy. Lancet Neurol. 22, 443–454. doi: 10.1016/S1474-4422(23)00008-X
Jucker, M. (2010). The benefits and limitations of animal models for translational research in neurodegenerative diseases. Nat. Med. 16, 1210–1214. doi: 10.1038/nm.2224
Kabat, J., and Król, P. (2012). Focal cortical dysplasia - review. Pol. J. Radiol. 77, 35–43. doi: 10.12659/pjr.882968
Kashii, H., Kasai, S., Sato, A., Hagino, Y., Nishito, Y., Kobayashi, T., et al. (2023). Tsc2 mutation rather than Tsc1 mutation dominantly causes a social deficit in a mouse model of tuberous sclerosis complex. Hum. Genomics 17:4. doi: 10.1186/s40246-023-00450-2
Kelley, K. W., Revah, O., Gore, F., Kaganovsky, K., Chen, X., Deisseroth, K., et al. (2024). Host circuit engagement of human cortical organoids transplanted in rodents. Nat. Protoc. 19, 3542–5367. doi: 10.1038/s41596-024-01029-4
Kiernan, M. C., Vucic, S., Cheah, B. C., Turner, M. R., Eisen, A., Hardiman, O., et al. (2011). Amyotrophic lateral sclerosis. Lancet 377, 942–955. doi: 10.1016/S0140-6736(10)61156-7
Klockgether, T., Mariotti, C., and Paulson, H. L. (2019). Spinocerebellar ataxia. Nat. Rev. Dis. Primers 5:24. doi: 10.1038/s41572-019-0074-3
Klofas, L. K., Short, B. P., Snow, J. P., Sinnaeve, J., Rushing, G. V., Westlake, G., et al. (2020). DEPDC5 haploinsufficiency drives increased mTORC1 signaling and abnormal morphology in human iPSC-derived cortical neurons. Neurobiol. Dis. 143:104975. doi: 10.1016/j.nbd.2020.104975
Knock, E., and Julian, L. M. (2021). Building on a solid foundation: adding relevance and reproducibility to neurological modeling using human pluripotent stem cells. Front. Cell Neurosci. 15:767457. doi: 10.3389/fncel.2021.767457
Kobayashi, T., Minowa, O., Kuno, J., Mitani, H., Hino, O., Noda, T., et al. (1999). Renal carcinogenesis, hepatic hemangiomatosis, and embryonic lethality caused by a germ-line Tsc2 mutation in mice. Cancer Res. 59, 1206–1211.
Koníěková, D., Menšíková, K., Tuěková, L., Hényková, E., Strnad, M., Friedeckı, D., et al. (2022). Biomarkers of neurodegenerative diseases: biology, taxonomy, clinical relevance, and current research status. Biomedicines 10:1760. doi: 10.3390/biomedicines10071760
Kosorok, M. R., and Laber, E. B. (2019). Precision medicine. Annu. Rev. Stat. Appl. 6, 263–286. doi: 10.1146/annurev-statistics-030718-105251
Kusumoto, D., Yuasa, S., and Fukuda, K. (2022). Induced pluripotent stem cell-based drug screening by use of artificial intelligence. Pharmaceuticals (Basel) 15:562. doi: 10.3390/ph15050562
Lancaster, M. A., and Knoblich, J. A. (2014). Generation of cerebral organoids from human pluripotent stem cells. Nat. Protoc. 9, 2329–2340. doi: 10.1038/nprot.2014.158
Laperle, A. H., Sances, S., Yucer, N., Dardov, V. J., Garcia, V. J., Ho, R., et al. (2020). iPSC modeling of young-onset Parkinson’s disease reveals a molecular signature of disease and novel therapeutic candidates. Nat. Med. 26, 289–299. doi: 10.1038/s41591-019-0739-1
Lavanga, M., Stumme, J., Yalcinkaya, B. H., Fousek, J., Jockwitz, C., Sheheitli, H., et al. (2023). The virtual aging brain: causal inference supports interhemispheric dedifferentiation in healthy aging. Neuroimage 283:120403. doi: 10.1016/j.neuroimage.2023.120403
Lewis, E. M. A., Meganathan, K., Baldridge, D., Gontarz, P., Zhang, B., Bonni, A., et al. (2019). Cellular and molecular characterization of multiplex autism in human induced pluripotent stem cell-derived neurons. Mol. Autism 10:51. doi: 10.1186/s13229-019-0306-0
Li, Y., Muffat, J., Omer, A., Bosch, I., Lancaster, M. A., Sur, M., et al. (2017). Induction of expansion and folding in human cerebral organoids. Cell Stem Cell 20, 385–396.e3. doi: 10.1016/j.stem.2016.11.017
Lippmann, E. S., Azarin, S. M., Kay, J. E., Nessler, R. A., Wilson, H. K., Al-Ahmad, A., et al. (2012). Derivation of blood-brain barrier endothelial cells from human pluripotent stem cells. Nat. Biotechnol. 30:783. doi: 10.1038/nbt.2247
Liu, G., David, B. T., Trawczynski, M., and Fessler, R. G. (2020). Advances in pluripotent stem cells: history, mechanisms, technologies, and applications. Stem Cell Rev. Rep. 16, 3–32. doi: 10.1007/s12015-019-09935-x
Liu, H., and Zhang, S. C. (2011). Specification of neuronal and glial subtypes from human pluripotent stem cells. Cell Mol. Life Sci. 68, 3995–4008. doi: 10.1007/s00018-011-0770-y
Liu, Y., Lopez-Santiago, L. F., Yuan, Y., Jones, J. M., Zhang, H., O’Malley, H. A., et al. (2013). Dravet syndrome patient-derived neurons suggest a novel epilepsy mechanism. Ann. Neurol. 74, 128–139. doi: 10.1002/ana.23897
Luchena, C., Zuazo-Ibarra, J., Valero, J., Matute, C., Alberdi, E., Capetillo-Zarate, E., et al. (2022). A neuron, microglia, and astrocyte triple co-culture model to study Alzheimer’s disease. Front. Aging Neurosci. 14:844534. doi: 10.3389/fnagi.2022.844534
Lui, K. N., Li, Z., Lai, F. P., Lau, S. T., and Ngan, E. S. (2023). Organoid models of breathing disorders reveal patterning defect of hindbrain neurons caused by PHOX2B-PARMs. Stem Cell Rep. 18, 1500–1515. doi: 10.1016/j.stemcr.2023.05.020
Macron, C., Núńez Galindo, A., Cominetti, O., and Dayon, L. (2019). A versatile workflow for cerebrospinal fluid proteomic analysis with mass spectrometry: a matter of choice between deep coverage and sample throughput. Methods Mol. Biol. 2044, 129–154. doi: 10.1007/978-1-4939-9706-0_9
Maestú, F., de Haan, W., Busche, M. A., and DeFelipe, J. (2021). Neuronal excitation/inhibition imbalance: core element of a translational perspective on Alzheimer pathophysiology. Ageing Res. Rev. 69:101372. doi: 10.1016/j.arr.2021.101372
Majolo, F., Marinowic, D. R., Palmini, A. L. F., DaCosta, J. C., and Machado, D. C. (2019). Migration and synaptic aspects of neurons derived from human induced pluripotent stem cells from patients with focal cortical dysplasia II. Neuroscience 408, 81–90. doi: 10.1016/j.neuroscience.2019.03.025
Makhoba, X. H., Viegas, C., Mosa, R. A., Viegas, F. P. D., and Pooe, O. J. (2020). Potential impact of the multi-target drug approach in the treatment of some complex diseases. Drug Des. Devel. Ther. 14, 3235–3249. doi: 10.2147/DDDT.S257494
Manzini, A., Jones, E. J. H., Charman, T., Elsabbagh, M., Johnson, M. H., Singh, I., et al. (2021). Ethical dimensions of translational developmental neuroscience research in autism. J. Child Psychol. Psychiatry 62, 1363–1373. doi: 10.1111/jcpp.13494
Marchetto, M. C., Carromeu, C., Acab, A., Yu, D., Yeo, G. W., Mu, Y., et al. (2010). A model for neural development and treatment of Rett syndrome using human induced pluripotent stem cells. Cell 143, 527–539. doi: 10.1016/j.cell.2010.10.016
Marshall, L. J., Bailey, J., Cassotta, M., Herrmann, K., and Pistollato, F. (2023). Poor translatability of biomedical research using animals - A narrative review. Altern. Lab. Anim. 51, 102–135. doi: 10.1177/02611929231157756
Masuda, T., Sankowski, R., Staszewski, O., and Prinz, M. (2020). Microglia heterogeneity in the single-cell era. Cell Rep. 30, 1271–1281. doi: 10.1016/j.celrep.2020.01.010
Mayhew, C. N., and Singhania, R. (2023). A review of protocols for brain organoids and applications for disease modeling. STAR Protoc. 4:101860. doi: 10.1016/j.xpro.2022.101860
Mehl, L. C., Manjally, A. V., Bouadi, O., Gibson, E. M., and Tay, T. L. (2022). Microglia in brain development and regeneration. Development 149:dev200425. doi: 10.1242/dev.200425
Mikitsh, J. L., and Chacko, A.-M. (2014). Pathways for small molecule delivery to the central nervous system across the blood-brain barrier. Perspect. Med. Chem. 6, 11–24. doi: 10.4137/pmc.s13384
Miura, Y., Li, M. Y., Birey, F., Ikeda, K., Revah, O., Thete, M. V., et al. (2020). Generation of human striatal organoids and cortico-striatal assembloids from human pluripotent stem cells. Nat. Biotechnol. 38, 1421–1430. doi: 10.1038/s41587-020-00763-w
Møller, R. S., Weckhuysen, S., Chipaux, M., Marsan, E., Taly, V., Bebin, E. M., et al. (2016). Germline and somatic mutations in the MTOR gene in focal cortical dysplasia and epilepsy. Neurol. Genet. 2:e118. doi: 10.1212/NXG.0000000000000118
Morimoto, S., Takahashi, S., Ito, D., Daté, Y., Okada, K., Kato, C., et al. (2023). Phase 1/2a clinical trial in ALS with ropinirole, a drug candidate identified by iPSC drug discovery. Cell Stem Cell 30, 766–780.e9. doi: 10.1016/j.stem.2023.04.017
Muffat, J., Li, Y., Yuan, B., Mitalipova, M., Omer, A., Corcoran, S., et al. (2016). Efficient derivation of microglia-like cells from human pluripotent stem cells. Nat. Med. 22, 1358–1367. doi: 10.1038/nm.4189
Murai, K., Sun, G., Ye, P., Tian, E., Yang, S., Cui, Q., et al. (2016). The TLX-miR-219 cascade regulates neural stem cell proliferation in neurodevelopment and schizophrenia iPSC model. Nat. Commun. 7:10965. doi: 10.1038/ncomms10965
Muratore, C. R., Srikanth, P., Callahan, D. G., and Young-Pearse, T. L. (2014). Comparison and optimization of hiPSC forebrain cortical differentiation protocols. PLoS One 9:e105807. doi: 10.1371/journal.pone.0105807
Nadadhur, A. G., Alsaqati, M., Gasparotto, L., Cornelissen-Steijger, P., van Hugte, E., Dooves, S., et al. (2019). Neuron-glia interactions increase neuronal phenotypes in tuberous sclerosis complex patient iPSC-derived models. Stem Cell Rep. 12, 42–56. doi: 10.1016/j.stemcr.2018.11.019
Negri, J., Menon, V., and Young-Pearse, T. L. (2020). Assessment of spontaneous neuronal activity. eNeuro 7: :ENEURO.0080-19.2019. doi: 10.1523/ENEURO.0080-19.2019
Nieto-Estévez, V., and Hsieh, J. (2020). Human brain organoid models of developmental epilepsies. Epilepsy Curr. 20, 282–290. doi: 10.1177/1535759720949254
Nishino, K., Takasawa, K., Okamura, K., Arai, Y., Sekiya, A., Akutsu, H., et al. (2021). Identification of an epigenetic signature in human induced pluripotent stem cells using a linear machine learning model. Hum. Cell 34, 99–110. doi: 10.1007/s13577-020-00446-3
Nowakowski, T. J., Pollen, A. A., Sandoval-Espinosa, C., and Kriegstein, A. R. (2016). Transformation of the radial glia scaffold demarcates two stages of human cerebral cortex development. Neuron 91, 1219–1227. doi: 10.1016/j.neuron.2016.09.005
Núńez Galindo, A., Macron, C., Cominetti, O., and Dayon, L. (2019). Analyzing cerebrospinal fluid proteomes to characterize central nervous system disorders: a highly automated mass spectrometry-based pipeline for biomarker discovery. Methods Mol. Biol. 1959, 89–112. doi: 10.1007/978-1-4939-9164-8_6
Nzou, G., Wicks, R. T., Wicks, E. E., Seale, S. A., Sane, C. H., Chen, A., et al. (2018). Human cortex spheroid with a functional blood brain barrier for high-throughput neurotoxicity screening and disease modeling. Sci. Rep. 8:7413. doi: 10.1038/s41598-018-25603-5
Ochalek, A., Mihalik, B., Avci, H. X., Chandrasekaran, A., Téglási, A., Bock, I., et al. (2017). Neurons derived from sporadic Alzheimer’s disease iPSCs reveal elevated TAU hyperphosphorylation, increased amyloid levels, and GSK3B activation. Alzheimers Res. Ther. 9:90. doi: 10.1186/s13195-017-0317-z
Oegema, R., Barakat, T. S., Wilke, M., Stouffs, K., Amrom, D., Aronica, E., et al. (2020). International consensus recommendations on the diagnostic work-up for malformations of cortical development. Nat. Rev. Neurol. 16, 618–635. doi: 10.1038/s41582-020-0395-6
Okano, H., and Morimoto, S. (2022). iPSC-based disease modeling and drug discovery in cardinal neurodegenerative disorders. Cell Stem Cell 29, 189–208. doi: 10.1016/j.stem.2022.01.007
Pardridge, W. M. (2012). Drug transport across the blood–brain barrier. J. Cereb. Blood Flow Metab. 32:1959. doi: 10.1038/jcbfm.2012.126
Paşca, S. P., Arlotta, P., Bateup, H. S., Camp, J. G., Cappello, S., Gage, F. H., et al. (2022). A nomenclature consensus for nervous system organoids and assembloids. Nature 609, 907–910. doi: 10.1038/s41586-022-05219-6
Paşca, S. P., Portmann, T., Voineagu, I., Yazawa, M., Shcheglovitov, A., Paşca, A. M., et al. (2011). Using iPSC-derived neurons to uncover cellular phenotypes associated with Timothy syndrome. Nat. Med. 17, 1657–1662. doi: 10.1038/nm.2576
Paull, D., Sevilla, A., Zhou, H., Hahn, A. K., Kim, H., Napolitano, C., et al. (2015). Automated, high-throughput derivation, characterization and differentiation of induced pluripotent stem cells. Nat. Methods 12:885. doi: 10.1038/nmeth.3507
Pediaditakis, I., Kodella, K. R., Manatakis, D. V., Le, C. Y., Barthakur, S., Sorets, A., et al. (2022). A microengineered Brain-Chip to model neuroinflammation in humans. iScience 25:104813. doi: 10.1016/j.isci.2022.104813
Pellegrini, L., Bonfio, C., Chadwick, J., Begum, F., Skehel, M., Lancaster, M. A., et al. (2020). Human CNS barrier-forming organoids with cerebrospinal fluid production. Science 369, eaaz5626. doi: 10.1126/science.aaz5626
Perucca, P., and Perucca, E. (2019). Identifying mutations in epilepsy genes: impact on treatment selection. Epilepsy Res. 152, 18–30. doi: 10.1016/j.eplepsyres.2019.03.001
Peters, J. U. (2013). Polypharmacology - foe or friend? J. Med. Chem. 56, 8955–8971. doi: 10.1021/jm400856t
Pilotto, F., Douthwaite, C., Diab, R., Ye, X., Al Qassab, Z., Tietje, C., et al. (2023). Early molecular layer interneuron hyperactivity triggers Purkinje neuron degeneration in SCA1. Neuron 111, 2523–2543.e10. doi: 10.1016/j.neuron.2023.05.016
Pradhan, J., and Bellingham, M. C. (2021). Neurophysiological mechanisms underlying cortical hyper-excitability in amyotrophic lateral sclerosis: a review. Brain Sci. 11:549. doi: 10.3390/brainsci11050549
Proix, T., Bartolomei, F., Guye, M., and Jirsa, V. K. (2017). Individual brain structure and modelling predict seizure propagation. Brain 140, 641–654. doi: 10.1093/brain/awx004
Qian, T., Maguire, S. E., Canfield, S. G., Bao, X., Olson, W. R., Shusta, E. V., et al. (2017). Directed differentiation of human pluripotent stem cells to blood-brain barrier endothelial cells. Sci. Adv. 3:e1701679. doi: 10.1126/sciadv.1701679
Reddy, P. H. (2009). Role of mitochondria in neurodegenerative diseases: mitochondria as a therapeutic target in Alzheimer’s disease. CNS Spectr. 14, 8–13; discussion 16–18. doi: 10.1017/s1092852900024901
Revah, O., Gore, F., Kelley, K. W., Andersen, J., Sakai, N., Chen, X., et al. (2022). Maturation and circuit integration of transplanted human cortical organoids. Nature 610, 319–326. doi: 10.1038/s41586-022-05277-w
Reymond, J. L. (2015). The chemical space project. Acc. Chem. Res. 48, 722–730. doi: 10.1021/ar500432k
Rocktäschel, P., Sen, A., and Cader, M. Z. (2019). High glucose concentrations mask cellular phenotypes in a stem cell model of tuberous sclerosis complex. Epilepsy Behav. 101:106581. doi: 10.1016/j.yebeh.2019.106581
Ronaldson, P. T., and Davis, T. P. (2020). Regulation of blood-brain barrier integrity by microglia in health and disease: a therapeutic opportunity. J. Cereb. Blood Flow Metab. 40, S6–S24. doi: 10.1177/0271678X20951995
Roqué, P. J., and Costa, L. G. (2017). Co-culture of neurons and microglia. Curr. Protoc. Toxicol. 74, 11.24.11–11.24.17. doi: 10.1002/cptx.32
Rousseaux, M. W. C., Tschumperlin, T., Lu, H. C., Lackey, E. P., Bondar, V. V., Wan, Y. W., et al. (2018). ATXN1-CIC complex is the primary driver of cerebellar pathology in spinocerebellar ataxia type 1 through a gain-of-function mechanism. Neuron 97, 1235–1243.e5. doi: 10.1016/j.neuron.2018.02.013
Ruddigkeit, L., van Deursen, R., Blum, L. C., and Reymond, J. L. (2012). Enumeration of 166 billion organic small molecules in the chemical universe database GDB-17. J. Chem. Inf. Model. 52, 2864–2875. doi: 10.1021/ci300415d
Saberi, A., Aldenkamp, A. P., Kurniawan, N. A., and Bouten, C. V. C. (2022). In-vitro engineered human cerebral tissues mimic pathological circuit disturbances in 3D. Commun. Biol. 5:254. doi: 10.1038/s42003-022-03203-4
Saito, A., Ooki, A., Nakamura, T., Onodera, S., Hayashi, K., Hasegawa, D., et al. (2018). Targeted reversion of induced pluripotent stem cells from patients with human cleidocranial dysplasia improves bone regeneration in a rat calvarial bone defect model. Stem Cell Res. Ther. 9:12. doi: 10.1186/s13287-017-0754-4
Sakai, C., Ijaz, S., and Hoffman, E. J. (2018). Zebrafish models of neurodevelopmental disorders: past, present, and future. Front. Mol. Neurosci. 11:294. doi: 10.3389/fnmol.2018.00294
Sances, S., Bruijn, L. I., Chandran, S., Eggan, K., Ho, R., Klim, J. R., et al. (2016). Modeling ALS with motor neurons derived from human induced pluripotent stem cells. Nat. Neurosci. 19, 542–553. doi: 10.1038/nn.4273
Sasaguri, H., Nilsson, P., Hashimoto, S., Nagata, K., Saito, T., De Strooper, B., et al. (2017). APP mouse models for Alzheimer’s disease preclinical studies. EMBO J. 36, 2473–2487. doi: 10.15252/embj.201797397
Savitt, J. M., Dawson, V. L., and Dawson, T. M. (2006). Diagnosis and treatment of Parkinson disease: molecules to medicine. J. Clin. Invest. 116, 1744–1754. doi: 10.1172/JCI29178
Schilde, L. M., Steinbach, S., Serschnitzki, B., Maass, F., Bähr, M., Lingor, P., et al. (2020). Human cerebrospinal fluid data for use as spectral library, for biomarker research. Data Brief. 32:106048. doi: 10.1016/j.dib.2020.106048
Schirner, M., Domide, L., Perdikis, D., Triebkorn, P., Stefanovski, L., Pai, R., et al. (2022). Brain simulation as a cloud service: the virtual brain on EBRAINS. Neuroimage 251:118973. doi: 10.1016/j.neuroimage.2022.118973
Schirner, M., McIntosh, A. R., Jirsa, V., Deco, G., and Ritter, P. (2018). Inferring multi-scale neural mechanisms with brain network modelling. Elife 7:e28927. doi: 10.7554/eLife.28927
Seidel, K., Bouzrou, M., Heidemann, N., Krüger, R., Schöls, L., den Dunnen, W. F. A., et al. (2017). Involvement of the cerebellum in Parkinson disease and dementia with Lewy bodies. Ann. Neurol. 81, 898–903. doi: 10.1002/ana.24937
Selkoe, D. J. (2001). Alzheimer’s disease: genes, proteins, and therapy. Physiol. Rev. 81, 741–766. doi: 10.1152/physrev.2001.81.2.741
Sengupta, P., and Samuel, A. D. (2009). Caenorhabditis elegans: a model system for systems neuroscience. Curr. Opin. Neurobiol. 19, 637–643. doi: 10.1016/j.conb.2009.09.009
Silva, T. P., Fernandes, T. G., Nogueira, D. E. S., Rodrigues, C. A. V., Bekman, E. P., Hashimura, Y., et al. (2020). Scalable generation of mature cerebellar organoids from human pluripotent stem cells and characterization by immunostaining. 160, J. Vis. Exp. doi: 10.3791/61143
Silverman, L., Campbell, R., and Broach, J. R. (1998). New assay technologies for high-throughput screening. Curr. Opin. Chem. Biol. 2:397. doi: 10.1016/s1367-5931(98)80015-x
Silvin, A., Uderhardt, S., Piot, C., Da Mesquita, S., Yang, K., Geirsdottir, L., et al. (2022). Dual ontogeny of disease-associated microglia and disease inflammatory macrophages in aging and neurodegeneration. Immunity 55, 1448–1465.e6. doi: 10.1016/j.immuni.2022.07.004
Sittampalam, G. S., Kahl, S. D., and Janzen, W. P. (1997). High-throughput screening: advances in assay technologies. Curr. Opin. Chem. Biol. 1:384. doi: 10.1016/s1367-5931(97)80078-6
Sloan, S. A., Andersen, J., Paşca, A. M., Birey, F., and Paşca, S. P. (2018). Generation and assembly of human brain region-specific three-dimensional cultures. Nat. Protoc. 13, 2062–2085. doi: 10.1038/s41596-018-0032-7
Sohn, Y. D., Han, J. W., and Yoon, Y. S. (2012). Generation of induced pluripotent stem cells from somatic cells. Prog. Mol. Biol. Transl. Sci. 111, 1–26. doi: 10.1016/B978-0-12-398459-3.00001-0
Solár, P., Zamani, A., Kubíěková, L., Dubovı, P., and Joukal, M. (2020). Choroid plexus and the blood-cerebrospinal fluid barrier in disease. Fluids Barriers CNS 17:35. doi: 10.1186/s12987-020-00196-2
Solodkin, A., Peri, E., Chen, E. E., Ben-Jacob, E., and Gomez, C. M. (2011). Loss of intrinsic organization of cerebellar networks in spinocerebellar ataxia type 1: correlates with disease severity and duration. Cerebellum 10, 218–232. doi: 10.1007/s12311-010-0214-5
Somanadhan, S., O’Donnell, R., Bracken, S., McNulty, S., Sweeney, A., O’Toole, D., et al. (2023). Children and young people’s experiences of living with rare diseases: an integrative review. J. Pediatr. Nurs. 68, e16–e26. doi: 10.1016/j.pedn.2022.10.014
Song, L., Yuan, X., Jones, Z., Vied, C., Miao, Y., Marzano, M., et al. (2019). Functionalization of brain region-specific spheroids with isogenic microglia-like cells. Sci. Rep. 9:11055. doi: 10.1038/s41598-019-47444-6
Speicher, A. M., Wiendl, H., Meuth, S. G., and Pawlowski, M. (2019). Generating microglia from human pluripotent stem cells: novel in vitro models for the study of neurodegeneration. Mol. Neurodegener. 14:46. doi: 10.1186/s13024-019-0347-z
Stern, S., Santos, R., Marchetto, M. C., Mendes, A. P. D., Rouleau, G. A., Biesmans, S., et al. (2018). Neurons derived from patients with bipolar disorder divide into intrinsically different sub-populations of neurons, predicting the patients’ responsiveness to lithium. Mol. Psychiatry 23, 1453–1465. doi: 10.1038/mp.2016.260
Sun, Y., and Dolmetsch, R. E. (2018). Investigating the therapeutic mechanism of cannabidiol in a human Induced Pluripotent Stem Cell (iPSC)-based model of dravet syndrome. Cold Spring Harb. Symp. Quant. Biol. 83, 185–191. doi: 10.1101/sqb.2018.83.038174
Sun, Y., Paşca, S. P., Portmann, T., Goold, C., Worringer, K. A., Guan, W., et al. (2016). A deleterious Nav1.1 mutation selectively impairs telencephalic inhibitory neurons derived from Dravet Syndrome patients. Elife 5:e13073. doi: 10.7554/eLife.13073
Tagliatti, E., Desiato, G., Mancinelli, S., Bizzotto, M., Gagliani, M. C., Faggiani, E., et al. (2024). Trem2 expression in microglia is required to maintain normal neuronal bioenergetics during development. Immunity 57, 86–105.e9. doi: 10.1016/j.immuni.2023.12.002
Takahashi, K., and Yamanaka, S. (2006). Induction of pluripotent stem cells from mouse embryonic and adult fibroblast cultures by defined factors. Cell 126, 663–676. doi: 10.1016/j.cell.2006.07.024
Takahashi, K., Tanabe, K., Ohnuki, M., Narita, M., Ichisaka, T., Tomoda, K., et al. (2007). Induction of pluripotent stem cells from adult human fibroblasts by defined factors. Cell 131, 861–872. doi: 10.1016/j.cell.2007.11.019
Tasnim, K., and Liu, J. (2022). Emerging bioelectronics for brain organoid electrophysiology. J. Mol. Biol. 434:167165. doi: 10.1016/j.jmb.2021.167165
Teles, D., Kim, Y., Ronaldson-Bouchard, K., and Vunjak-Novakovic, G. (2021). Machine learning techniques to classify healthy and diseased cardiomyocytes by contractility profile. ACS Biomater. Sci. Eng. 7, 3043–3052. doi: 10.1021/acsbiomaterials.1c00418
Terstegge, S., Laufenberg, I., Pochert, J., Schenk, S., Itskovitz-Eldor, J., Endl, E., et al. (2007). Automated maintenance of embryonic stem cell cultures. Biotechnol. Bioeng. 96:195. doi: 10.1002/bit.21061
Thion, M. S., Ginhoux, F., and Garel, S. (2018). Microglia and early brain development: an intimate journey. Science 362, 185–189. doi: 10.1126/science.aat0474
Thomas, R. J., Anderson, D., Chandra, A., Smith, N. M., Young, L. E., Williams, D., et al. (2009). Automated, scalable culture of human embryonic stem cells in feeder-free conditions. Biotechnol. Bioeng. 102:1636. doi: 10.1002/bit.22187
Tian, Y., Zhu, P., Liu, S., Jin, Z., Li, D., Zhao, H., et al. (2019). IL-4-polarized BV2 microglia cells promote angiogenesis by secreting exosomes. Adv. Clin. Exp. Med. 28, 421–430. doi: 10.17219/acem/91826
Timmerman, R., Burm, S. M., and Bajramovic, J. J. (2018). An overview of in vitro methods to study microglia. Front. Cell Neurosci. 12:242. doi: 10.3389/fncel.2018.00242
Tremlett, H., and Marrie, R. A. (2021). The multiple sclerosis prodrome: emerging evidence, challenges, and opportunities. Mult. Scler. 27, 6–12. doi: 10.1177/1352458520914844
Tristan, C. A., Ormanoglu, P., Slamecka, J., Malley, C., Chu, P. H., Jovanovic, V. M., et al. (2021). Robotic high-throughput biomanufacturing and functional differentiation of human pluripotent stem cells. Stem Cell Rep. 16:3076. doi: 10.1016/j.stemcr.2021.11.004
Umair, M., and Waqas, A. (2023). Undiagnosed rare genetic disorders: importance of functional characterization of variants. Genes (Basel) 14:1469. doi: 10.3390/genes14071469
Usui-Ouchi, A., Giles, S., Harkins-Perry, S., Mills, E. A., Bonelli, R., Wei, G., et al. (2023). Integrating human iPSC-derived macrophage progenitors into retinal organoids to generate a mature retinal microglial niche. Glia 71, 2372–2382. doi: 10.1002/glia.24428
Vahsen, B., Gray, E., Candalija, A., Cramb, K. M. L., Scaber, J., Dafinca, R., et al. (2022). Human iPSC co-culture model to investigate the interaction between microglia and motor neurons. Sci. Rep. 12:12606. doi: 10.1038/s41598-022-16896-8
Valamehr, B., Abujarour, R., Robinson, M., Le, T., Robbins, D., Shoemaker, D., et al. (2012). A novel platform to enable the high-throughput derivation and characterization of feeder-free human iPSCs. Sci. Rep. 2:213. doi: 10.1038/srep00213
Vo, Q. D., Saito, Y., Ida, T., Nakamura, K., and Yuasa, S. (2024). The use of artificial intelligence in induced pluripotent stem cell-based technology over 10-year period: a systematic scoping review. PLoS One 19:e0302537. doi: 10.1371/journal.pone.0302537
Voigt, I., Inojosa, H., Dillenseger, A., Haase, R., Akgün, K., Ziemssen, T., et al. (2021). Digital twins for multiple sclerosis. Front. Immunol. 12:669811. doi: 10.3389/fimmu.2021.669811
Wevers, N. R., Kasi, D. G., Gray, T., Wilschut, K. J., Smith, B., van Vught, R., et al. (2018). A perfused human blood–brain barrier on-a-chip for high-throughput assessment of barrier function and antibody transport. Fluids Barriers CNS 15:23. doi: 10.1186/s12987-018-0108-3
Willis, E. F., MacDonald, K. P. A., Nguyen, Q. H., Garrido, A. L., Gillespie, E. R., Harley, S. B. R., et al. (2020). Repopulating microglia promote brain repair in an IL-6-dependent manner. Cell 180, 833–846.e16. doi: 10.1016/j.cell.2020.02.013
Winden, K. D., Sundberg, M., Yang, C., Wafa, S. M. A., Dwyer, S., Chen, P. F., et al. (2019). Biallelic mutations in TSC2 lead to abnormalities associated with cortical tubers in human iPSC-derived neurons. J. Neurosci. 39, 9294–9305. doi: 10.1523/JNEUROSCI.0642-19.2019
Wingo, T. S., Liu, Y., Gerasimov, E. S., Vattathil, S. M., Wynne, M. E., Liu, J., et al. (2022). Shared mechanisms across the major psychiatric and neurodegenerative diseases. Nat. Commun. 13:4314. doi: 10.1038/s41467-022-31873-5
Wörsdörfer, P., Dalda, N., Kern, A., Krüger, S., Wagner, N., Kwok, C. K., et al. (2019). Generation of complex human organoid models including vascular networks by incorporation of mesodermal progenitor cells. Sci. Rep. 9:15663. doi: 10.1038/s41598-019-52204-7
Xiang, Y., Tanaka, Y., Cakir, B., Patterson, B., Kim, K. Y., Sun, P., et al. (2019). hESC-derived thalamic organoids form reciprocal projections when fused with cortical organoids. Cell Stem Cell 24, 487–497.e7. doi: 10.1016/j.stem.2018.12.015
Xiang, Y., Tanaka, Y., Patterson, B., Kang, Y. J., Govindaiah, G., Roselaar, N., et al. (2017). Fusion of regionally specified hPSC-derived organoids models human brain development and interneuron migration. Cell Stem Cell 21, 383–398.e7. doi: 10.1016/j.stem.2017.07.007
Xu, R., Boreland, A. J., Li, X., Erickson, C., Jin, M., Atkins, C., et al. (2021). Developing human pluripotent stem cell-based cerebral organoids with a controllable microglia ratio for modeling brain development and pathology. Stem Cell Rep. 16, 1923–1937. doi: 10.1016/j.stemcr.2021.06.011
Yagi, T., Ito, D., Okada, Y., Akamatsu, W., Nihei, Y., Yoshizaki, T., et al. (2011). Modeling familial Alzheimer’s disease with induced pluripotent stem cells. Hum. Mol. Genet. 20, 4530–4539. doi: 10.1093/hmg/ddr394
Yan, J., Kuzhiumparambil, U., Bandodkar, S., Dale, R. C., and Fu, S. (2021). Cerebrospinal fluid metabolomics: detection of neuroinflammation in human central nervous system disease. Clin. Transl. Immunol. 10:e1318. doi: 10.1002/cti2.1318
Yang, S., Chang, R., Yang, H., Zhao, T., Hong, Y., Kong, H. E., et al. (2017). CRISPR/Cas9-mediated gene editing ameliorates neurotoxicity in mouse model of Huntington’s disease. J. Clin. Invest. 127, 2719–2724. doi: 10.1172/JCI92087
Yang, X., Forró, C., Li, T. L., Miura, Y., Zaluska, T. J., Tsai, C. T., et al. (2024). Kirigami electronics for long-term electrophysiological recording of human neural organoids and assembloids. Nat. Biotechnol. 42, 1836–1843. doi: 10.1038/s41587-023-02081-3
Yu, F. H., Mantegazza, M., Westenbroek, R. E., Robbins, C. A., Kalume, F., Burton, K. A., et al. (2006). Reduced sodium current in GABAergic interneurons in a mouse model of severe myoclonic epilepsy in infancy. Nat. Neurosci. 9, 1142–1149. doi: 10.1038/nn1754
Zeiss, C. J. (2017). From reproducibility to translation in neurodegenerative disease. ILAR J. 58, 106–114. doi: 10.1093/ilar/ilx006
Zerr, I., and Parchi, P. (2018). Sporadic creutzfeldt-jakob disease. Handb. Clin. Neurol. 153, 155–174. doi: 10.1016/B978-0-444-63945-5.00009-X
Zetterberg, H., and Burnham, S. C. (2019). Blood-based molecular biomarkers for Alzheimer’s disease. Mol. Brain 12:26. doi: 10.1186/s13041-019-0448-1
Keywords: precision medicine, hiPSCs, brain disorders, biomarkers, generative AI, brain organoids, neurophysiology
Citation: Imani Farahani N, Lin L, Nazir S, Naderi A, Rokos L, McIntosh AR and Julian LM (2025) Advances in physiological and clinical relevance of hiPSC-derived brain models for precision medicine pipelines. Front. Cell. Neurosci. 18:1478572. doi: 10.3389/fncel.2024.1478572
Received: 10 August 2024; Accepted: 13 December 2024;
Published: 06 January 2025.
Edited by:
Enrico Cherubini, European Brain Research Institute, ItalyReviewed by:
Mohammad Hasanain, University of Miami Health System, United StatesYohei Hayashi, RIKEN BioResource Research Center (BRC), Japan
Nishant Singhal, National Centre for Cell Science, India
Copyright © 2025 Imani Farahani, Lin, Nazir, Naderi, Rokos, McIntosh and Julian. This is an open-access article distributed under the terms of the Creative Commons Attribution License (CC BY). The use, distribution or reproduction in other forums is permitted, provided the original author(s) and the copyright owner(s) are credited and that the original publication in this journal is cited, in accordance with accepted academic practice. No use, distribution or reproduction is permitted which does not comply with these terms.
*Correspondence: Lisa M. Julian, bGp1bGlhbkBzZnUuY2E=