- 1Institute of Bioengineering, Universidad Miguel Hernández de Elche, Elche, Spain
- 2Biomedical Research Networking Center in Bioengineering, Biomaterials and Nanomedicine (CIBER-BBN), Madrid, Spain
- 3Departamento de Ciencia de Materiales, Óptica y Tecnología Electrónica, Universidad Miguel Hernández de Elche, Elche, Spain
Current cortical visual prosthesis approaches are primarily unidirectional and do not consider the feed-back circuits that exist in just about every part of the nervous system. Herein, we provide a brief overview of some recent developments for better controlling brain stimulation and present preliminary human data indicating that closed-loop strategies could considerably enhance the effectiveness, safety, and long-term stability of visual cortex stimulation. We propose that the development of improved closed-loop strategies may help to enhance our capacity to communicate with the brain.
Introduction
Visual impairment has a profound impact on the lives of those who experience it (Bourne et al., 2017). Although some novel clinical approaches are becoming available (Higuchi et al., 2017; De Silva and Moore, 2022; Panikker et al., 2022; Van Gelder et al., 2022), unfortunately, there is no treatment for all causes of blindness (Fernandez, 2018; Fernandez et al., 2020). Thus, there are many blind patients for whom there is still no medical treatment. As a consequence of this growing and clearly unmet need, numerous groups worldwide are pursuing other approaches to provide at least a rudimentary sense of vision to the blind.
Visual prostheses are promising solutions to restore functional vision (i.e., visual percepts that could help blind people to recognize objects or to navigate in complex environments). Retinal prostheses are the most successful approach in this field to date (Nowik et al., 2020; Picaud and Sahel, 2020; Nanegrungsunk et al., 2022), but patients with severe retinal degeneration, glaucoma, or optic atrophy cannot get benefit from a retinal prosthesis. Therefore, there are compelling reasons for the development of alternative approaches that can bypass the retina to restore a functional sense of vision.
In this framework, although the optic nerve or lateral geniculate nucleus could be good targets (Nguyen et al., 2016; Gaillet et al., 2020; Borda et al., 2022; Rassia et al., 2022), several groups are trying to develop visual prostheses designed to directly stimulate the visual cortex (Lee et al., 2016; Beauchamp et al., 2020; Fernandez et al., 2021; Bosking et al., 2022). However, the biological and engineering problems for the success of cortical implants are much more complex than originally believed and involve, for example, long-term biocompatibility issues and challenges related to the encoding of visual information and the delivery of information to implants (Fernandez et al., 2020). In addition, we should be aware that the human brain is arguably one of the most complex systems in nature and that cortical stimulation should be safe, precise, and effective.
To achieve the ambitious objectives envisioned by cortical visual prostheses, we should be able to stimulate the occipital cortex in a way as similar as possible to the physiological response to visual stimuli, mimicking the human visual pathway (Nirenberg and Pandarinath, 2012; Qiao et al., 2019; Brackbill et al., 2020; Li et al., 2022; Price and Gavornik, 2022). In this framework, we should consider that closed-loop circuits exist in just about every part of the nervous system (Farkhondeh Tale Navi et al., 2022; Khodagholy et al., 2022). However, current cortical visual prosthesis approaches are primarily unidirectional and do not incorporate any adaptive system for the modulation of the electrical stimulation used to induce visual perception. Herein, we briefly introduce some recent advances for better control of brain stimulation and present preliminary human data suggesting that a closed-loop approach could significantly improve the performance, safety, and long-term stability of the stimulation of visual cortex neurons.
Learning to control brain electrical stimulation
Electrical stimulation of the brain is the basis of many technologies for the restoration of sensory and motor functions. Brain stimulation has been used for reducing tremors in Parkinson’s patients, controlling epileptiform activity, and improving mood in patients with severe depression (Lozano et al., 2019). Additionally, it is now possible to create artificial sensations, with unprecedented resolution, via delivery of intracortical microstimulation (Fernandez et al., 2021; Fernandez, 2022; Fifer et al., 2022). However, most current brain stimulation approaches cannot flexibly control the patterns of activity because, for it to work, we need to know the activity of the neurons surrounding the electrodes and modulate the electrical stimulation in function of this neural activity.
Although stimulating electrodes allow control of the dynamics of populations of neurons, they do not provide insights into the electrophysiological activity of the neurons surrounding the electrodes. Thus, a critical step in the development of closed-loop approaches is the creation of microelectrodes and technologies capable of performing simultaneous stimulation and recording or neural activity.
Currently, bidirectional electrodes that allow stimulation and recording of neural activity at the same time exist, but are limited by the artifacts generated in the recordings by the stimulation (Xu et al., 2018). The detailed description of techniques and materials that allow for the recording of neural activity has been described elsewhere (Stevenson and Kording, 2011; Chen et al., 2017; Hong et al., 2021), but extracellular recordings are the more common type associated with in vivo brain recordings. Briefly, electrodes of the order of microns are implanted into the brain and positioned close enough to the neurons of interest to detect the fluctuations in voltage across their membranes. To record from several neurons, a series of microelectrodes can be organized to form a microelectrode array. The main advantage of these microelectrode arrays is that by recording from a number of neurons simultaneously, we can extract more accurately the complex patterns of neuronal activity and get some insights into the information flow (Hong et al., 2021).
Some recent works have led to the development of novel forms of neuromodulation, which are facilitating the ability to manipulate populations of neurons in near real-time. These techniques are based on recording the neural activity around the electrodes and adjusting the electrical stimulation in function of the observed neural activity (closed-loop stimulation). According to the use of the closed-loop approach, these techniques can be divided in device fitting techniques and therapy/efficacy techniques (Table 1).
This procedures allow an improved control of some neurological conditions such as epilepsy (Ranjandish and Schmid, 2020; Farkhondeh Tale Navi et al., 2022), and can also be used for better control of obsessive-compulsive disorders and depression (Figee et al., 2022; Visser-Vandewalle et al., 2022). Furthermore, it has been shown that the outcome of brain stimulation to treat Parkinson’s disease can be improved by recording brain activity and stimulating only when the local field potentials collected by electrodes inserted in the subthalamic nucleus exceed a certain threshold (Little et al., 2013), or by associating the brain stimulation to specific phases of patients’ tremor activity (Cagnan et al., 2017). Also, the electrical stimulation of the spinal cord for pain therapy can be adjusted based on the evoked compound action potential (ECAP) (Mekhail et al., 2020), while the movement output in spinal cord stimulation for motor recovery can be controlled in closed-loop changing the stimulation parameters (Wenger et al., 2014).
The above-mentioned approaches can also be applied to the field of sensory prostheses. Thus, the automatic tuning of stimulation thresholds in cochlear implants can be done by measuring the contraction of the stapedius muscle. This muscle contracts to protect the inner ear from very loud sounds (Borg and Zakrisson, 1973) and measuring its contraction provides objective feedback on the loudness of the sound induced by the electrical stimulation (Weiss et al., 2021). Other measures like ECAP (McKay et al., 2013), electrically evocated auditory brainstem response (EABR) (Guenser et al., 2015), cortical auditory evoked potentials (CAEPs) (Visram et al., 2015) have been studied to automatically fit cochlear implants, but none of them reached a clinical application.
In retinal prosthesis, research on closed-loop stimulation have been done to optimize the stimulation parameters to obtain the desired retinal ganglion cells output in response to a given visual input (Guo et al., 2018; Spencer et al., 2019; Shah and Chichilnisky, 2020). The same approach could be applied in cortical visual prosthesis using the activity of cortical neurons instead of retinal ganglion cells. For this to be optimal, a larger part of the visual field should be covered by the electrodes in cortical visual prostheses with respect to the current research devices.
However, even with a smaller covering of the visual field it should be feasible to design and develop similar approaches in the field of cortical visual prosthesis for controlling the timing of stimulation, reducing charge requirements, and fitting the device faster. In this framework, a recent study in monkey visual cortex shows that the activity of neurons in V4 provides direct insight into the efficacy of the stimulation in V1 (Chen et al., 2020). This suggests that neurons in higher visual areas could be used, for example, to estimate and adjust V1 thresholds on hundreds of electrodes. Furthermore, besides adjusting the thresholds, the brain signals collected by the electrodes in the visual cortex could reveal a brain state in which it is easier to induce perception. Some preliminary studies in rats support this point of view and show that it is possible to use the information from the local field potentials (LFPs) as control signals to specify the precise timing of stimulation to reduce charge requirements (Allison-Walker et al., 2020). Moreover, it has been shown in humans that there is a causal relationship between cortical excitation and phosphenes perception so that the phase of pre-stimulus oscillatory activity seems linked to visual perception (Dugue et al., 2011), and other studies suggest that the power spectral density at low frequency (f < 30 Hz) contains information about visual perception (Gail et al., 2004). Hence, we can hypothesize that the incorporation of measures of neural activity around stimulating electrodes could be helpful to enhance the effectiveness and safety of any cortical visual prostheses. In addition, we could also incorporate other measures such as linear combinations of brain signals in different bandwidths and even information about the pupil size and eye movements to improve the safety, robustness, and reliability of conscious visual perceptions (van Vugt et al., 2018). Table 1 presents some closed-loop neural stimulation approaches currently used, specifying if they are in clinical or research status.
Toward personalized closed-loop stimulation in cortical visual prostheses
Currently, most cortical visual prostheses are primarily unidirectional or open-loop, passing the visual information from the outside world captured by the image acquisition sensors to the implanted microelectrode arrays. In the future, it is expected that a high number of microelectrodes can be implanted into the brain to provide a functional vision, and such a large number of implanted electrodes may pose several stimulation problems (Fernandez, 2018; Rotermund et al., 2019). Therefore, we have to start reconsidering and improving our methods of cortical stimulation for example with closed-loop approaches (Figure 1A; Rotermund et al., 2019).
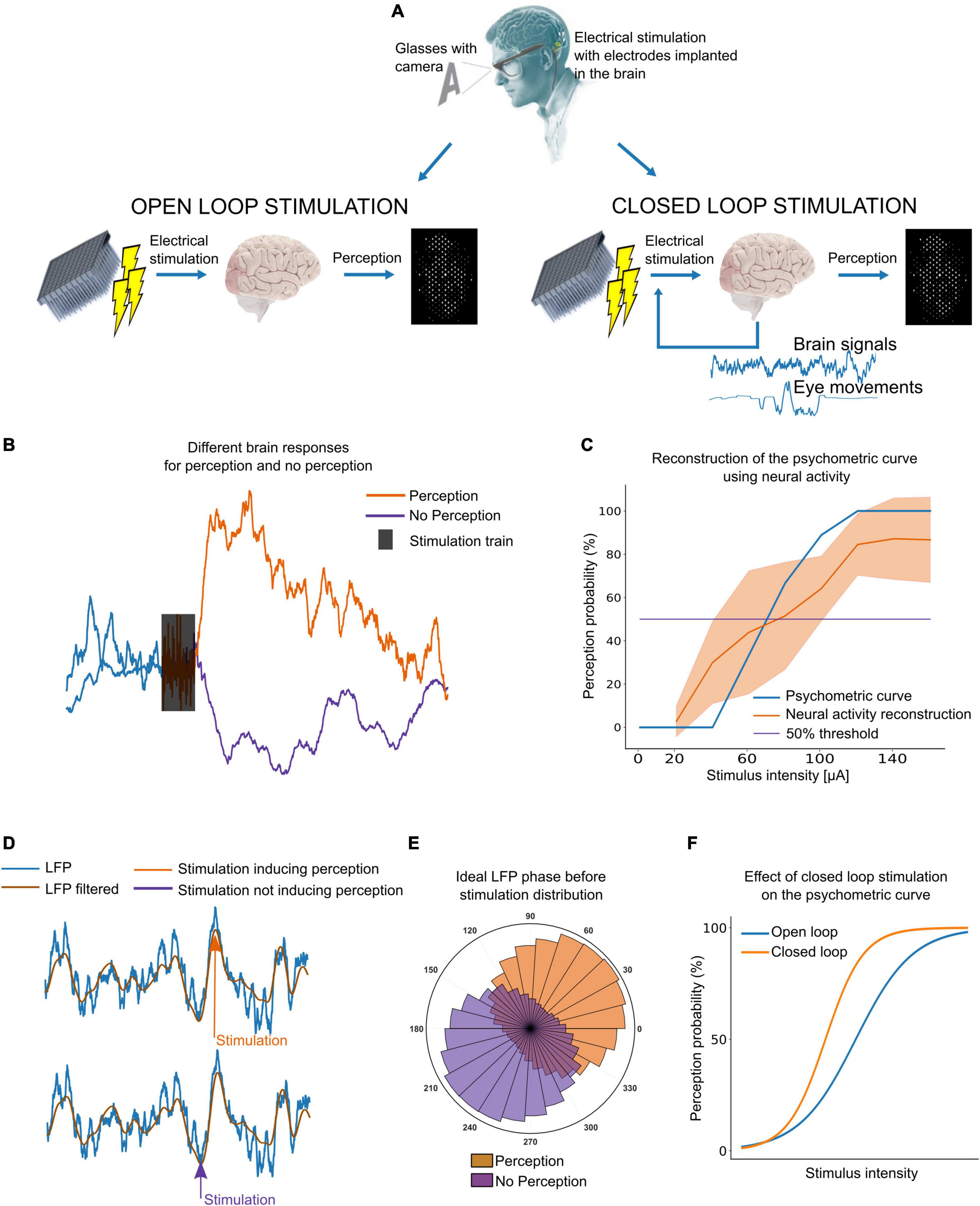
Figure 1. Closed-loop stimulation of the visual cortex. (A) Diagram of open-loop versus closed-loop stimulation approaches. (B) Example of intracortical brain signals. A clear difference between perception and no perception is needed to adjust the current to induce perception without the user’s feedback. (C) Example of a psychometric curve obtained with user’s feedback (blue) and neural signals (orange). (D) Local field potentials (LFP) phase dependent response to stimulation. Inducing perception might be easier by stimulating the right LFP phase. (E) Ideally, the distribution of LFP phase should be different for perception and no perception. (F) Stimulating at the right LFP phase, we could decrease the charge required to reliably evoke phosphenes.
It has been estimated that we need at least 625 electrodes implanted in visual areas for reading (although at lower speeds) and navigating through complex visual environments (Cha et al., 1992). However, finding the lowest current thresholds able to induce visual perceptions from each single electrode is a time-consuming procedure that requires the user’s feedback. Moreover, perception thresholds could vary over time, requiring the users to calibrate each electrode many times. As the brain signals surrounding the electrodes contain information about the spread of currents and brain dynamics, we could determine if the currents used are enough to induce perception simply by measuring the brain response to electrical stimulation. For this to be possible, the brain signals during (or after) stimulation should have distinguishable features in case of perception or no perception. Figure 1B shows an example from our ongoing experiments with intracortical microelectrodes in blind volunteers in which the neuronal activity after stimulation increases when the stimulation intensity is enough to induce perception (40 μA in this case). This approach can also be used to construct psychometric curves (relation between stimulus intensity and perception probability) that are practically indistinguishable of the standard psychometric curves using users’ feed-back. Figure 1C shows an example for a single electrode using current intensities from 0 to 140 μA. However, the most robust and reliable features to automatically find perception thresholds still need to be investigated.
On the other hand, there is a need to reduce power consumption and the charge required to elicit reliable phosphenes. In this context, brain activity and other physiological signals could be used to find a brain state in which inducing visual perception is easier, thus decreasing the currents needed to induce the visual perceptions. Using the same stimulation parameters, a given pulse train might produce a visual perception or not according to the LFP phase at which the stimulation is sent (Figures 1D,E). This has been reported in experiments in rats (Allison-Walker et al., 2020) and using non-invasive transcranial magnetic stimulation (TMS) in sighted humans (Dugue et al., 2011), but the feasibility of LFP phase-locked stimulation with intracortical electrodes in humans still remains unexplored. Nevertheless, targeting the right LFP phase before stimulation could allow to reduce charge requirements. Figure 1F shows an example.
Algorithms for closed-loop stimulation
Figure 2 introduces some possible algorithms for closed-loop stimulation in the framework of a cortical visual prosthesis. Briefly, to search for perception thresholds (Figure 2A), a stimulation with an initial current level I0 is sent from one electrode or a group of electrodes. Then, the brain signals during and after the stimulation (up to 1 s) are recorded and used to extract perception-related features. If perception is detected, the current I used to stimulate is set as the perception threshold for that electrode or group of electrodes. If perception is not detected from the extracted features, a new stimulation train is sent with a higher current intensity I = I–1 + ΔI, where I–1 is the previous current intensity and ΔI is the increment of current intensity for each step. The velocity of this algorithm to find perception thresholds depends on the initial current intensity I0 and on ΔI size. Bigger ΔI values will speed up the threshold finding at the cost of reducing the precision of the threshold. Furthermore, we can start with I0 values close to the last perception thresholds to improve processing speed.
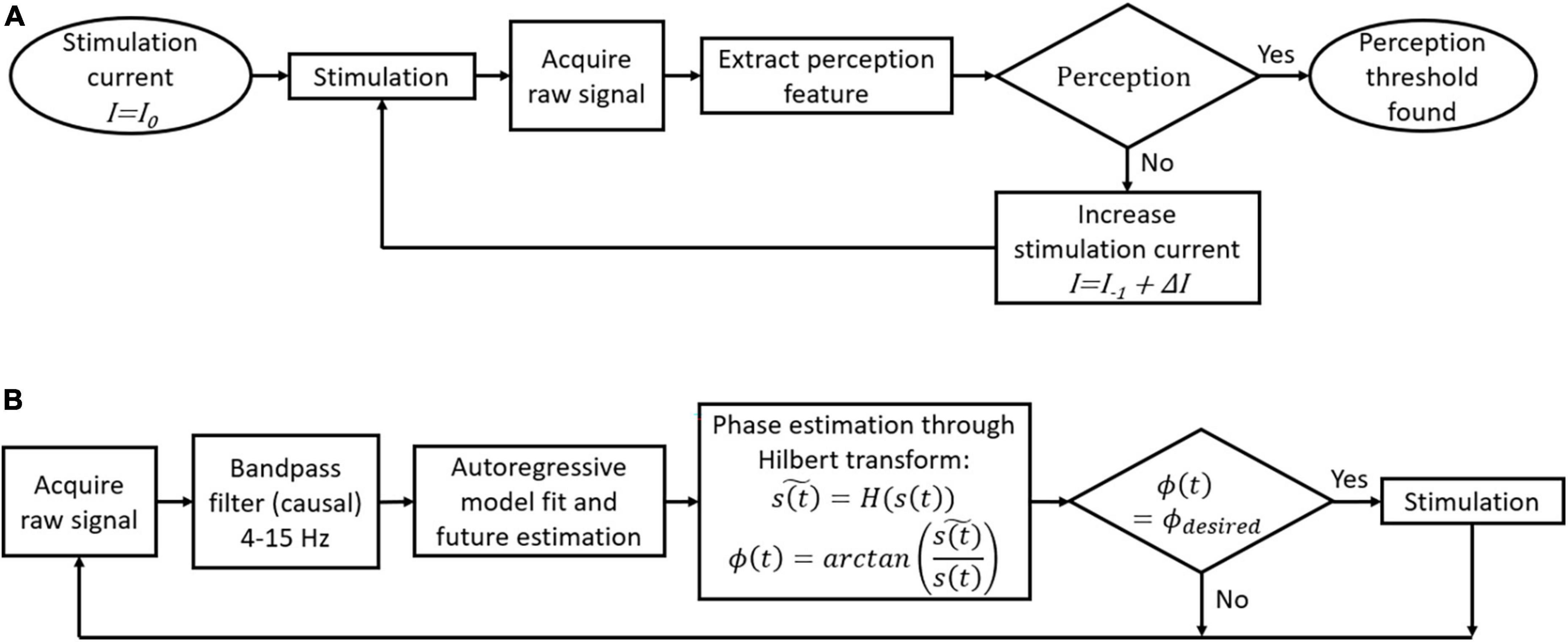
Figure 2. Flowcharts of possible closed-loop algorithms for a cortical visual prosthesis. (A) Automatic threshold adjustment for perception. (B) Local field potentials (LFP)-phase locked stimulation.
To stimulate the desired LFP phase in real time, we can use the approach proposed by Blackwood et al. (2018). First, we have to record 1-second windows of the raw signal and then filter this signal between 4 and 15 Hz. As the phase estimation of the last point in the window data is not accurate without knowing the future behavior of the signal, an autoregressive model is fitted to estimate the future trend. The Hilbert transform is then used to estimate the current phase, and a stimulation train is sent only if the current phase is the desired one (Figure 2B). We have recently tested this algorithm using intracortical signals from the visual cortex of a human blind volunteer at a sampling rate of 30 kHz and we obtained an error of ±20° in the LFP phase estimation (Grani et al., 2022b).
Discussion
Research on real-time closed-loop neural systems has built upon contributions from neuroscientists, engineers and clinicians, and may prove essential for future cortical visual prostheses, especially when high-number microelectrodes are used. As a result, the next frontier in cortical visual prosthesis may be the development of bidirectional implantable systems with enhanced abilities to modulate and manipulate populations of neurons in real-time. These closed-loop approaches could be able to use information from neural recordings to adjust the optimal stimulation, reduce charge requirements, and improve stimulation performance. However, there are still a number of important issues and challenges to overcome. For example, the stability of signals over time, the influence of movements on signal quality, and the higher energy consumption needed to perform closed-loop stimulation.
Although closed-loop stimulation might increase the safety, performance and usability of cortical visual prostheses, many questions need to be solved before it can be implemented in clinical devices able to continuously record and stimulate from hundreds of electrodes. For instance, the battery of the system needs to last at least for an entire day but adding a real-time brain signal analysis processor to the device would increase the energy consumption. This represents a significant challenge as the sampling frequency often used to get reliable neural signals is 30 kHz. Moreover, in order not to add complexity to the whole device, many signals should be excluded from the closed-loop approach. For example, perception could be inferred from EEG signals in the occipital cortex (Gail et al., 2004), but adding a standard EEG cap to the prosthesis will decrease the overall wearability, and the users might not want to use it on a daily basis.
Intracortical signals captured from the same electrodes used to stimulate cortical areas are the best candidates to build these closed-loop stimulation approaches. However, the signals collected during electrical stimulation are usually corrupted by the stimulation artifacts. Different signal processing techniques and electronic front-end designs can be used to retrieve the signals from stimulation artifacts (Wagenaar and Potter, 2002; Zhou et al., 2018) but these techniques do not work when the amplifiers are saturated. If this is the case, the blanking or exclusion of data during stimulation could be a good option. In addition, a discrimination between perception features and artifacts could be possible assuming that the artifact features increase linearly with the current intensity, while features linked to perception should have a different behavior, starting to increase only after the perception threshold.
On the other hand, as the microelectrodes have to be permanently implanted in the user’s brain, it is important that the signals on which closed-loop stimulation is based are stable over time. Some studies show that the number of reliable spikes captured by intracortical electrodes decreases with time (Sharma et al., 2015), while LFPs are more stable (Grani et al., 2022a). Therefore, closed-loop approaches based on LFP recordings could be more stable over time than approaches based on single neuron spikes and become the basis for future closed-loop approaches.
Datasets using current intensities able to induce perception 50% of the time, could be of great help to get better insight into these issues and help to investigate in which brain state it is easier to induce visual perceptions. Ideally, the LFP phase before stimulation could be significantly different in case of perception and no perception as it is shown in Figure 1E. However, we do not yet know if the same phase of the LFP is valid for all the electrodes. Thus, the neural population around each electrode could determine the preferred LFP phase, which means that to modulate the neural response to stimulation, perhaps we should consider the specific dynamics of every single electrode. In addition, the real-time detection of this parameter can also be associated with uncertainties that reduce the accuracy of phase estimation. Further, although different algorithms have been proposed for continuous phase estimation in real-time (Kim et al., 2016; Blackwood et al., 2018), this locked phase stimulation can also limit the time resolution of the stimulation. Thus, it seems that the frequency of ongoing oscillations is around 10 Hz (Dugue et al., 2011), which means that the preferred phase should appear approximately every 100 ms, limiting to this time the refresh rate of the visual prosthesis. Therefore, all these results must be confirmed in real-life environments, and there is still not enough information about the period of the local field potential that corresponds to maximum excitability nor about how many feedback channels can be reliably provided in parallel.
Another complementary and not mutually exclusive approach could be to reproduce the responses of cortical neurons to different visual stimuli (Guo et al., 2018; Spencer et al., 2019; Shah and Chichilnisky, 2020). Sighted animal models with intracortical electrodes in the visual cortex could be used to obtain visual cortex responses to different visual patterns. Then, knowing the neural activity elicited by a visual stimulus and the neural activity elicited by each electrical stimulation parameter, the stimulation parameters could be shaped to obtain the desired neural activity for a certain visual perception. However, there is no guarantee that eliciting with electrical stimulation the same activity of a natural image in V1 creates the same image perception. In addition, we have to consider that the perception experience is modulated by higher cortical areas (van Vugt et al., 2018) and could also be different in a brain deprived of visual information (Merabet et al., 2007; Fernandez, 2018).
All the progress in neural technologies, neuroscience, electronics, and bioengineering together with increased intelligence in neural systems can help to foster the development of improved custom-tailored devices, which will incorporate advanced closed-loop algorithms for restoring some functional sight to blind people. Therefore, we expect that in the future, closed-loop stimulation will offer more safety, precision, and personalization of cortical visual neuroprostheses approaches.
Data availability statement
The original contributions presented in this study are included in the article/supplementary material, further inquiries can be directed to the corresponding author.
Ethics statement
The studies involving human participants were reviewed and approved by Hospital General Universitario de Elche Clinical Research Committee. The patients/participants provided their written informed consent to participate in this study.
Author contributions
FG, CS-S, AF, and EF contributed to the design, implementation of the research, and writing of the manuscript. All authors contributed to the article and approved the submitted version.
Funding
Funding was provided by grant PROMETEO/2019/119 from the Generalitat Valenciana (Spain), by the European Union’s Horizon 2020 Research and Innovation Programme under grant agreement No. 899287 (project NeuraViPer) and under the Marie Skłodowska-Curie grant agreement No. 861423 (enTRAIN Vision), and by Ministerio de Ciencia e Innovación (Spain) by grant PDC2022-133952-I00.
Conflict of interest
The authors declare that the research was conducted in the absence of any commercial or financial relationships that could be construed as a potential conflict of interest.
Publisher’s note
All claims expressed in this article are solely those of the authors and do not necessarily represent those of their affiliated organizations, or those of the publisher, the editors and the reviewers. Any product that may be evaluated in this article, or claim that may be made by its manufacturer, is not guaranteed or endorsed by the publisher.
References
Allison-Walker, T. J., Ann Hagan, M., Chiang Price, N. S., and Tat Wong, Y. (2020). Local field potential phase modulates neural responses to intracortical electrical stimulation. Annu. Int. Conf. IEEE Eng. Med. Biol. Soc. 2020, 3521–3524. doi: 10.1109/EMBC44109.2020.9176186
Beauchamp, M. S., Oswalt, D., Sun, P., Foster, B. L., Magnotti, J. F., Niketeghad, S., et al. (2020). Dynamic stimulation of visual cortex produces form vision in sighted and blind humans. Cell 181, 774.e5–783.e5. doi: 10.1016/j.cell.2020.04.033
Blackwood, E., Lo, M. C., and Alik Widge, S. (2018). Continuous phase estimation for phase-locked neural stimulation using an autoregressive model for signal prediction. Annu. Int. Conf. IEEE Eng. Med. Biol. Soc. 2018, 4736–4739. doi: 10.1109/EMBC.2018.8513232
Borda, E., Gaillet, V., Airaghi Leccardi, M. J. I., Zollinger, E. G., Moreira, R. C., and Ghezzi, D. (2022). Three-dimensional multilayer concentric bipolar electrodes restrict spatial activation in optic nerve stimulation. J. Neural Eng. 19:036016. doi: 10.1088/1741-2552/ac6d7e
Borg, E., and Zakrisson, J. E. (1973). Letter: stapedius reflex and speech features. J. Acoust. Soc. Am. 54, 525–527. doi: 10.1121/1.1913610
Bosking, W. H., Oswalt, D. N., Foster, B. L., Sun, P., Beauchamp, M. S., and Yoshor, D. (2022). Percepts evoked by multi-electrode stimulation of human visual cortex. Brain Stimul. 15, 1163–1177. doi: 10.1016/j.brs.2022.08.007
Bourne, R. R. A., Flaxman, S. R., Braithwaite, T., Cicinelli, M. V., Das, A., Jonas, J. B., et al. (2017). Magnitude, temporal trends, and projections of the global prevalence of blindness and distance and near vision impairment: a systematic review and meta-analysis. Lancet Glob. Health 5, e888–e897. doi: 10.1016/S2214-109X(17)30293-0
Brackbill, N., Rhoades, C., Kling, A., Shah, N. P., Sher, A., Litke, A. M., et al. (2020). Reconstruction of natural images from responses of primate retinal ganglion cells. eLife 9:e58516. doi: 10.7554/eLife.58516
Cagnan, H., Pedrosa, D., Little, S., Pogosyan, A., Cheeran, B., Aziz, T., et al. (2017). Stimulating at the right time: phase-specific deep brain stimulation. Brain 140, 132–145. doi: 10.1093/brain/aww286
Cha, K., Horch, K. W., and Normann, R. A. (1992). Mobility performance with a pixelized vision system. Vision Res. 32, 1367–1372.
Chen, R., Canales, A., and Anikeeva, P. (2017). Neural recording and modulation technologies. Nat. Rev. Mater. 2:16093. doi: 10.1038/natrevmats.2016.93
Chen, X., Wang, F., Fernandez, E., and Roelfsema, P. R. (2020). Shape perception via a high-channel-count neuroprosthesis in monkey visual cortex. Science 370, 1191–1196. doi: 10.1126/science.abd7435
De Silva, S. R., and Moore, A. T. (2022). Optogenetic approaches to therapy for inherited retinal degenerations. J. Physiol. 600, 4623–4632. doi: 10.1113/JP282076
Dugue, L., Marque, P., and VanRullen, R. (2011). The phase of ongoing oscillations mediates the causal relation between brain excitation and visual perception. J. Neurosci. 31, 11889–11893. doi: 10.1523/JNEUROSCI.1161-11.2011
Farkhondeh Tale Navi, F., Heysieattalab, S., Ramanathan, D. S., Raoufy, M. R., and Nazari, M. A. (2022). Closed-loop modulation of the self-regulating brain: a review on approaches, emerging paradigms, and experimental designs. Neuroscience 483, 104–126. doi: 10.1016/j.neuroscience.2021.12.004
Fernandez, E. (2018). Development of visual Neuroprostheses: trends and challenges. Bioelectron. Med. 4:12. doi: 10.1186/s42234-018-0013-8
Fernandez, E. (2022). Selective induction of fingertip sensations for better neuroprosthetic control. Neurology 98, 261–262. doi: 10.1212/WNL.0000000000013177
Fernandez, E., Alfaro, A., Soto-Sanchez, C., Gonzalez-Lopez, P., Lozano, A. M., Pena, S., et al. (2021). Visual percepts evoked with an intracortical 96-channel microelectrode array inserted in human occipital cortex. J. Clin. Invest. 131:e151331. doi: 10.1172/JCI151331
Fernandez, E., Alfaro-Saez, A., and Gonzalez-Lopez, P. (2020). Toward long-term communication with the brain in the blind by intracortical stimulation: challenges and future prospects. Front. Neurosci. 11:681. doi: 10.3389/fnins.2020.00681
Fifer, M. S., McMullen, D. P., Osborn, L. E., Thomas, T. M., Christie, B., Nickl, R. W., et al. (2022). Intracortical somatosensory stimulation to elicit fingertip sensations in an individual with spinal cord injury. Neurology 98, e679–e687. doi: 10.1212/WNL.0000000000013173
Figee, M., Riva-Posse, P., Choi, K. S., Bederson, L., Mayberg, H. S., and Kopell, B. H. (2022). Deep brain stimulation for depression. Neurotherapeutics 19, 1229–1245. doi: 10.1007/s13311-022-01270-3
Gail, A., Brinksmeyer, H. J., and Eckhorn, R. (2004). Perception-related modulations of local field potential power and coherence in primary visual cortex of awake monkey during binocular rivalry. Cereb. Cortex 14, 300–313. doi: 10.1093/cercor/bhg129
Gaillet, V., Cutrone, A., Artoni, F., Vagni, P., Mega Pratiwi, A., Romero, S. A., et al. (2020). Spatially selective activation of the visual cortex via intraneural stimulation of the optic nerve. Nat. Biomed. Eng. 4, 181–194. doi: 10.1038/s41551-019-0446-8
Grani, F., Soto-Sanchez, C., Farfan, F. D., Alfaro, A., Grima, M. D., Rodil Doblado, A., et al. (2022a). Time stability and connectivity analysis with an intracortical 96-channel microelectrode array inserted in human visual cortex. J. Neural Eng. 19:045001. doi: 10.1088/1741-2552/ac801d
Grani, F., Soto-Sanchez, C., Rodil, A., Grima, M. D., Farfan, F., Calvo, M., et al. (2022b). “Performance evaluation of a real-time phase estimation algorithm applied to intracortical signals from human visual cortex,” in Artificial Intelligence in Neuroscience: Affective Analysis and Health Applications, eds J. M. Ferrández Vicente, J. R. Álvarez-Sánchez, F. de la Paz López, and H. Adeli (Cham: Springer), 516–525.
Guenser, G., Laudanski, J., Phillipon, B., Backus, B. C., Bordure, P., Romanet, P., et al. (2015). The relationship between electrical auditory brainstem responses and perceptual thresholds in Digisonic(R) SP cochlear implant users. Cochlear Implants Int. 16, 32–38. doi: 10.1179/1754762814Y.0000000082
Guo, T., Yang, C. Y., Tsai, D., Muralidharan, M., Suaning, G. J., Morley, J. W., et al. (2018). Closed-loop efficient searching of optimal electrical stimulation parameters for preferential excitation of retinal ganglion cells. Front. Neurosci. 12:168. doi: 10.3389/fnins.2018.00168
Higuchi, A., Kumar, S. S., Benelli, G., Alarfaj, A. A., Munusamy, M. A., Umezawa, A., et al. (2017). Stem cell therapies for reversing vision loss. Trends Biotechnol. 35, 1102–1117. doi: 10.1016/j.tibtech.2017.06.016
Hong, J. W., Yoon, C., Jo, K., Won, J. H., and Park, S. (2021). Recent advances in recording and modulation technologies for next-generation neural interfaces. iScience 24:103550. doi: 10.1016/j.isci.2021.103550
Khodagholy, D., Ferrero, J. J., Park, J., Zhao, Z., and Gelinas, J. N. (2022). Large-scale, closed-loop interrogation of neural circuits underlying cognition. Trends Neurosci. 45, 968–983. doi: 10.1016/j.tins.2022.10.003
Kim, L., Harer, J., Rangamani, A., Moran, J., Parks, P. D., Widge, A., et al. (2016). Predicting local field potentials with recurrent neural networks. Annu. Int. Conf. IEEE Eng. Med. Biol. Soc. 2016, 808–811. doi: 10.1109/EMBC.2016.7590824
Lee, S. W., Fallegger, F., Casse, B. D., and Fried, S. I. (2016). Implantable microcoils for intracortical magnetic stimulation. Sci. Adv. 2:e1600889. doi: 10.1126/sciadv.1600889
Li, Y., Tan, Z., Wang, J., Wang, M., and Wang, L. (2022). Neural substrates of external and internal visual sensations induced by human intracranial electrical stimulation. Front. Neurosci. 16:918767. doi: 10.3389/fnins.2022.918767
Little, S., Pogosyan, A., Neal, S., Zavala, B., Zrinzo, L., Hariz, M., et al. (2013). Adaptive deep brain stimulation in advanced Parkinson disease. Ann. Neurol. 74, 449–457. doi: 10.1002/ana.23951
Lozano, A. M., Lipsman, N., Bergman, H., Brown, P., Chabardes, S., Chang, J. W., et al. (2019). Deep brain stimulation: current challenges and future directions. Nat. Rev. Neurol. 15, 148–160. doi: 10.1038/s41582-018-0128-2
McKay, C. M., Chandan, K., Akhoun, I., Siciliano, C., and Kluk, K. (2013). Can ECAP measures be used for totally objective programming of cochlear implants? J. Assoc. Res. Otolaryngol. 14, 879–890. doi: 10.1007/s10162-013-0417-9
Mekhail, N., Levy, R. M., Deer, T. R., Kapural, L., Li, S., Amirdelfan, K., et al. (2020). Long-term safety and efficacy of closed-loop spinal cord stimulation to treat chronic back and leg pain (Evoke): a double-blind, randomised, controlled trial. Lancet Neurol. 19, 123–134. doi: 10.1016/S1474-4422(19)30414-4
Merabet, L. B., Rizzo, J. F. III, Pascual-Leone, A., and Fernandez, E. (2007). ‘Who is the ideal candidate?’: decisions and issues relating to visual neuroprosthesis development, patient testing and neuroplasticity. J. Neural Eng. 4, S130–S135. doi: 10.1088/1741-2560/4/1/S15
Nanegrungsunk, O., Au, A., Sarraf, D., and Sadda, S. R. (2022). New frontiers of retinal therapeutic intervention: a critical analysis of novel approaches. Ann. Med. 54, 1067–1080. doi: 10.1080/07853890.2022.2066169
Nguyen, H. T., Tangutooru, S. M., Rountree, C. M., Kantzos, A. J., Tarlochan, F., Yoon, W. J., et al. (2016). Thalamic visual prosthesis. IEEE Trans. Biomed. Eng. 63, 1573–1580. doi: 10.1109/TBME.2016.2567300
Nirenberg, S., and Pandarinath, C. (2012). Retinal prosthetic strategy with the capacity to restore normal vision. Proc. Natl. Acad. Sci. U.S.A. 109, 15012–15017. doi: 10.1073/pnas.1207035109
Nowik, K., Langwinska-Wosko, E., Skopinski, P., Nowik, K. E., and Szaflik, J. P. (2020). Bionic eye review - An update. J. Clin. Neurosci. 78, 8–19. doi: 10.1016/j.jocn.2020.05.041
Panikker, P., Roy, S., Ghosh, A., Poornachandra, B., and Ghosh, A. (2022). Advancing precision medicines for ocular disorders: diagnostic genomics to tailored therapies. Front. Med. 9:906482. doi: 10.3389/fmed.2022.906482
Picaud, S., and Sahel, J. A. (2020). [Vision restoration: science fiction or reality?]. Med. Sci. 36, 1038–1044. doi: 10.1051/medsci/2020213
Price, B. H., and Gavornik, J. P. (2022). Efficient temporal coding in the early visual system: existing evidence and future directions. Front. Comput. Neurosci. 16:929348. doi: 10.3389/fncom.2022.929348
Qiao, K., Chen, J., Wang, L., Zhang, C., Zeng, L., Tong, L., et al. (2019). Category decoding of visual stimuli from human brain activity using a bidirectional recurrent neural network to simulate bidirectional information flows in human visual cortices. Front. Neurosci. 13:692. doi: 10.3389/fnins.2019.00692
Ranjandish, R., and Schmid, A. (2020). A review of microelectronic systems and circuit techniques for electrical neural recording aimed at closed-loop epilepsy control. Sensors 20:5716. doi: 10.3390/s20195716
Rassia, K. E. K., Moutoussis, K., and Pezaris, J. S. (2022). Reading text works better than watching videos to improve acuity in a simulation of artificial vision. Sci. Rep. 12:12953. doi: 10.1038/s41598-022-10719-6
Rotermund, D., Ernst, U. A., and Pawelzik, K. R. (2019). Open Hardware for neuro-prosthesis research: a study about a closed-loop multi-channel system for electrical surface stimulations and measurements. HardwareX 6:e00078. doi: 10.1016/j.ohx.2019.e00078
Scangos, K. W., Khambhati, A. N., Daly, P. M., Makhoul, G. S., Sugrue, L. P., Zamanian, H., et al. (2021). Closed-loop neuromodulation in an individual with treatment-resistant depression. Nat Med. 27, 1696–1700. doi: 10.1038/s41591-021-01480-w
Shah, N. P., and Chichilnisky, E. J. (2020). Computational challenges and opportunities for a bi-directional artificial retina. J. Neural Eng. 17:055002. doi: 10.1088/1741-2552/aba8b1
Sharma, G., Anneta, N., Fridenberg, D., Blanco, T., Vasconcelos, D., Shaikhouni, A., et al. (2015). Time stability and coherence analysis of multiunit, single-unit and local field potential neuronal signals in chronically implanted brain electrodes. Bioelectron. Med. 2, 63–71. doi: 10.15424/bioelectronmed.2015.00010
Spencer, M. J., Kameneva, T., Grayden, D. B., Meffin, H., and Burkitt, A. N. (2019). Global activity shaping strategies for a retinal implant. J. Neural Eng. 16:026008. doi: 10.1088/1741-2552/aaf071
Stevenson, I. H., and Kording, K. P. (2011). How advances in neural recording affect data analysis. Nat. Neurosci. 14, 139–142. doi: 10.1038/nn.2731
Van Gelder, R. N., Chiang, M. F., Dyer, M. A., Greenwell, T. N., Levin, L. A., Wong, R. O., et al. (2022). Regenerative and restorative medicine for eye disease. Nat. Med. 28, 1149–1156. doi: 10.1038/s41591-022-01862-8
van Vugt, B., Dagnino, B., Vartak, D., Safaai, H., Panzeri, S., Dehaene, S., et al. (2018). The threshold for conscious report: signal loss and response bias in visual and frontal cortex. Science 360, 537–542. doi: 10.1126/science.aar7186
Visram, A. S., Innes-Brown, H., El-Deredy, W., and McKay, C. M. (2015). Cortical auditory evoked potentials as an objective measure of behavioral thresholds in cochlear implant users. Hear. Res. 327, 35–42.
Vissani, M., Nanda, P., Bush, A., Neudorfer, C., Dougherty, D., and Richardson, R. M. (2022). Toward closed-loop intracranial neurostimulation in obsessive-compulsive disorder. Biol. Psychiatry 16:S0006-3223(22)01432-9. doi: 10.1016/j.biopsych.2022.07.003
Visser-Vandewalle, V., Andrade, P., Mosley, P. E., Greenberg, B. D., Schuurman, R., McLaughlin, N. C., et al. (2022). Deep brain stimulation for obsessive-compulsive disorder: a crisis of access. Nat. Med. 28, 1529–1532. doi: 10.1038/s41591-022-01879-z
Wagenaar, D. A., and Potter, S. M. (2002). Real-time multi-channel stimulus artifact suppression by local curve fitting. J. Neurosci. Methods 120, 113–120. doi: 10.1016/s0165-0270(02)00149-8
Weiss, N. M., Ovari, A., Oberhoffner, T., Demaret, L., Bicer, A., Schraven, S., et al. (2021). Automated detection of electrically evoked stapedius reflexes (eSR) during cochlear implantation. Eur. Arch. Otorhinolaryngol. 278, 1773–1779. doi: 10.1007/s00405-020-06226-x
Wenger, N., Moraud, E. M., Raspopovic, S., Bonizzato, M., DiGiovanna, J., Musienko, P., et al. (2014). Closed-loop neuromodulation of spinal sensorimotor circuits controls refined locomotion after complete spinal cord injury. Sci. Transl. Med. 6:255ra133. doi: 10.1126/scitranslmed.3008325
Xu, J., Guo, H., Nguyen, A. T., Lim, H., and Yang, Z. (2018). A bidirectional neuromodulation technology for nerve recording and stimulation. Micromachines 9:538. doi: 10.3390/mi9110538
Keywords: closed-loop stimulation, visual prostheses, neural interfaces, brain stimulation, local field potentials
Citation: Grani F, Soto-Sánchez C, Fimia A and Fernández E (2022) Toward a personalized closed-loop stimulation of the visual cortex: Advances and challenges. Front. Cell. Neurosci. 16:1034270. doi: 10.3389/fncel.2022.1034270
Received: 01 September 2022; Accepted: 24 November 2022;
Published: 13 December 2022.
Edited by:
Maesoon Im, Korea Institute of Science and Technology (KIST), South KoreaReviewed by:
Mohit Naresh Shivdasani, University of New South Wales, AustraliaCopyright © 2022 Grani, Soto-Sánchez, Fimia and Fernández. This is an open-access article distributed under the terms of the Creative Commons Attribution License (CC BY). The use, distribution or reproduction in other forums is permitted, provided the original author(s) and the copyright owner(s) are credited and that the original publication in this journal is cited, in accordance with accepted academic practice. No use, distribution or reproduction is permitted which does not comply with these terms.
*Correspondence: Eduardo Fernández, ZS5mZXJuYW5kZXpAdW1oLmVz
†ORCID: Antonio Fimia, orcid.org/0000-0002-0609-0567