- 1Department of Neurology, Cerebrovascular Diseases Research Institute, Xuanwu Hospital of Capital Medical University, Beijing, China
- 2Department of Pharmaceutical Sciences, University of New Mexico Health Sciences Center, Albuquerque, NM, United States
Hemorrhagic transformation (HT), which occurs with or without reperfusion treatments (thrombolysis and/or thrombectomy), deteriorates the outcomes of ischemic stroke patients. It is essential to find clinically reliable biomarkers that can predict HT. In this study, we screened for potential serum biomarkers from an existing blood bank and database with 243 suspected acute ischemic stroke (AIS) patients. A total of 37 patients were enrolled, who were diagnosed as AIS without receiving reperfusion treatment. They were divided into two groups based on whether they were accompanied with HT or not (five HT and 32 non-HT). Serum samples were labeled by isobaric tags for relative and absolute quantitation (iTRAQ) and analyzed by liquid chromatography coupled with tandem mass spectrometry (LC-MS/MS) and compared under NCBInr database. A total of 647 proteins in sera samples were captured, and the levels of 17 proteins (12 upregulated and five downregulated) were significantly different. These differentially expressed proteins were further categorized with Gene Ontology functional classification annotation and Kyoto Encyclopedia of Genes and Genomes metabolic pathway analysis into biological processes. Further protein–protein interaction analysis using String database discovered that, among the differentially expressed proteins, 10 pairs of proteins were found to have crosstalk connections, which may have direct (physical) and indirect (functional) interactions for the development of HT. Our findings suggest that these differentially expressed proteins could serve as potential biomarkers for predicting HT after ischemic stroke.
Introduction
Ischemic stroke is a leading cause of death in the world. The severe complications, especially hemorrhagic transformation (HT), deteriorate the clinical condition and lead to exacerbated brain damages. HT may occur naturally or as a result of recanalization treatment (thrombolysis and/or thrombectomy). Since therapeutic interventions used during the acute phase of ischemic stroke (AIS) could significantly increase the risk of developing HT, it is essential and urgent to find biomarkers for predicting HT before recanalization treatment. Blood-based biomarker would be particularly useful, as blood can be easily obtained in emergency room. Several serum markers involving various mechanisms have been reported, such as matrix metalloproteinases (MMPs), S100 calcium-binding protein B (S-100B), cellular fibronectin (cFn), and neuron-specific enolase (NSE; Shirasaki et al., 2004; Castellanos et al., 2007; Zaheer et al., 2013; Zhong et al., 2017). However, so far, none of these serum markers is specific enough to be used in clinic to predict HT.
It was well recognized that an abnormally permeable blood–brain barrier (BBB) resulting from ischemia of the capillary endothelium allows the extravasation of blood products (Álvarez-Sabín et al., 2013). Our recent experimental study in rats showed that occludin, one of the tight junction proteins in BBB, can be cleaved into fragments after cerebral ischemia and then released into blood circulation. The level of blood occludin fragments was correlated with BBB permeability, serving as a biomarker for BBB damage (Pan et al., 2017). Our recent study in AIS patients showed that the blood occludin was significantly elevated in the early period of acute stroke (Li et al., 2020). However, the value of area under the receiver operating characteristic (ROC) curve (AUC) was less than 0.8 (0.73 in recanalization treatment and 0.77 in non-recanalization patients), suggesting that, without further improvement in occludin fragment detection specificity, blood occludin alone is not sufficient enough as a single biomarker for predicting HT. In this study, we screened for other potential serum biomarkers in serum samples from AIS patients using the isobaric tags for relative and absolute quantitation (iTRAQ)-based quantitative serum proteomics analysis.
Materials and Methods
Source of Clinical Data and Serum Specimens
This retrospective study was approved by the Ethics Committee of Xuanwu Hospital of Capital Medical University. We obtained the clinical data and serum specimens from our previous study (Li et al., 2020). The established database includes 243 suspected AIS patients who were administered to the emergency room at Xuanwu Hospital from November 2018 to March 2019. The Stroke Diagnosis and Treatment Quality Control and Improvement Center in Beijing, Xuanwu Hospital of Capital Medical University, is a comprehensive stroke center [acute treatments available 24/7; median door-to-needle time (DNT) < 30 min].
Blood samples were collected as soon as patients arrived at the emergency room using 21G needle and coagulated for 4 h at room temperature in Vacuette tubes (without additives, anticoagulants, and coagulant-promoting ingredients), which were routine care for stroke patients at Xuanwu Hospital. Serum was collected at 3,000 rpm for 10 min. Specimen with hemolysis was discarded. The sera were stored at −80°C without freezing and thawing for further study.
Case Enrollment
In this study, we aim to analyze the differences of serum proteomics between HT and non-HT patients after ischemic stroke and to try to find potential biomarkers for predicting HT. HT occurrence in patients with reperfusion (thrombolysis and/or thrombectomy) could be caused by a variety of factors, including mechanical damages that vary greatly among different operators. As such, HT occurrence in patients without recanalization may better reflect the natural outcome of the disease. Therefore, for this initial study to investigate potential biomarkers, we focused on AIS patients without recanalization (n = 91, including five HT and 86 non-HT cases). The fundamental characteristics of enrolled 37 cases and total 91 confirmed ischemic stroke patients without reperfusion are shown in Table 1.
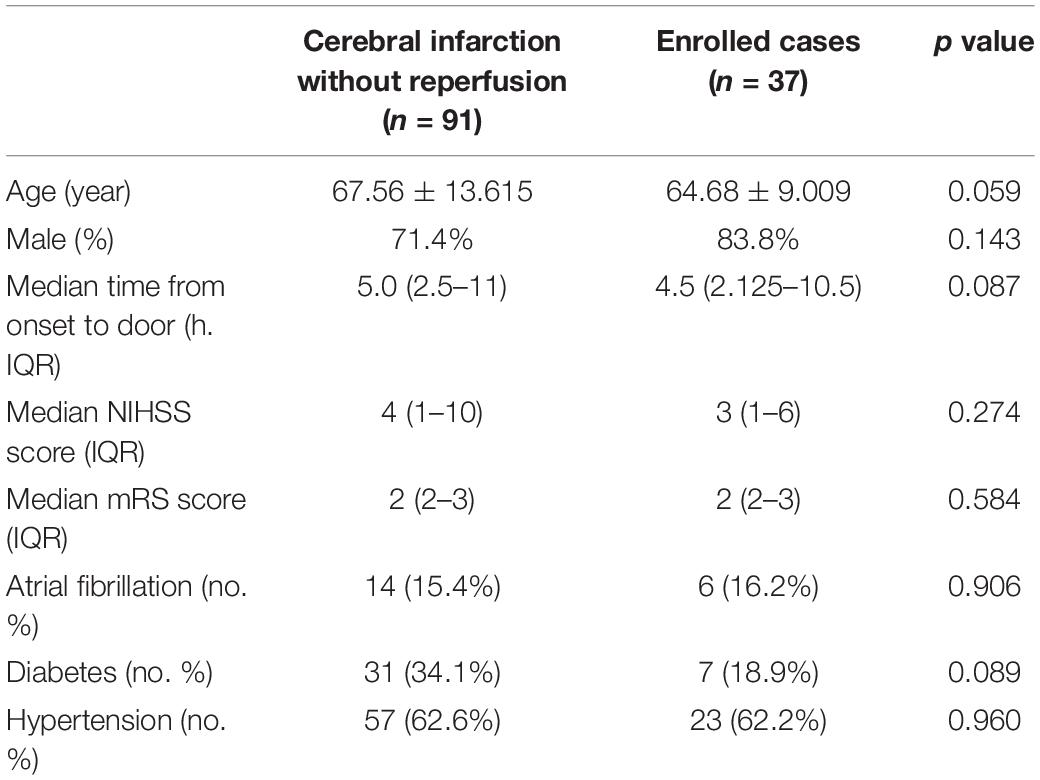
Table 1. Baseline characteristics of enrolled cases and cerebral infarction without reperfusion in database.
Our previous study showed that the serum occludin concentration was a little higher in the over 80 years’ group (Li et al., 2020), suggesting that the serum components of the elderly patients may be different from those of other age patients. The five HT patients were younger than 80 years. In order to better match the HT group, we excluded the cases >80 years from the non-HT group in this study.
A total of 37 cases (HT, n = 5; non-HT, n = 32) were finally enrolled for this study based on the inclusion and exclusion criteria below. The characteristics and related clinical information of the two groups are shown in Table 2. The flowchart of enrollment is shown in Figure 1. Inclusion criteria: (1) diagnosed as acute cerebral infarction by the Department of Emergency Neurology of Xuanwu Hospital and confirmed by computed tomography (CT) or MRI scan and (2) not receiving reperfusion treatments (thrombolysis and/or thrombectomy). Exclusion criteria: (1) age <18 or >80 years; (2) stroke onset >12 h or unclear; (3) complicating tumors and epilepsy; (4) hemolysis specimens; (5) incomplete information; and (6) available volume of serum <300 μl.
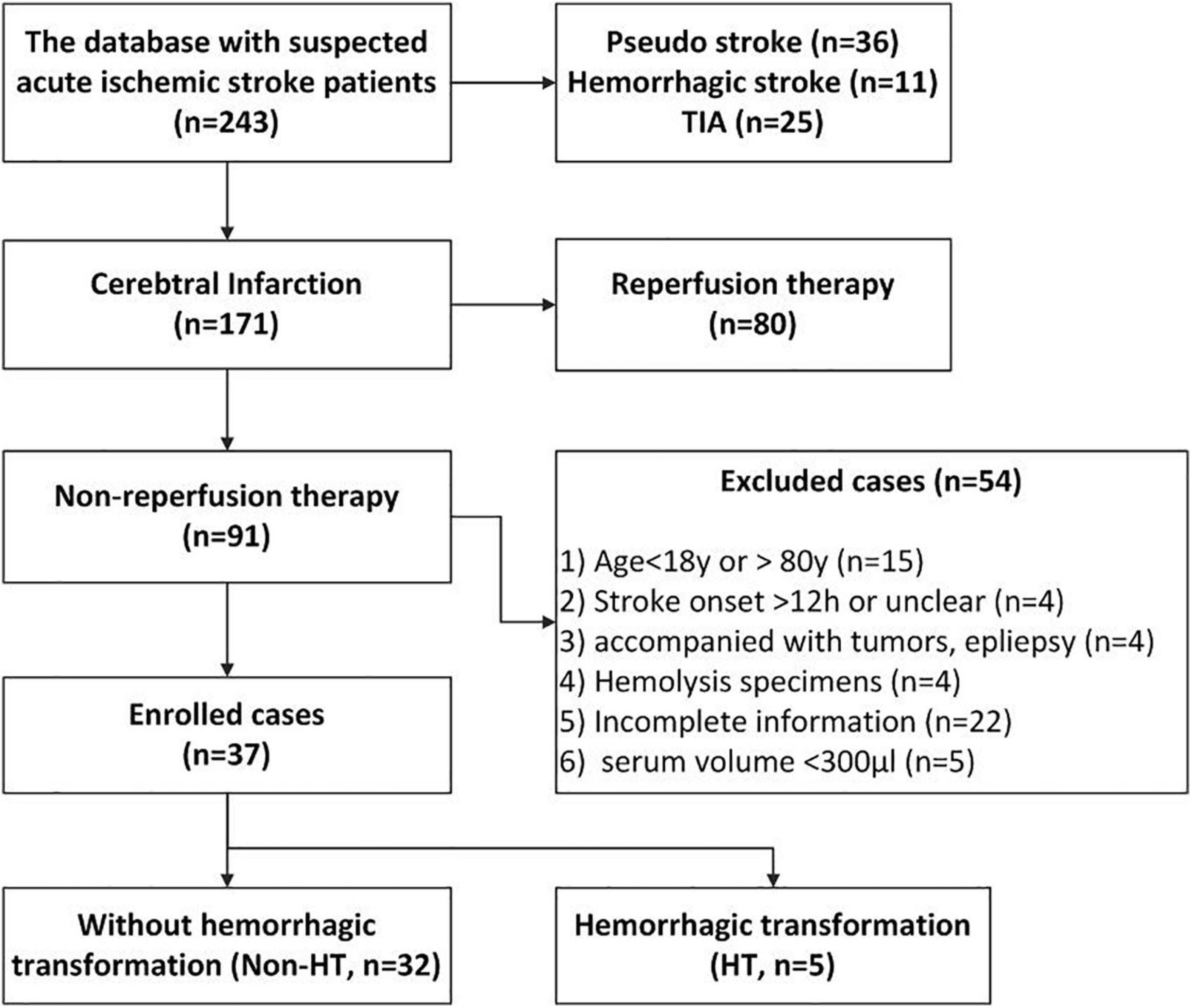
Figure 1. The flowchart of case enrollment. We obtained the clinical data and serum specimens from a database that was established in our previous study. This database includes 243 suspected acute ischemic stroke (AIS) patients who were administered at the emergency room at Xuanwu Hospital from November 2018 to March 2019. In this study, we enrolled the 37 AIS patients [hemorrhagic transformation (HT), n = 5; non-HT, n = 32] based on our inclusion and exclusion criteria.
Evaluation of Hemorrhagic Transformation and National Institutes of Health Stroke Scale Score
Intracranial HT was defined as secondary intracranial bleeding following cerebral infarction, occurring naturally or related to treatment. Intracranial HT was diagnosed by the Department of Neurology of Xuanwu Hospital and confirmed by CT or MRI images since stroke onset, according to the European Cooperative Acute Stroke Study (ECASS) III criteria (Hacke et al., 2008). Briefly, stroke patients received CT scan (first imaging) to distinguish ischemic stroke and hemorrhagic stroke as soon as they arrived at the hospital. For patients with ischemic stroke, they underwent MRI examination (second imaging) for evaluation of the degree of cerebral infarction. Those who were suspected of HT would have an additional CT scan to confirm the diagnosis of HT. CT scan (third imaging) would be repeated before discharge (5–7 days). In this study, five HT cases belonged to hemorrhagic infarction-2 (HI; n = 4) and parenchymal hematoma-2 (PH2, n = 1), respectively.
National Institutes of Health stroke scale (NIHSS) score was assessed by two neurologists in the Emergency Access Department to ensure the accuracy of the assessment. Stroke severity was assessed on the basis of NIHSS score upon hospital admission and was classified as mild (0–6), moderate (7–15), and severe (≥16).
Grouping and Serum Specimen Rearrangement
Based on HT occurrence or not within 72 h since stroke onset, the enrolled 37 cases were divided into two groups (HT, n = 5; non-HT, n = 32). Since iTRAQ method has eight different labels, it can label up to eight samples at the same time. Therefore, we matched the cases of the two groups according to the clinical information. Since the incidence of HT significantly increased in patients with severe neurological dysfunction (high NIHSS score) and long duration of ischemia (time from onset to door >6 h), the serum specimens were matched and mixed into eight samples (n = 4 in both groups), based on NIHSS score and stroke onset time (Seo et al., 2020). The sample rearrangement and matching are shown in Table 3.
Abundant Protein Depletion and Quality Testing
Abundant proteins in serum, including albumin, IgG, and 10 other high-abundance proteins that can make up more than 70% of total serum protein were removed according to the instruction of Pierce Top 12 Abundant Protein Depletion Spin Columns (Thermo Fisher Scientific, Waltham, MA, United States). Samples were concentrated with 3KD ultrafiltration centrifugal tube (Millipore, Billerica, MA, United States) and replaced using urea solution (8 M, containing protease inhibitor) for three times. Total protein concentration was determined using Pierce BCA protein assay kit (Thermo Fisher Scientific). Sodium dodecyl sulfate–polyacrylamide gel electrophoresis (SDS-PAGE) gel was stained using Coomassie blue of BiofurawTM Fast Protein Stain (Tanon, Shanghai, China) to make sure that the samples meet the requirement of iTRAQ analysis (diverse proteins without degradation).
Isobaric Tags for Relative and Absolute Quantitation Labeling and Reversed-Phase Chromatography
The sample labeling is shown in Table 3. The methods of sample digestion, iTRAQ labeling, and high pH ultra-performance liquid chromatography (UPLC) were described in previous studies (Wang et al., 2017). After reduction, alkylation, and trypsin digestion, the peptides were dried by vacuum and dissolved by 0.5 M of triethylammonium bicarbonate (TEAB). iTRAQ reagent (AB SCIEX, Redwood City, CA, United States; Lot. 4390812) was added to each tube and incubated at room temperature for 2 h. Equal amounts of labeled products were mixed in one tube and drained by vacuum concentrator. The peptides were dissolved in UPLC loading buffer (2% acetonitrile, adjusted to pH 10 with ammonia) and loaded on a reversed-phase C18 column (ACQUITY UPLC BEH C18 Column 1.7 μm, 2.1 mm × 150 mm, Waters Corporation, Milford, MA, United States) and fractionated by a ultra-high-performance LC (UHPLC) system (Thermo Scientific Vanquish Flex, Thermo Fisher Scientific, United States) at a flow rate of 200 μl/min. Then fractions were collected for LC–mass spectrometry (MS)/MS analysis.
Liquid Chromatography Coupled With Tandem Mass Spectrometry Analysis
The fractions were then separated by EASY-nLC 1200 (Thermo Fisher Scientific, United States) and connected to Q_Exactive HF-X (Thermo Fisher Scientific, United States). Briefly, the peptides were separated by a C18 column (75 μm × 25 cm, Thermo Fisher Scientific, United States) in a linear gradient, from 0.1% formic acid/2% acetonitrile to 0.1% formic acid/80% acetonitrile at 200 nl/min for 120 min. A single Orbitrap MS scan from 350 to 1,500 m/z at a resolution of 60,000 with AGC set at 3e6 was followed by up to 20 ms/ms scans at a resolution of 15,000 with AGC set at 5e4. The top 20 were selected for fragmentation per cycle with dynamic exclusion time of 20 s.
Protein Identification
The raw data were analyzed using ProteomeDiscovererTM Software 2.4 (Thermo Fisher Scientific, United States) and the NCBInr and SwissProt/UniProt sequence databases. A standard parameter set was used for the search, including Cys alkylation by iodoacetamide, dynamic modifications (oxidation, acetyl, iTRAQ8plex, Met-loss, Met-loss+Acetyl), Homo sapiens, and trypsin digestion [max. missed cleavage sites ≤2; false discovery rate (FDR) ≤ 0.01].
Quality Control, Statistics, and Bioinformatics Analysis
In this study, blinding was applied to collect serum, clinical data, and HPLC/MS and to conduct proteomics analysis to control any potential bias. Significant difference was calculated using t-test: p < 0.05 and (fold cutoff <0.83 down-expression or >1.20 up-expression). The differential proteins were classified and analyzed using following databases: Gene Ontology (GO) for functional annotation analysis,1 Kyoto Encyclopedia of Genes and Genomes (KEGG) mapping for protein signal enrichment analysis,2 and String database3 for searching known and predicted protein–protein interaction network.
Results
Basic Clinical Information
In this initial study, to investigate potential biomarkers, we focused on AIS patients without recanalization (n = 91, including five HT and 86 non-HT cases). Statistical analysis showed that there was no significant difference in fundamental factors between enrolled 37 cases and total 91 confirmed ischemic stroke patients without reperfusion (Table 1). According to HT occurrence, the enrolled 37 cases were divided into two groups (HT, n = 5; non-HT, n = 32). The baseline characteristics of HT and non-HT patients in enrolled cases are shown in Table 2.
In this study, all HT occurred within 48 h and was confirmed by the second imaging scans. No new bleeding was observed by CT scan before discharge. The time from onset to the three times of imaging are shown in Table 2.
Dual antiplatelet treatments (aspirin enteric-coated tablets and clopidogrel hydrogen sulfate tablets) after the cerebrovascular event are routine therapies for confirmed cerebral infarction patients in Xuanwu Hospital (Wang et al., 2013; Zaidat et al., 2015; Johnston et al., 2018). For those who suffered from cardiogenic embolism due to atrial fibrillation, anticoagulant therapies were given at different times post-stroke onset following the management guideline of atrial fibrillation from European Heart Association and European Heart Rate Society (Kirchhof et al., 2016; Blacquiere et al., 2017; Steffel et al., 2018). In this study, there are two cases with atrial fibrillation in the HT group. They were treated with dual or single antiplatelet treatments at the beginning of hospitalization. Antiplatelet treatments were stopped as soon as signs of bleeding were discovered. In the non-HT group, there were four patients with atrial fibrillation. Three of them (NIHSS < 7) accepted anticoagulant therapy at 3 days, while the other patient (NIHSS 7–15) did not receive anticoagulant therapies during hospitalization until discharge at 7 days. The applications of antiplatelet and anticoagulant within 48 h are shown in Table 2.
Except NIHSS score, there was no significant difference in other characteristics between the two groups. For further proteomics analysis, the serum specimens were matched based on NIHSS score and stroke onset time (Table 3), making the samples comparable between the two groups.
Screen Differentially Expressed Proteins in Serum
Isobaric tags for relative and absolute quantitation labeling and LC-MS/MS were used to screen differentially expressed proteins in serum between HT and non-HT samples, and a total of 647 proteins were quantified. According to the log2 of fold change (HT/non-HT) and p-value between the two groups, the identified proteins were exported as a volcano plot (Figure 2). It was found that 17 proteins were expressed significantly differently between the HT and non-HT groups (12 upregulated shown as red triangles and five downregulated as blue triangles), while others, which were represented as black dots, were not significantly differently expressed. The differentially expressed proteins in sera between HT and non-HT patients were shown in order of p-value (Table 4). The detailed proteomics data are available at the website https://www.ebi.ac.uk/pride.
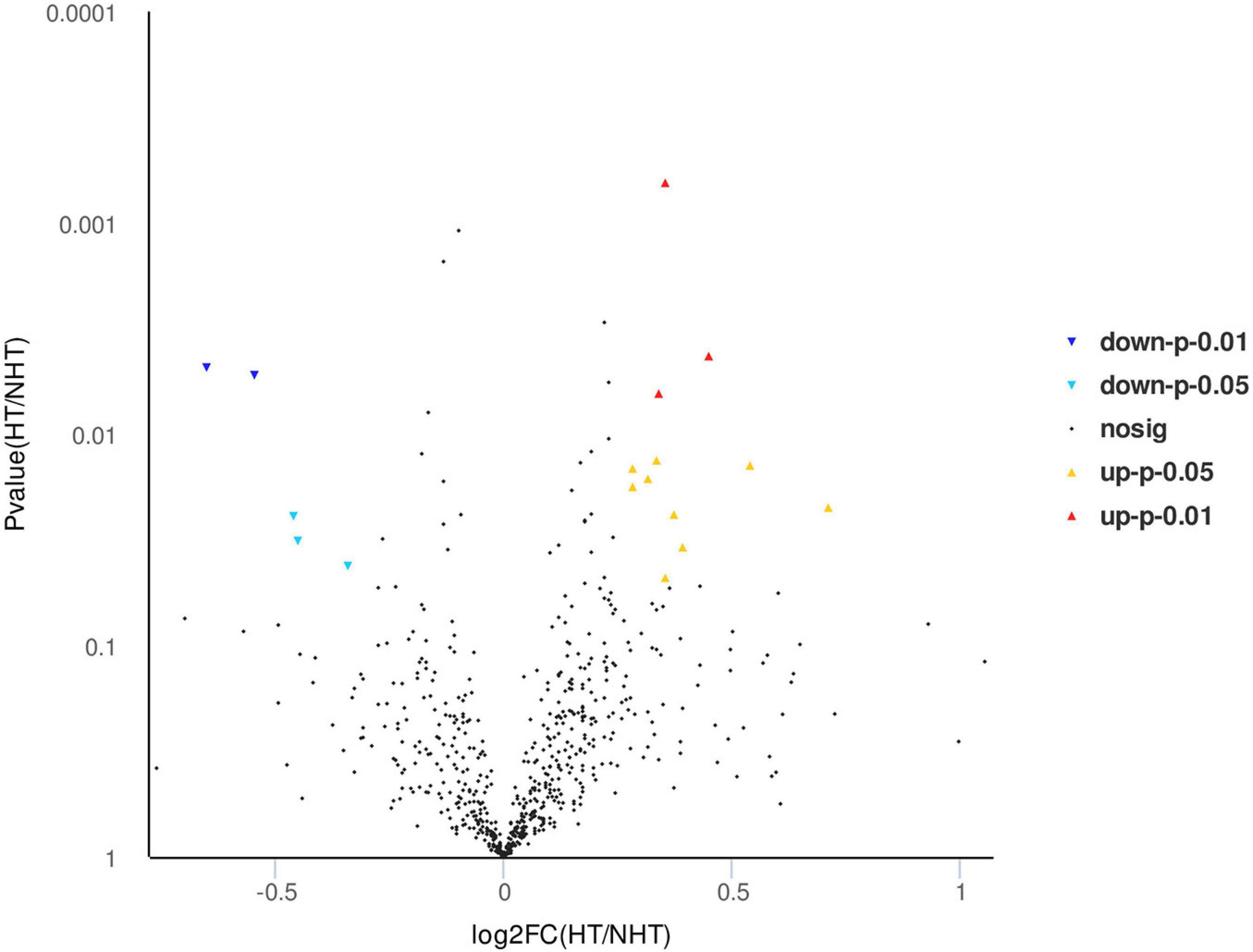
Figure 2. Volcano map of differential proteins between hemorrhagic transformation (HT) and non-HT groups. After isobaric tags for relative and absolute quantitation (iTRAQ) labeling and liquid chromatography coupled with tandem mass spectrometry (LC-MS/MS) identification, 17 differentially expressed proteins were quantified from a total of 647 proteins between HT and non-HT groups. The identified proteins were exported according to the log2 of fold change (HT/non-HT) and p-value. Yellow, upregulated under p < 0.05; red, upregulated under p < 0.01; light blue, downregulated under p < 0.05; blue, downregulated under p < 0.01; black, no significant difference proteins.
Functional Analysis of the Differentially Expressed Proteins
In order to gain global view of the functions of 17 differentially expressed proteins, the functional classification annotation and metabolic pathway analysis were performed using GO and KEGG databases, respectively.
Gene Ontology database can explain the functional enrichment of differential proteins and clarify the differences between samples at the functional level. GO enrichment analysis showed that the annotations of the differentially expressed proteins cover biological process, molecular function, and cellular component (Figure 3). The top five functions involve binding in molecular function (the number of proteins, 11; down, 4), cellular anatomical entity in cellular component (up, 9; down, 5), cellular process (up, 8; down, 5), biological regulation (up, 7; down, 4), and response to stimuli (up, 5; down, 3) in biological processes.
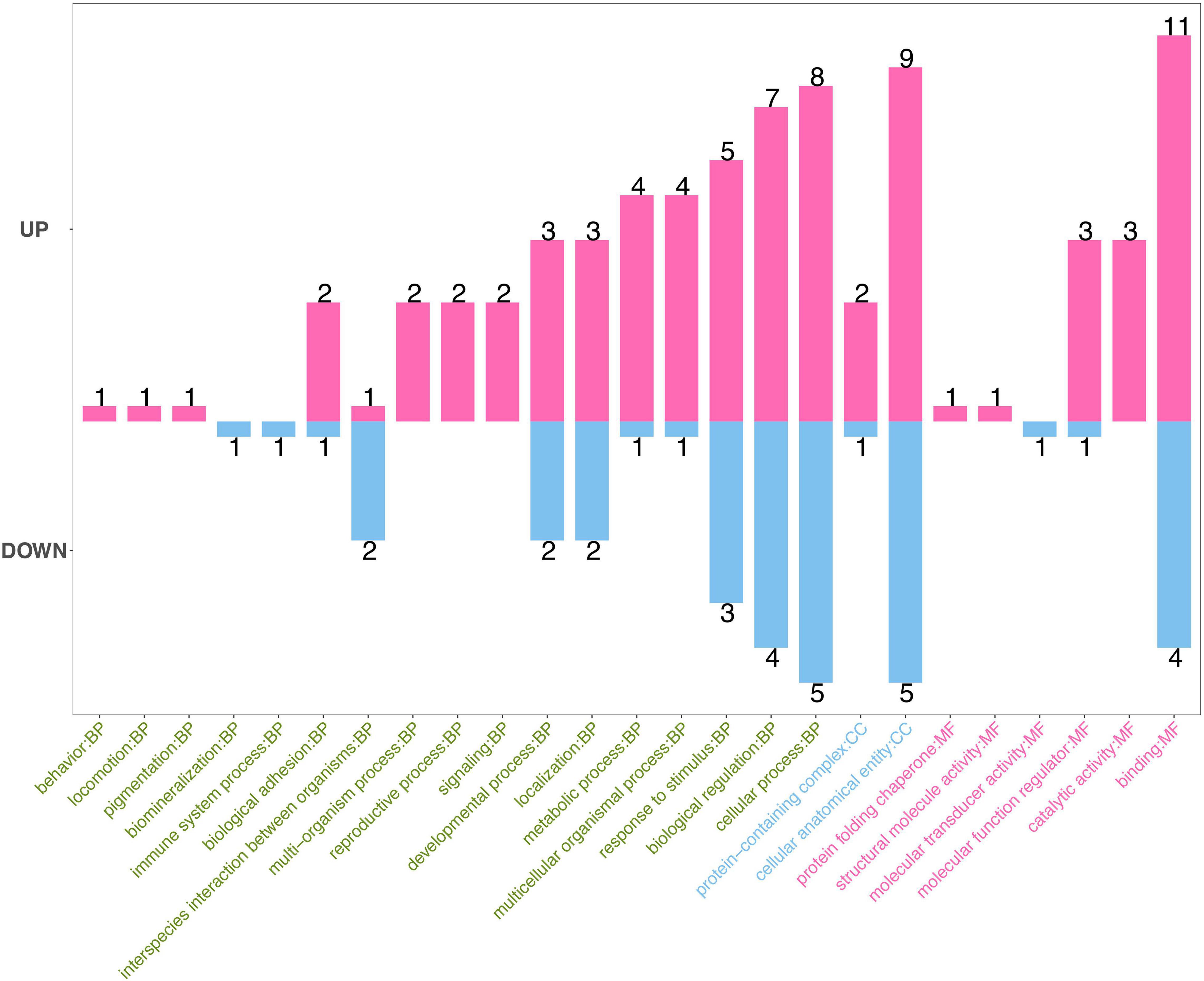
Figure 3. Gene Ontology (GO) enrichment analysis of differential proteins. GO enrichment analysis showed that the annotations of the differentially expressed proteins cover biological process (green), molecular function (red), and cellular component (blue). The numbers on the top of bars represent the number of annotations of the differentially expressed proteins.
The differentially expressed proteins were also classified based on KEGG database, which mainly focuses on whether a group of proteins appear at a certain function. Enrichment analysis by KEGG is developed from single protein annotation analysis to protein ensemble annotation analysis in order to improve the reliability of the study and identify the most relevant biological processes of abiotic phenomena (Figure 4). The x-axis represents the ratio of enrichment (Rich factor = Sample Number/Background Number). KEGG analysis showed that the top three pathways were extracellular matrix (ECM)–receptor interaction, nucleotide binding oligomerization domain-like receptor (NOD-like receptor) pathway, and peroxisome proliferator activated-receptor (PPAR) signaling pathway, suggesting that these pathways might be more likely related to or involved in HT after ischemic stroke.
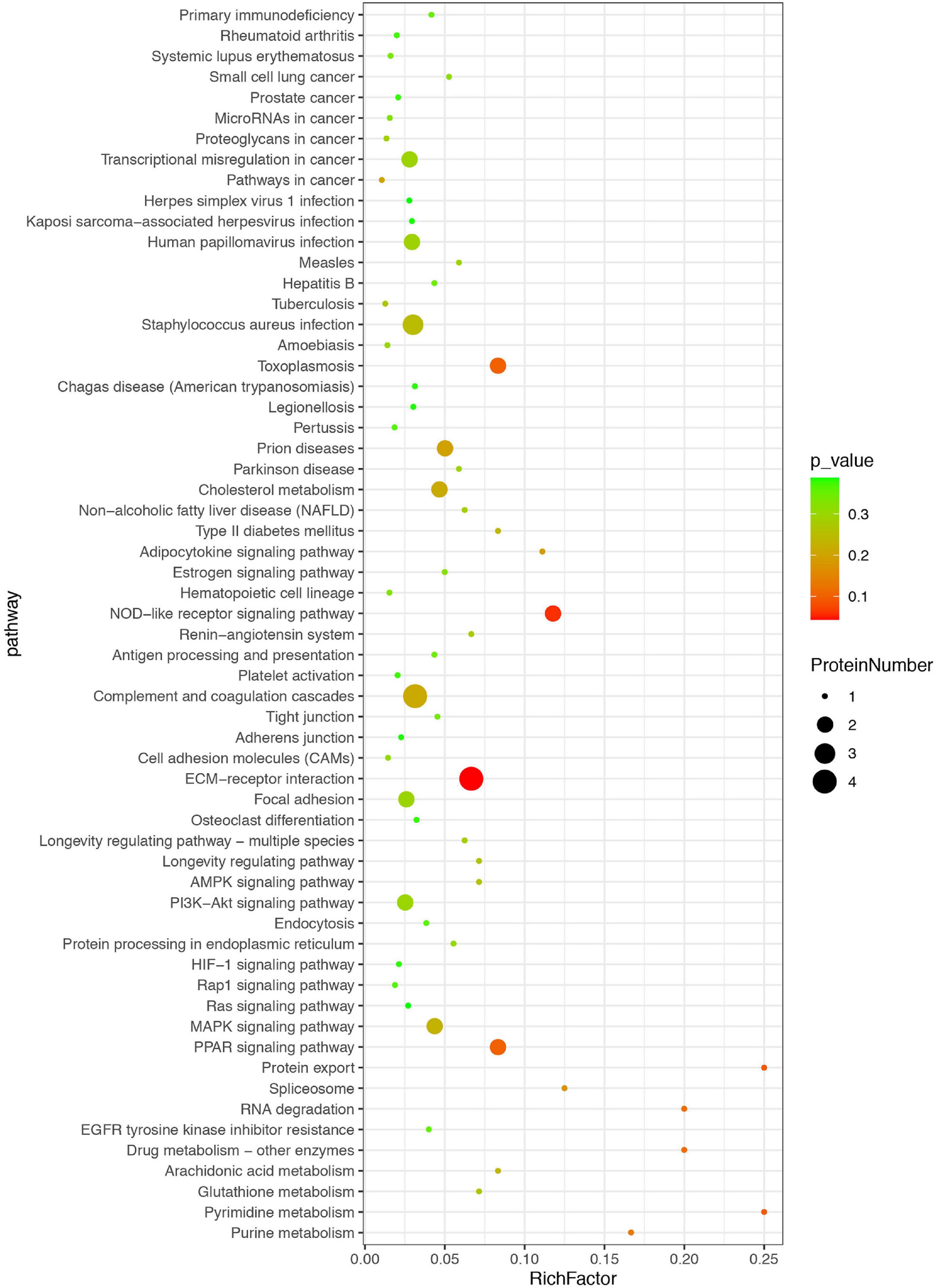
Figure 4. Kyoto Encyclopedia of Genes and Genomes (KEGG) enrichment analysis of differential proteins. The differentially expressed proteins were classified based on KEGG database. Each bubble represents one pathway, and the size of the bubbles reflects the protein number in each pathway. Bubble color represents the p-value of enrichment.
Interaction Network Analysis of Differentially Expressed Proteins
We further analyzed the interaction network of differentially expressed proteins using String database for protein–protein interactions, including direct physical interaction and indirect functional correlation between proteins (Table 5 and Figure 5). In addition to the experimental data, the results of text mining from PubMed abstracts and other database data, and bioinformatics methods are also used to predict the interactions. A comprehensive score (0–1) was obtained according to the weights given to different methods. Among the 17 differentially expressed proteins, we found 10 pairs of proteins that have crosstalk networks. The color of lines between proteins indicates the source of connection scores: co-expression (dark), experimentally determined interaction (blue), database annotated (pink), and automated text mining (green). These data suggested that they may have direct (physical) and indirect (functional) interactions for the development of HT. It is worth noting that there are two pairs of connections with high scores (≥0.7): ADIPOQ/SHBG (Adiponectin/Sex hormone-binding globulin, comprehensive score = 0.9); FBLN1/MFAP4 (Fibulin-1/Microfibril-associated glycoprotein 4, comprehensive score = 0.7). These data suggested that the two pairs of proteins might interact with each other to play comprehensive roles in the progress of HT after ischemia stroke.
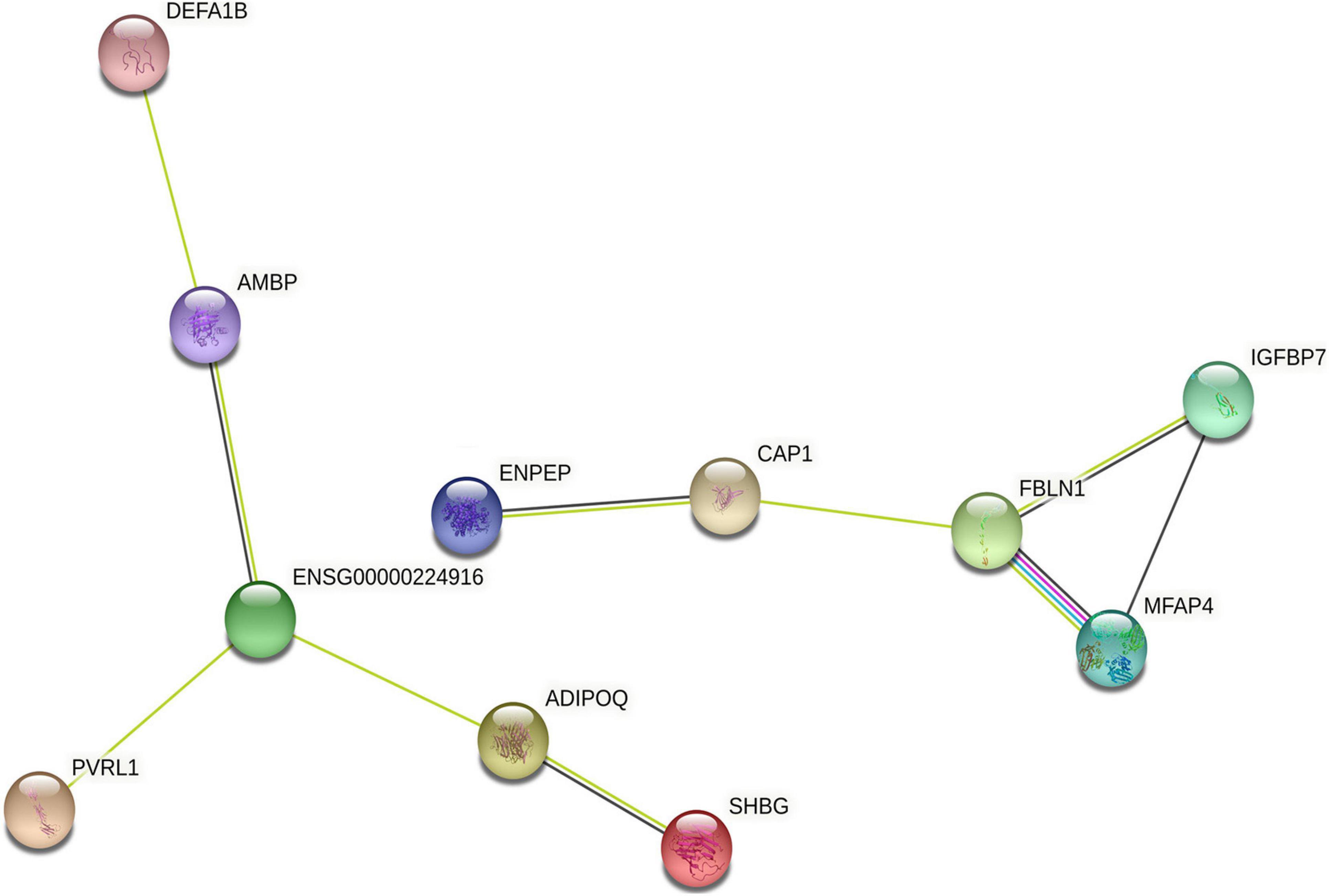
Figure 5. The interaction network of differentially expressed proteins. The interaction network of differentially expressed proteins was analyzed using String database. Each node represents one differential protein, and each connection reflects the interaction of proteins. The color of lines between proteins indicates the source of connection scores: co-expression (dark), experimentally determined interaction (blue), atabase annotated (pink), and automated text mining (green). The full names of the proteins are shown in Table 5.
Discussion
This is the first serum proteomics study focusing on differences between HT and non-HT patients after AIS. In this study, we screened a blood bank with 243 suspected AIS cases, which was established in our previous study, and enrolled 37 AIS patients (five HT and 32 non-HT). With the use of iTRAQ-based quantitative serum proteomics analysis, 17 proteins (12 upregulated and five downregulated) were found to be significantly different between HT and non-HT patients.
HT was defined as intracranial hemorrhage following cerebral infarction, which is not observed in the first but appeared in the following imaging examination. It is very important in clinic to identify patients with high risk of HT ahead of recanalization and help choose better treatments. As part of the natural process of ischemic stroke, HT can also be precipitated or enhanced by interventions in the acute phase of stroke (Yaghi et al., 2015). Recanalization therapies save penumbral tissues from deteriorating but could make the situation more complicated, such as mechanical damages in thrombectomy and activation of fibrinolysis system in thrombolysis. Studies showed that thrombolysis and/or thrombectomy significantly increased the occurrence of HT (Yaghi et al., 2017; Kim et al., 2020). Therefore, in this study, we specifically focused on the patients who did not receive recanalization treatment and identified differential serum proteins between the HT and non-HT groups. Our findings suggest that the differential serum proteins are potential biomarkers to distinguish patients with high risk of HT, who may not be suitable for recanalization treatment, or need to be more cautious about undergoing recanalization treatment. Further studies are warranted to investigate whether the potential biomarkers screened from patients without recanalization are applicable for the patients with recanalization.
In this study, we used eight-labeled iTRAQ reagents to measure 37 patient serum samples at the same time. We mixed the serum samples according to the NIHSS scores in each group, as the NIHSS score has been considered as an important risk factor for HT (Kidwell et al., 2002; Strbian et al., 2012). Adjusting the sample size of the two groups to 1:1 improves the statistical efficiency and reduces the study costs (Seo et al., 2020). However, it is worth to note that pooled mixed samples represent a strong limitation rather than an advantage in clinical studies, given the strong heterogeneity of stroke patients. Further studies need to enlarge the sample size to test the efficacy of these differential proteins for HT prediction.
Our recent animal study showed that the level of blood occludin fragments was correlated with BBB permeability after cerebral ischemia and may serve as a potential biomarker for BBB damage (Pan et al., 2017). Further pilot clinical study showed that although blood occludin significantly elevated in AIS compared with pseudo-stroke patients, blood occludin alone is not sufficient enough for HT prediction (AUC 0.73–0.77) (Li et al., 2020). In this study, the iTRAQ approach did not detect occludin fragments from the blood samples, likely due to its very low abundance in serum. On the other hand, 17 differentially expressed proteins were identified as candidates of biomarkers to predict HT. Further studies are warranted to verify the prediction accuracy of combined or comprehensive biomarkers.
In this study, KEGG metabolic pathway analysis further indicated that most of differentially expressed proteins belong to ECM–receptor interaction, NOD-like receptor pathway, and PPAR signaling pathway, involved in the progress of HT. Studies showed that ECM receptors (such as integrins and dystroglycan) NLRP3 and PPAR, which are expressed in the brain microvasculature, mediate the connections between endothelial cells and matrix components in response to cerebral ischemia/reperfusion (Tedgui and Mallat, 2001; Baeten and Akassoglou, 2011; Gong et al., 2018; Zhou et al., 2019). These studies suggest that HT may be related to vascular damages and that the proteins related to vascular endothelium may serve as biomarkers for the progression of HT. Further studies are needed to investigate the mechanism of these vascular-related proteins in HT development.
Quantitative real-time polymerase chain reaction (RT-qPCR) or enzyme linked immunosorbent assay (ELISA) is usually preformed to validate iTRAQ findings. In this study, we did not test the proteomics results using RT-PCR or ELISA. There are two main reasons: first, the differentially expressed proteins in blood may come from injured tissues (such as brain tissue). The mRNA might not correlate with the level of related proteins. Second, most of the differential proteins we identified are lacking suitable commercial antibodies or ELISA kits. Further studies with large sample size are warranted to confirm these potential biomarkers for HT and validate using self-made antibodies or ELISA kits.
This study showed that NIHSS is significantly different between the two groups (HT vs. no HT patients), which is consistent with other studies (Emberson et al., 2014; Li et al., 2020). Moreover, NIHSS score has been brought into several clinic prediction models for HT (Lou et al., 2008; Mazya et al., 2013). In order to make the samples comparable between the two groups, the serum specimens were matched based on NIHSS score and stroke onset time in this study (Table 3). On the other hand, it is less probable for a patient with a small, mild stroke to develop HT. It would be more interesting to explore in patients with moderate-to-severe stroke the differential elements between those who did or did not develop HT. Further studies are warranted to focus on the HT biomarkers especially for moderate-to-severe stroke.
Studies showed that a small amount of bleeding also affects the prognosis of patients and also needs to be analyzed (Park et al., 2012). Although asymptomatic HT did not influence the 3-month and 1-year mortality like symptomatic HT, it affected the long-term prognosis of neurological function after acute cerebral infarction, especially the long-term cognition of patients or transformed into symptomatic bleeding (Dzialowski et al., 2007; Libman et al., 2012; Lei et al., 2014). Therefore, besides symptomatic HT (PH2, according to ECASS-III criteria), we also included the patients with asymptomatic HT (HI-1, HI-2, and PH1, according to ECASS-III criteria) for analysis in this study. We will further focus on the biomarkers of symptomatic HT.
Limitations
This is a preliminary study focusing on differences between HT and non-HT patients after AIS. There are several limitations: (1) the sample size in this retrospective study was small, and the results need to be further verified in large-size studies. (2) In order to improve the statistical efficiency and reduce the study costs, we adjusted the sample size of the two groups to 1:1 by pooled and mixed samples. The analysis of mixed sample may obscure the strong heterogeneity of stroke patients in clinic. Further studies need to enlarge the sample size to test the efficacy of these differential proteins for HT prediction. (3) In this study, we did not test the proteomics results using RT-PCR or ELISA. Further studies with large sample size are warranted to confirm these potential biomarkers for HT and to validate using self-made antibodies or ELISA kits.
In conclusion, we screened the potential serum biomarkers in AIS patients using iTRAQ-based quantitative serum proteomics analysis and identified 17 differentially expressed proteins between HT and non-HT patients. These findings suggest that these differentially expressed proteins, either alone or in combination, could be promising biomarkers for predicting HT after ischemic stroke.
Data Availability Statement
The original contributions presented in the study are publicly available. This data can be found here: ProteomeXchange, PXD026950.
Ethics Statement
The studies involving human participants were reviewed and approved by Ethics Committee of Xuanwu Hospital of Capital Medical University. The ethics committee waived the requirement of written informed consent for participation.
Author Contributions
ZQ, XZ, XJ, and KL made the study design. ZQ and SY performed the experiments and data analysis. ZQ wrote the manuscript. XZ, XJ, and KL revised the manuscript. All authors contributed to the article and approved the submitted version.
Funding
This work was supported in part by grants from the National Natural Science Foundation of China (81620108011) and Beijing Nova Program Interdisciplinary Cooperation Project (Z191100001119002).
Conflict of Interest
The authors declare that the research was conducted in the absence of any commercial or financial relationships that could be construed as a potential conflict of interest.
Publisher’s Note
All claims expressed in this article are solely those of the authors and do not necessarily represent those of their affiliated organizations, or those of the publisher, the editors and the reviewers. Any product that may be evaluated in this article, or claim that may be made by its manufacturer, is not guaranteed or endorsed by the publisher.
Footnotes
References
Álvarez-Sabín, J., Maisterra, O., Santamarina, E., and Kase, C. S. (2013). Factors influencing haemorrhagic transformation in ischaemic stroke. Lancet Neurol. 12, 689–705. doi: 10.1016/s1474-4422(13)70055-3
Baeten, K. M., and Akassoglou, K. (2011). Extracellular matrix and matrix receptors in blood-brain barrier formation and stroke. Dev. Neurobiol. 71, 1018–1039. doi: 10.1002/dneu.20954
Blacquiere, D., Lindsay, M. P., Foley, N., Taralson, C., Alcock, S., Balg, C., et al. (2017). Canadian stroke best practice recommendations: telestroke best practice guidelines update 2017. Int. J. Stroke Offi. J. Int. Stroke Soc. 12, 886–895. doi: 10.1177/1747493017706239
Castellanos, M., Sobrino, T., Millán, M., García, M., Arenillas, J., Nombela, F., et al. (2007). Serum cellular fibronectin and matrix metalloproteinase-9 as screening biomarkers for the prediction of parenchymal hematoma after thrombolytic therapy in acute ischemic stroke: a multicenter confirmatory study. Stroke 38, 1855–1859. doi: 10.1161/strokeaha.106.481556
Dzialowski, I., Pexman, J. H., Barber, P. A., Demchuk, A. M., Buchan, A. M., and Hill, M. D. (2007). Asymptomatic hemorrhage after thrombolysis may not be benign: prognosis by hemorrhage type in the Canadian alteplase for stroke effectiveness study registry. Stroke 38, 75–79. doi: 10.1161/01.str.0000251644.76546.62
Emberson, J., Lees, K. R., Lyden, P., Blackwell, L., Albers, G., Bluhmki, E., et al. (2014). Effect of treatment delay, age, and stroke severity on the effects of intravenous thrombolysis with alteplase for acute ischaemic stroke: a meta-analysis of individual patient data from randomised trials. Lancet 384, 1929–1935. doi: 10.1016/s0140-6736(14)60584-5
Gong, Z., Pan, J., Shen, Q., Li, M., and Peng, Y. (2018). Mitochondrial dysfunction induces NLRP3 inflammasome activation during cerebral ischemia/reperfusion injury. J. Neuroinflam. 15:242.
Hacke, W., Kaste, M., Bluhmki, E., Brozman, M., Dávalos, A., Guidetti, D., et al. (2008). Thrombolysis with alteplase 3 to 4.5 hours after acute ischemic stroke. N. Engl. J. Med. 359, 1317–1329.
Johnston, S. C., Easton, J. D., Farrant, M., Barsan, W., Conwit, R. A., Elm, J. J., et al. (2018). Clopidogrel and aspirin in acute ischemic stroke and high-risk TIA. N. Engl. J. Med. 379, 215–225. doi: 10.1056/nejmoa1800410
Kidwell, C. S., Saver, J. L., Carneado, J., Sayre, J., Starkman, S., Duckwiler, G., et al. (2002). Predictors of hemorrhagic transformation in patients receiving intra-arterial thrombolysis. Stroke 33, 717–724. doi: 10.1161/hs0302.104110
Kim, B. J., Menon, B. K., Kim, J. Y., Shin, D. W., Baik, S. H., Jung, C., et al. (2020). Endovascular treatment after stroke due to large vessel occlusion for patients presenting very late from time last known well. JAMA Neurol. [Epub ahead of print],
Kirchhof, P., Benussi, S., Kotecha, D., Ahlsson, A., Atar, D., Casadei, B., et al. (2016). 2016 ESC guidelines for the management of atrial fibrillation developed in collaboration with EACTS. Eur. J. Cardiothorac. Surg. 50, e1–e88.
Lei, C., Wu, B., Liu, M., and Chen, Y. (2014). Asymptomatic hemorrhagic transformation after acute ischemic stroke: is it clinically innocuous? J. Stroke Cerebrovascular Dis. Offi. J. Natl. Stroke Assoc. 23, 2767–2772. doi: 10.1016/j.jstrokecerebrovasdis.2014.06.024
Li, W., Qi, Z., Kang, H., Qin, X., Song, H., Sui, X., et al. (2020). Serum occludin as a biomarker to predict the severity of acute ischemic stroke, hemorrhagic transformation, and patient prognosis. Aging Dis. 11, 1395–1406. doi: 10.14336/ad.2020.0119
Libman, R. B., Kwiatkowski, T., El-Zammar, Z. M., and Levine, S. R. (2012). Is asymptomatic hemorrhagic transformation really innocuous? Neurology 78, 1703, author reply 1703–1704; 1704; author reply 1704.
Lou, M., Safdar, A., Mehdiratta, M., Kumar, S., Schlaug, G., Caplan, L., et al. (2008). The HAT score: a simple grading scale for predicting hemorrhage after thrombolysis. Neurology 71, 1417–1423. doi: 10.1212/01.wnl.0000330297.58334.dd
Mazya, M. V., Bovi, P., Castillo, J., Jatuzis, D., Kobayashi, A., Wahlgren, N., et al. (2013). External validation of the SEDAN score for prediction of intracerebral hemorrhage in stroke thrombolysis. Stroke 44, 1595–1600. doi: 10.1161/strokeaha.113.000794
Pan, R., Yu, K., Weatherwax, T., Zheng, H., Liu, W., and Liu, K. J. (2017). Blood occludin level as a potential biomarker for early blood brain barrier damage following ischemic stroke. Sci. Rep. 7:40331.
Park, J. H., Ko, Y., Kim, W. J., Jang, M. S., Yang, M. H., Han, M. K., et al. (2012). Is asymptomatic hemorrhagic transformation really innocuous? Neurology 78, 421–426. doi: 10.1212/wnl.0b013e318245d22c
Seo, G., Han, H., Vargas, R. E., Yang, B., Li, X., and Wang, W. (2020). MAP4K interactome reveals STRN4 as a key STRIPAK complex component in hippo pathway regulation. Cell Rep. 32:107860. doi: 10.1016/j.celrep.2020.107860
Shirasaki, Y., Edo, N., and Sato, T. (2004). Serum S-100b protein as a biomarker for the assessment of neuroprotectants. Brain Res. 1021, 159–166. doi: 10.1016/j.brainres.2004.06.012
Steffel, J., Verhamme, P., Potpara, T. S., Albaladejo, P., Antz, M., Desteghe, L., et al. (2018). The 2018 european heart rhythm association practical guide on the use of non-vitamin K antagonist oral anticoagulants in patients with atrial fibrillation. Eur. Heart J. 39, 1330–1393.
Strbian, D., Engelter, S., Michel, P., Meretoja, A., Sekoranja, L., Ahlhelm, F. J., et al. (2012). Symptomatic intracranial hemorrhage after stroke thrombolysis: the SEDAN score. Ann. Neurol. 71, 634–641. doi: 10.1002/ana.23546
Tedgui, A., and Mallat, Z. (2001). Anti-inflammatory mechanisms in the vascular wall. Circ. Res. 88, 877–887. doi: 10.1161/hh0901.090440
Wang, K., Shan, Z., Duan, L., Gong, T., Liu, F., Zhang, Y., et al. (2017). iTRAQ-based quantitative proteomic analysis of Yamanaka factors reprogrammed breast cancer cells. Oncotarget 8, 34330–34339. doi: 10.18632/oncotarget.16125
Wang, Y., Wang, Y., Zhao, X., Liu, L., Wang, D., Wang, C., et al. (2013). Clopidogrel with aspirin in acute minor stroke or transient ischemic attack. N. Engl. J. Med. 369, 11–19.
Yaghi, S., Boehme, A. K., Dibu, J., Leon Guerrero, C. R., Ali, S., Martin-Schild, S., et al. (2015). Treatment and outcome of thrombolysis-related hemorrhage: a multicenter retrospective study. JAMA Neurol. 72, 1451–1457. doi: 10.1001/jamaneurol.2015.2371
Yaghi, S., Willey, J. Z., Cucchiara, B., Goldstein, J. N., Gonzales, N. R., Khatri, P., et al. (2017). Treatment and outcome of hemorrhagic transformation after intravenous alteplase in acute ischemic stroke: a scientific statement for healthcare professionals from the american heart association/american stroke association. Stroke 48, e343–e361.
Zaheer, S., Beg, M., Rizvi, I., Islam, N., Ullah, E., and Akhtar, N. (2013). Correlation between serum neuron specific enolase and functional neurological outcome in patients of acute ischemic stroke. Ann. Indian Acad. Neurol. 16, 504–508. doi: 10.4103/0972-2327.120442
Zaidat, O. O., Fitzsimmons, B. F., Woodward, B. K., Wang, Z., Killer-Oberpfalzer, M., Wakhloo, A., et al. (2015). Effect of a balloon-expandable intracranial stent vs medical therapy on risk of stroke in patients with symptomatic intracranial stenosis: the VISSIT randomized clinical trial. JAMA 313, 1240–1248. doi: 10.1001/jama.2015.1693
Zhong, C., Yang, J., Xu, T., Xu, T., Peng, Y., Wang, A., et al. (2017). Serum matrix metalloproteinase-9 levels and prognosis of acute ischemic stroke. Neurology 89, 805–812. doi: 10.1212/wnl.0000000000004257
Keywords: isobaric tags for relative and absolute quantitation, serum proteomics, hemorrhagic transformation, acute ischemic stroke, biomarker
Citation: Qi Z, Yuan S, Zhou X, Ji X and Liu KJ (2021) Isobaric Tags for Relative and Absolute Quantitation-Based Quantitative Serum Proteomics Analysis in Ischemic Stroke Patients With Hemorrhagic Transformation. Front. Cell. Neurosci. 15:710129. doi: 10.3389/fncel.2021.710129
Received: 15 May 2021; Accepted: 27 July 2021;
Published: 25 August 2021.
Edited by:
Zhijuan Cao, School of Medicine, Stanford University, United StatesReviewed by:
Creed Stary, School of Medicine, Stanford University, United StatesAurora Semerano, San Raffaele Scientific Institute (IRCCS), Italy
Copyright © 2021 Qi, Yuan, Zhou, Ji and Liu. This is an open-access article distributed under the terms of the Creative Commons Attribution License (CC BY). The use, distribution or reproduction in other forums is permitted, provided the original author(s) and the copyright owner(s) are credited and that the original publication in this journal is cited, in accordance with accepted academic practice. No use, distribution or reproduction is permitted which does not comply with these terms.
*Correspondence: Zhifeng Qi, cWl6aGlmZW5nQHh3aC5jY211LmVkdS5jbg==