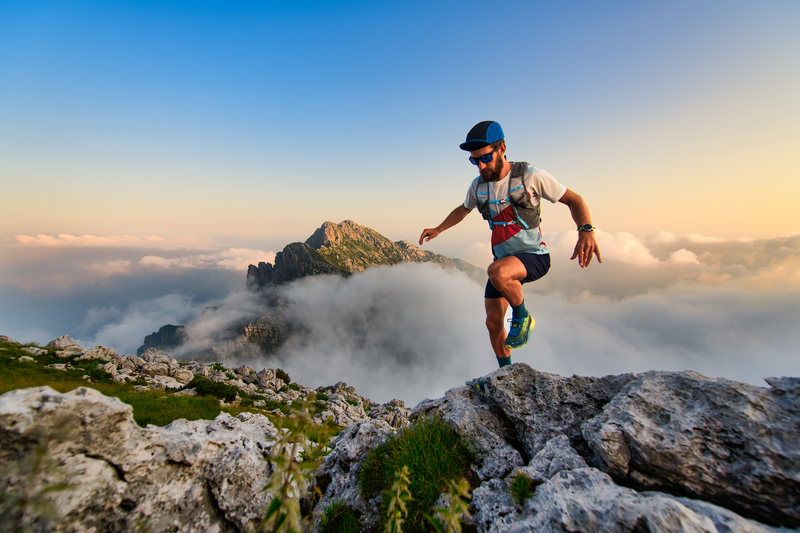
94% of researchers rate our articles as excellent or good
Learn more about the work of our research integrity team to safeguard the quality of each article we publish.
Find out more
REVIEW article
Front. Cell. Neurosci. , 03 August 2021
Sec. Cellular Neurophysiology
Volume 15 - 2021 | https://doi.org/10.3389/fncel.2021.684742
This article is part of the Research Topic Sensory Processing in Vision and Olfaction – Common Features of Key Players View all 32 articles
Olfactory stimuli are encountered across a wide range of odor concentrations in natural environments. Defining the neural computations that support concentration invariant odor perception, odor discrimination, and odor-background segmentation across a wide range of stimulus intensities remains an open question in the field. In principle, adaptation could allow the olfactory system to adjust sensory representations to the current stimulus conditions, a well-known process in other sensory systems. However, surprisingly little is known about how adaptation changes olfactory representations and affects perception. Here we review the current understanding of how adaptation impacts processing in the first two stages of the vertebrate olfactory system, olfactory receptor neurons (ORNs), and mitral/tufted cells.
Adaptation modulates the input-output transformation of a neuron or brain region based upon the recent history of an organism’s sensory experience (Barlow, 1961; Wark et al., 2007; Whitmire and Stanley, 2016; Weber and Fairhall, 2019; Benda, 2021). Nearly all sensory systems exhibit some form of adaptation in which prolonged exposure to a stimulus evokes a change in the neural response. Defining the functional transformation(s) that take place as a result of adaptation remains a fundamental field of study in neuroscience and a critical step in understanding sensory processing.
Sensory stimuli are experienced across a large range of intensities, but peripheral sensory neurons usually have a relatively small dynamic range. One function of adaptation is to shift this dynamic range toward relevant stimuli, a process that both expands the coding capacity of the sensory system and optimizes it (Barlow, 1961; Brenner et al., 2000; Wark et al., 2007; Whitmire and Stanley, 2016; Weber and Fairhall, 2019). For example, light can be experienced across ~10 log units of stimulus intensity (Skalicky, 2016), yet individual photoreceptors saturate across ~1–2 log units of light intensity located within their receptive field (Boynton and Whitten, 1970; Normann and Perlman, 1979; Valeton and van Norren, 1983; Perlman and Normann, 1998). Changing the background luminance level causes the sensitivity range of photoreceptors to shift along the intensity axis (Figure 1A; Boynton and Whitten, 1970; Normann and Perlman, 1979; Valeton and van Norren, 1983; Fain et al., 2001). This transformation allows a match between the photoreceptor coding capacity and the mean stimulus intensity. However, a changing neural response does not always reflect a shift in the dynamic range of a neuron. For example, the auditory system deals with a similar dynamic range problem (Viemeister, 1988; Chepesiuk, 2005) as auditory nerve fibers saturate within ~3–4 log units of sound intensity at their characteristic frequency, but the presence of a background stimulus causes reductions in auditory nerve spiking rather than a change in sensitivity (Figure 1B; Smith, 1977, 1979; Smith et al., 1983; but see Wen et al., 2009, 2012). Auditory neurons that exhibit dynamic range shifts exist, but at later stages of processing in the inferior colliculus and cortex (Dean et al., 2005; Nagel and Doupe, 2006; Watkins and Barbour, 2008). In general, matching the sensor’s dynamic range to the stimulus and optimizing its encoding in neural activity is the major goal of an adaptive change in sensory processing. But can we make similar observations in olfaction? Does adaptation adjust the dynamic range of olfactory neurons? And if so, which features of an odor stimulus are optimally encoded?
Figure 1. Two ways adaptation can transform neural responses. (A) Background stimuli of higher intensity (color coded) shift the neuron dynamic range toward higher concentrations, lowering its sensitivity to the stimulus. (B) Background stimuli of higher intensity (color coded) compress the dynamic range of the neuron. The neuron sensitivity is not changed, but the response saturates earlier, and the coding capacity is lower.
For many animals, the sense of smell is critical to recognize and locate food, mates, and dangers. Recognition requires the identification of a specific smell embedded in a complex chemical context. Localization, instead, requires the animal to extract information from a concentration profile that varies in time and space depending on the fluid-dynamic regime of the medium that transports these volatile molecules (Celani et al., 2014; Connor et al., 2018). The olfactory system, therefore, faces three challenges: (1) it has to segment the perception of a specific cue from other chemical stimuli present in the environment; (2) it has to maintain an invariant representation of the odor of interest when its concentration changes, and (3) at the same time, it has to recognize the direction of the changing gradient (Linster et al., 2007; Uchida and Mainen, 2007; Homma et al., 2009; Gottfried, 2010; Rokni et al., 2014). In this review, we summarize the functional changes in odor encoding driven by adaptation to sustained stimuli and discuss which cellular and network mechanisms could support background segmentation, concentration invariance, and contrast coding in the vertebrate olfactory system.
Olfactory receptor neurons (ORNs) located in the olfactory epithelium send their axons to one or two glomeruli in the Olfactory Bulb (OB; Ressler et al., 1994; Vassar et al., 1994; Zapiec and Mombaerts, 2015) where they synapse on Mitral and Tufted Cells (MTCs), which then project to the olfactory cortices (Figure 2, green processes, Sosulski et al., 2011; Imamura et al., 2020). The OB input-output transformation is shaped by a complex synaptic network that includes many different populations of interneurons that surround each glomerulus (Parrish-Aungst et al., 2007; Nagayama et al., 2014; Burton, 2017), and granule cells that form dendro-dendritic synapses with the lateral processes of MTCs (Rall et al., 1966; Yokoi et al., 1995; Figure 2, blue and black cells and processes). Adaptation of ORNs has been extensively studied, although the functional role of the identified mechanisms remains a source of debate. Since odor stimuli are encoded combinatorially in populations of ORNs, the adaptation of olfactory representation likely involves coordinated changes across this population of cells, mediated by lateral connections within the OB. Moreover, in breathing animals, respiration plays a key role in modulating the sampling of the stimulus by controlling the times and durations of the odorous plumes that reach the sensory neurons. Here, we will compare results from in vitro experimental approaches that recorded odor responses from ORN somata in the epithelium, and in vivo experiments in breathing animals that quantified the response of the ORNs at their presynaptic site in the OB and the response of their postsynaptic MTCs. We will describe the concentration dependency of the response dynamics of these neurons and then focus on the changes in activity induced by repeated and sustained odor stimuli. These changes can occur over a wide range of timescales (seconds to days; Wang et al., 1993; Dalton and Wysocki, 1996; Chaudhury et al., 2010; Kass et al., 2016), but here we will focus on relatively short–term adaptation that is most likely to be relevant for odor navigation. Moreover, we focus on the vertebrate literature as adaptation in invertebrates has been recently covered (Brandão et al., 2021).
Figure 2. Schematic of the Olfactory Bulb (OB; adapted from Storace and Cohen, 2017). Panel (B) reflects an expansion of the box in panel (A). nc, nasal cavity; oe, olfactory epithelium; ob, olfactory bulb.
In vertebrates, olfactory stimuli are detected by ORNs that express one out of a large family (>1,000) of odorant receptors (ORs; Buck and Axel, 1991; Ngai et al., 1993; Ressler et al., 1994). ORNs extend cilia into the olfactory mucosa, where they are exposed to odor molecules. In vitro voltage or current clamp experiments from isolated ORNs allowed the identification of key players in the signaling cascade activated by an odor response (Schild and Restrepo, 1998). In short, the binding of an odor molecule to the receptor triggers the activation of a metabotropic pathway that leads to the increase of cAMP and opening of cyclic nucleotide gated (CNG) channels (Kleene, 2008). Calcium influx through CNG channels further enhances ORN depolarization by activating a Cl− current (Reisert and Zhao, 2011). This amplification step is responsible for a large percentage of the odorant-induced transduction current. Consistent with classical models of receptor-ligand binding kinetics (Dougherty et al., 2005), the amplitude of the transduction current associated with this signaling cascade exhibits a sigmoidal monotonic relationship with odor concentrations, with most studies reporting a dynamic range of ~10-fold (Firestein et al., 1993; Menini et al., 1995; Kurahashi and Menini, 1997; Ma et al., 1999; Reisert and Matthews, 2001b; but see Grosmaitre et al., 2006). Before additional mechanisms kick in, ORN peak firing rates also show a similarly narrow dynamic range (Reisert and Matthews, 1999, 2001a,b; Bozza et al., 2002). However, the dynamics of the transduction current is concentration-dependent and develops a faster transient component at higher concentrations (Menini et al., 1995; Reisert and Matthews, 1999). Driven by the transduction current, ORN firing rates also become more transient and the overall number of elicited spikes decreases for stronger stimuli, eventually reducing to only a few spikes at the onset of a high odor concentration (Getchell and Shepherd, 1978a,b; Duchamp-Viret et al., 1999, 2003; Reisert and Matthews, 1999, 2001a,b; Figure 3A). Current injection in ORNs induces much less complex firing dynamics (Ma et al., 1999) demonstrating a key role for the transduction current in determining ORN response dynamics.
Figure 3. Comparisons of olfactory receptor neurons (ORNs) odor responses measured from the soma and at their glomerular projections in the olfactory bulb. (A) Odor stimuli elicit transient response dynamics as concentration increases, resulting in higher firing rates elicited in a very short time window and an overall decrease in the number of spikes fired. Cartoon inspired to data from Reisert and Matthews (1999). (B) Calcium measurements from ORN glomerular projections do not show transient dynamics nor reduced calcium levels at higher odor concentrations. Data reproduced from Storace et al. (2019). (C) Paired-pulse experiments showing two ORNs with different sensitivity and response dynamics. Consistent with published data, ORN1 is more sensitive to the odor and its response to a consecutive odor pulse is attenuated (2 s interstimulus). ORN1 response fully recovers in 4 s. ORN2 is less sensitive and does not show paired-pulse response attenuation. (D) Prediction of the response of the two ORNs to a continuous stimulus inhaled through several respiratory cycles (respiratory traces are unpublished data from D.A. Storace).
Recording from isolated ORNs allows for the tight control of the stimulus and direct quantification of their electrical properties but shortcuts several steps that are important in vivo, such as respiration and the absorption of odor molecules in the mucus (Reisert and Matthews, 1999). For example, testing an odor response requires delivering odors in solution rather than as a volatile airborne stimulus. Electrode measurements from ORNs in vivo are possible (Duchamp-Viret et al., 1999), but the approach is not widely used due to the technical challenges posed by the physical organization of the ORNs and the location and structure of the olfactory mucosa (Duchamp-Viret and Chaput, 2018). Calcium imaging offers an alternative approach for quantifying ORN responses in vivo. ORNs can be anatomically labeled with organic dyes (Friedrich and Korsching, 1997; Ma and Shepherd, 2000; Wachowiak and Cohen, 2001; Fried et al., 2002; Wachowiak et al., 2002; Korsching, 2005), and genetic targeting strategies have used the olfactory marker protein (OMP) promoter (Farbman and Margolis, 1980; Danciger et al., 1989) to generate transgenic mice expressing different reporters of neural activity in ORNs (Bozza et al., 2004; McGann et al., 2005; Albeanu et al., 2018; Dewan et al., 2018; Platisa et al., 2020).
Functional imaging from single cells in the olfactory epithelium in vivo has reported monotonic relationships between calcium transients and odor concentration for many odor-receptor combinations (Inagaki et al., 2020, 2021; Xu et al., 2020; Zak et al., 2020). This is in agreement with the role of calcium in the initial amplification step in the signaling cascade (Leinders-Zufall et al., 1997, 1998). However, these measurements of somatic calcium dynamics did not show the transient activity measured in electrophysiological experiments (Reisert and Matthews, 1999, 2001a,b) and thus may not be an appropriate reporter of ORN firing rates.
How do peripheral firing dynamics translate to synaptic inputs to the bulb? Functional imaging has been widely used to quantify the neural activity measured from ORN axon terminals innervating the glomerular layer. ORN glomerular imaging trades single-cell resolution for a gain of information at the population level measured across the ~200 glomeruli that are located on the dorsal surface of the OB (Vincis et al., 2012). Measurements from the ORN glomerular projections reflect some combination of ORN firing activity as well as modulation by the OB network via presynaptic GABA and dopamine receptors expressed on the ORN axon terminals (Nickell et al., 1991, 1994; Wachowiak and Cohen, 1998, 1999; Koster et al., 1999; Berkowicz and Trombley, 2000; Ennis et al., 2001; McGann, 2013). In principle, pooling the response of the multiple ORNs within a single glomerulus (Cleland and Linster, 1999; Cleland et al., 2011; Cleland and Borthakur, 2020), as well presynaptic modulation at the axon terminals (Cleland and Linster, 2012; McGann, 2013) should broaden the dynamic range of the glomerular ORN response. However, a direct comparison between calcium transients measured from ORN somata and from their terminals in the OB revealed no major differences (Zak et al., 2020). Importantly, with one exception (Lecoq et al., 2009), all these studies reported primarily monotonic relationships between presynaptic activity and odor concentration and tonic responses dynamics, in contrast with the concentration dependency of ORN somatic firing patterns (Figures 3A,B). These differences could appear to be a minor discrepancy, but understanding the response dynamics of ORNs to changes in stimulus intensity is critical for understanding their adaptive features.
Natural odor stimuli are carried by air, often in turbulent regimes that break diffusive odor signals into filaments of different sizes. This has the effect of generating an intermittent and stochastic concentration profile (Celani et al., 2014; Connor et al., 2018). Moreover, in breathing animals, respiration modulates the sampling of an odor stimulus by controlling the times and durations of the odor plumes that reach the ORNs. Consequently, nearly no odor stimulus is sensed in complete isolation, and it is, therefore, crucial to understand how ORN responses are affected by stimulus history.
Paired-pulse experiments have been used to mimic the arrival of consecutive plumes or the intermittency imposed by respiration on odor perception. Measurements in vitro show that ORNs exhibit a form of adaptation that attenuates their response up to inter-stimulus intervals of 6–10 s (Kurahashi and Menini, 1997; Leinders-Zufall et al., 1998; Ma et al., 1999). This is a surprisingly long timescale and suggests that in freely breathing animals ORN responses should change drastically across breathing cycles (Figures 3C,D). As one would expect, this effect depends on the odor concentration and the stimulation or respiratory rate. ORNs reliably fire spikes in vitro at every odor pulse of a moderate concentration when delivered at 2 Hz intervals, but spiking is less reliable and firing responses attenuate and eventually disappear at higher stimulation rates (5 Hz) or at higher odor concentrations (Ghatpande and Reisert, 2011). Although an exact quantification of the ORN integration time has not been attempted in vertebrates, these experiments show that it likely falls in the range of the respiratory period (200–500 ms). Whether this is a limitation of the olfactory periphery in precisely encoding odor stimuli or serves a functional role in filtering sensory inputs sent to the brain remains unclear. The in vitro data suggest that for a fixed stimulation frequency, only the ORNs that are mildly activated by the odor would contribute to encoding the full stimulus sequence, while more sensitive ones would signal only the onset of the sequence and remain otherwise silent (Figure 3D, compare ORN1 and ORN2).
Stimulus-driven changes in response do not only originate at the periphery. In vitro and in vivo studies have shown that repeated electrical stimulation of the olfactory nerve (which shortcuts peripheral adaptation) affects the amount of glutamate released onto post-synaptic neurons (Aroniadou-Anderjaska et al., 2000; Ennis et al., 2001; Murphy and Isaacson, 2003). Consistently, calcium imaging from ORN terminals in vitro and in vivo reported adaptation to paired-pulse electrical stimulation of the olfactory nerve that recovered with interstimulus intervals longer than 1 s (McGann et al., 2005; Wachowiak et al., 2005; Pírez and Wachowiak, 2008). This adaptation appears to be due to depression at the level of the ORN terminals as it similarly affects simultaneously recorded post-synaptic neurons (Murphy et al., 2004). Application of a GABA antagonist reduced the effect of the paired-pulse depression and increased the rate of recovery to paired-pulse stimulation in vitro (Aroniadou-Anderjaska et al., 2000; Ennis et al., 2001; Wachowiak et al., 2005), suggesting a key role for inhibition in the temporal filtering of olfactory information. Similarly, studies in insects have shown that depression at the synapses between ORNs and PNs (the invertebrate homolog of MTCs) act on multiple time scales to filter incoming signals (Kazama and Wilson, 2008; Martelli and Fiala, 2019), while the dynamics of lateral inhibition controls the width of these filtering steps (Nagel et al., 2015). The dynamic interplay between feedforward depression and lateral inhibition remains to be investigated in the mammalian brain in vivo, especially in the context of adaptation in breathing animals.
The combination of peripheral and central mechanisms that attenuate the response to consecutive odor pulses should lead to a major change in the OB activation across consecutive respiratory cycles. However, in vivo imaging from the ORN terminals in awake and anesthetized rats and mice reported attenuation only for high respiratory frequencies (>4 Hz) and for specific odor receptor combinations (Verhagen et al., 2007; Carey et al., 2009; Carey and Wachowiak, 2011). Inter-stimulus intervals of 330–1,000 ms, which corresponds to respiratory frequencies of 1–3 Hz, have been reported to induce substantial depression in vitro (Kurahashi and Menini, 1997; Leinders-Zufall et al., 1998; Zufall and Leinders-Zufall, 2000; Wachowiak et al., 2005), but similar rates only evoke minor effects in the activity measured from the glomeruli in vivo (Verhagen et al., 2007; Carey and Wachowiak, 2011). It is unclear whether these inconsistencies are due to different experimental conditions or due to odor-driven and state-dependent presynaptic processing in the bulb. More specific approaches are needed to understand the relationship between the peripheral processes and the presynaptic activity in the bulb. One key open question is whether the OR expressed plays a significant role in determining the ORN response dynamics. If this is not the case, then one could conclude that differences in the activity of different glomeruli depend on lateral connectivity. Most of the earliest studies have been performed from randomly selected cells, but the development of transgenic animals with genetically labeled ORN types allows for a comparison of the same receptor types across preparations (Bozza et al., 2002; Grosmaitre et al., 2006; Ghatpande and Reisert, 2011). This approach could be further exploited to investigate receptor-specific response properties and would clarify the contribution of intrinsic cellular mechanisms that originate at the periphery and presynaptic modulation which is mediated by lateral inputs in the OB.
A classical approach to study adaptive properties of sensory neurons is to compare the response to a stimulus presented in isolation and on a background. Experiments in vitro showed that adaptation to an odor background lowers the ORN transduction current, as well as the firing rate response to an odor (Reisert and Matthews, 1999, 2000). In adapted conditions, response saturation is reached at lower peak firing rates and at similar concentrations than in the absence of a background, causing a compression of the ORN dynamic range. Similar results were reported in Drosophila ORNs (Martelli et al., 2013; Brandão et al., 2021).
The functional consequences of a decreased dynamic range remain unclear. Such compression does not support contrast invariant responses, and therefore single ORNs likely do not signal stimulus contrast. However, the data indicate that in the presence of a background, ORN firing is more parsimonious, consisting of transient responses of a smaller number of spikes (Figure 3A). Therefore, at the population level, this could still constitute a strategy to efficiently signal changes in concentration and segregate relevant stimuli from the background. Indeed, odors are detected by a large array of ORNs, each expressing an odorant receptor with a different affinity to odor molecules. Therefore, the olfactory system is, by constitution, endowed with a large array of sensors tuned to different intensities of the same stimulus (i.e., different concentrations of a monomolecular odorant; Cleland et al., 2011; Zak et al., 2020). For example, when the background is too high an ORN will go silent, rather than shifting its dynamic range, which is energetically convenient given that the system can rely on the activation of other ORNs of lower sensitivity. Thus, adjusting the dynamic range of the response of single sensory neurons may not be the primary function of adaptation in olfaction.
Such hypotheses should be tested at the level of the OB by quantification of the population response to stimuli presented in isolation or on a background. However, the stimulus protocol can hardly be the same in vivo and in vitro, as respiration adds a level of complexity to the encoding of odor information. Respiratory frequency determines the degree by which adaptation affects the response to a chemically different odor superimposed on a background (Verhagen et al., 2007). At low inhalation rates, glomeruli that are sensitive to both background and test odors responded strongly to their superposition, but higher respiratory rates caused significant adaptation to the background and a highly attenuated response to the test odor. Unfortunately, the interpretation of these results cannot be solely based on adaptation, as mixture interaction at the level of olfactory receptor activation and at the OB local network could have effects on the observed dynamics (Inagaki et al., 2020; Xu et al., 2020; Zak et al., 2020). However, the data suggest so far that at low respiratory frequencies, ORNs have enough time to recover from adaptation during the exhalation phase. This would mean that tuning respiration could be used as a tool to adapt and dis-adapt the sensory neurons, facilitating background segregation in one direction and enhancing mixture integration in the other. Stimulus-specific adaptation should be further investigated using background stimuli of the same chemical identity as the test one.
The molecular bases of olfactory transduction have been investigated extensively over many decades. In short, calcium has been identified as a key player in suppressing ORN responses in paired-pulse experiments (Zufall et al., 1991; Kurahashi and Menini, 1997). The calcium-calmodulin (Ca2+-CaM) complex had been originally proposed as the main modulator of cAMP affinity to the CNG channels (Chen and Yau, 1994; Bradley et al., 2001). However, a subsequent study showed that the Ca2+-CaM feedback does not regulate cAMP sensitivity of the channel, but rather controls response termination (Song et al., 2008). Similarly, a genetic approach has revealed a minor role for the Ca2+-CaM feedback on ACIII (Reisert and Zhao, 2011), a molecular pathway that had been initially proposed as a mechanism to adapt sensitivity in the presence of prolonged stimulation (Leinders-Zufall et al., 1999). Mechanisms involved in the hydrolyzation of cAMP and in the removal of ciliary calcium have been proposed as important regulators of ORN response termination that requires the closing of CNG channels and calcium dependent Cl− channels (Reisert and Zhao, 2011).
Whether it is possible to mechanistically separate response termination from an adaptation of sensitivity remains unclear. These two phenomena remain coupled if their dynamics are not taken experimentally apart. Paired-pulse experiments, for example, do not allow a net separation of the two mechanisms as the response to following stimuli could be lowered either by a slow response termination or by a change in sensitivity. However, this is crucial in the context of breathing animals, and it makes sense that the olfactory system has implemented mechanisms to control the speed of response onset and termination (rather than to adjust sensitivity), as this is fundamental to the perception of stimuli wrapped in the inhalation phases. Failure to keep up with respiration can lead to unreliable responses. Whether these response mechanisms are adaptive in the sense that their properties adjust to the respiratory cycle or are just limited by respiratory frequency remains unclear. An analysis of the temporal aspects of ORN firing rate responses with more complex stimuli is needed to further understand the peripheral processing of odor stimuli and the specific role of identified molecular pathways.
Experiments across a variety of different model organisms, preparation types, and experimental techniques reported a complex and heterogeneous relationship between MTC activity and odor concentration. Individual cells exhibit excitatory or inhibitory responses and cell-specific temporal dynamics to different concentrations of the same odor. Electrophysiological studies have shown that there is no obvious rule for the encoding of stimulus concentration in the firing rate of single MTCs (Sirotin et al., 2015). Some MTCs show monotonic (increasing or decreasing) responses to changes in stimulus concentration, some MTCs show nonmonotonic responses, and others have concentration invariant responses (Mathews, 1972; Kauer, 1974; Kauer and Shepherd, 1977; Meredith and Moulton, 1978; Mair, 1982; Meredith, 1986; Reinken and Schmidt, 1986; Chaput and Lankheet, 1987; Hamilton and Kauer, 1989; Motokizawa, 1996; Chalansonnet and Chaput, 1998; Niessing and Friedrich, 2010; Banerjee et al., 2015; Yamada et al., 2017). Similar diversity is observed in 2-photon imaging experiments that quantified activity from the MTC dendritic arborizations in the glomerular layer (Economo et al., 2016; Moran et al., 2019; Storace et al., 2019). This range of concentration dependence across MTCs is strikingly wider than what has been observed in the ORN input to the glomerular layer, which is mostly monotonic with odor concentration (Figure 3B). This suggests a major function of the OB circuit in shaping the encoding of odor concentration.
Maintaining both a concentration-invariant representation of an odor as well as concentration-specific information are both critical for robust and flexible behavior (Figure 4A). Can these aspects of an odor stimulus be decoded from the population activity of the OB rather than from single MTCs? Clearly, information about absolute concentration is not discarded in the OB since animals can discriminate different concentrations of the same odor (Jordan et al., 2018a) and use concentration information for tracking an odor to its source (Catania, 2013; Findley et al., 2021). Information about concentration is retained in the combinatorial activity of the glomeruli (Storace and Cohen, 2017; Storace et al., 2019). Higher odor concentrations not only recruit ORNs with lower sensitivity (Wachowiak and Cohen, 2001; Bozza et al., 2004) but also drive more lateral inputs in the OB (Banerjee et al., 2015; Storace et al., 2019). Thus, concentration changes will activate both excitatory and inhibitory synapses, which will affect the overall MTC population activity. In zebrafish, MTC population responses have been shown to be robust within a certain range of odor concentrations (Niessing and Friedrich, 2010). However, studies in mice did not confirm these observations and rather show that different odor concentrations elicit a continuum of distinguishable representations at the population level (Bathellier et al., 2008). Therefore, it seems too simplistic to try to assign a single function to the OB circuit.
Figure 4. (A) In order to localize an odor source, the animal should keep a concentration invariant representation of the odor stimulus, while tracking its changing concentration and segmenting the source-specific smell from other background odors. (B) Three kinds of transformations that occur in the olfactory bulb could support these computations. (Top) Global interglomerular inhibition performs a divisive normalization of the OB output. Increasing odor concentration increases feedforward excitation and lateral inhibition leading to a concentration invariant representation in MTCs. (Middle) Specific interglomerular inhibitory inputs may support background segmentation. (Bottom) Intraglomerular inhibition could shift the sensitivity of MTCs, which would allow them to retain contrast information in different odor backgrounds.
There are at least three types of lateral inputs with possibly different functions in the OB (Figure 4B). First, lateral inhibitory neurons that broadly innervate the OB rescale the excitatory feedforward responses of individual glomeruli by the overall activation of the bulb (Cleland and Sethupathy, 2006; Banerjee et al., 2015). Similar to the insect antennal lobe network, this kind of connectivity could support a canonical computation, named divisive normalization (Olsen et al., 2010; Carandini and Heeger, 2011). Theoretical considerations suggest that divisive normalization may lead to concentration invariant representations in the OB output (Figure 4B, top). Dual-color voltage and calcium imaging have shown that odor representations become indeed more concentration-invariant across this synaptic step (Storace and Cohen, 2017; Storace et al., 2019). However, in addition to broad inhibitory inputs, several examples of selective inhibitory inputs have been reported, which most likely play a key role in shaping odor-specific OB output activity patterns (Fantana et al., 2008; Economo et al., 2016). As proposed in a model of the OB (Koulakov and Rinberg, 2011), this kind of inhibitory feedback could lead to transient output responses and, therefore, enhance segregation of background from foreground stimuli (Figure 4B, middle). Finally, the OB output is modulated by high-sensitivity periglomerular interneurons that can suppress MTC responses to weak ORN input within a single glomerulus, shifting their sensitivity to higher concentrations (Gire and Schoppa, 2009; Cleland and Linster, 2012). This sensitivity shift could, in principle, support contrast invariant responses of MTCs (Figure 4B, bottom). These three computations probably run in parallel, as none of them seem to be sufficient to explain the response of all MTCs.
We conclude that, even though the architecture of the OB circuit can support both concentration invariance, background segmentation, and contrast encoding (Figure 4), it remains unclear whether these three computations lead to distinct parallel readouts at the level of the OB or rather require further processing downstream of the OB. Better insight into the function of the OB circuit should be obtained by looking at the adaptive changes in the function of these circuit motifs and how they shape MTC responses to sustained and repeated stimulation.
How do MTCs respond to a sustained odor stimulus? Because subsets of MTCs are strongly coupled to inhalation, population activity on short timescales is driven by the respiration frequency, and population dynamics elicited by a continuous odor stimulus evolves on cyclic patterns (Bathellier et al., 2008). Single MTCs show a large diversity of responses across these breathing cycles. Relatively sustained spiking activity has been observed in rats when using an artificial sniffing paradigm at rates between 2–5 Hz (Sobel and Tank, 1993), and in freely breathing rats in response to 40–50 s long odor stimuli (Wilson, 1998; Cang and Isaacson, 2003; Kadohisa and Wilson, 2006). However, other studies reported marked attenuation of MTC firing rates in the presence of continuous or repetitive odor stimulation (Potter and Chorover, 1976) and similarly in freely breathing mice across respiratory cycles (Meredith and Moulton, 1978; Døving, 1987; Sobel and Tank, 1993; Wilson, 2000; Margrie et al., 2001; Sirotin et al., 2015; Bolding and Franks, 2018; Ogg et al., 2018; Moran et al., 2019; Parabucki et al., 2019).
Can the large diversity of temporal integration properties in MTCs be quantified? Similarly to results from insect olfactory projection neurons (Geffen et al., 2009; Martelli and Fiala, 2019), convolution of the stimulus with a linear filter is sufficient to predict the response of single MTCs to odor pulses of different lengths and temporal dynamics (Gupta et al., 2015). Each MTC-odor pair can be fitted by a linear filter of a specific shape: some with a positive polarity indicating excitatory responses, and some with a negative polarity indicating inhibitory responses. In most cases, these linear filters are biphasic (i.e., with a positive lobe followed by a smaller negative one, or vice versa), which suggests that these neurons calculate a derivative of the incoming stimuli. In other words, at least a subset of MTCs respond to changes in concentration and adapt their firing to a sustained stimulus.
Although the shape of the linear filters is variable across cells, in most cases they are about 0.5–1 s wide, indicating that filtering and integration of the stimulus occur on timescales longer than a single respiratory cycle. This is consistent with the observation that population dynamics evolve on timescales longer than the respiratory frequency (Bathellier et al., 2008). On the contrary, MTC linear filters extracted in response to current injection when all synaptic inputs were blocked, have much shorter integration times on the order of 20 ms (Padmanabhan and Urban, 2010), which argues for a major role of synaptic inputs in determining MTC dynamics on longer timescales. The linear filters however do not predict the response to different concentrations of the same stimulus, confirming again the non-linearity of MTC responses to odor intensity and suggesting that scaling odor concentration does not simply scale feedforward signals. Importantly, MTC responses in breathing animals can be predicted by the convolution of the cell-odor specific filter with the respiratory flow (Gupta et al., 2015), and therefore the actual odor reaching the receptor neurons can be assumed to be a simple product of odor stimulus and respiration. This model predicts that if the breathing period is shorter than the filter width, then the response to consecutive inhalations will fuse, resulting in a reduced locking of the response to respiration. Different degrees of respiration locking have been observed across individual MTCs (Patterson et al., 2013; Eiting and Wachowiak, 2020), but whether this linear model captures such a broad spectrum of properties has not been tested. Specifically, mitral cells exhibit longer response durations than tufted cells (Short and Wachowiak, 2019), and tufted cells are more locked to respiration (Nagayama et al., 2004; Igarashi et al., 2012; Díaz-Quesada et al., 2018; Eiting and Wachowiak, 2020). One interesting possibility is that the degree of respiration locking depends on the integration timescale (i.e., the width of the linear filter) of different cells. A shorter integration time would lead to stronger respiratory coupling, but a lower capability to compare stimuli across respiratory cycles. Whether these different dynamics could reflect intrinsic properties of mitral and tufted cells or different synaptic inputs in these cell types remain an open question.
Further analysis is required to identify cell-intrinsic and network mechanisms that determine the different temporal properties of mitral and tufted cells. But do these properties imply that different information about the stimulus is encoded in different MTCs?
Delivering an isolated odor pulse involves presenting a new chemical at some concentration. Is the response of a neuron determined by the specific odor, its absolute intensity, or the relative change in concentration compared to the background? Very few studies have quantified how MTCs respond to changes in odor stimuli starting from an adapted state. One study reported that MTCs show minimal adaptation to a background odor and respond to the addition of a second odor as the sum of the response to the two odors presented individually (Kadohisa and Wilson, 2006). However, this seems to be a special case. More recent investigations clarified that generally, background odor adaptation significantly affects MTC responses in a cell- and odor-dependent manner (Vinograd et al., 2017; Parabucki et al., 2019). Three types of MTCs have been identified based on their functional response to step increases in odor concentration. Type I encodes absolute concentration, type II is concentration invariant, and type III responds to relative changes in concentration (Parabucki et al., 2019). These, however, are not genetically distinct cell types with assigned functions, as the same MTC can be concentration invariant or encode concentration changes depending on the specific stimulus delivered. These observations are therefore consistent with the diversity of dynamics described in the previous paragraph, with a range of capabilities to differentiate and integrate incoming stimuli in a cell- and odor-specific manner.
One possibility is that the OB network is designed to encode different features of a smell in different subsets of MTCs: stimulus intensity in type I responses, stimulus identity in type II responses, and stimulus contrast in type III responses. But further experiments are needed to understand whether these categories are robust to larger concentrations ranges, respiration rates, and odor identity. Since these functional classes of MTC responses do not seem to define cell types, they are most likely determined by lateral connectivity and the specific activation within their local network. One exciting possibility is that the majority of lateral inputs on a MTC differs in different contexts (Figure 4B). This hypothesis further raises the question of whether these MTC subtypes belong to the same glomerulus or rather sibling MTCs from the same glomerulus are wired to inhibitory neurons of different classes.
Following the diverse dynamics of single neurons, the odor representation in the population of MTCs evolves spatially over breathing cycles. Several studies have shown that the correlation between the current population response and the initial response decays over breathing cycles (Cleland and Linster, 2012; Patterson et al., 2013; Friedrich and Wiechert, 2014; Díaz-Quesada et al., 2018; Eiting and Wachowiak, 2020). This indicates an overall reorganization of the OB activity that is not a simple linear attenuation. But where does this transformation lead to? One possibility is that odor representations evolve based on a categorization process, somehow intrinsic to the OB network connectivity and driven by the coactivation of certain glomerular patterns. Morphing experiments have been used to quantify the degree of categorization within the OB using a continuum of proportional mixtures of two odors. In Zebrafish it was shown that temporal decorrelation in population activity supports the classification of gradually changing stimuli into discrete output patterns (Niessing and Friedrich, 2010). However, a similar approach in rats reached opposite conclusions (Khan et al., 2008), suggesting that categorization might or might not occur depending on the specific concentration range or odorant mixtures used. Even so, it remains clear that this time-dependent and stimulus-induced population plasticity could help disentangle temporally intermingled odor stimuli as they occur in the wild (Figure 4A). One possibility is that the autocorrelation of distinct odor sources will drive adaptation in different odor-specific sets of coactivated glomeruli that will independently evolve in different directions in the response space, leading to the separate categorization of coherent components from non-coherent ones. This process would support background segregation of complex chemical mixtures.
One intriguing aspect of olfactory coding in breathing vertebrates is the capability of animals to modulate respiration in a task-dependent manner to sample odor stimuli (Verhagen et al., 2007; Wesson et al., 2008; Koldaeva et al., 2019). Differences in respiration determine differences in the statistics of the perceived odor stimuli. The example in Figure 5 shows that the mean stimulus intensity, as well as the shape of the stimulus distribution, strongly depend on the respiration pattern [here we assumed that the odor concentration activating the ORNs is a simple product of odor stimulus and inhalation airflow (Gupta et al., 2015)]. The distribution of the inputs determines the degree of adaptation and stimulus-driven plasticity in sensory pathways, and therefore modulation of sniffing changes both the input stimulus as well as the OB network state that processes incoming stimuli.
Figure 5. Respiratory rate shapes the statistics of the stimulus reaching the ORNs. (A) Example of odor stimulus reaching the animal: (left) constant concentration, (right) random flickering odor stimulus with the same fixed concentration. (B) Time-dependent odor concentration reaching the ORNs calculated as the product between the inhalation phase of the normalized respiratory trace and the odor stimulus. (C) Distribution of the odor concentration reaching the ORNs. Different stimulation protocols and respiratory patterns give rise to different distributions even though the delivered odor concentration was the same.
As with ORNs, the respiratory rate also influences MTC activity (Carey and Wachowiak, 2011; Díaz-Quesada et al., 2018) with diverse effects across individual MTCs. Increased respiration rate can drive a decrease or an increase in firing rate, or in some cases induce a higher degree of locking to the inhalation phase. The timescales on which these changes occur have not been explicitly quantified, but it seems unlikely that the data can be explained with a single mechanism. Rather, a range of different mechanisms that act on multiple timescales up to several seconds seems to be activated depending on the respiration rate (Díaz-Quesada et al., 2018). These observations challenge the linear filter model of MTC responses, which would only fit dynamics up to 1 s, and suggest that they probably only capture steady-state properties of MTCs. While some of the stimulus-induced changes in MTCs may reflect ORN adaptation (Ogg et al., 2015), MTCs can exhibit attenuated responses even when the corresponding presynaptic input is sustained (Storace and Cohen, 2019). Moreover, the preferred phase of individual MTC responses shift with inhalation frequency (Díaz-Quesada et al., 2018), a property that has not been observed in ORNs and is thus unlikely to be inherited from the periphery.
In general, higher inhalation frequencies result in stronger changes in MTC activity, but other parameters such as inhalation duration or amplitude might play an important role in freely breathing animals (Courtiol et al., 2011a,b). Changes in sniffing can mimic the effect of increased concentration on MTC firing, even though at the behavioral level information about odor concentration remains intact (Jordan et al., 2018a). This implies the existence of a mechanism to decouple the effect of respiration from the odor stimulus and suggests an important role of respiratory-driven MTCs in odor information transmission. Animals tune their respiration in a task-specific manner (Jordan et al., 2018b), and therefore, respiration could be modulated to shape the statistics of the perceived odor stimulus without compromising its information content (Figure 5). It would be interesting to understand how the different features of respiration (i.e., inhalation frequency, duration, and amplitude) differently affect adaptation in olfactory processing. In principle, longer inhalation should drive more activity-dependent plasticity, while higher frequencies should engage mechanisms for response termination and, finally, inter-inhalation times should allow for recovery from adaptation. Respiration is a filter imposed on a stimulus already rich in dynamics, although the stimulus properties that drive a change in respiration remain unclear. This feedback modulation of the sampling strategy is likely accompanied by top-down processes that serve to modulate odor inhalation and odor processing in a task-specific manner (Reisert et al., 2020), and based on the saliency of the specific odor and previous experience (Wesson et al., 2008; Jordan et al., 2018b).
Adaptation of MTCs is to some degree due to adaptation inherited from presynaptic ORNs and further modulated by properties of feedforward synapses (such as depression). However, three other mechanisms might contribute to the adaptive responses of MTCs: (1) cell-intrinsic physiological mechanisms; (2) integration of lateral inputs; and (3) feedback modulation from other brain areas.
MTCs express many different receptors and channels which underlie diverse intrinsic biophysical and functional properties (Angelo and Margrie, 2011; Angelo et al., 2012). Injecting current into MTCs can evoke diverse responses from periodic bursts of firing (Chen and Shepherd, 1997; Desmaisons et al., 1999; Balu et al., 2004), to more sustained spiking activity that gradually declines (Fadool et al., 2011; Tucker et al., 2013; Burton and Urban, 2014). These properties are still visible when synaptic transmission is pharmacologically blocked suggesting that they reflect intrinsic biophysical properties of MTCs (Burton and Urban, 2014). MTCs express the Kv1.3 potassium channel (Fadool et al., 2000, 2004), which can be locked in an inactive state in response to repeated stimulation (Marom and Levitan, 1994) and could play a role in adaptation. However, Kv1.3 knockdown also changes the OB glomerular structure (Fadool et al., 2004), therefore the precise functional role in odor encoding is hard to pinpoint. It would be interesting to know whether differences between mitral and tufted cells can be attributed to different expression levels of this or other channels.
The dynamic evolution of OB odor representations is certainly strongly determined by the dynamics of specific types of lateral inputs. MTC adaptation was reduced by the application of the GABA-A antagonist bicuculine (Margrie et al., 2001), and similarly by the NMDA antagonists MK-801 in vivo (Chaudhury et al., 2010). Moreover, lateral inhibition acts with its own temporal dynamics. For example, synapses between GABAergic granule cells and mitral cells exhibit significant paired-pulse depression for inter-stimulus intervals up to 10 s (Dietz and Murthy, 2005). Understanding the dynamics of different inhibitory neurons and manipulating their connectivity in a cell-specific manner would shed light on their functional role in the context of olfactory adaptation.
Finally, the OB receives feedback projections from other brain areas (Macrides et al., 1981; Luskin and Price, 1983; Petzold et al., 2009; Rothermel et al., 2014; In’t Zandt et al., 2019; Padmanabhan et al., 2019; Schneider et al., 2020), some of which are related to state-dependent modulation (Linster and Cleland, 2016; McIntyre et al., 2017), for example in the context of feeding regulation (Pager et al., 1972; Wu et al., 2020), and therefore are not strictly stimulus-driven. Centrifugal feedback to the bulb can modulate the response to sustained or repeated odor presentation on long timescales, between minutes or days in the context of habituation (Wilson, 2009; Ogg et al., 2018), associative learning (Kiselycznyk et al., 2006), or context-specific behavior (Yamada et al., 2017). Most of these studies, however, focused either on response attenuation or pattern separation in the context of odor discrimination and stimulus salience. An exciting possibility is that behavioral state or behavioral outcome can alter temporal processing of odor stimuli in the OB via these feedback pathways, similarly to behaviorally dependent processing that has been described in the visual system (Maimon, 2011). However, the specific role(s) of centrifugal mechanisms in adaptive coding remains to be investigated.
This review was motivated by the need to define the current understanding of the role of adaptation in olfactory coding and to identify the directions in which further research should be aimed to link cellular and circuit mechanisms to their behavioral function. In other sensory systems, peripheral adaptation mediates a shift of the sensory response to match stimulus statistics (Figure 1A). However, only a handful of studies have experimentally approached olfactory adaptation asking whether it fulfills a similar role. This is likely due to the combinatorial and temporal complexity of the olfactory system. Combinatorial complexity is associated with the large repertoire of odorant receptors, which participate in odor coding when their sensitivity matches the stimulus concentration. Temporal complexity is related to the nature of the stimuli (volatile molecules transported by air flow), as well as to the filtering function applied by respiration in breathing animals. These two special aspects of the olfactory system suggest that we should think about adaptation differently than in other sensory modalities. What can we conclude from these observations? First, the computational role of peripheral ORN adaptation does not seem to be a shift in sensitivity. From electrophysiological studies, strong stimulus intensity, as well as sustained stimulation, reduces ORN responsiveness, either through saturation or adaptation. Although ORN adaptation has been quantified in a relatively small number of experimental paradigms, the data mostly support a model in which ORN adaptation reduces coding capacity by compressing the dynamic range of the response (Figure 1B). In this context, it remains unclear to which degree ORN firing rates encode stimulus intensity or contrast and further experiments are necessary to answer this question. Moreover, mechanisms that had been proposed to mediate a change in response sensitivity have been subsequently associated with response termination, a crucial step to control the temporal precision of odor perception, specifically in breathing animals. The field certainly calls for a better characterization of ORN firing dynamics by means of more complex stimuli that would mimic natural statistics and the modulations imposed by respiration. The possibility to genetically target specific ORNs should further unveil the degree of diversity of responses across the repertoire of receptors.
Our second observation is a degree of inconsistency between ORN response dynamics reported in electrophysiological studies and those quantified in vivo from the corresponding calcium transients in imaging experiments in the bulb (Figure 3). If we were to extrapolate from firing rate properties of single ORNs, we would predict a major rearrangement of ORN population activity depending on the stimulus. For example, the response of the most sensitive glomeruli should be extremely transient because at saturating concentrations ORNs only fire a few spikes and then go silent. Similarly, adaptation to a background should lead to no response from the most sensitive glomeruli. On the contrary, functional calcium imaging studies in the OB reported more robust and less adaptive ORN responses than expected. Although this reflects in some degree methodological differences, we believe that a major role is played by multimodal regulation of presynaptic calcium signaling. Studies in insects have attributed a major role in odor coding to presynaptic processing. In vertebrates, ORN glomerular activity is often considered the input into the olfactory system, however, it should be investigated as the output of the first processing step in olfaction. Specifically, it would be important to clarify how molecular mechanisms for ORN response adaptation and termination identified in vitro affect calcium dynamics in the bulb in vivo, especially in the context of combinatorial coding and breathing modulation. Additionally, the dynamics and adaptive properties of lateral inputs on ORN presynaptic terminals deserve further investigation.
Our third observation is that coding principles of single MTC responses hardly generalize to other MTCs. This population of cells is diverse not only intrinsically (mitral and tufted cells are genetically different) but also functionally. The function of single MTCs depends on the specific stimulus used and the animal’s state (e.g., respiration) and therefore can be only interpreted with respect to the whole population. In this context, asking whether single MTCs adapt to sustained stimuli by shifting or by compressing their dynamic range might not be the right question. One possibility is that MTCs can be flexibly assigned to different subpopulations with distinct functions: reporting breathing rate, encoding odor identity, or changes in concentration. These functional differences should be associated with different temporal properties and possibly regulated by the activation of lateral inputs in a stimulus-specific manner. This points to the need of analyzing the coding properties of single MTCs while selectively perturbing synaptic inputs.
While the different coding functions of single MTCs might reflect the way in which they integrate synaptic inputs on short timescales (<1 s), there is plenty of evidence that MTC responses evolve over longer times (1–10 s) with a consequent rearrangement of the combinatorial representation. The timescales on which these changes occur depend on breathing rate, indicating that they are indeed stimulus-driven and controllable by the animal (Figure 5). This poses two important questions: what is changing in terms of information content (about the current stimulus) and what is changing in terms of coding capacity (of future stimuli) at the population level? One possibility is that these adaptive changes mediate task-specific categorization of the odor representation. For example, in morphing experiments, adaptive changes can support the formation of mixture categories, in odor-background segregation tasks the identification of the stimulus of relevance, and in learning experiments the separation of rewarded from not-rewarded stimuli. These situations will differentially enroll stimulus-driven feedforward mechanisms (synaptic depression and lateral local inputs in the OB) and state-driven top-down regulation (from cortical areas to the OB). While tracking an odor cue to the source, modulation of sniffing could be used to better separate the target odor from a background by tuning the degree of adaptation in the OB circuit and following the increasing gradient as encoded in MTC subpopulations. Understanding the physiological bases and context-dependent role(s) of these adaptive mechanisms remains the major goal in the field.
CM and DS both contributed to writing of the article. All authors contributed to the article and approved the submitted version.
This work was supposed by DFG grant MA 7804/2-1, NIH Grant DC016133, an award from the State of Florida’s Consortium for Medical Marijuana Clinical Outcomes Research, and internal funds from Florida State University and The University of Mainz.
The authors declare that the research was conducted in the absence of any commercial or financial relationships that could be construed as a potential conflict of interest.
All claims expressed in this article are solely those of the authors and do not necessarily represent those of their affiliated organizations, or those of the publisher, the editors and the reviewers. Any product that may be evaluated in this article, or claim that may be made by its manufacturer, is not guaranteed or endorsed by the publisher.
Thanks to Lawrence Cohen for helpful comments on an early draft of the manuscript, and Mr. Charles Badland for artistic assistance.
Albeanu, D. F., Provost, A. C., Agarwal, P., Soucy, E. R., Zak, J. D., and Murthy, V. N. (2018). Olfactory marker protein (OMP) regulates formation and refinement of the olfactory glomerular map. Nat. Commun. 9:5073. doi: 10.1038/s41467-018-07544-9
Angelo, K., and Margrie, T. W. (2011). Population diversity and function of hyperpolarization-activated current in olfactory bulb mitral cells. Sci. Rep. 1:50. doi: 10.1038/srep00050
Angelo, K., Rancz, E. A., Pimentel, D., Hundahl, C., Hannibal, J., Fleischmann, A., et al. (2012). A biophysical signature of network affiliation and sensory processing in mitral cells. Nature 488, 375–378. doi: 10.1038/nature11291
Aroniadou-Anderjaska, V., Zhou, F. M., Priest, C. A., Ennis, M., and Shipley, M. T. (2000). Tonic and synaptically evoked presynaptic inhibition of sensory input to the rat olfactory bulb via GABA(B) heteroreceptors. J. Neurophysiol. 84, 1194–1203. doi: 10.1152/jn.2000.84.3.1194
Balu, R., Larimer, P., and Strowbridge, B. W. (2004). Phasic stimuli evoke precisely timed spikes in intermittently discharging mitral cells. J. Neurophysiol. 92, 743–753. doi: 10.1152/jn.00016.2004
Banerjee, A., Marbach, F., Anselmi, F., Koh, M. S., Davis, M. B., Garcia da Silva, P., et al. (2015). An interglomerular circuit gates glomerular output and implements gain control in the mouse olfactory bulb. Neuron 87, 193–207. doi: 10.1016/j.neuron.2015.06.019
Barlow, H. (1961). “Possible principles underlying the transformations of sensory messages,” in Sensory Communication, ed W. Rosenblith (Cambridge, MA: MIT Press), 217–234.
Bathellier, B., Buhl, D. L., Accolla, R., and Carleton, A. (2008). Dynamic ensemble odor coding in the mammalian olfactory bulb: sensory information at different timescales. Neuron 57, 586–598. doi: 10.1016/j.neuron.2008.02.011
Berkowicz, D. A., and Trombley, P. Q. (2000). Dopaminergic modulation at the olfactory nerve synapse. Brain Res. 855, 90–99. doi: 10.1016/s0006-8993(99)02342-2
Bolding, K. A., and Franks, K. M. (2018). Recurrent cortical circuits implement concentration-invariant odor coding. Science 361:eaat6904. doi: 10.1126/science.aat6904
Boynton, R. M., and Whitten, D. N. (1970). Visual adaptation in monkey cones: recordings of late receptor potentials. Science 170, 1423–1426. doi: 10.1126/science.170.3965.1423
Bozza, T., Feinstein, P., Zheng, C., and Mombaerts, P. (2002). Odorant receptor expression defines functional units in the mouse olfactory system. J. Neurosci. 22, 3033–3043. doi: 10.1523/JNEUROSCI.22-08-03033.2002
Bozza, T., McGann, J. P., Mombaerts, P., and Wachowiak, M. (2004). In vivo imaging of neuronal activity by targeted expression of a genetically encoded probe in the mouse. Neuron 42, 9–21. doi: 10.1016/s0896-6273(04)00144-8
Bradley, J., Reuter, D., and Frings, S. (2001). Facilitation of calmodulin-mediated odor adaptation by cAMP-gated channel subunits. Science 294, 2176–2178. doi: 10.1126/science.1063415
Brandão, S. C., Silies, M., and Martelli, C. (2021). Adaptive temporal processing of odor stimuli. Cell Tissue Res. 383, 125–141. doi: 10.1007/s00441-020-03400-9
Brenner, N., Bialek, W., and de Ruyter van Steveninck, R. (2000). Adaptive rescaling maximizes information transmission. Neuron 26, 695–702. doi: 10.1016/s0896-6273(00)81205-2
Buck, L., and Axel, R. (1991). A novel multigene family may encode odorant receptors: a molecular basis for odor recognition. Cell 65, 175–187. doi: 10.1016/0092-8674(91)90418-x
Burton, S. D. (2017). Inhibitory circuits of the mammalian main olfactory bulb. J. Neurophysiol. 118, 2034–2051. doi: 10.1152/jn.00109.2017
Burton, S. D., and Urban, N. N. (2014). Greater excitability and firing irregularity of tufted cells underlies distinct afferent-evoked activity of olfactory bulb mitral and tufted cells. J. Physiol. 592, 2097–2118. doi: 10.1113/jphysiol.2013.269886
Cang, J., and Isaacson, J. S. (2003). In vivo whole-cell recording of odor-evoked synaptic transmission in the rat olfactory bulb. J. Neurosci. 23, 4108–4116. doi: 10.1523/JNEUROSCI.23-10-04108.2003
Carandini, M., and Heeger, D. J. (2011). Normalization as a canonical neural computation. Nat. Rev. Neurosci. 13, 51–62. doi: 10.1038/nrn3136
Carey, R. M., Verhagen, J. V., Wesson, D. W., Pírez, N., and Wachowiak, M. (2009). Temporal structure of receptor neuron input to the olfactory bulb imaged in behaving rats. J. Neurophysiol. 101, 1073–1088. doi: 10.1152/jn.90902.2008
Carey, R. M., and Wachowiak, M. (2011). Effect of sniffing on the temporal structure of mitral/tufted cell output from the olfactory bulb. J. Neurosci. 31, 10615–10626. doi: 10.1523/JNEUROSCI.1805-11.2011
Catania, K. C. (2013). Stereo and serial sniffing guide navigation to an odor source in a mammal. Nat. Commun. 4:1441. doi: 10.1038/ncomms2444
Celani, A., Villermaux, E., and Vergassola, M. (2014). Odor landscapes in turbulent environments. Phys. Rev. X 4:4. doi: 10.1103/physrevx.4.041015
Chalansonnet, M., and Chaput, M. A. (1998). Olfactory bulb output cell temporal response patterns to increasing odor concentrations in freely breathing rats. Chem. Senses 23, 1–9. doi: 10.1093/chemse/23.1.1
Chaput, M. A., and Lankheet, M. J. (1987). Influence of stimulus intensity on the categories of single-unit responses recorded from olfactory bulb neurons in awake freely-breathing rabbits. Physiol. Behav. 40, 453–462. doi: 10.1016/0031-9384(87)90030-8
Chaudhury, D., Manella, L., Arellanos, A., Escanilla, O., Cleland, T. A., and Linster, C. (2010). Olfactory bulb habituation to odor stimuli. Behav. Neurosci. 124, 490–499. doi: 10.1037/a0020293
Chen, W. R., and Shepherd, G. M. (1997). Membrane and synaptic properties of mitral cells in slices of rat olfactory bulb. Brain Res. 745, 189–196. doi: 10.1016/s0006-8993(96)01150-x
Chen, T. Y., and Yau, K. W. (1994). Direct modulation by Ca2+-calmodulin of cyclic nucleotide-activated channel of rat olfactory receptor neurons. Nature 368, 545–548. doi: 10.1038/368545a0
Chepesiuk, R. (2005). Decibel hell: the effects of living in a noisy world. Environ. Health Perspect. 113, A34–A41. doi: 10.1289/ehp.113-a34
Cleland, T. A., and Borthakur, A. (2020). A systematic framework for olfactory bulb signal transformations. Front. Comput. Neurosci. 14:579143. doi: 10.3389/fncom.2020.579143
Cleland, T. A., Chen, S.-Y., Hozer, K. W., Ukatu, H. N., Wong, K. J., and Zheng, F. (2011). Sequential mechanisms underlying concentration invariance in biological olfaction. Front. Neuroeng. 4:21. doi: 10.3389/fneng.2011.00021
Cleland, T. A., and Linster, C. (1999). Concentration tuning mediated by spare receptor capacity in olfactory sensory neurons: a theoretical study. Neural Comput. 11, 1673–1690. doi: 10.1162/089976699300016188
Cleland, T. A., and Linster, C. (2012). On-center/inhibitory-surround decorrelation via intraglomerular inhibition in the olfactory bulb glomerular layer. Front. Integr. Neurosci. 6:5. doi: 10.3389/fnint.2012.00005
Cleland, T. A., and Sethupathy, P. (2006). Non-topographical contrast enhancement in the olfactory bulb. BMC Neurosci. 7:7. doi: 10.1186/1471-2202-7-7
Connor, E. G., McHugh, M. K., and Crimaldi, J. P. (2018). Quantification of airborne odor plumes using planar laser-induced fluorescence. Exp. Fluids 59:9. doi: 10.1007/s00348-018-2591-3
Courtiol, E., Amat, C., Thévenet, M., Messaoudi, B., Garcia, S., and Buonviso, N. (2011a). Reshaping of bulbar odor response by nasal flow rate in the rat. PLoS One 6:e16445. doi: 10.1371/journal.pone.0016445
Courtiol, E., Hegoburu, C., Litaudon, P., Garcia, S., Fourcaud-Trocmé, N., and Buonviso, N. (2011b). Individual and synergistic effects of sniffing frequency and flow rate on olfactory bulb activity. J. Neurophysiol. 106, 2813–2824. doi: 10.1152/jn.00672.2011
Dalton, P., and Wysocki, C. J. (1996). The nature and duration of adaptation following long-term odor exposure. Percept. Psychophys. 58, 781–792. doi: 10.3758/bf03213109
Danciger, E., Mettling, C., Vidal, M., Morris, R., and Margolis, F. (1989). Olfactory marker protein gene: its structure and olfactory neuron-specific expression in transgenic mice. Proc. Natl. Acad. Sci. U S A 86, 8565–8569. doi: 10.1073/pnas.86.21.8565
Dean, I., Harper, N. S., and McAlpine, D. (2005). Neural population coding of sound level adapts to stimulus statistics. Nat. Neurosci. 8, 1684–1689. doi: 10.1038/nn1541
Desmaisons, D., Vincent, J. D., and Lledo, P. M. (1999). Control of action potential timing by intrinsic subthreshold oscillations in olfactory bulb output neurons. J. Neurosci. 19, 10727–10737. doi: 10.1523/JNEUROSCI.19-24-10727.1999
Dewan, A., Cichy, A., Zhang, J., Miguel, K., Feinstein, P., Rinberg, D., et al. (2018). Single olfactory receptors set odor detection thresholds. Nat. Commun. 9:2887. doi: 10.1038/s41467-018-05129-0
Díaz-Quesada, M., Youngstrom, I. A., Tsuno, Y., Hansen, K. R., Economo, M. N., and Wachowiak, M. (2018). Inhalation frequency controls reformatting of mitral/tufted cell odor representations in the olfactory bulb. J. Neurosci. 38, 2189–2206. doi: 10.1523/JNEUROSCI.0714-17.2018
Dietz, S. B., and Murthy, V. N. (2005). Contrasting short-term plasticity at two sides of the mitral-granule reciprocal synapse in the mammalian olfactory bulb. J. Physiol. 569, 475–488. doi: 10.1113/jphysiol.2005.095844
Dougherty, D. P., Wright, G. A., and Yew, A. C. (2005). Computational model of the cAMP-mediated sensory response and calcium-dependent adaptation in vertebrate olfactory receptor neurons. Proc. Natl. Acad. Sci. U S A 102, 10415–10420. doi: 10.1073/pnas.0504099102
Døving, K. B. (1987). Response properties of neurones in the rat olfactory bulb to various parameters of odor stimulation. Acta Physiol. Scand. 130, 285–298. doi: 10.1111/j.1748-1716.1987.tb08139.x
Duchamp-Viret, P., and Chaput, M. (2018). In vivo electrophysiological recordings of olfactory receptor neuron units and electro-olfactograms in anesthetized rats. Methods Mol. Biol. 1820, 123–135. doi: 10.1007/978-1-4939-8609-5_10
Duchamp-Viret, P., Chaput, M. A., and Duchamp, A. (1999). Odor response properties of rat olfactory receptor neurons. Science 284, 2171–2174. doi: 10.1126/science.284.5423.2171
Duchamp-Viret, P., Duchamp, A., and Chaput, M. A. (2003). Single olfactory sensory neurons simultaneously integrate the components of an odor mixture. Eur. J. Neurosci. 18, 2690–2696. doi: 10.1111/j.1460-9568.2003.03001.x
Economo, M. N., Hansen, K. R., and Wachowiak, M. (2016). Control of mitral/tufted cell output by selective inhibition among olfactory bulb glomeruli. Neuron 91, 397–411. doi: 10.1016/j.neuron.2016.06.001
Eiting, T. P., and Wachowiak, M. (2020). Differential impacts of repeated sampling on odor representations by genetically-defined mitral and tufted cell subpopulations in the mouse olfactory bulb. J. Neurosci. 40, 6177–6188. doi: 10.1523/JNEUROSCI.0258-20.2020
Ennis, M., Zhou, F. M., Ciombor, K. J., Aroniadou-Anderjaska, V., Hayar, A., Borrelli, E., et al. (2001). Dopamine D2 receptor-mediated presynaptic inhibition of olfactory nerve terminals. J. Neurophysiol. 86, 2986–2997. doi: 10.1152/jn.2001.86.6.2986
Fadool, D. A., Tucker, K., and Pedarzani, P. (2011). Mitral cells of the olfactory bulb perform metabolic sensing and are disrupted by obesity at the level of the Kv1.3 ion channel. PLoS One 6:e24921. doi: 10.1371/journal.pone.0024921
Fadool, D. A., Tucker, K., Perkins, R., Fasciani, G., Thompson, R. N., Parsons, A. D., et al. (2004). Kv1.3 channel gene-targeted deletion produces “Super-Smeller Mice” with altered glomeruli, interacting scaffolding proteins, and biophysics. Neuron 41, 389–404. doi: 10.1016/s0896-6273(03)00844-4
Fadool, D. A., Tucker, K., Phillips, J. J., and Simmen, J. A. (2000). Brain insulin receptor causes activity-dependent current suppression in the olfactory bulb through multiple phosphorylation of Kv1.3. J. Neurophysiol. 83, 2332–2348. doi: 10.1152/jn.2000.83.4.2332
Fain, G. L., Matthews, H. R., Cornwall, M. C., and Koutalos, Y. (2001). Adaptation in vertebrate photoreceptors. Physiol. Rev. 81, 117–151. doi: 10.1152/physrev.2001.81.1.117
Fantana, A. L., Soucy, E. R., and Meister, M. (2008). Rat olfactory bulb mitral cells receive sparse glomerular inputs. Neuron 59, 802–814. doi: 10.1016/j.neuron.2008.07.039
Farbman, A. I., and Margolis, F. L. (1980). Olfactory marker protein during ontogeny: immunohistochemical localization. Dev. Biol. 74, 205–215. doi: 10.1016/0012-1606(80)90062-7
Findley, T. M., Wyrick, D. G., Cramer, J. L., Brown, M. A., Holcomb, B., Attey, R., et al. (2021). Sniff-synchronized, gradient-guided olfactory search by freely moving mice. eLife 10:e58523. doi: 10.7554/eLife.58523
Firestein, S., Picco, C., and Menini, A. (1993). The relation between stimulus and response in olfactory receptor cells of the tiger salamander. J. Physiol. 468, 1–10. doi: 10.1113/jphysiol.1993.sp019756
Fried, H. U., Fuss, S. H., and Korsching, S. I. (2002). Selective imaging of presynaptic activity in the mouse olfactory bulb shows concentration and structure dependence of odor responses in identified glomeruli. Proc. Natl. Acad. Sci. U S A 99, 3222–3227. doi: 10.1073/pnas.052658399
Friedrich, R. W., and Korsching, S. I. (1997). Combinatorial and chemotopic odorant coding in the zebrafish olfactory bulb visualized by optical imaging. Neuron 18, 737–752. doi: 10.1016/s0896-6273(00)80314-1
Friedrich, R. W., and Wiechert, M. T. (2014). Neuronal circuits and computations: pattern decorrelation in the olfactory bulb. FEBS Lett. 588, 2504–2513. doi: 10.1016/j.febslet.2014.05.055
Geffen, M. N., Broome, B. M., Laurent, G., and Meister, M. (2009). Neural encoding of rapidly fluctuating odors. Neuron 61, 570–586. doi: 10.1016/j.neuron.2009.01.021
Getchell, T. V., and Shepherd, G. M. (1978a). Adaptive properties of olfactory receptors analysed with odor pulses of varying durations. J. Physiol. 282, 541–560. doi: 10.1113/jphysiol.1978.sp012480
Getchell, T. V., and Shepherd, G. M. (1978b). Responses of olfactory receptor cells to step pulses of odor at different concentrations in the salamander. J. Physiol. 282, 521–540. doi: 10.1113/jphysiol.1978.sp012479
Ghatpande, A. S., and Reisert, J. (2011). Olfactory receptor neuron responses coding for rapid odor sampling. J. Physiol. 589, 2261–2273. doi: 10.1113/jphysiol.2010.203687
Gire, D. H., and Schoppa, N. E. (2009). Control of on/off glomerular signaling by a local GABAergic microcircuit in the olfactory bulb. J. Neurosci. 29, 13454–13464. doi: 10.1523/JNEUROSCI.2368-09.2009
Gottfried, J. A. (2010). Central mechanisms of odor object perception. Nat. Rev. Neurosci. 11, 628–641. doi: 10.1038/nrn2883
Grosmaitre, X., Vassalli, A., Mombaerts, P., Shepherd, G. M., and Ma, M. (2006). Odorant responses of olfactory sensory neurons expressing the odorant receptor MOR23: a patch clamp analysis in gene-targeted mice. Proc. Natl. Acad. Sci. U S A 103, 1970–1975. doi: 10.1073/pnas.0508491103
Gupta, P., Albeanu, D. F., and Bhalla, U. S. (2015). Olfactory bulb coding of odors, mixtures and sniffs is a linear sum of odor time profiles. Nat. Neurosci. 18, 272–281. doi: 10.1038/nn.3913
Hamilton, K. A., and Kauer, J. S. (1989). Patterns of intracellular potentials in salamander mitral/tufted cells in response to odor stimulation. J. Neurophysiol. 62, 609–625. doi: 10.1152/jn.1989.62.3.609
Homma, R., Cohen, L. B., Kosmidis, E. K., and Youngentob, S. L. (2009). Perceptual stability during dramatic changes in olfactory bulb activation maps and dramatic declines in activation amplitudes. Eur. J. Neurosci. 29, 1027–1034. doi: 10.1111/j.1460-9568.2009.06644.x
Igarashi, K. M., Ieki, N., An, M., Yamaguchi, Y., Nagayama, S., Kobayakawa, K., et al. (2012). Parallel mitral and tufted cell pathways route distinct odor information to different targets in the olfactory cortex. J. Neurosci. 32, 7970–7985. doi: 10.1523/JNEUROSCI.0154-12.2012
Imamura, F., Ito, A., and LaFever, B. J. (2020). Subpopulations of projection neurons in the olfactory bulb. Front. Neural Circuits 14:561822. doi: 10.3389/fncir.2020.561822
In’t Zandt, E. E., Cansler, H. L., Denson, H. B., and Wesson, D. W. (2019). Centrifugal innervation of the olfactory bulb: a reappraisal. eNeuro 6:ENEURO.0390-18.2019. doi: 10.1523/ENEURO.0390-18.2019
Inagaki, S., Iwata, R., and Imai, T. (2021). In vivo optical access to olfactory sensory neurons in the mouse olfactory epithelium. Bioprotocol 11:e4055. doi: 10.21769/bioprotoc.4055
Inagaki, S., Iwata, R., Iwamoto, M., and Imai, T. (2020). Widespread inhibition, antagonism, and synergy in mouse olfactory sensory neurons in vivo. Cell Rep. 31:107814. doi: 10.1016/j.celrep.2020.107814
Jordan, R., Fukunaga, I., Kollo, M., and Schaefer, A. T. (2018a). Active sampling state dynamically enhances olfactory bulb odor representation. Neuron 98, 1214.e5–1228.e5. doi: 10.1016/j.neuron.2018.05.016
Jordan, R., Kollo, M., and Schaefer, A. T. (2018b). Sniffing fast: paradoxical effects on odor concentration discrimination at the levels of olfactory bulb output and behavior. eNeuro 5:10.1523/ENEURO.0148-18.2018. doi: 10.1523/ENEURO.0148-18.2018
Kadohisa, M., and Wilson, D. A. (2006). Olfactory cortical adaptation facilitates detection of odors against background. J. Neurophysiol. 95, 1888–1896. doi: 10.1152/jn.00812.2005
Kass, M. D., Guang, S. A., Moberly, A. H., and McGann, J. P. (2016). Changes in olfactory sensory neuron physiology and olfactory perceptual learning after odorant exposure in adult mice. Chem. Senses 41, 123–133. doi: 10.1093/chemse/bjv065
Kauer, J. S. (1974). Response patterns of amphibian olfactory bulb neurones to odor stimulation. J. Physiol. 243, 695–715. doi: 10.1113/jphysiol.1974.sp010772
Kauer, J. S., and Shepherd, G. M. (1977). Analysis of the onset phase of olfactory bulb unit responses to odor pulses in the salamander. J. Physiol. 272, 495–516. doi: 10.1113/jphysiol.1977.sp012056
Kazama, H., and Wilson, R. I. (2008). Homeostatic matching and nonlinear amplification at identified central synapses. Neuron 58, 401–413. doi: 10.1016/j.neuron.2008.02.030
Khan, A. G., Thattai, M., and Bhalla, U. S. (2008). Odor representations in the rat olfactory bulb change smoothly with morphing stimuli. Neuron 57, 571–585. doi: 10.1016/j.neuron.2008.01.008
Kiselycznyk, C. L., Zhang, S., and Linster, C. (2006). Role of centrifugal projections to the olfactory bulb in olfactory processing. Learn. Mem. 13, 575–579. doi: 10.1101/lm.285706
Kleene, S. J. (2008). The electrochemical basis of odor transduction in vertebrate olfactory cilia. Chem. Senses 33, 839–859. doi: 10.1093/chemse/bjn048
Koldaeva, A., Schaefer, A. T., and Fukunaga, I. (2019). Rapid task-dependent tuning of the mouse olfactory bulb. eLife 8:e43558. doi: 10.7554/eLife.43558
Korsching, S. (2005). Selective imaging of the receptor neuron population in the olfactory bulb of zebrafish and mice. Chem. Senses 30, i101–i102. doi: 10.1093/chemse/bjh134
Koster, N. L., Norman, A. B., Richtand, N. M., Nickell, W. T., Puche, A. C., Pixley, S. K., et al. (1999). Olfactory receptor neurons express D2 dopamine receptors. J. Comp. Neurol. 411, 666–673. doi: 10.1002/(sici)1096-9861(19990906)411:4<666::aid-cne10>3.0.co;2-s
Koulakov, A. A., and Rinberg, D. (2011). Sparse incomplete representations: a potential role of olfactory granule cells. Neuron 72, 124–136. doi: 10.1016/j.neuron.2011.07.031
Kurahashi, T., and Menini, A. (1997). Mechanism of odorant adaptation in the olfactory receptor cell. Nature 385, 725–729. doi: 10.1038/385725a0
Lecoq, J., Tiret, P., and Charpak, S. (2009). Peripheral adaptation codes for high odor concentration in glomeruli. J. Neurosci. 29, 3067–3072. doi: 10.1523/JNEUROSCI.6187-08.2009
Leinders-Zufall, T., Greer, C. A., Shepherd, G. M., and Zufall, F. (1998). Imaging odor-induced calcium transients in single olfactory cilia: specificity of activation and role in transduction. J. Neurosci. 18, 5630–5639. doi: 10.1523/JNEUROSCI.18-15-05630.1998
Leinders-Zufall, T., Ma, M., and Zufall, F. (1999). Impaired odor adaptation in olfactory receptor neurons after inhibition of Ca2+/calmodulin kinase II. J. Neurosci. 19:RC19. doi: 10.1523/JNEUROSCI.19-14-j0005.1999
Leinders-Zufall, T., Rand, M. N., Shepherd, G. M., Greer, C. A., and Zufall, F. (1997). Calcium entry through cyclic nucleotide-gated channels in individual cilia of olfactory receptor cells: spatiotemporal dynamics. J. Neurosci. 17, 4136–4148. doi: 10.1523/JNEUROSCI.17-11-04136.1997
Linster, C., and Cleland, T. A. (2016). Neuromodulation of olfactory transformations. Curr. Opin. Neurobiol. 40, 170–177. doi: 10.1016/j.conb.2016.07.006
Linster, C., Henry, L., Kadohisa, M., and Wilson, D. A. (2007). Synaptic adaptation and odor-background segmentation. Neurobiol. Learn. Mem. 87, 352–360. doi: 10.1016/j.nlm.2006.09.011
Luskin, M. B., and Price, J. L. (1983). The topographic organization of associational fibers of the olfactory system in the rat, including centrifugal fibers to the olfactory bulb. J. Comp. Neurol. 216, 264–291. doi: 10.1002/cne.902160305
Ma, M., Chen, W. R., and Shepherd, G. M. (1999). Electrophysiological characterization of rat and mouse olfactory receptor neurons from an intact epithelial preparation. J. Neurosci. Methods 92, 31–40. doi: 10.1016/s0165-0270(99)00089-8
Ma, M., and Shepherd, G. M. (2000). Functional mosaic organization of mouse olfactory receptor neurons. Proc. Natl. Acad. Sci. U S A 97, 12869–12874. doi: 10.1073/pnas.220301797
Macrides, F., Davis, B. J., Youngs, W. M., Nadi, N. S., and Margolis, F. L. (1981). Cholinergic and catecholaminergic afferents to the olfactory bulb in the hamster: a neuroanatomical, biochemical, and histochemical investigation. J. Comp. Neurol. 203, 495–514. doi: 10.1002/cne.902030311
Maimon, G. (2011). Modulation of visual physiology by behavioral state in monkeys, mice, and flies. Curr. Opin. Neurobiol. 21, 559–564. doi: 10.1016/j.conb.2011.05.001
Mair, R. G. (1982). Response properties of rat olfactory bulb neurones. J. Physiol. 326, 341–359. doi: 10.1113/jphysiol.1982.sp014197
Margrie, T. W., Sakmann, B., and Urban, N. N. (2001). Action potential propagation in mitral cell lateral dendrites is decremental and controls recurrent and lateral inhibition in the mammalian olfactory bulb. Proc. Natl. Acad. Sci. U S A 98, 319–324. doi: 10.1073/pnas.011523098
Marom, S., and Levitan, I. B. (1994). State-dependent inactivation of the Kv3 potassium channel. Biophys. J. 67, 579–589. doi: 10.1016/S0006-3495(94)80517-X
Martelli, C., Carlson, J. R., and Emonet, T. (2013). Intensity invariant dynamics and odor-specific latencies in olfactory receptor neuron response. J. Neurosci. 33, 6285–6297. doi: 10.1523/JNEUROSCI.0426-12.2013
Martelli, C., and Fiala, A. (2019). Slow presynaptic mechanisms that mediate adaptation in the olfactory pathway of Drosophila. eLife 8:e43735. doi: 10.7554/eLife.43735
Mathews, D. F. (1972). Response patterns of single units in the olfactory bulb of the rat to odor. Brain Res. 47, 389–400. doi: 10.1016/0006-8993(72)90647-6
McGann, J. P. (2013). Presynaptic inhibition of olfactory sensory neurons: new mechanisms and potential functions. Chem. Senses 38, 459–474. doi: 10.1093/chemse/bjt018
McGann, J. P., Pirez, N., Gainey, M. A., Muratore, C., Elias, A. S., and Wachowiak, M. (2005). Odorant representations are modulated by intra- but not interglomerular presynaptic inhibition of olfactory sensory neurons. Neuron 48, 1039–1053. doi: 10.1016/j.neuron.2005.10.031
McIntyre, J. C., Thiebaud, N., McGann, J. P., Komiyama, T., and Rothermel, M. (2017). Neuromodulation in chemosensory pathways. Chem. Senses 42, 375–379. doi: 10.1093/chemse/bjx014
Menini, A., Picco, C., and Firestein, S. (1995). Quantal-like current fluctuations induced by odorants in olfactory receptor cells. Nature 373, 435–437. doi: 10.1038/373435a0
Meredith, M. (1986). Patterned response to odor in mammalian olfactory bulb: the influence of intensity. J. Neurophysiol. 56, 572–597. doi: 10.1152/jn.1986.56.3.572
Meredith, M., and Moulton, D. G. (1978). Patterned response to odor in single neurones of goldfish olfactory bulb: influence of odor quality and other stimulus parameters. J. Gen. Physiol. 71, 615–643. doi: 10.1085/jgp.71.6.615
Moran, A. K., Eiting, T. P., and Wachowiak, M. (2019). Diverse dynamics of glutamatergic input underlie heterogeneous response patterns of olfactory bulb outputs in vivo. BioRxiv [Preprint]. doi: 10.1101/692574
Motokizawa, F. (1996). Odor representation and discrimination in mitral/tufted cells of the rat olfactory bulb. Exp. Brain Res. 112, 24–34. doi: 10.1007/BF00227174
Murphy, G. J., Glickfeld, L. L., Balsen, Z., and Isaacson, J. S. (2004). Sensory neuron signaling to the brain: properties of transmitter release from olfactory nerve terminals. J. Neurosci. 24, 3023–3030. doi: 10.1523/JNEUROSCI.5745-03.2004
Murphy, G. J., and Isaacson, J. S. (2003). Presynaptic cyclic nucleotide-gated ion channels modulate neurotransmission in the mammalian olfactory bulb. Neuron 37, 639–647. doi: 10.1016/s0896-6273(03)00057-6
Nagayama, S., Homma, R., and Imamura, F. (2014). Neuronal organization of olfactory bulb circuits. Front. Neural Circuits 8:98. doi: 10.3389/fncir.2014.00098
Nagayama, S., Takahashi, Y. K., Yoshihara, Y., and Mori, K. (2004). Mitral and tufted cells differ in the decoding manner of odor maps in the rat olfactory bulb. J. Neurophysiol. 91, 2532–2540. doi: 10.1152/jn.01266.2003
Nagel, K. I., and Doupe, A. J. (2006). Temporal processing and adaptation in the songbird auditory forebrain. Neuron 51, 845–859. doi: 10.1016/j.neuron.2006.08.030
Nagel, K. I., Hong, E. J., and Wilson, R. I. (2015). Synaptic and circuit mechanisms promoting broadband transmission of olfactory stimulus dynamics. Nat. Neurosci. 18, 56–65. doi: 10.1038/nn.3895
Ngai, J., Chess, A., Dowling, M. M., Necles, N., Macagno, E. R., and Axel, R. (1993). Coding of olfactory information: topography of odorant receptor expression in the catfish olfactory epithelium. Cell 72, 667–680. doi: 10.1016/0092-8674(93)90396-8
Nickell, W. T., Behbehani, M. M., and Shipley, M. T. (1994). Evidence for GABAB-mediated inhibition of transmission from the olfactory nerve to mitral cells in the rat olfactory bulb. Brain Res. Bull. 35, 119–123. doi: 10.1016/0361-9230(94)90091-4
Nickell, W. T., Norman, A. B., Wyatt, L. M., and Shipley, M. T. (1991). Olfactory bulb DA receptors may be located on terminals of the olfactory nerve. Neuroreport 2, 9–12. doi: 10.1097/00001756-199101000-00002
Niessing, J., and Friedrich, R. W. (2010). Olfactory pattern classification by discrete neuronal network states. Nature 465, 47–52. doi: 10.1038/nature08961
Normann, R. A., and Perlman, I. (1979). The effects of background illumination on the photoresponses of red and green cones. J. Physiol. 286, 491–507. doi: 10.1113/jphysiol.1979.sp012633
Ogg, M. C., Bendahamane, M., and Fletcher, M. L. (2015). Habituation of glomerular responses in the olfactory bulb following prolonged odor stimulation reflects reduced peripheral input. Front. Mol. Neurosci. 8:53. doi: 10.3389/fnmol.2015.00053
Ogg, M. C., Ross, J. M., Bendahmane, M., and Fletcher, M. L. (2018). Olfactory bulb acetylcholine release dishabituates odor responses and reinstates odor investigation. Nat. Commun. 9:1868. doi: 10.1038/s41467-018-04371-w
Olsen, S. R., Bhandawat, V., and Wilson, R. I. (2010). Divisive normalization in olfactory population codes. Neuron 66, 287–299. doi: 10.1016/j.neuron.2010.04.009
Padmanabhan, K., Osakada, F., Tarabrina, A., Kizer, E., Callaway, E. M., Gage, F. H., et al. (2019). Centrifugal inputs to the main olfactory bulb revealed through whole brain circuit-mapping. Front. Neuroanat. 12:115. doi: 10.3389/fnana.2018.00115
Padmanabhan, K., and Urban, N. N. (2010). Intrinsic biophysical diversity decorrelates neuronal firing while increasing information content. Nat. Neurosci. 13, 1276–1282. doi: 10.1038/nn.2630
Pager, J., Giachetti, I., Holley, A., and Le Magnen, J. (1972). A selective control of olfactory bulb electrical activity in relation to food deprivation and satiety in rats. Physiol. Behav. 9, 573–579. doi: 10.1016/0031-9384(72)90014-5
Parabucki, A., Bizer, A., Morris, G., Munoz, A. E., Bala, A. D. S., Smear, M., et al. (2019). Odor concentration change coding in the olfactory bulb. eNeuro 6:ENEURO.0396-18.2019. doi: 10.1523/ENEURO.0396-18.2019
Parrish-Aungst, S., Shipley, M. T., Erdelyi, F., Szabo, G., and Puche, A. C. (2007). Quantitative analysis of neuronal diversity in the mouse olfactory bulb. J. Comp. Neurol. 501, 825–836. doi: 10.1002/cne.21205
Patterson, M. A., Lagier, S., and Carleton, A. (2013). Odor representations in the olfactory bulb evolve after the first breath and persist as an odor afterimage. Proc. Natl. Acad. Sci. U S A 110, E3340–E3349. doi: 10.1073/pnas.1303873110
Perlman, I., and Normann, R. A. (1998). Light adaptation and sensitivity controlling mechanisms in vertebrate photoreceptors. Prog. Retin. Eye Res. 17, 523–563. doi: 10.1016/s1350-9462(98)00005-6
Petzold, G. C., Hagiwara, A., and Murthy, V. N. (2009). Serotonergic modulation of odor input to the mammalian olfactory bulb. Nat. Neurosci. 12, 784–791. doi: 10.1038/nn.2335
Pírez, N., and Wachowiak, M. (2008). In vivo modulation of sensory input to the olfactory bulb by tonic and activity-dependent presynaptic inhibition of receptor neurons. J. Neurosci. 28, 6360–6371. doi: 10.1523/JNEUROSCI.0793-08.2008
Platisa, J., Zeng, H., Madisen, L., Cohen, L. B., Pieribone, V. A., and Storace, D. A. (2020). Voltage imaging using transgenic mouse lines expressing the GEVI ArcLight in two olfactory cell types. BioRxiv [Preprint]. doi: 10.1101/2020.08.26.268904
Potter, H., and Chorover, S. L. (1976). Response plasticity in hamster olfactory bulb: peripheral and central processes. Brain Res. 116, 417–429. doi: 10.1016/0006-8993(76)90490-x
Rall, W., Shepherd, G. M., Reese, T. S., and Brightman, M. W. (1966). Dendrodendritic synaptic pathway for inhibition in the olfactory bulb. Exp. Neurol. 14, 44–56. doi: 10.1016/0014-4886(66)90023-9
Reinken, U., and Schmidt, U. (1986). Reactions of olfactory bulb neurons to different stimulus intensities in laboratory mice. Exp. Brain Res. 63, 151–157. doi: 10.1007/BF00235657
Reisert, J., Golden, G. J., Dibattista, M., and Gelperin, A. (2020). Dynamics of odor sampling strategies in mice. PLoS One 15:e0237756. doi: 10.1371/journal.pone.0237756
Reisert, J., and Matthews, H. R. (1999). Adaptation of the odor-induced response in frog olfactory receptor cells. J. Physiol. 519, 801–813. doi: 10.1111/j.1469-7793.1999.0801n.x
Reisert, J., and Matthews, H. R. (2000). Adaptation-induced changes in sensitivity in frog olfactory receptor cells. Chem. Senses 25, 483–486. doi: 10.1093/chemse/25.4.483
Reisert, J., and Matthews, H. R. (2001a). Responses to prolonged odor stimulation in frog olfactory receptor cells. J. Physiol. 534, 179–191. doi: 10.1111/j.1469-7793.2001.t01-1-00179.x
Reisert, J., and Matthews, H. R. (2001b). Response properties of isolated mouse olfactory receptor cells. J. Physiol. 530, 113–122. doi: 10.1111/j.1469-7793.2001.0113m.x
Reisert, J., and Zhao, H. (2011). Perspectives on: information and coding in mammalian sensory physiology: response kinetics of olfactory receptor neurons and the implications in olfactory coding. J. Gen. Physiol. 138, 303–310. doi: 10.1085/jgp.201110645
Ressler, K. J., Sullivan, S. L., and Buck, L. B. (1994). Information coding in the olfactory system: evidence for a stereotyped and highly organized epitope map in the olfactory bulb. Cell 79, 1245–1255. doi: 10.1016/0092-8674(94)90015-9
Rokni, D., Hemmelder, V., Kapoor, V., and Murthy, V. N. (2014). An olfactory cocktail party: figure-ground segregation of odorants in rodents. Nat. Neurosci. 17, 1225–1232. doi: 10.1038/nn.3775
Rothermel, M., Carey, R. M., Puche, A., Shipley, M. T., and Wachowiak, M. (2014). Cholinergic inputs from Basal forebrain add an excitatory bias to odor coding in the olfactory bulb. J. Neurosci. 34, 4654–4664. doi: 10.1523/JNEUROSCI.5026-13.2014
Schild, D., and Restrepo, D. (1998). Transduction mechanisms in vertebrate olfactory receptor cells. Physiol. Rev. 78, 429–466. doi: 10.1152/physrev.1998.78.2.429
Schneider, N. Y., Chaudy, S., Epstein, A. L., Viollet, C., Benani, A., Penicaud, L., et al. (2020). Centrifugal projections to the main olfactory bulb revealed by transsynaptic retrograde tracing in mice. J. Comp. Neurol. 528, 1805–1819. doi: 10.1002/cne.24846
Short, S. M., and Wachowiak, M. (2019). Temporal dynamics of inhalation-linked activity across defined subpopulations of mouse olfactory bulb neurons imaged in vivo. eNeuro 6:ENEURO.0189-19.2019. doi: 10.1523/ENEURO.0189-19.2019
Sirotin, Y. B., Shusterman, R., and Rinberg, D. (2015). Neural coding of perceived odor intensity. eNeuro 2:ENEURO.0083-15.2015. doi: 10.1523/ENEURO.0083-15.2015
Skalicky, S. (2016). Ocular and Visual Physiology. Clinical Application. 1st Edn. Singapore: Springer.
Smith, R. L. (1977). Short-term adaptation in single auditory nerve fibers: some poststimulatory effects. J. Neurophysiol. 40, 1098–1111. doi: 10.1152/jn.1977.40.5.1098
Smith, R. L. (1979). Adaptation, saturation and physiological masking in single auditory-nerve fibers. J. Acoust. Soc. Am. 65, 166–178. doi: 10.1121/1.382260
Smith, R. L., Brachman, M. L., and Goodman, D. A. (1983). Adaptation in the auditory periphery. Ann. N Y Acad. Sci. 405, 79–93. doi: 10.1111/j.1749-6632.1983.tb31621.x
Sobel, E. C., and Tank, D. W. (1993). Timing of odor stimulation does not alter patterning of olfactory bulb unit activity in freely breathing rats. J. Neurophysiol. 69, 1331–1337. doi: 10.1152/jn.1993.69.4.1331
Song, Y., Cygnar, K. D., Sagdullaev, B., Valley, M., Hirsh, S., Stephan, A., et al. (2008). Olfactory CNG channel desensitization by Ca2+/CaM via the B1b subunit affects response termination but not sensitivity to recurring stimulation. Neuron 58, 374–386. doi: 10.1016/j.neuron.2008.02.029
Sosulski, D. L., Bloom, M. L., Cutforth, T., Axel, R., and Datta, S. R. (2011). Distinct representations of olfactory information in different cortical centres. Nature 472, 213–216. doi: 10.1038/nature09868
Storace, D. A., and Cohen, L. B. (2017). Measuring the olfactory bulb input-output transformation reveals a contribution to the perception of odorant concentration invariance. Nat. Commun. 8:81. doi: 10.1038/s41467-017-00036-2
Storace, D. A., and Cohen, L. B. (2019). The olfactory bulb contributes to the adaptation of odor responses: the input-output transformation. BioRxiv [Preprint]. doi: 10.1101/829531
Storace, D. A., Cohen, L. B., and Choi, Y. (2019). Using genetically encoded voltage indicators (GEVIs) to study the input-output transformation of the mammalian olfactory bulb. Front. Cell. Neurosci. 13:342. doi: 10.3389/fncel.2019.00342
Tucker, K., Cho, S., Thiebaud, N., Henderson, M. X., and Fadool, D. A. (2013). Glucose sensitivity of mouse olfactory bulb neurons is conveyed by a voltage-gated potassium channel. J. Physiol. 591, 2541–2561. doi: 10.1113/jphysiol.2013.254086
Uchida, N., and Mainen, Z. F. (2007). Odor concentration invariance by chemical ratio coding. Front. Syst. Neurosci. 1:3. doi: 10.3389/neuro.06.003.2007
Valeton, J. M., and van Norren, D. (1983). Light adaptation of primate cones: an analysis based on extracellular data. Vis. Res. 23, 1539–1547. doi: 10.1016/0042-6989(83)90167-0
Vassar, R., Chao, S. K., Sitcheran, R., Nunez, J. M., Vosshall, L. B., and Axel, R. (1994). Topographic organization of sensory projections to the olfactory bulb. Cell 79, 981–991. doi: 10.1016/0092-8674(94)90029-9
Verhagen, J. V., Wesson, D. W., Netoff, T. I., White, J. A., and Wachowiak, M. (2007). Sniffing controls an adaptive filter of sensory input to the olfactory bulb. Nat. Neurosci. 10, 631–639. doi: 10.1038/nn1892
Viemeister, N. F. (1988). Intensity coding and the dynamic range problem. Hear. Res. 34, 267–274. doi: 10.1016/0378-5955(88)90007-x
Vincis, R., Gschwend, O., Bhaukaurally, K., Beroud, J., and Carleton, A. (2012). Dense representation of natural odorants in the mouse olfactory bulb. Nat. Neurosci. 15, 537–539. doi: 10.1038/nn.3057
Vinograd, A., Livneh, Y., and Mizrahi, A. (2017). History-dependent odor processing in the mouse olfactory bulb. J. Neurosci. 37, 12018–12030. doi: 10.1523/JNEUROSCI.0755-17.2017
Wachowiak, M., and Cohen, L. B. (1998). Presynaptic afferent inhibition of lobster olfactory receptor cells: reduced action-potential propagation into axon terminals. J. Neurophysiol. 80, 1011–1015. doi: 10.1152/jn.1998.80.2.1011
Wachowiak, M., and Cohen, L. B. (1999). Presynaptic inhibition of primary olfactory afferents mediated by different mechanisms in lobster and turtle. J. Neurosci. 19, 8808–8817. doi: 10.1523/JNEUROSCI.19-20-08808.1999
Wachowiak, M., and Cohen, L. B. (2001). Representation of odorants by receptor neuron input to the mouse olfactory bulb. Neuron 32, 723–735. doi: 10.1016/s0896-6273(01)00506-2
Wachowiak, M., Falk, C. X., Cohen, L. B., and Zochowski, M. R. (2002). “Voltage and calcium imaging of brain activity: examples from the turtle and the mouse,” in Brain Mapping: The Methods, 2nd Edition, eds A. W. Toga and J. C. Mazziotta (Amsterdam; Boston: Academic Press), 877.
Wachowiak, M., McGann, J. P., Heyward, P. M., Shao, Z., Puche, A. C., and Shipley, M. T. (2005). Inhibition [corrected] of olfactory receptor neuron input to olfactory bulb glomeruli mediated by suppression of presynaptic calcium influx. J. Neurophysiol. 94, 2700–2712. doi: 10.1152/jn.00286.2005
Wang, H. W., Wysocki, C. J., and Gold, G. H. (1993). Induction of olfactory receptor sensitivity in mice. Science 260, 998–1000. doi: 10.1126/science.8493539
Wark, B., Lundstrom, B. N., and Fairhall, A. (2007). Sensory adaptation. Curr. Opin. Neurobiol. 17, 423–429. doi: 10.1016/j.conb.2007.07.001
Watkins, P. V., and Barbour, D. L. (2008). Specialized neuronal adaptation for preserving input sensitivity. Nat. Neurosci. 11, 1259–1261. doi: 10.1038/nn.2201
Weber, A. I., and Fairhall, A. L. (2019). The role of adaptation in neural coding. Curr. Opin. Neurobiol. 58, 135–140. doi: 10.1016/j.conb.2019.09.013
Wen, B., Wang, G. I., Dean, I., and Delgutte, B. (2009). Dynamic range adaptation to sound level statistics in the auditory nerve. J. Neurosci. 29, 13797–13808. doi: 10.1523/JNEUROSCI.5610-08.2009
Wen, B., Wang, G. I., Dean, I., and Delgutte, B. (2012). Time course of dynamic range adaptation in the auditory nerve. J. Neurophysiol. 108, 69–82. doi: 10.1152/jn.00055.2012
Wesson, D. W., Carey, R. M., Verhagen, J. V., and Wachowiak, M. (2008). Rapid encoding and perception of novel odors in the rat. PLoS Biol. 6:e82. doi: 10.1371/journal.pbio.0060082
Whitmire, C. J., and Stanley, G. B. (2016). Rapid sensory adaptation redux: a circuit perspective. Neuron 92, 298–315. doi: 10.1016/j.neuron.2016.09.046
Wilson, D. A. (1998). Habituation of odor responses in the rat anterior piriform cortex. J. Neurophysiol. 79, 1425–1440. doi: 10.1152/jn.1998.79.3.1425
Wilson, D. A. (2000). Comparison of odor receptive field plasticity in the rat olfactory bulb and anterior piriform cortex. J. Neurophysiol. 84, 3036–3042. doi: 10.1152/jn.2000.84.6.3036
Wilson, D. A. (2009). Olfaction as a model system for the neurobiology of mammalian short-term habituation. Neurobiol. Learn. Mem. 92, 199–205. doi: 10.1016/j.nlm.2008.07.010
Wu, J., Liu, P., Chen, F., Ge, L., Lu, Y., and Li, A. (2020). Excitability of neural activity is enhanced, but neural discrimination of odors is slightly decreased, in the olfactory bulb of fasted mice. Genes 11:433. doi: 10.3390/genes11040433
Xu, L., Li, W., Voleti, V., Zou, D. J., Hillman, E. M. C., and Firestein, S. (2020). Widespread receptor-driven modulation in peripheral olfactory coding. Science 368:eaaz5390. doi: 10.1126/science.aaz5390
Yamada, Y., Bhaukaurally, K., Madarasz, T. J., Pouget, A., Rodriguez, I., and Carleton, A. (2017). Context- and output layer-dependent long-term ensemble plasticity in a sensory circuit. Neuron 93, 1198.e5–1212.e5. doi: 10.1016/j.neuron.2017.02.006
Yokoi, M., Mori, K., and Nakanishi, S. (1995). Refinement of odor molecule tuning by dendrodendritic synaptic inhibition in the olfactory bulb. Proc. Natl. Acad. Sci. U S A 92, 3371–3375. doi: 10.1073/pnas.92.8.3371
Zak, J. D., Reddy, G., Vergassola, M., and Murthy, V. N. (2020). Antagonistic odor interactions in olfactory sensory neurons are widespread in freely breathing mice. Nat. Commun. 11:3350. doi: 10.1038/s41467-020-17124-5
Zapiec, B., and Mombaerts, P. (2015). Multiplex assessment of the positions of odorant receptor-specific glomeruli in the mouse olfactory bulb by serial two-photon tomography. Proc. Natl. Acad. Sci. U S A 112, E5873–E5882. doi: 10.1073/pnas.1512135112
Zufall, F., and Leinders-Zufall, T. (2000). The cellular and molecular basis of odor adaptation. Chem. Senses 25, 473–481. doi: 10.1093/chemse/25.4.473
Keywords: adaptation, olfactory bulb, olfaction, olfactory receptor neurons, mitral and tufted cells
Citation: Martelli C and Storace DA (2021) Stimulus Driven Functional Transformations in the Early Olfactory System. Front. Cell. Neurosci. 15:684742. doi: 10.3389/fncel.2021.684742
Received: 23 March 2021; Accepted: 06 July 2021;
Published: 03 August 2021.
Edited by:
Thor Eysteinsson, University of Iceland, IcelandReviewed by:
Qian Li, Shanghai Jiao Tong University, ChinaCopyright © 2021 Martelli and Storace. This is an open-access article distributed under the terms of the Creative Commons Attribution License (CC BY). The use, distribution or reproduction in other forums is permitted, provided the original author(s) and the copyright owner(s) are credited and that the original publication in this journal is cited, in accordance with accepted academic practice. No use, distribution or reproduction is permitted which does not comply with these terms.
*Correspondence: Douglas Anthony Storace, ZHN0b3JhY2VAZnN1LmVkdQ==
Disclaimer: All claims expressed in this article are solely those of the authors and do not necessarily represent those of their affiliated organizations, or those of the publisher, the editors and the reviewers. Any product that may be evaluated in this article or claim that may be made by its manufacturer is not guaranteed or endorsed by the publisher.
Research integrity at Frontiers
Learn more about the work of our research integrity team to safeguard the quality of each article we publish.