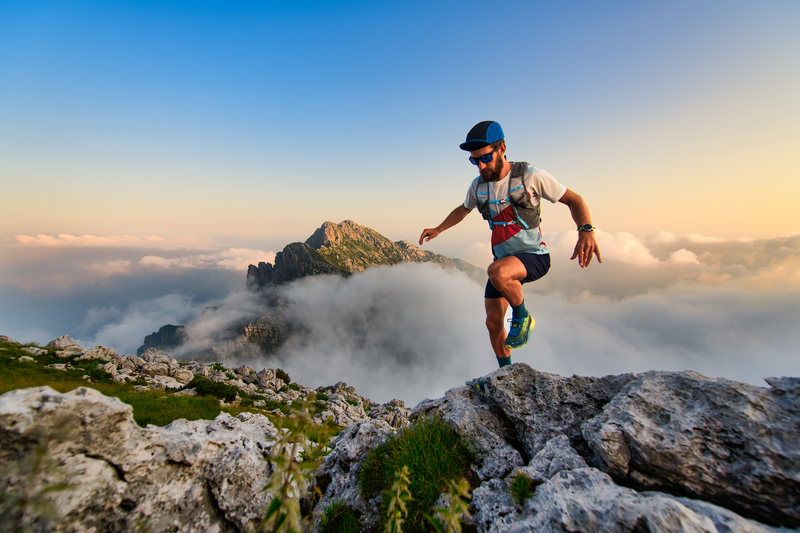
94% of researchers rate our articles as excellent or good
Learn more about the work of our research integrity team to safeguard the quality of each article we publish.
Find out more
ORIGINAL RESEARCH article
Front. Cell. Infect. Microbiol.
Sec. Clinical Microbiology
Volume 15 - 2025 | doi: 10.3389/fcimb.2025.1582553
The final, formatted version of the article will be published soon.
You have multiple emails registered with Frontiers:
Please enter your email address:
If you already have an account, please login
You don't have a Frontiers account ? You can register here
The human vaginal virome is a critical component of the vaginal microbiome, yet its diversity and potential role in health and disease remain poorly understood. In this study, we performed metagenomic sequencing on 24 vaginal swab libraries from 267 women, including both healthy individuals and those diagnosed with vaginitis. Our analysis revealed that DNA viruses predominated in the vaginal virome, with Anelloviridae and Papillomaviridae being the most prevalent eukaryotic viruses, while Siphoviridae and Microviridae were the dominant bacteriophages. Compared to the healthy control group, the vaginitis group exhibited significantly lower alpha diversity (Shannon, Richness, and Pielou indices) and greater beta diversity dispersion, indicating distinct viral community compositions. Anelloviruses, detected in both groups, showed extensive lineage diversity, frequent recombination events, and substantial phylogenetic divergence, suggesting a complex evolutionary history and potential immunological interactions. HPV diversity and richness were significantly higher in the vaginitis group, with a more unbalanced viral lineage distribution. Furthermore, our findings highlighted novel phage-bacterial associations, suggesting a potential role of bacteriophages in modulating the vaginal microbiome.These results provide valuable insights into the composition of the vaginal virome, its relationship with vaginal dysbiosis, and potential implications for reproductive health. Future studies should incorporate individual-level sequencing and metatranscriptomic approaches to explore intra-host viral variability, assess viral activity, and further elucidate the functional roles of the vaginal virome in host-microbiome interactions.
Keywords: Metagenomics, Anellovirus, papillomavirus, Vaginitis, Bacteriophage
Received: 24 Feb 2025; Accepted: 17 Mar 2025.
Copyright: © 2025 Lu, Lu, Zhu, Sun, Chen, Ge, Jiang, Wang, Zhang, Zhang and Dai. This is an open-access article distributed under the terms of the Creative Commons Attribution License (CC BY). The use, distribution or reproduction in other forums is permitted, provided the original author(s) or licensor are credited and that the original publication in this journal is cited, in accordance with accepted academic practice. No use, distribution or reproduction is permitted which does not comply with these terms.
* Correspondence:
Ziyuan Dai, Yancheng Third People's Hospital, Yancheng, China
Disclaimer: All claims expressed in this article are solely those of the authors and do not necessarily represent those of their affiliated organizations, or those of the publisher, the editors and the reviewers. Any product that may be evaluated in this article or claim that may be made by its manufacturer is not guaranteed or endorsed by the publisher.
Research integrity at Frontiers
Learn more about the work of our research integrity team to safeguard the quality of each article we publish.