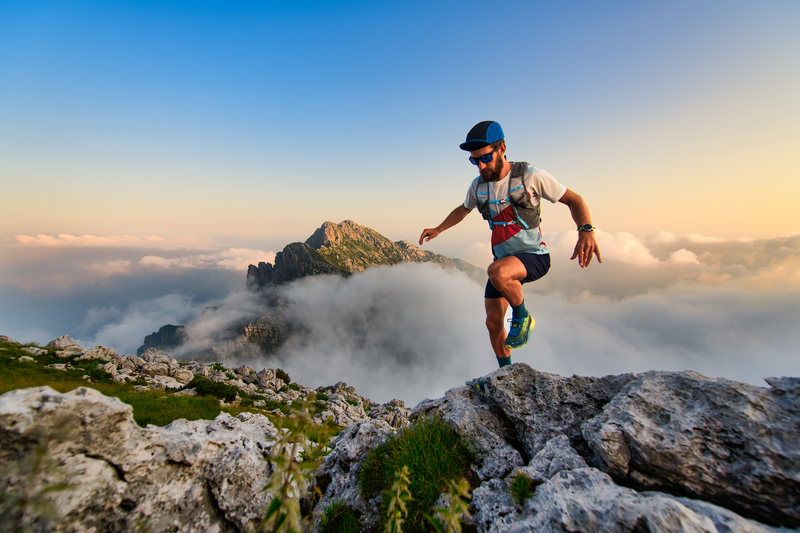
95% of researchers rate our articles as excellent or good
Learn more about the work of our research integrity team to safeguard the quality of each article we publish.
Find out more
ORIGINAL RESEARCH article
Front. Cell. Infect. Microbiol.
Sec. Clinical Infectious Diseases
Volume 15 - 2025 | doi: 10.3389/fcimb.2025.1545979
This article is part of the Research Topic Molecular mechanisms and clinical studies of multi-organ dysfunction in sepsis associated with pathogenic microbial infection View all 9 articles
The final, formatted version of the article will be published soon.
You have multiple emails registered with Frontiers:
Please enter your email address:
If you already have an account, please login
You don't have a Frontiers account ? You can register here
Background: Sepsis associated encephalopathy (SAE) is prevalent among elderly patients in the ICU and significantly affects patient prognosis. Due to the symptom similarity with other neurological disorders and the absence of specific biomarkers, early clinical diagnosis remains challenging. This study aimed to develop a predictive model for SAE in elderly ICU patients.The data of elderly sepsis patients were extracted from the MIMIC IV database (version 3.1) and divided into training and test sets in a 7:3 ratio. Feature variables were selected using the LASSO-Boruta combined algorithm, and five machine learning (ML) models, including Extreme Gradient Boosting (XGBoost), Categorical Boosting ( CatBoost ) ,Light Gradient Boosting Machine (LGBM), Multilayer Perceptron (MLP), and Support Vector Machines (SVM), were subsequently developed using these variables. A comprehensive set of performance metrics was used to assess the predictive accuracy, calibration, and clinical applicability of these models.For the machine learning model with the best performance, we employed the SHapley Additive Explanations(SHAP) method to visualize the model.Based on strict inclusion and exclusion criteria, a total of 3,156 elderly sepsis patients were enrolled in the study, with an SAE incidence rate of 48.7%. The mortality rate of elderly sepsis patients who developed SAE was significantly higher than that of patients in the non-SAE group (28.78% vs. 12.59%, P < 0.001). A total of 18 feature variables were selected for the construction of the ML model using the LASSO-Boruta combined algorithm. Compared to the other four models and traditional scoring systems, the XGBoost model demonstrated the best overall predictive performance, with Area Under the Curve(AUC) =0.898, accuracy=0.830, recall=0.819, specificity=0.840, and Precision=0.821. Furthermore, the results from the Decision Curve Analysis (DCA) and calibration curves demonstrated that the XGBoost model has significant clinical value and stable predictive performance. The ten-fold cross-validation method further confirmed the robustness and generalizability of the model. In addition, we simplified the model based on the SHAP feature importance ranking, and the results indicated that the simplified XGBoost model retains excellent predictive ability (AUC=0.858).The XGBoost model effectively predicts SAE in elderly ICU patients and may serve as a reliable tool for clinicians to identify high-risk patients.
Keywords: machine learning, Early prediction, Sepsis associated encephalopathy, Elderly, MIMIC-IV
Received: 16 Dec 2024; Accepted: 31 Mar 2025.
Copyright: © 2025 Han, Xie, Qiu, Tang, Song, Li and Wu. This is an open-access article distributed under the terms of the Creative Commons Attribution License (CC BY). The use, distribution or reproduction in other forums is permitted, provided the original author(s) or licensor are credited and that the original publication in this journal is cited, in accordance with accepted academic practice. No use, distribution or reproduction is permitted which does not comply with these terms.
* Correspondence:
Xiaodan Wu, Department of Anesthesiology, Fujian Provincial Hospital, Shengli Clinical Medical College of Fujian Medical University, Fujian Medical University, Fuzhou, China
Disclaimer: All claims expressed in this article are solely those of the authors and do not necessarily represent those of their affiliated organizations, or those of the publisher, the editors and the reviewers. Any product that may be evaluated in this article or claim that may be made by its manufacturer is not guaranteed or endorsed by the publisher.
Research integrity at Frontiers
Learn more about the work of our research integrity team to safeguard the quality of each article we publish.