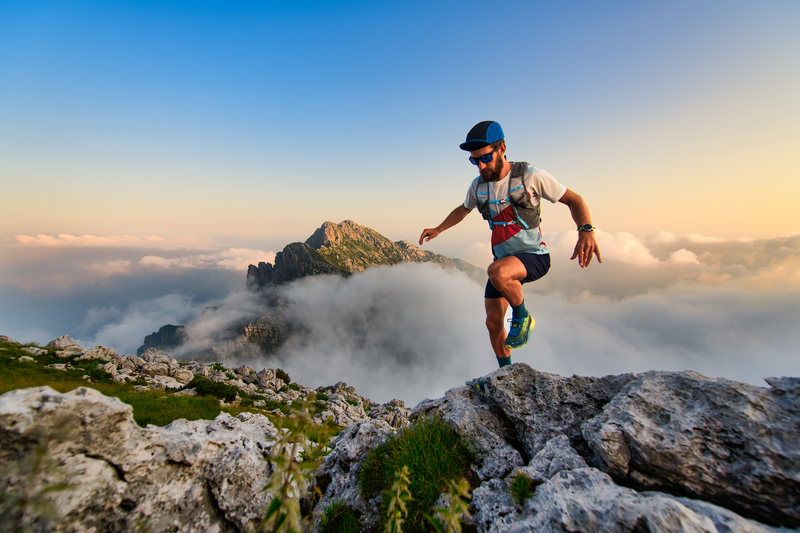
95% of researchers rate our articles as excellent or good
Learn more about the work of our research integrity team to safeguard the quality of each article we publish.
Find out more
ORIGINAL RESEARCH article
Front. Cell. Infect. Microbiol.
Sec. Oral Microbes and Host
Volume 15 - 2025 | doi: 10.3389/fcimb.2025.1543100
The final, formatted version of the article will be published soon.
You have multiple emails registered with Frontiers:
Please enter your email address:
If you already have an account, please login
You don't have a Frontiers account ? You can register here
The rising use of dental implants is accompanied by an expected increase in peri-implant diseases, particularly peri-implantitis (PI), which poses a significant threat to implant success and necessitates a thorough understanding of its pathogenesis for effective management. To gain deeper insights into the role and impact of the peri-implant microbiome in the pathogenesis and progression of PI, we analyzed 100 samples of saliva and subgingival biofilm from 40 participants with healthy implants (HI group) or with co-occurrence of diagnosed PI-affected implants and healthy implants (PI group) using shotgun metagenomic sequencing. We identified the most discriminative species distinguishing healthy from diseased study groups through log ratios and differential ranking analyses. Mogibacterium timidum, Schaalia cardiffensis, Parvimonas micra, Filifactor alocis, Porphyromonas endodontalis, Porphyromonas gingivalis and Olsenella uli were associated with the subgingival peri-implant biofilm. In contrast, Neisseria sp oral taxon 014, Haemophilus parainfluenzae, Actinomyces naeslundii, Rothia mucilaginosa and Rothia aeria were more prevalent in the healthy peri-implant biofilm. Functional pathways such as arginine and polyamine biosynthesis, including putrescine and citrulline biosynthesis, showed stronger correlations with PI-affected implants. In contrast, peri-implant health was characterized by the predominance of pathways involved in purine and pyrimidine deoxyribonucleotide de novo biosynthesis, glucose and glucose-1-phosphate degradation, and tetrapyrrole biosynthesis. Our findings reveal that healthy implants in PI-free oral cavities differ significantly in microbial composition and functional pathways compared to healthy implants cooccurring with PI-affected implants, which more closely resemble PI-associated profiles. This pattern extended to salivary samples, where microbial and functional biomarkers follow similar trends.
Keywords: Peri-Implantitis, peri-implant microbiome, Saliva microbiome, functional pathways, Shotgun metagenomic sequencing, Differential rankings, Compositional change
Received: 10 Dec 2024; Accepted: 26 Mar 2025.
Copyright: © 2025 Bessa, Egas, Pires, Proença, Mascarenhas, Pais, Barroso, Machado, Botelho, Alcoforado, Mendes and Alves. This is an open-access article distributed under the terms of the Creative Commons Attribution License (CC BY). The use, distribution or reproduction in other forums is permitted, provided the original author(s) or licensor are credited and that the original publication in this journal is cited, in accordance with accepted academic practice. No use, distribution or reproduction is permitted which does not comply with these terms.
* Correspondence:
Lucinda J. Bessa, Egas Moniz Center for Interdisciplinary Research (CiiEM), Egas Moniz School of Health & Science, Almada, Portugal
Disclaimer: All claims expressed in this article are solely those of the authors and do not necessarily represent those of their affiliated organizations, or those of the publisher, the editors and the reviewers. Any product that may be evaluated in this article or claim that may be made by its manufacturer is not guaranteed or endorsed by the publisher.
Research integrity at Frontiers
Learn more about the work of our research integrity team to safeguard the quality of each article we publish.