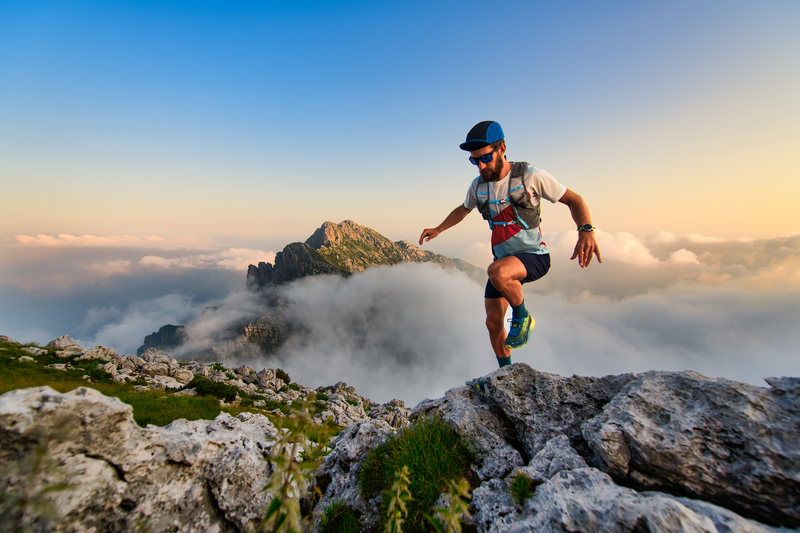
94% of researchers rate our articles as excellent or good
Learn more about the work of our research integrity team to safeguard the quality of each article we publish.
Find out more
ORIGINAL RESEARCH article
Front. Cell. Infect. Microbiol.
Sec. Clinical Infectious Diseases
Volume 15 - 2025 | doi: 10.3389/fcimb.2025.1499154
This article is part of the Research Topic Recent Advancements in the Research Models of Infectious Diseases View all 6 articles
The final, formatted version of the article will be published soon.
You have multiple emails registered with Frontiers:
Please enter your email address:
If you already have an account, please login
You don't have a Frontiers account ? You can register here
COVID-19 caused by the coronavirus SARS-CoV-2 has resulted in a global pandemic. Considering some patients with COVID-19 rapidly develop respiratory distress and hypoxemia, early assessment of the prognosis for COVID-19 patients is important, yet there is currently a lack of research on a comprehensive multi-marker approach for disease prognosis assessment.Here, we utilized a large sample of hospitalized individuals with COVID-19 to systematically compare the clinical characteristics at admission and developed a nomogram model that was used to predict prognosis. In all cases, those with pneumonia, older age, and higher PT-INR had a poor prognosis. Besides, pneumonia patients with older age and higher PT-INR also had a poor prognosis. A nomogram model incorporating presence of pneumonia, age and PT-INR could evaluate the prognosis in all patients with SARS-CoV-2 infections well, while a nomogram model incorporating age and PT-INR could evaluate the prognosis in those with pneumonia well. Together, our study establishes a prognostic prediction model that aids in the timely identification of patients with poor prognosis and helps facilitate the improvement of treatment strategies in clinical practice in the future.
Keywords: PT-INR, age, predictor, Mortality, SARS-CoV-2
Received: 20 Sep 2024; Accepted: 03 Mar 2025.
Copyright: © 2025 Xu, Tang, Guo, Lin, Guo, Fang, Lin, Shi, Lai, Pan, Tang, You, Li, Song, Wang, Cai and Fu. This is an open-access article distributed under the terms of the Creative Commons Attribution License (CC BY). The use, distribution or reproduction in other forums is permitted, provided the original author(s) or licensor are credited and that the original publication in this journal is cited, in accordance with accepted academic practice. No use, distribution or reproduction is permitted which does not comply with these terms.
* Correspondence:
Jialin Song, Fujian Maternity and Child Health Hospital, Fuzhou, 350001, Fujian Province, China
Liang Wang, Fujian Medical University, Fuzhou, China
Weidong Cai, Fujian Medical University, Fuzhou, China
Ya Fu, Fujian Medical University, Fuzhou, China
Disclaimer: All claims expressed in this article are solely those of the authors and do not necessarily represent those of their affiliated organizations, or those of the publisher, the editors and the reviewers. Any product that may be evaluated in this article or claim that may be made by its manufacturer is not guaranteed or endorsed by the publisher.
Research integrity at Frontiers
Learn more about the work of our research integrity team to safeguard the quality of each article we publish.