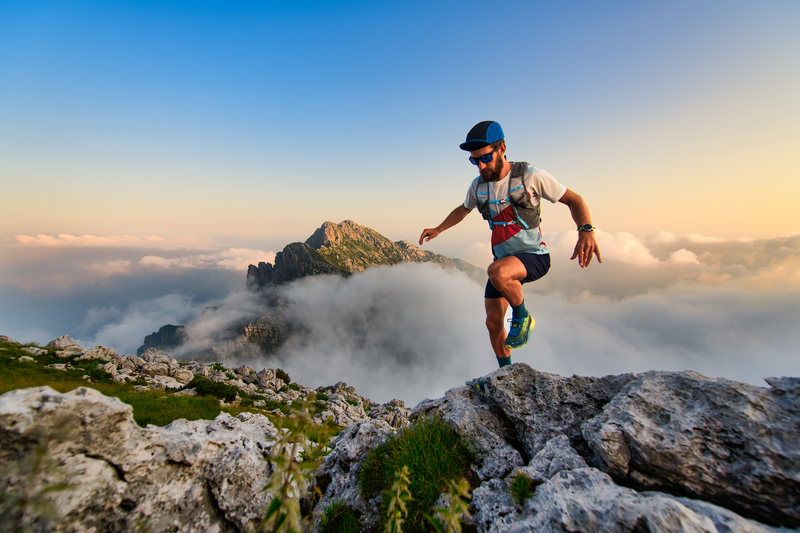
95% of researchers rate our articles as excellent or good
Learn more about the work of our research integrity team to safeguard the quality of each article we publish.
Find out more
ORIGINAL RESEARCH article
Front. Cell. Infect. Microbiol. , 18 March 2025
Sec. Intestinal Microbiome
Volume 15 - 2025 | https://doi.org/10.3389/fcimb.2025.1485048
Introduction: Obesity, a pressing global health issue, is intricately associated with distinct gut microbiota profiles. Bariatric surgeries, such as Laparoscopic Sleeve Gastrectomy (LSG), Sleeve Gastrectomy (SG), and Roux-en-Y Gastric Bypass (RYGB), induce substantial weight loss and reshape gut microbiota composition and functionality, yet their comparative impacts remain underexplored.
Methods: This study integrated four published metagenomic datasets, encompassing 500 samples, and employed a unified bioinformatics workflow for analysis. We assessed gut microbiota α-diversity, identified species biomarkers using three differential analysis approaches, and constructed high-quality Metagenome-Assembled Genomes (MAGs). Comparative genomic, functional profiling and KEGG pathway analyses were performed, alongside estimation of microbial growth rates via Peak-to-Trough Ratios (PTRs).
Results: RYGB exhibited the most pronounced enhancement of gut microbiota α-diversity compared to LSG and SG. Cross-cohort analysis identified 39 species biomarkers: 27 enriched in the non-obesity group (NonOB_Enrich) and 12 in the obesity group (OB_Enrich). Among the MAGs, 177 were NonOB_Enrich and 14 were OB_Enrich. NonOB_Enrich MAGs displayed enriched carbohydrate degradation profiles (e.g., GH105, GH2, GH23, GH43, and GT0 families) and higher gene diversity in fatty acid biosynthesis and secondary metabolite pathways, alongside significant enrichment in amino acid metabolism (KEGG analysis). Post-surgery, Akkermansia muciniphila and Bacteroides uniformis showed elevated growth rates based on PTRs.
Discussion: These findings underscore RYGB’s superior impact on gut microbiota diversity and highlight distinct microbial functional adaptations linked to weight loss, offering insights for targeted therapeutic strategies.
The gut microbiota is essential for human health, impacting metabolism, immune function, and overall well-being (Clemente et al., 2012). Obesity, a widespread global health concern, is linked to distinct gut microbiota profiles (Turnbaugh et al., 2006). Bariatric surgeries, such as Laparoscopic Sleeve Gastrectomy (LSG), Sleeve Gastrectomy (SG), and Roux-en-Y gastric bypass (RYGB), are highly effective treatments for severe obesity, resulting in significant weight loss and metabolic improvements (Zhang et al., 2009). These procedures also lead to notable changes in the composition and function of the gut microbiota (Aron-Wisnewsky et al., 2019; Lee et al., 2019). Additionally, identifying novel weight loss-related microbes and understanding their mechanisms of action are crucial for advancing human health and the development of the health industry (O’Toole et al., 2017). This study integrates data from multi-cohort data to identify weight loss-related microbe biomarkers.
Variations in study designs, experimental procedures, and bioinformatics workflows have contributed to inconsistencies in research findings. For example, studies have reported conflicting outcomes regarding the relative abundance of the phylum Bacteroidetes and the populations of Faecalibacterium and Bifidobacterium species following bariatric surgery, with some indicating an increase and others a decrease (Chen et al., 2017; Fouladi et al., 2021; Furet et al., 2010; Murphy et al., 2017). Furthermore, some studies investigating the impact of RYGB on gut microbiota (Palleja et al., 2016) have used the Wilcoxon test to identify differential species, which may result in unacceptably high false positive rates (Nearing et al., 2022). In this study, by employing three differential analysis methods—LEfSe, ANCOM, and edgeR—we identified consistently significant differential species across cohorts. This highlights the necessity of integrating multiple methods for a comprehensive analysis to identify more reliable species-level biomarkers related to obesity or weight loss. Additionally, there is currently no comparative study examining the impact of different surgical methods on gut microbiota diversity. In this study, we compared the effects of gut microbiota recovery under different surgical methods using a unified and standardized bioinformatics analysis workflow.
As the study (Fouladi et al., 2021) analyzing multi-cohort data to identify replicable post-RYGB gut microbiota and metabolic pathway shifts (e.g., increased Veillonella/Akkermansia and decreased Blautia) conducted a meta-analysis mainly based on existing 16S rRNA research, there is a notable lack of meta-analyses at the metagenomic level. This study addresses this gap by utilizing four published metagenomic datasets, encompassing 500 samples related to bariatric surgery, and applying a unified bioinformatics analysis workflow. Metagenome-Assembled Genomes (MAGs) construction, a culturing-independent and reference-free approach, offers a promising strategy for uncovering microbial diversity and genomic features (Saheb Kashaf et al., 2022; Zeng et al., 2022). This study aims to address this gap by using MAGs to conduct a comparative genomic analysis of NonOB_Enrich MAGs and OB_Enrich MAGs across diverse geographical locations to elucidate the potential genetic mechanisms underlying weight gain or loss.
This study highlights the importance of metagenomic approaches in understanding the complex interplay between gut microbiota and obesity, offering potential avenues for optimizing bariatric surgery and enhancing patient health (Qin et al., 2012). By elucidating the microbial shifts associated with bariatric surgery, this research offers valuable insights for developing targeted probiotic therapies to enhance obesity treatment.
This study comprises four distinct cohorts designed to analyze the metagenomic profiles of gut microbiota in obese individuals before and after bariatric surgery across different geographical locations. The study population undergoing weight loss interventions excluded individuals with basal metabolic diseases, cancer, and other related conditions. The samples were derived from consistent tissue types and uniform sequencing methods were employed. After screening, a total of four cohorts were included in the study (Table 1), as detailed below:
Table 1. The four distinct cohorts designed to analyze the metagenomic profiles of gut microbiota in obese individuals before and after bariatric surgery across different geographical locations in this study.
Cohort 1 - PRJEB12123 (Liu et al., 2017). This cohort includes 256 fecal samples collected from Beijing, China. The samples include 105 healthy individuals, 88 obese individuals, and 63 individuals at various stages post-Sleeve Gastrectomy (SG). Specifically, the post-SG samples are categorized into SG 0M (23 samples), SG 1M (17 samples), and SG 3M (23 samples). This cohort uses paired-end sequencing with a read length of 100bp.
Cohort 2 - PRJNA597839 (Nie et al., 2020): Comprising 76 fecal samples from the USA, this cohort focuses on the longitudinal analysis of patients undergoing laparoscopic Sleeve Gastrectomy (LSG). It includes 30 healthy individuals and 46 obese individuals pre- and post-surgery. The samples collected include LSG pre (32 samples) and LSG 6M (14 samples). Sequencing is performed with paired-end reads of 150bp.
Cohort 3 - PRJEB12947 (Palleja et al., 2016): This cohort consists of 33 fecal samples collected from Beijing, China, specifically focusing on patients undergoing Roux-en-Y gastric bypass (RYGB). This cohort focuses on longitudinal samples from patients undergoing RYGB, without healthy controls: RYGB pre (13 samples), RYGB 1M (1 sample), RYGB 3M (12 samples), and RYGB 12M (8 samples). Paired-end sequencing with 2x100bp reads is utilized for this cohort.
Cohort 4 - PRJNA668357 (Fouladi et al., 2021): The final cohort involves 135 fecal samples from the USA, targeting the analysis of gut microbiota in patients undergoing RYGB surgery. This cohort includes 52 obese individuals and post-surgery samples: RYGB pre (38 samples), RYGB 1M (0 samples), RYGB 3M (27 samples), and RYGB 12M (18 samples). The sequencing for this cohort is performed with paired-end reads of 2x150bp.
Sequence read archive (SRA) files of all samples were downloaded using prefetch software. Raw sequence data were subjected to quality control using fastp (https://github.com/OpenGene/fastp). Human-derived sequences were removed by aligning the reads to the human genome using Bowtie2 (v2.3.4.3). Next, these non-human sequences were aligned to a reference database with Kraken2 (Wood et al., 2019). As a reference database, we used the Kraken-build utility to download bacterial, archaeal, viral and fungal libraries including National Centre for Biotechnology Information (NCBI) taxonomic information as well as complete genome sequences from RefSeq (Jing et al., 2021; Nearing et al., 2022; Sola-Leyva et al., 2021). In addition, we also incorporated the human and protozoa genome into the Kraken2 database to further reduce false-positive microbes introduced by human-derived or other eukaryotic sequences. Based on the effective clean data volume from the sequencing of each sample, we used the RPTM (Reads Per Ten Million) normalization method to standardize the data at the species level, followed by subsequent diversity and differential species analyses. Besides, the filtered reads were then assembled denovo using megahit (https://github.com/voutcn/megahit). Metagenome-Assembled Genomes (MAGs) were constructed using metaWRAP (https://github.com/bxlab/metaWRAP). The bin refinement module in metaWRAP was employed to optimize MAG quality, followed by dereplication using dRep (https://github.com/MrOlm/drep,v3.2.2) to remove redundant MAGs. Taxonomic classification of the MAGs was performed using the classify-wf module in GTDB-Tk. Genomic k-mer distances among MAGs were calculated using mash (v2.3, https://mash.readthedocs.io/en/latest/). A phylogenetic tree was constructed with the Neighbor-Joining method (nj) using the ape package in R. The resulting tree was visualized using the Interactive Tree Of Life (iTOL) web tool. Genes within the MAGs were predicted by prodigal (v2.6.3) and annotated by aligning them to the CAZy and KEGG databases using the blastp module of DIAMOND (https://github.com/bbuchfink/diamond). Functional genes were identified and their abundances were quantified for each MAG. CoPTR (v1.1.6) was used to compute peak-to-trough ratios (PTRs) from MAGs to accurately reflect microbial growth rates. Gapseq (v1.3.1) was used to predict metabolic processes (Zimmermann et al., 2021). Prediction of secondary metabolites in each MAG was performed using antiSMASH (v7.0.1).
The effects of different cohorts, sample locations, and experimental groups on microbial community composition were assessed using the PERMANOVA test in the vegan package of R (p-value < 0.05 was considered significant). Differential abundance testing to identify differentially abundant species between obese and healthy individuals (OB-Healthy) and between obese individuals pre- and post-treatment (OB-Treat) employed three methods: edgeR v3.36.0 (FDR < 0.05, FC = 5), ANCOM v2.1 (detection threshold 0.9), and LEfSe v1.0 (logarithmic LDA score > 2). Core differential species were identified based on consensus results from these methods across multiple cohorts, ensuring robust identification of key species affected by obesity and treatment. The Kruskal-Wallis test was used to compare PTR differences between groups. Differential KOs were subjected to KEGG enrichment analysis using R clusterProfiler. We calculated the within-sample α-diversity using Shannon and ACE index on species level to estimate the richness of samples using QIIME (Caporaso et al., 2010) v1.9.1. Besides, the post-surgery α-diversity index increased ratio refers to the proportional increase in α-diversity index after surgery compared to preoperative levels. Specifically, we calculated this ratio by: (postoperative α-diversity index - preoperative α-diversity index)/preoperative α-diversity index for each cohort. Variance partitioning analysis was performed by varpart function of vegan packages.
Three surgical methods—SG, LSG, and RYGB—all enhanced the α-diversity index of the gut microbiota, which was consistent with previous studies (Liu et al., 2017; Paganelli et al., 2019)(Figures 1A, B; Supplementary Table S1). Additionally, even within the same group (Healthy or OB) across different cohorts, significant differences were observed in alpha diversity (Supplementary Figure S1). To compare the effects of different surgical methods on gut microbiota diversity, for each cohort, we computed the ratio of the post-surgical α-diversity index increase relative to the OB group. The results showed that, in terms of species richness (ACE index), LSG > RYGB > SG. From the perspective of both species richness and evenness (Shannon index), RYGB > LSG > SG (Supplementary Table S2).
Figure 1. Microbial community diversity comparison. (A) shannon index and (B) ace index comparison of different groups for each cohort. (C) PCoA plot showing significant differences between the four cohorts (PRJEB12123, PRJEB12947, PRJNA597839, and PRJNA668357) (D) PCoA plot presenting the distribution of microbial communities among OB, health and treat status. The curves represent the trend lines of the diversity index changes for each surgical method. (E) Venn diagram showing variance partitioning analysis (VPA) for project, site, and treatment factors. Site is grouped by the geographical variation between samples from China, Denmark and the USA.
Principal Coordinates Analysis (PCoA) and PERMANOVA can be used to assess the impact of different studies and confounding factors on microbiota outcomes (Wang et al., 2020). The analysis revealed that cohort locations and surgical interventions significantly influence microbial community structure, with the impacts of obesity and surgical intervention being the most pronounced (indicated by higher R2 values). Figure 1C shows significant differences between the four cohorts (PRJEB12123, PRJEB12947, PRJNA597839, and PRJNA668357), with an PERMANOVA R2 value of 0.03539 and a p-value of 0.001, indicating significant grouping by cohort. Figure 1D illustrates the distribution of microbial communities across various health and treatment statuses, including healthy individuals and those undergoing LSG, RYGB, and SG. The analysis yielded a PERMANOVA R2 value of 0.06557 and a p-value of 0.001, indicating significant differences among these groups, with the impact of surgical treatment (a higher R2) being more pronounced than the influence of cohorts. To further validate the robustness of PERMANOVA results, we conducted variance partitioning analysis (VPA) (Figure 1E), which confirmed that the dominant effect of Treat is not influenced by the collinearity of project cohorts or country site, while the PERMANOVA R² of country site is inflated due to collinearity. Specifically, Treat exhibited an independent contribution of 6.75% (after excluding project cohorts and country site), whereas project cohorts contributed only 0.77% (after excluding country site and Treat), and country site showed no significant independent contribution (after excluding project cohorts and Treat), reinforcing that Treat is the primary variable with significant independent explanatory power. Overall, these results highlight the significant effects of cohort and surgical treatment on gut microbiota diversity.
Due to the significant impact of different cohorts on gut microbiota, and to uncover significantly enriched microbes for subsequent MAGs analysis, we compared the results by three differential analysis methods for each cohort to identify differential species which enhances the accuracy of differential species analysis. For LEfSe differential analysis method: using LDA > 2, 51 differential species were identified between the OB and Treat groups, and 32 differential species were identified between the OB and Healthy groups. For ANCOM differential analysis method: using a detection threshold > 0.9, 32 differential species were identified between the OB and Treat groups, and 16 differential species were identified between the OB and Healthy groups. For edgeR differential analysis method: using FDR < 0.05 and fold-change > 5, 542 differential species were identified between the OB and Treat groups, and 244 differential species were identified between the OB and Healthy groups. The three differential analysis methods yielded varying results, with the number of identified markers ranked from highest to lowest as follows: edgeR, LEfSe, and ANCOM. However, there was relatively little overlap between the results of the three methods (Figure 2). Specifically, the three methods identified only 3 common markers in the “Healthy vs. OB” group and 7 common markers in the “Treat vs. OB” group, which is significantly fewer than the unique markers identified by each method. This highlights the necessity of integrating different cohorts and multiple differential analysis methods to comprehensively identify differential species.
Figure 2. The Venn diagrams compare species markers identified by different methods (ANCOM, LEfSe, edgeR) for “Healthy vs. OB” (A) and “Treat vs. OB” (B) groups, highlighting unique and shared markers.
Theoretically, potential beneficial microbes are more likely to be enriched in healthy or treatment groups compared to the OB group, a strategy also utilized in a published study (Wu et al., 2024). More cohort data and difference methodological support could help minimize the likelihood of false-positive markers. In this context, the more diverse the differential analysis methods and the more supported cohorts, the more reliable the identified biomarkers will be. Supplementary Table S3 lists species that are significantly enriched in the healthy group (16 species in total) and treatment group (11 species in total), focusing on those with the strongest support. For instance, Coprobacter fastidiosus and Lachnospira eligens were identified in two cohorts (PRJEB12123 and PRJNA597839) and supported by three differential analysis methods (edgeR, ANCOM, and LEfSe for the former; and ANCOM and LEfSe for the latter). Other species, such as Bacteroides stercorisrooris, Bacteroides timonensis, and Brotilimocola acetigignens, were identified in two cohorts and supported by edgeR analysis. In the treatment group, several species were identified across four cohorts, indicating strong support for their enrichment post-treatment. For example, Bifidobacterium dentium, Raoultella planticola, and Streptococcus mutans were consistently enriched across all four cohorts (PRJEB12123, PRJNA597839, PRJEB12947, and PRJNA668357), supported by edgeR analysis. Additionally, species such as Veillonella atypica and Veillonella parvula were identified in three cohorts and supported by three differential analysis methods (edgeR, ANCOM, and LEfSe). Similarly, we also analyzed the species enriched in OB group (Supplementary Table S4), including Faecalimonas umbilicata, Fusobacterium varium, Acidaminococcus provencensis, Alkalihalobacillus bogoriensis, Collinsella tanakaei, Ellagibacter isourolithinifaciens, Enterococcus casseliflavus, Fusobacterium ulcerans, Limosilactobacillus mucosae, Peptacetobacter hiranonis, Slackia isoflavoniconvertens, and Turicibacter bilis. Overall, 39 species biomarkers were identified, including 27 microbes enriched in the NonOB_Enrich group and 12 microbes enriched in the OB_Enrich group. These 39 identified species biomarkers could serve as potential beneficial or non-beneficial microbes for future wet-lab functional validation.
To elucidate the genomic mechanisms underlying postoperative species abundance variations, we conducted a MAG construction analysis. We obtained 7,336 high-quality MAGs (completeness >90%, contamination <5%). After dRep-based deduplication, 4,201 unique MAGs were retained. We plotted the assembly completeness and contamination statistics for these MAGs. The quality statistics of the MAGs (Figure 3A) show that 69.2% of MAGs exceed 95% completeness, with the majority (57.87%) having contamination rates below 1%. The phylogenetic tree (Supplementary Figure S2) illustrates the diversity and distribution of MAGs across different groups, projects, and phyla. From the overall structure of the phylogenetic tree, MAGs from different phyla are classified into distinct branches based on their evolutionary relationships. The genomes are distributed across different research cohorts and groups, demonstrating overall high genomic diversity. According to Figure 3B, Firmicutes_A has the highest representation across all categories, particularly in the PRJEB12123 project and the Healthy group. Bacteroidota and Actinobacteriota also show significant presence, with notable counts in both the Healthy and Treat groups across multiple projects. Other phyla, such as Desulfobacterota_I, Fusobacteriota, and Verrucomicrobiota, have lower representation but are still present across different categories, indicating a diverse genomic composition.
Figure 3. Comprehensive analysis of MAGs quality, distribution, and functional annotations. (A) Distribution of the 4,201 unique MAGs based on completeness or contamination levels. (B) The heatmap displays the distribution of MAGs across different corhots (PRJEB12123, PRJNA597839, PRJEB12947, PRJNA668357) and groups (Healthy, OB, Treat), organized by phylum. The color intensity and numerical values indicate the number of MAGs in each category. (C) Number of high-quality MAGs corresponding to potential species enriched in Healthy, OB and Treat group. (D) Phylogenetic tree of the MAGs. The rings from inner to outer represent species classifications, enriced-group, phylum and group affiliations (Healthy, Treat, OB). (E) NMDS analysis based on CAZy enzymes families relative abundance profile of MAGs in the NonOB_Enrich and OB_Enrich group. The clustering circles represent the 80% confidence ellipse based on s.class function in ade4 (F) Bubble chart showing the distribution of top 50 enzyme genes from MAGs annotated to CAZy enzyme families for MAGs in the NonOB_Enrich and OB_Enrich group.
Based on the significantly enriched microbe lists in Supplementary Tables S3, S4, the number of MAGs identified through binning for each bacterium was summarized (Supplementary Table S5; Figure 3C). The analysis identified 185 gut microbiota MAGs (171 MAGs in the NonOB_Enrich group and 14 MAGs in the OB_Enrich group). These MAGs include five core species in the healthy group: Lachnospira eligens, Bacteroides uniformis, Coprobacter fastidiosus, Desulfovibrio fairfieldensis, and Klebsiella michiganensis. In the treatment group, eight core species were identified: Akkermansia muciniphila, Streptococcus salivarius, Eisenbergiella tayi, Bifidobacterium dentium, Enterocloster aldenensis, Enterocloster lavalensis, Streptococcus mutans, and Klebsiella variicola. Notably, Lachnospira eligens exhibited the highest number of MAGs in the healthy group, followed by Bacteroides uniformis and Coprobacter fastidiosus. In the treatment group, Akkermansia muciniphila had the highest number of MAGs. Additionally, five core species were identified, including Faecalimonas umbilicata, Collinsella tanakaei, Ellagibacter isourolithinifaciens, Peptacetobacter hiranonis, and Acidaminococcus provencensis. The phylogenetic tree results (Figure 3D) show that Bacteroides uniformis, Coprobacter fastidiosus, and Akkermansia muciniphila in the NonOB_Enrich group, cluster into one branch, while Collinsella tanakaei and Ellagibacter isourolithinifaciens in the OB_Enrich group, form a separate branch. These species may exhibit certain similarities in their biological functions. We further conducted a literature review to validate the aforementioned microbes as potential beneficial or non-beneficial ones (Supplementary Table S6).
A key characteristic of obesity-related microorganisms is their ability to metabolize carbohydrates, converting specific sugars into propionic acid, lactic acid, or acetic acid. This metabolic activity plays a significant role in modulating the host’s response to a carbohydrate-rich diet, which is particularly relevant in the context of obesity development (Derrien and van Hylckama Vlieg, 2015; Le Barz et al., 2015; Schwiertz et al., 2010). NMDS based on CAZY enzymes count of MAGs (Figure 3E) showed a significant separation between microbes in the NonOB_Enrich and OB_Enrich group, indicating distinct carbohydrate degradation and utilization profiles. The bubble plot (Figure 3F) further shows the top 50 enzyme families by relative gene abundance across various species. The analysis reveals that key enzyme families, including Carbohydrate-Binding Modules (CBM), Carbohydrate Esterases (CE), Glycoside Hydrolases (GH), GlycosylTransferases (GT), and Polysaccharide Lyases (PL), have a higher relative proportion of metabolic enzyme genes detected in MAGs of the NonOB_Enrich group, such as Bacteroides uniformis, Lachnospira eligens, Akkermansia muciniphila, and Eisenbergiella tayi, compared to the MAGs in OB_Enrich group, implying that they have a stronger ability to metabolize and utilize a variety of complex carbohydrates.
We compared the shared and unique CAZy enzymes detected in microbes between the NonOB_Enrich and OB_Enrich group (Figure 4A; Supplementary Table S7). The results indicate that microbes in NonOB_Enrich group exhibit a significantly higher number of CAZy enzyme types compared to the OB_Enrich group microbes. The top three enzymes are associated with Bacteroides uniformis, Eisenbergiella tayi, and Coprobacter fastidiosus, while the bottom three are linked to Ellagibacter isourolithinifaciens, Peptacetobacter hiranonis, and Acidaminococcus provencensis. This suggests a greater diversity in the carbohydrate-active enzyme profiles of microbes in the NonOB_Enrich group. Additionally, Bacteroides uniformis possesses the highest number of unique enzymes, followed by a small number of enzyme families shared among some microbes. The relative proportion of genes from GH105, GH2, GH23, GH43, and GT0 gene families is significantly higher in microbes of the NonOB_Enrich group compared to the microbes in OB_Enrich group (Figure 4B).
Figure 4. Statistical and enrichment analysis of functional annotation results MAGs in the NonOB_Enrich and OB_Enrich group. (A) Shared and unique CAZy enzymes detected in microorganisms. Vertical bars (left): Represent the total number of CAZy enzymes in each microbe individually. Horizontal bars (above): Show the size of intersections, indicating the number of enzymes shared among microbes or unique to each group. Dots and connecting lines: Illustrate the specific combinations of sets being compared. (B) Significantly different enzyme families between the NonOB_Enrich group and the OB_Enrich group. (C) KEGG enrichment analysis based on the significantly different KEGG KOs. (D) Cluster analysis of secondary metabolite prediction based on MAGs. Normalization of the average number of secondary metabolites detected in each species’ MAG using Z-scores. * indicates statistical significance at P < 0.05, while ** denotes high statistical significance at P < 0.01.
Based on the 74 significantly different KOs (Supplementary Table S8), these KOs were significantly enriched in four pathways: “amino acid biosynthesis,” “glycine, serine, and threonine metabolism,” “phenylalanine, tyrosine, and tryptophan biosynthesis,” and “galactose metabolism” (Figure 4C). The result in Figure 4D shows that species enriched in the NonOB_Enrich group produced more secondary metabolites compared to those in the OB_Enrich group. Furthermore, the predominant secondary metabolites produced by different species varied. For instance, Akkermansia muciniphila produced more terpenes, Bacteroides uniformis produced more RRE-containing compounds, and Lachnospira eligens produced more cyclic-lactone autoinducers. Additionally, the clustering results for the NonOB_Enrich and OB_Enrich groups displayed a clear separation trend, with the exception of Bifidobacterium dentium. In healthy human gut microbiota, secondary metabolite genes, such as those involved in NRPS, terpenes, and RiPPs, helped maintain microbial diversity and inhibited excessive pathogen growth. In contrast, in obese individuals, secondary metabolite gene diversity of MAGs was reduced, and the metabolite profile was altered, potentially contributing to chronic low-grade inflammation and metabolic disorders.
Microbes that activate the fatty acid biosynthesis pathway can induce body weight gain (Henneke et al., 2022). We compared the presence of fatty acid biosynthesis genes across species in the OB_Enrich and NonOB_Enrich (each species containing at least three MAGs included in the analysis). The Venn analysis results in Figure 5A show that more genes were detected in the NonOB_Enrich group, with seven unique genes, while both groups shared nine detected genes. This is consistent with a previous report, where the activity of genes involved in fatty acid metabolism is relatively low, particularly those related to enzymes in the tricarboxylic acid (TCA) cycle, with their function being significantly reduced (Liu et al., 2017). Figure 5B shows that the MAGs of different species clustered together, indicating a preference for the fatty acid biosynthesis genes carried by each species. The beneficial gut bacterium Lachnospira eligens carried the most diverse set of genes, including fabG, MCH, accB/bccP, fabK, fabD, accC, fabF, fabZ, accA, and accD. Bacteroides uniformis carried fabG, fabD, fabF, fabI, IpxC-fabZ, fabH, and ACSL/fadD. Akkermansia muciniphila carried fabG, fabD, fabF, fabI, IpxC-fabZ, and fabH. The species enriched in OB_Enrich, Collinsella tanakaei, carried the fewest genes, only including fabF and fabZ. The result in Figure 5C further shows a tendency for separation between the OB_Enrich MAGs and NonOB_Enrich MAGs, indicating a distinct difference in the detection profiles of fatty acid biosynthesis genes between the two groups. Overall, NonOB_Enrich MAGs exhibit higher gene diversity, which may contribute to SCFA production, promote fatty acid oxidation rather than storage, and reduce lipid accumulation, thereby regulating and improving host obesity (Den Besten et al., 2013; Gurung et al., 2020). This may explain why obesity is significantly associated with a reduction in SCFA concentration (Ecklu-Mensah et al., 2023).
Figure 5. Analysis of fatty acid biosynthesis genes and reactions distribution. (A) Venn diagram showing the distribution of fatty acid biosynthesis genes in the “NonOB_Enrich” and “OB_Enrich” groups. The numbers indicate the number of unique and shared genes between the two groups. (B) The heatmap illustrates the presence of various fatty acid biosynthesis genes across different species. Clustering was performed on both rows and columns using the ward.D2 method, with normalization applied by row (C) Non-metric multidimensional scaling (NMDS) plot showing the distribution of species in the OB_Enrich and NonOB_Enrich groups. The clustering circles represent the 80% confidence ellipse based on s.class function in ade4. (D) a clustering heatmap analysis comparing the average number of reactions predicted for each MAG in the fatty acid biosynthesis pathway across species containing three or more MAGs based on gapseq.
We performed a clustering heatmap analysis (Figure 5D) to compare the average number of key reactions (z-score normalized) related to the fatty acid biosynthesis pathway, which were predicted from the genome fasta sequences of species in the obesity-associated microbiome group (OB_Enrich) and the non-obesity-associated group (NonOB_Enrich). The analysis revealed that beneficial gut microbes related to short-chain fatty acid production, such as Bacteroides uniformis, Akkermansia muciniphila, Streptococcus salivarius, and Lachnospira eligens, clustered into one subgroup. This indicates that these four species exhibit more similar fatty acid biosynthesis pathway reactions, suggesting potential synergistic effects. However, Coprobacter fastidiosus, Eisenbergiella tayi, and Desulfovibrio fairfieldensis, which were enriched in the NonOB_Enrich group, did not cluster with the other four species mentioned above, indicating distinct metabolic regulation mechanism. The enrichment of these three species in NonOB_Enrich may not directly regulate body weight through fatty acid biosynthesis, but rather exert their effects through other mechanisms, such as improving inflammatory responses.
Microbial growth rate, as reflected by PTR, was linked to disease status and largely independent of relative abundances capturing a unique biological variation that complements relative abundance data (Joseph et al., 2022a). Based on PTR analysis (Supplementary Table S9), we discovered that microbes in the NonOB_Enrich group and OB_Enrich group mainly clustered into two distinct branches (Supplementary Figure S3A). The growth rates of the same species exhibited varying distribution patterns across different dimensions, such as geographic regions, study cohorts, and participant groups. Further analysis using differential boxplots revealed that in the PRJNA668357 cohort, Akkermansia muciniphila (Supplementary Figure S3B) and Bacteroides uniformis (Supplementary Figure S3C) had significantly higher PTRs in the RYGB group compared to the OB group. Considering the longitudinal data across different postoperative time points, Akkermansia muciniphila showed a significant increasing trend in PTR from the 1st to the 6th month after RYGB surgery (Supplementary Figure S3D), although this trend became non-significant by the 12th month (p-value = 0.075). For Bacteroides uniformis, there was a significant increase in PTR from the 1st to the 12th month post-surgery (Supplementary Figure S3E), with the increase at the 6th month being less pronounced (p value = 0.18). No statistically significant differences were observed in the PTRs of other microbes after surgery. This further indicates that Akkermansia muciniphila and Bacteroides uniformis, play a more crucial role in improving obesity, with different microbes playing crucial roles at various stages of recovery.
Bariatric surgeries, effective for severe obesity, result in significant changes to gut microbiota, highlighting the importance of identifying weight loss-related microbes and understanding their mechanisms to advance both human health and the health industry. As the first metagenome-based comprehensive analysis integrating multiple studies on bariatric surgery and gut microbiota (Figure 6), this study’s methodology involves 4 cohorts and surgical interventions (Sleeve Gastrectomy, Laparoscopic Sleeve Gastrectomy, Roux-en-Y Gastric Bypass) in 3 countries (China, Denmark, USA) using a standardized bioinformatics analysis pipeline. Through cross-cohort integration of multiple differential analysis methods, the study identified significantly enriched microbial biomarkers, followed by a MAGs-based genomic comparison to explore their metabolic functions and contributions to gut health, including KEGG KO comparison, CAZy enzyme comparison, secondary metabolites, key fatty acid biosynthesis genes and reactions comparison. This research provides valuable insights into the discovery of weight loss microbes and their underlying mechanisms.
Figure 6. The diagram outlines a gut microbiota study comparison of MAGs in the NonOB_Enrich and OB_Enrich group across different cohorts, including various obesity and bariatric surgery groups. It begins with sample collection and MAG construction, followed by the exploration of microbial species and genomic composition. The study identified significantly enriched microbial biomarkers, followed by a MAGs-based genomic comparison to explore their metabolic functions and contributions to gut health, including KEGG KO comparison, CAZy enzyme comparison, secondary metabolites, key fatty acid biosynthesis (FABiosynthesis) genes comparison. This research offers valuable insights into the discovery of weight loss microbes and their underlying mechanisms.
SG, LSG, and RYGB are common bariatric procedures used to treat obesity. However, RYGB is generally considered more effective in achieving long-term weight loss and remission of metabolic diseases (Mingrone et al., 2012; Schauer et al., 2017). The results of this study indicate that RYGB has a superior impact on restoring gut microbiota α-diversity (shannon index) compared to the other two procedures, which may contribute to more effective treatment of obesity. RYGB surgery reduces the size of the stomach and bypasses a portion of the small intestine, thereby significantly altering the digestive pathway. As a result, food no longer passes through the duodenum and proximal jejunum, which changes the absorption of nutrients and, in turn, impacts the microbial environment and composition of the gut microbiota. Additionally, changes in bile acid metabolism following RYGB may promote the growth of beneficial bacterial species, thereby contributing to the restoration of microbial α-diversity (Furet et al., 2010).
Identifying differentially abundant microbes is a key objective in microbiome research, with various methods often used interchangeably in the literature (Nearing et al., 2022). However, studies frequently report inconsistent results regarding the microbial effects of specific bacteria (D’Elios et al., 2020; Suez et al., 2019), and a method that performs well in one study may be absent from another (Nearing et al., 2022). In our study, based on species-level relative abundance, three methods (edgeR, ANCOM, and LEfSe) identified only 3 shared markers in the “Healthy vs. OB” group and 7 in the “Treat vs. OB” group, which is substantially fewer than the number of unique markers identified by each method. These findings underscore the need for integrating diverse cohorts and utilizing multiple differential analysis methods to achieve a more comprehensive identification of differential species.
A key characteristic of beneficial microorganisms is their ability to metabolize carbohydrates, converting specific sugars into propionic, lactic, or acetic acid. Microbes in the NonOB_Enrich group exhibit a significantly higher number of CAZy enzyme types compared to the OB_Enrich group, with gene families such as GH105, GH2, GH23, GH43, and GT0 being significantly more abundant. This is primarily because beneficial microbes typically inhabit the host’s gut (e.g., humans), forming a symbiotic relationship closely tied to the host’s nutritional needs (Lee and Mazmanian, 2010). Gut beneficial microbes help the host digest food, break down complex carbohydrates, synthesize essential amino acids, and maintain gut microecological balance. These functions require specific metabolic capabilities, such as the breakdown of carbohydrates by enzymes like alpha-galactosidase and the synthesis of tryptophan by tryptophan synthase. For example, Coprobacter fastidiosus expresses a significant number of glycoside hydrolase (GH)-encoding genes and possesses the highest diversity of GH families, thereby promoting the host’s ability to digest an appropriate high-fiber diet (Henneke et al., 2022).
In the analysis of fatty acid biosynthesis, Coprobacter fastidiosus, Eisenbergiella tayi, and Desulfovibrio fairfieldensis, which were enriched in the NonOB_Enrich group, did not cluster with the other species. A possible explanation for this could be related to the unique metabolic pathways of Coprobacter fastidiosus. Propionate, acetate, and succinate are generated through the Wood-Werkman cycle and a partial tricarboxylic acid (TCA) cycle. Genomic analysis of the core metabolism of C. fastidiosus suggests the presence of a Wood-Werkman cycle and a partial TCA cycle, which lacks succinyl-CoA hydrolase, similar to the pathway described for Propionibacterium freudenreichii. Additionally, C. fastidiosus possesses a respiratory chain that can be utilized in propionic acid production. Instead of following the classic fatty acid biosynthesis pathway, acetyl-CoA in C. fastidiosus is likely synthesized via pyruvate-flavodoxin oxidoreductase (gene NSB1T_03985) (Chen et al., 2024). In longevity populations, D. fairfieldensis is more abundant and contributes to the biosynthesis of menaquinone (vitamin K2), which may help prevent age-related diseases, such as osteoporosis-induced fractures. E. tayi in the gut plays a key role through enzymes encoded in its genome, including 1.17.1.8: 4-hydroxy-tetrahydrodipicolinate reductase (EC 1.17.1.8), 4.3.3.7: 4-hydroxy-tetrahydrodipicolinate synthase (EC 4.3.3.7), and 2.7.2.4: Aspartate kinase (EC 2.7.2.4), which are important for distinguishing the transcriptional states of keystone taxa (Bauchinger et al., 2024). Moreover, studies on the gut microbiome of longevity populations have found that E. tayi is more abundant in these groups. The bacterial protein N-glycosylation involving E. tayi may play a significant role in regulating physiological and pathological processes during aging (Chen et al., 2024). The enrichment of these three species in the NonOB_Enrich group may not directly influence body weight through fatty acid biosynthesis, but rather may exert effects via other mechanisms.
PTRs have the potential to be a valuable tool for investigating microbiome dynamics (Joseph et al., 2022b). From the perspective of PTRs based on the constructed MAGs, we found that in the USA cohort (PRJNA668357), Akkermansia muciniphila and Bacteroides uniformis exhibited significantly higher PTRs in the RYGB-treated group compared to the obese (OB) group, suggesting enhanced growth post-surgery. The longitudinal increase in PTRs for these species across different postoperative time points further highlights their potential roles in recovery and weight management. These PTRs increase emphasize the importance of specific biomarkers, particularly Akkermansia muciniphila and Bacteroides uniformis. Known for its ability to improve gut barrier integrity and metabolic health, Akkermansia muciniphila has been shown to reduce body fat, enhance insulin sensitivity, and decrease inflammation, making it a promising candidate for obesity treatment (Xu et al., 2020). Similarly, Bacteroides uniformis may support weight loss by improving metabolic functions and modulating gut microbiota, especially in obese individuals with intestinal dysbiosis. This beneficial effect has been observed in animal models, demonstrating potential for future applications in weight management (Gomez Del Pulgar et al., 2020; Van Hul and Cani, 2023). While PTR analysis provides insights into microbial replication dynamics, its interpretation should be tempered by methodological constraints. The accuracy of PTR estimates inherently depends on the completeness of MAG reconstruction and sequencing depth, as fragmented assemblies or low-coverage genomes may introduce biases in peak-to-trough ratio calculations. Therefore, this approach is primarily applicable for comparative analyses of high-quality MAGs, while its utility as an absolute measure of metabolic activity across heterogeneous microbial communities may be limited.
However, this study has three limitations. Firstly, it relies solely on metagenomic data, lacking comprehensive clinical metrics for deeper correlation analysis. Secondly, the use of Kraken for species identification is restricted to known microbial databases, leaving potential unknown microbes uninvestigated. Thirdly, the study is limited to metagenomic analysis without integrating multi-omics data or conducting further validation experiments on the identified microbe biomarkers.
This study contributes to the understanding of obesity treatment by identifying microbial species associated with weight loss, through cross-cohort integration and multi-method analysis. It emphasizes the importance of gut microbiota restoration and microbial diversity, offering valuable insights for potential therapeutic applications.
The datasets presented in this study can be found in online repositories. The names of the repository/repositories and accession number(s) can be found in the article/Supplementary Material.
The study was conducted according to the guidelines of the Declaration of Helsinki, and approved by the Institutional Review Board of Department of Plastic Surgery Hospital, Chinese Academy of Medical Sciences & Peking Union Medical College (protocol code 201737 and 2017/9/21 was approval). The studies were conducted in accordance with the local legislation and institutional requirements. Written informed consent for participation was not required from the participants or the participants’ legal guardians/next of kin in accordance with the national legislation and institutional requirements.
HS: Conceptualization, Data curation, Formal Analysis, Investigation, Methodology, Project administration, Software, Validation, Visualization, Writing – original draft. JL: Conceptualization, Funding acquisition, Investigation, Methodology, Project administration, Resources, Supervision, Visualization, Writing – review & editing.
The author(s) declare that financial support was received for the research and/or publication of this article. This work was supported by the CAMS Innovation Fund for Medical Sciences (CAMS-I2M-1-007, 2021-I2M-1-052).
We thank Jianpeng Gao and Lijuan Yuan for helpful discussions on this work.
The authors declare that the research was conducted in the absence of any commercial or financial relationships that could be construed as a potential conflict of interest.
All claims expressed in this article are solely those of the authors and do not necessarily represent those of their affiliated organizations, or those of the publisher, the editors and the reviewers. Any product that may be evaluated in this article, or claim that may be made by its manufacturer, is not guaranteed or endorsed by the publisher.
The Supplementary Material for this article can be found online at: https://www.frontiersin.org/articles/10.3389/fcimb.2025.1485048/full#supplementary-material
Aron-Wisnewsky, J., Prifti, E., Belda, E., Ichou, F., Kayser, B. D., Dao, M. C., et al. (2019). Major microbiota dysbiosis in severe obesity: fate after bariatric surgery. Gut 68, 70–82. doi: 10.1136/gutjnl-2018-316103
Bauchinger, F., Seki, D., Berry, D. (2024). Characteristics of putative keystones in the healthy adult human gut microbiota as determined by correlation network analysis. Front. Microbiol. 15, 1454634. doi: 10.3389/fmicb.2024.1454634
Caporaso, J. G., Kuczynski, J., Stombaugh, J., Bittinger, K., Bushman, F. D., Costello, E. K., et al. (2010). QIIME allows analysis of high-throughput community sequencing data. Nat. Methods 7, 335–336. doi: 10.1038/nmeth.f.303
Chen, H., Qian, L., Lv, Q., Yu, J., Wu, W., Qian, H. (2017). Change in gut microbiota is correlated with alterations in the surface molecule expression of monocytes after Roux-en-Y gastric bypass surgery in obese type 2 diabetic patients. Am. J. Trans. Res. 9, 1243–1254.
Chen, S., Zhang, Z., Liu, S., Chen, T., Lu, Z., Zhao, W., et al. (2024). Consistent signatures in the human gut microbiome of longevous populations. Gut Microbes 16, 2393756. doi: 10.1080/19490976.2024.2393756
Clemente, J. C., Ursell, L. K., Parfrey, L. W., Knight, R. (2012). The impact of the gut microbiota on human health: an integrative view. Cell 148, 1258–1270. doi: 10.1016/j.cell.2012.01.035
D’Elios, S., Trambusti, I., Verduci, E., Ferrante, G., Rosati, S., Marseglia, G. L., et al. (2020). Probiotics in the prevention and treatment of atopic dermatitis. Pediatr. Allergy Immunol. 31 Suppl 26, 43–45. doi: 10.1111/pai.v31.s26
Den Besten, G., Van Eunen, K., Groen, A. K., Venema, K., Reijngoud, D.-J., Bakker, B. M. (2013). The role of short-chain fatty acids in the interplay between diet, gut microbiota, and host energy metabolism. J. Lipid Res. 54, 2325–2340. doi: 10.1194/jlr.R036012
Derrien, M., van Hylckama Vlieg, J. E. (2015). Fate, activity, and impact of ingested bacteria within the human gut microbiota. Trends Microbiol. 23, 354–366. doi: 10.1016/j.tim.2015.03.002
Ecklu-Mensah, G., Choo-Kang, C., Maseng, M. G., Donato, S., Bovet, P., Viswanathan, B., et al. (2023). Gut microbiota and fecal short chain fatty acids differ with adiposity and country of origin: the METS-microbiome study. Nat. Commun. 14, 5160. doi: 10.1038/s41467-023-40874-x
Fouladi, F., Carroll, I. M., Sharpton, T. J., Bulik-Sullivan, E., Heinberg, L., Steffen, K. J., et al. (2021). A microbial signature following bariatric surgery is robustly consistent across multiple cohorts. Gut Microbes 13, 1930872. doi: 10.1080/19490976.2021.1930872
Furet, J. P., Kong, L. C., Tap, J., Poitou, C., Basdevant, A., Bouillot, J. L., et al. (2010). Differential adaptation of human gut microbiota to bariatric surgery-induced weight loss: links with metabolic and low-grade inflammation markers. Diabetes 59, 3049–3057. doi: 10.2337/db10-0253
Gomez Del Pulgar, E. M., Benitez-Paez, A., Sanz, Y. (2020). Safety assessment of bacteroides uniformis CECT 7771, a symbiont of the gut microbiota in infants. Nutrients 12(2):551. doi: 10.3390/nu12020551
Gurung, M., Li, Z., You, H., Rodrigues, R., Jump, D. B., Morgun, A., et al. (2020). Role of gut microbiota in type 2 diabetes pathophysiology. EBioMedicine 51:102590. doi: 10.1016/j.ebiom.2019.11.051
Henneke, L., Schlicht, K., Andreani, N. A., Hollstein, T., Demetrowitsch, T., Knappe, C., et al. (2022). A dietary carbohydrate–gut Parasutterella–human fatty acid biosynthesis metabolic axis in obesity and type 2 diabetes. Gut Microbes 14, 2057778. doi: 10.1080/19490976.2022.2057778
Jing, C., Chen, H., Liang, Y., Zhong, Y., Wang, Q., Li, L., et al. (2021). Clinical evaluation of an improved metagenomic next-generation sequencing test for the diagnosis of bloodstream infections. Clin. Chem. 67, 1133–1143. doi: 10.1093/clinchem/hvab061
Joseph, T. A., Chlenski, P., Litman, A., Korem, T., Pe’er, I. (2022a). Accurate and robust inference of microbial growth dynamics from metagenomic sequencing reveals personalized growth rates. Genome Res. 32, 558–568. doi: 10.1101/gr.275533.121
Joseph, T. A., Chlenski, P., Litman, A., Korem, T., Pe’er, I. (2022b). Accurate and robust inference of microbial growth dynamics from metagenomic sequencing reveals personalized growth rates. Genome Res. 32, 558–568. doi: 10.1101/gr.275533.121
Le Barz, M., Anhe, F. F., Varin, T. V., Desjardins, Y., Levy, E., Roy, D., et al. (2015). Probiotics as complementary treatment for metabolic disorders. Diabetes Metab. J. 39, 291–303. doi: 10.4093/dmj.2015.39.4.291
Lee, C. J., Florea, L., Sears, C. L., Maruthur, N., Potter, J. J., Schweitzer, M., et al. (2019). Changes in gut microbiome after bariatric surgery versus medical weight loss in a pilot randomized trial. Obes. Surg. 29, 3239–3245. doi: 10.1007/s11695-019-03976-4
Lee, Y. K., Mazmanian, S. K. (2010). Has the microbiota played a critical role in the evolution of the adaptive immune system? Science 330, 1768–1773. doi: 10.1126/science.1195568
Liu, R., Hong, J., Xu, X., Feng, Q., Zhang, D., Gu, Y., et al. (2017). Gut microbiome and serum metabolome alterations in obesity and after weight-loss intervention. Nat. Med. 23, 859–868. doi: 10.1038/nm.4358
Mingrone, G., Panunzi, S., De Gaetano, A., Guidone, C., Iaconelli, A., Leccesi, L., et al. (2012). Bariatric surgery versus conventional medical therapy for type 2 diabetes. New Engl. J. Med. 366, 1577–1585. doi: 10.1056/NEJMoa1200111
Murphy, R., Tsai, P., Jullig, M., Liu, A., Plank, L., Booth, M. (2017). Differential changes in gut microbiota after gastric bypass and sleeve gastrectomy bariatric surgery vary according to diabetes remission. Obes. Surg. 27, 917–925. doi: 10.1007/s11695-016-2399-2
Nearing, J. T., Douglas, G. M., Hayes, M. G., MacDonald, J., Desai, D. K., Allward, N., et al. (2022). Microbiome differential abundance methods produce different results across 38 datasets. Nat. Commun. 13, 342. doi: 10.1038/s41467-022-28034-z
Nie, X., Chen, J., Ma, X., Ni, Y., Shen, Y., Yu, H., et al. (2020). A metagenome-wide association study of gut microbiome and visceral fat accumulation. Comput. Struct. Biotechnol. J. 18, 2596–2609. doi: 10.1016/j.csbj.2020.09.026
O’Toole, P. W., Marchesi, J. R., Hill, C. (2017). Next-generation probiotics: the spectrum from probiotics to live biotherapeutics. Nat. Microbiol. 2, 17057. doi: 10.1038/nmicrobiol.2017.57
Paganelli, F. L., Luyer, M., Hazelbag, C. M., Uh, H.-W., Rogers, M. R., Adriaans, D., et al. (2019). Roux-Y gastric bypass and sleeve gastrectomy directly change gut microbiota composition independent of surgery type. Sci. Rep. 9, 10979. doi: 10.1038/s41598-019-47332-z
Palleja, A., Kashani, A., Allin, K. H., Nielsen, T., Zhang, C., Li, Y., et al. (2016). Roux-en-Y gastric bypass surgery of morbidly obese patients induces swift and persistent changes of the individual gut microbiota. Genome Med. 8, 67. doi: 10.1186/s13073-016-0312-1
Qin, J., Li, Y., Cai, Z., Li, S., Zhu, J., Zhang, F., et al. (2012). A metagenome-wide association study of gut microbiota in type 2 diabetes. Nature 490, 55–60. doi: 10.1038/nature11450
Saheb Kashaf, S., Proctor, D. M., Deming, C., Saary, P., Holzer, M., Program, N. C. S., et al. (2022). Integrating cultivation and metagenomics for a multi-kingdom view of skin microbiome diversity and functions. Nat. Microbiol. 7, 169–179. doi: 10.1038/s41564-021-01011-w
Schauer, P. R., Bhatt, D. L., Kirwan, J. P., Wolski, K., Aminian, A., Brethauer, S. A., et al. (2017). Bariatric surgery versus intensive medical therapy for diabetes - 5-year outcomes. New Engl. J. Med. 376, 641–651. doi: 10.1056/NEJMoa1600869
Schwiertz, A., Taras, D., Schafer, K., Beijer, S., Bos, N. A., Donus, C., et al. (2010). Microbiota and SCFA in lean and overweight healthy subjects. Obesity 18, 190–195. doi: 10.1038/oby.2009.167
Sola-Leyva, A., Andres-Leon, E., Molina, N. M., Terron-Camero, L. C., Plaza-Diaz, J., Saez-Lara, M. J., et al. (2021). Mapping the entire functionally active endometrial microbiota. Hum. Reprod. 36, 1021–1031. doi: 10.1093/humrep/deaa372
Suez, J., Zmora, N., Segal, E., Elinav, E. (2019). The pros, cons, and many unknowns of probiotics. Nat. Med. 25, 716–729. doi: 10.1038/s41591-019-0439-x
Turnbaugh, P. J., Ley, R. E., Mahowald, M. A., Magrini, V., Mardis, E. R., Gordon, J. I. (2006). An obesity-associated gut microbiome with increased capacity for energy harvest. Nature 444, 1027–1031. doi: 10.1038/nature05414
Van Hul, M., Cani, P. D. (2023). The gut microbiota in obesity and weight management: microbes as friends or foe? Nat. Rev. Endocrinol. 19, 258–271. doi: 10.1038/s41574-022-00794-0
Wang, Z., Yang, Y., Yan, Z., Liu, H., Chen, B., Liang, Z., et al. (2020). Multi-omic meta-analysis identifies functional signatures of airway microbiome in chronic obstructive pulmonary disease. ISME J. 14, 2748–2765. doi: 10.1038/s41396-020-0727-y
Wood, D. E., Lu, J., Langmead, B. (2019). Improved metagenomic analysis with Kraken 2. Genome Biol. 20, 1–13. doi: 10.1186/s13059-019-1891-0
Wu, S., Feng, T., Tang, W., Qi, C., Gao, J., He, X., et al. (2024). metaProbiotics: a tool for mining probiotic from metagenomic binning data based on a language model. Briefings Bioinf. 25(2), bbae085. doi: 10.1093/bib/bbae085
Xu, Y., Wang, N., Tan, H. Y., Li, S., Zhang, C., Feng, Y. (2020). Function of Akkermansia muciniphila in obesity: interactions with lipid metabolism, immune response and gut systems. Front. Microbiol. 11, 219. doi: 10.3389/fmicb.2020.00219
Zeng, S., Patangia, D., Almeida, A., Zhou, Z., Mu, D., Paul Ross, R., et al. (2022). A compendium of 32,277 metagenome-assembled genomes and over 80 million genes from the early-life human gut microbiome. Nat. Commun. 13, 5139. doi: 10.1038/s41467-022-32805-z
Zhang, H., DiBaise, J. K., Zuccolo, A., Kudrna, D., Braidotti, M., Yu, Y., et al. (2009). Human gut microbiota in obesity and after gastric bypass. Proceedings of the National Academy of Sciences of the United States of America. 106(7), 2365–2370. doi: 10.1073/pnas.0812600106
Keywords: obesity, bariatric surgeries, gut microbiota, MAGs, fatty acid biosynthesis
Citation: Shi H and Li J (2025) MAGs-based genomic comparison of gut significantly enriched microbes in obese individuals pre- and post-bariatric surgery across diverse locations. Front. Cell. Infect. Microbiol. 15:1485048. doi: 10.3389/fcimb.2025.1485048
Received: 23 August 2024; Accepted: 27 February 2025;
Published: 18 March 2025.
Edited by:
Jaime Garcia-Mena, National Polytechnic Institute of Mexico (CINVESTAV), MexicoReviewed by:
James Butcher, University of Ottawa, CanadaCopyright © 2025 Shi and Li. This is an open-access article distributed under the terms of the Creative Commons Attribution License (CC BY). The use, distribution or reproduction in other forums is permitted, provided the original author(s) and the copyright owner(s) are credited and that the original publication in this journal is cited, in accordance with accepted academic practice. No use, distribution or reproduction is permitted which does not comply with these terms.
*Correspondence: Jia Li, bGlqaWE4MjAzMjNAMTYzLmNvbQ==
Disclaimer: All claims expressed in this article are solely those of the authors and do not necessarily represent those of their affiliated organizations, or those of the publisher, the editors and the reviewers. Any product that may be evaluated in this article or claim that may be made by its manufacturer is not guaranteed or endorsed by the publisher.
Research integrity at Frontiers
Learn more about the work of our research integrity team to safeguard the quality of each article we publish.